Abstract
Static hemodynamic or neuroelectric images of brain regions activated during particular tasks do not convey the information of how these regions communicate to each other. Cortical connectivity estimation aims at describing these interactions as connectivity patterns which hold the direction and strength of the information flow between cortical areas. In this study, we attempted to estimate the causality between distributed cortical systems during a movement volition task in preparation for execution of simple movements by a group of normal healthy subjects and by a group of Spinal Cord Injured (SCI) patients. To estimate the causality between the spatial distributed patterns of cortical activity in the frequency domain, we applied a series of processing steps on the recorded EEG data. From the high-resolution EEG recordings we estimated the cortical waveforms for the regions of interest (ROIs), each representing a selected sensor group population. The solutions of the linear inverse problem returned a series of cortical waveforms for each ROI considered and for each trial analyzed. For each subject, the cortical waveforms were then subjected to Independent Component Analysis (ICA) pre-processing. The independent components obtained by the application of the ThinICA algorithm were further processed by a Partial Directed Coherence algorithm, in order to extract the causality between spatial cortical patterns of the estimated data. The source-target cortical dependencies found in the group of normal subjects were relatively similar in all frequency bands analyzed. For the normal subjects we observed a common source pattern in an ensemble of cortical areas including the right parietal and right lip primary motor areas and bilaterally the primary foot and posterior SMA areas. The target of this cortical network, in the Granger-sense of causality, was shown to be a smaller network composed mostly by the primary foot motor areas and the posterior SMA bilaterally. In the case of the SCI population, both the source and the target cortical patterns had larger sizes than in the normal population. The source cortical areas included always the primary foot and lip motor areas, often bilaterally. In addition, the right parietal area and the bilateral premotor area 6 were also involved. Again, the patterns remained substantially stable across the different frequency bands analyzed. The target cortical patterns observed in the SCI population had larger extensions when compared to the normal ones, since in most cases they involved the bilateral activation of the primary foot movement areas as well as the SMA, the primary lip areas and the parietal cortical areas.
Similar content being viewed by others
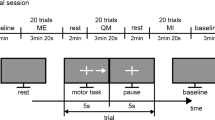
References
Akaike H. A new look at statistical model identification. IEEE Trans Automat Control AC, 1974;19:716–23.
Astolfi L, Cincotti F, Babiloni C, Carducci F, Basilisco A, Rossini PM, Salinari S, Mattia D, Cerutti S, Ben Dayan D, Ding L, Ni Y, He B, Babiloni F Estimation of the cortical connectivity by high resolution EEG and structural equation modeling: simulations and application to finger tapping data. IEEE Trans Biomed Eng 2005a;52(5):757–68.
Astolfi L, Cincotti F, Mattia D, Babiloni C, Carducci F, Basilisco A, Rossini PM, Salinari S, Ding L, Ni Y, He B, Babiloni F. Assessing cortical functional connectivity by linear inverse estimation and directed transfer function: simulations and application to real data. Clin Neurophysiol 2005b;116(4):920–32.
Astolfi L, Cincotti F, Mattia D, Marciani MG, Baccala LA, de Vico Fallani F, Salinari S, Ursino M, Zavaglia M, Babiloni F. Assessing cortical functional connectivity by partial directed coherence: simulations and application to real data. IEEE Trans Biomed Eng 2006;53(9):1802–12.
Babiloni F, Babiloni C, Carducci F, Romani GL, Rossini PM, Angelone LM, Cincotti F. Multimodal integration of EEG and MEG data: a simulation study with variable signal-to-noise ratio and number of sensors. Hum Brain Mapp 2004;22(1):52–62.
Babiloni F, Babiloni C, Carducci F, Romani GL, Rossini PM, Angelone LM, Cincotti F. Multimodal integration of high-resolution EEG and functional magnetic resonance imaging data: a simulation study. Neuroimage 2003;19(1):1–15.
Babiloni F, Babiloni C, Locche L, Cincotti F, Rossini PM, Carducci F. High-resolution electroencephalogram: source estimates of Laplacian-transformed somatosensory-evoked potentials using a realistic subject head model constructed from magnetic resonance images. Med Biol Eng Comput 2000;38(5):512–9.
Babiloni F, Cincotti F, Babiloni C, Carducci F, Basilisco A, Rossini PM, Mattia D, Astolfi L, Ding L, Ni Y, Cheng K, Christine K, Sweeney J, He B. Estimation of the cortical functional connectivity with the multimodal integration of high resolution EEG and fMRI data by Directed Transfer Function. Neuroimage 2005;24(1):118–31.
