Abstract
We consider a family of rich vehicle routing problems (RVRP) which have the particularity to combine a heterogeneous fleet with other attributes, such as backhauls, multiple depots, split deliveries, site dependency, open routes, duration limits, and time windows. To efficiently solve these problems, we propose a hybrid metaheuristic which combines an iterated local search with variable neighborhood descent, for solution improvement, and a set partitioning formulation, to exploit the memory of the past search. Moreover, we investigate a class of combined neighborhoods which jointly modify the sequences of visits and perform either heuristic or optimal reassignments of vehicles to routes. To the best of our knowledge, this is the first unified approach for a large class of heterogeneous fleet RVRPs, capable of solving more than 12 problem variants. The efficiency of the algorithm is evaluated on 643 well-known benchmark instances, and 71.70% of the best known solutions are either retrieved or improved. Moreover, the proposed metaheuristic, which can be considered as a matheuristic, produces high quality solutions with low standard deviation in comparison with previous methods. Finally, we observe that the use of combined neighborhoods does not lead to significant quality gains. Contrary to intuition, the computational effort seems better spent on more intensive route optimization rather than on more intelligent and frequent fleet re-assignments.

Similar content being viewed by others
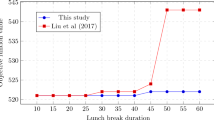
References
Amorim, P., Parragh, S., Sperandio, F., & Almada-Lobo, B. (2014). A rich vehicle routing problem dealing with perishable food: A case study. TOP, 22(2), 489–508.
Baldacci, R., Battarra, M., & Vigo, D. (2008). The vehicle routing problem: Latest advances and new challenges. Chap. Routing a heterogeneous fleet of vehicles (pp. 11–35). Berlin: Springer.
Baldacci, R., Battarra, M., & Vigo, D. (2009). Valid inequalities for the fleet size and mix vehicle routing problem with fixed costs. Networks, 54(4), 178–189.
Baldacci, R., Bartolini, E., Mingozzi, A., & Roberti, R. (2010a). An exact solution framework for a broad class of vehicle routing problems. Computational Management Science, 7(3), 229–268.
Baldacci, R., Toth, P., & Vigo, D. (2010b). Exact algorithms for routing problems under vehicle capacity constraints. Annals of Operations Research, 175(1), 213–245.
Belfiore, P., & Yoshizaki, H. T. Y. (2009). Scatter search for a real-life heterogeneous fleet vehicle routing problem with time windows and split deliveries in Brazil. European Journal of Operational Research, 199, 750–758.
Belmecheri, F., Prins, C., Yalaoui, F., & Amodeo, L. (2013). Particle swarm optimization algorithm for a vehicle routing problem with heterogeneous fleet, mixed backhauls, and time windows. Journal of Intelligent Manufacturing, 24(4), 775–789.
Berghida, M., & Boukra, A. (2015). EBBO: An enhanced biogeography-based optimization algorithm for a vehicle routing problem with heterogeneous fleet, mixed backhauls, and time windows. The International Journal of Advanced Manufacturing Technology, 77(9–12), 1711–1725.
Bettinelli, A., Ceselli, A., & Righini, G. (2011). A branch-and-cut-and-price algorithm for the multi-depot heterogeneous vehicle routing problem with time windows. Transportation Research Part C: Emerging Technologies, 19(5), 723–740.
Blum, C., & Roli, A. (2003). Metaheuristics in combinatorial optimization: Overview and conceptual comparison. ACM Computing Surveys (CSUR), 35(3), 268–308.
Boudia, M., Prins, C., & Reghioui, M. (2007). An effective memetic algorithm with population management for the split delivery vehicle routing problem. In T. Bartz-Beielstein, M. Blesa Aguilera, C. Blum, B. Naujoks, A. Roli, G. Rudolph, & M. Sampels (Eds.), Hybrid metaheuristics. Lecture notes in computer science (Vol. 4771, pp. 16–30). Berlin: Springer.
Brandão, J. (2009). A deterministic tabu search algorithm for the fleet size and mix vehicle routing problem. European Journal of Operational Research, 195, 716–728.
Brandão, J. (2011). A tabu search algorithm for the heterogeneous fixed fleet vehicle routing problem. Computers & Operations Research, 38, 140–151.