Babiloni F, Carducci F, Cincotti F, Del Gratta C, Pizzella V, Romani GL, Rossini PM, Tecchio F, Babiloni C. Linear inverse source estimate of combined EEG and MEG data related to voluntary movements. Human Brain Mapp 2001;14(3).
Baccalà LA. On the efficient computation of partial coherence from multivariate autoregressive model. In: Callaos N, Rosario D, Sanches B editors. Proceedings of the 5th world conference cybernect is systemics and informatics SCI 2001, Orlando; 2001.
Baccalà LA, Sameshima K. Partial directed coherence: a new concept in neural structure determination. Biol Cybern 2001;84:463–74.
Bakardjian H. Common independent components for motion-based brain-computer-interfaces. In: Proc. of the society for NeuroScience meeting, San Diego, USA, Oct 23–27; 2004.
Brovelli A, Battaglini PP, Naranjo JR, Budai R. Medium-range oscillatory network and the 20-Hz sensorimotor induced potential. Neuroimage 2002;16(1):130–41.
Brovelli A, Ding M, Ledberg A, Chen Y, Nakamura R, Bressler SL. Beta oscillations in a large-scale sensorimotor cortical network: directional influences revealed by Granger causality. Proc Natl Acad Sci USA 2004;101(26):9849–54.
Buchel C, Friston KJ (1997) Modulation of connectivity in visual pathways by attention: cortical interactions evaluated with structural equation modeling and fMRI. Cereb Cortex 7(8):768–78.
Cichocki A, Amari S. Adaptive blind signal and image processing: learning algorithms and applications. West Sussex, UK: John Wiley & Sons; 2003.
Clifford Carter G. Coherence and time delay estimation. Proc IEEE 1987;75:236–55.
Cruces-Alvarez S, Cichocki A. Combining blind source extraction with joint approximate diagonalization: thin algorithms for ICA. In: Proceedings of 4th international symposium on independent component analysis and blind signal separation (ICA2003), Kyoto, Japan, Riken, ICA; Apr., 2003, p. 463–8.
Cruces-Alvarez S, Cichocki A, De Lathauwer L. Thin QR and SVD factorizations for simultaneous blind signal extraction. In: Proceedings of the European Signal Processing Conference (EUSIPCO), Vienna, Austria; 2004, p. 217–220, ISBN: 3-200-00165-8.
David O, Cosmelli D, Friston KJ. Evaluation of different measures of functional connectivity using a neural mass model. Neuroimage 2004;21(2):659–73.
Ding M, Bressler SL, Yang W, Liang H. Short-window spectral analysis of cortical event related potentials by adaptive multivariate autoregressive modeling: data preprocessing, model validation and variability assessment. Biol Cybern 2000;83:35–45.
Franaszczuk PJ, Blinowska KJ, Kowalczyk M. The application of parametric multichannel spectral estimates in the study of electrical brain activity. Biol Cybern 1985;51:239–47.
Friston KJ. Functional and effective connectivity in neuroimaging: a synthesis. Human Brain Mapp 1994;2:56–78.
Gerloff C, Richard J, Hadley J, Schulman AE, Honda M, Hallett M. Functional coupling and regional activation of human cortical motor areas during simple, internally paced and externally paced finger movements. Brain 1998;121(Pt 8):1513–31.
Gevins AS, Cutillo BA, Bressler SL, Morgan NH, White RM, Illes J, Greer DS. Event-related covariances during a bimanual visuomotor task. II. Preparation and feedback. Electroencephalogr Clin Neurophysiol 1989;74:147–60.
Granger CWJ. Investigating causal relations by econometric models and cross-spectral methods. Econometrica 1969;37: 424–38.
Grave de Peralta Menendez R, Gonzalez Andino SL. Distributed source models: standard solutions and new developments. In: Uhl C, editor. Analysis of neurophysiological brain functioning. Springer Verlag; 1999. p. 176–201.
Gross J, Kujala J, Hämäläinen M, Timmermann L, Schnitzler A, Salmelin R. Dynamic imaging of coherent sources: studying neural interactions in the human brain. Proc Natl Acad Sci USA 2001;98(2):694–99.
Gross J, Timmermann L, Kujala J, Salmelin R, Schnitzler A. Properties of MEG tomographic maps obtained with spatial filtering. NeuroImage 2003;19:1329–36.
Hansen PC. Analysis of discrete ill-posed problems by means of the L-curve. SIAM Rev 1992a;34:561–80.
Hansen PC. Numerical tools for the analysis and solution of Fredholm integral equations of the first kind. Inverse Prob 1992b;8:849–72.
He B, Lian J. Spatio-temporal functional neuroimaging of brain electric activity. Crit Rev Biomed Eng 2002;30:283–306.
Horwitz B. The elusive concept of brain connectivity. Neuroimage 2003;19(2Pt1):466–70.
Inouye T, Iyama A, Shinosaki K, Toi S, Matsumoto Y. Inter-site EEG relationships before widespread epileptiform discharges. Int J Neurosci 1995;82:143–53.
Jancke L, Loose R, Lutz K, Specht K, Shah NJ. Cortical activations during paced finger-tapping applying visual and auditory pacing stimuli. Brain Res Cogn Brain Res 2000;10(1–2):51–66.
Kaminski M, Ding M, Truccolo WA, Bressler S. Evaluating causal relations in neural systems: Granger causality, directed transfer function and statistical assessment of significance. Biol Cybern 2001;85:145–57.
Korzeniewska A, Manczak M, Kaminski M, Blinowska K, Kasicki S. Determination of information flow direction between brain structures by a modified Directed Transfer Function method (dDTF). J Neurosci Methods 2003;125:195–207.
Lee L, Harrison LM, Mechelli A. The functional brain connectivity workshop: report and commentary. Neuroimage 2003;19:457–465.
Miwakeichi F, Martinez-Montes E, Valdes-Sosa PA, Nishiyama N, Mizuhara H, Yamaguchi Y. Decomposing EEG data into space-time-frequency components using parallel factor analysis. NeuroImage 2004;22:1035–45.
Mizuhara H, Wang L, Kobayashi K, Yamaguchi Y. Long-range EEG phase synchronization during an arithmetic task indexes a coherent cortical network simultaneously measured by fMRI. NeuroImage 2005;27(3):553–63.
Nunez PL. Neocortical dynamics and human EEG rhythms. Oxford University Press, New York; 1995.
Pascual-Marqui RD. Reply to comments by Hama lainen, Ilmoniemi and Nunez. In: Skrandies W, editor. ISBET Newsletter No. 6; December, 1995. p. 16–28.
Pfurtscheller G, Lopes da Silva FH. Event-related EEG/MEG synchronization and desynchronization: basic principles. Clin Neurophysiol 1999;110(11):1842–57.
Picard N, Strick PL. Imaging the premotor areas. Curr Opin Neurobiol 1996;11(6):663–72.
Pollok B, Gross J, Muller K, Aschersleben G, Schnitzler A. The cerebral oscillatory network associated with auditorily paced finger movements. NeuroImage 2005a;24:646–55.
Pollok B, Gross J, Muller K, Aschersleben G, Schnitzler A. The oscillatory network of simple repetitive bimanual movements. Cogn Brain Res 2005b;25:300–11.
Quian Quiroga R, Kraskov A, Kreuz T, Grassberger P. Performance of different synchronization measures in real data: a case study on electroencephalographic signals. Phys Rev;2002 E 65p., 041903.
Rosenblum MG, Pikovsky AS. Detecting direction of coupling in interacting oscillators. Phys Rev, E Stat. Nonlinear Soft Matter Phys 2001;64:045202.
Stam CJ, Breakspear M, van Cappellen, van Walsum AM, van Dijk BW. Nonlinear synchronization in EEG and whole head MEG recordings of healthy subjects. Hum Brain Mapp 2003;19(2):63–78.
Stam CJ, van Dijk BW. Synchronization likelihood: an unbiased measure of generalized synchronization in multivariate data sets. Physica D 2002;163: 236–51.
Taniguchi M, Kato A, Fujita N, Hirata M, Tanaka H, Kihara T, Ninomiya H, Hirabuki N, Nakamura H, Robinson SE, Cheyne D, Yoshimine T. Movement-related desynchronization of the cerebral cortex studied with spatially filtered magnetoencephalography. Neuroimage 2000;12(3):298–306.
Tononi G, Sporns O, Edelman GM. A measure for brain complexity: relating functional segregation and integration in the nervous system. Proc Natl Acad Sci USA 1994;91: 5033–7.
Urbano A, Babiloni C, Onorati P, Babiloni F. Dynamic functional coupling of high resolution EEG potentials related to unilateral internally triggered one-digit movements. Electroencephalogr Clin Neurophysiol 1998;106(6):477–87.
Acknowledgment
This work was supported by a grant of RIKEN Institute for the years 2005–2007
Author information
Authors and Affiliations
Corresponding author
Rights and permissions
About this article
Cite this article
Astolfi, L., Bakardjian, H., Cincotti, F. et al. Estimate of Causality Between Independent Cortical Spatial Patterns During Movement Volition in Spinal Cord Injured Patients. Brain Topogr 19, 107–123 (2007). https://doi.org/10.1007/s10548-007-0018-1
Accepted:
Published:
Issue Date:
DOI: https://doi.org/10.1007/s10548-007-0018-1