Bräysy, O., Dullaert, W., Hasle, G., Mester, D., & Gendreau, M. (2008). An effective multirestart deterministic annealing metaheuristic for the fleet size and mix vehicle-routing problem with time windows. Transportation Science, 42(3), 371–386.
Bräysy, O., Porkka, P. P., Dullaert, W., Repoussis, P. P., & Tarantilis, C. D. (2009). A well-scalable metaheuristic for the fleet size and mix vehicle routing problem with time windows. Expert Systems with Applications, 36, 8460–8475.
Cáceres-Cruz, J., Arias, P., Guimarans, D., Riera, D., & Juan, A. A. (2014a). Rich vehicle routing problem: Survey. ACM Computing Surveys, 47(2), 1–28.
Cáceres-Cruz, J., Grasas, A., Ramalhinho, H., & Juan, A. A. (2014b). A savings-based randomized heuristic for the heterogeneous fixed fleet vehicle routing problem with multi-trips. Journal of Applied Operational Research, 6(2), 69–81.
Cáceres-Cruz, J., Riera, D., Buil, R., & Juan, A. A. (2013). Applying a savings algorithm for solving a rich vehicle routing problem in a real urban context. In 5th International conference on applied operational research. Lecture notes in management science (Vol. 5, pp. 84–92).
Ceselli, A., Righini, G., & Salani, M. (2009). A column generation algorithm for a rich vehicle-routing problem. Transportation Science, 43(1), 56–69.
Choi, E., & Tcha, D. W. (2007). A column generation approach to the heterogeneous fleet vehicle routing problem. Computers & Operations Research, 34, 2080–2095.
Clarke, G., & Wright, J. W. (1964). Scheduling of vehicles from a central depot to a number of delivery points. Operations Research, 12, 568–581.
Cordeau, J. F., & Laporte, G. (2001). A tabu search algorithm for the site dependent vehicle routing problem with time windows. INFOR, 39, 292–8.
Cordeau, J. F., Laporte, G., & Mercier, A. (2001). A unified tabu search heuristic for vehicle routing problems with time windows. Journal of the Operational Research Society, 52, 928–936.
Cordeau, J. F., Laporte, G., & Mercier, A. (2004). Improved tabu search algorithm for the handling of route duration constraints in vehicle routing problems with time windows. The Journal of the Operational Research Society, 55(5), 542–546.
Cordeau, J. F., & Maischberger, M. (2012). A parallel iterated tabu search heuristic for vehicle routing problems. Computers & Operations Research, 39(9), 2033–2050.
Dantzig, G. B., & Ramser, J. H. (1959). The truck dispatching problem. Management Science, 6, 80–91.
de Armas, J., & Melián-Batista, B. (2015). Variable neighborhood search for a dynamic rich vehicle routing problem with time windows. Computers & Industrial Engineering, 85, 120–131.
de Armas, J., Melián-Batista, B., Moreno-Pérez, J. A., & Brito, J. (2015). GVNS for a real-world rich vehicle routing problem with time windows. Engineering Applications of Artificial Intelligence, 42, 45–56.
Dell’Amico, M., Monaci, M., Pagani, C., & Vigo, D. (2007). Heuristic approaches for the fleet size and mix vehicle routing problem with time windows. Transportation Science, 41(4), 516–526.
Derigs, U., & Vogel, U. (2014). Experience with a framework for developing heuristics for solving rich vehicle routing problems. Journal of Heuristics, 20(1), 75–106.
Dominguez, O., Juan, A. A., Barrios, B., Faulin, J., & Agustin, A. (2016). Using biased randomization for solving the two-dimensional loading vehicle routing problem with heterogeneous fleet. Annals of Operations Research, 236(2), 383–404.
Dondo, R., & Cerdá, J. (2007). A cluster-based optimization approach for the multi-depot heterogeneous fleet vehicle routing problem with time windows. European Journal of Operational Research, 176(3), 1478–1507.
Dongarra, J. J. (2010). Performance of various computers using standard linear equations software. Technical Report CS-89-85, Computer Science Department, University of Tennessee
Dror, M., & Trudeau, P. (1990). Split delivery routing. Naval Research Logistics, 37(3), 383–402.
Duhamel, C., Gouinaud, C., Lacomme, P., & Prodhon, C. (2013). A multi-thread GRASPxELS for the heterogeneous capacitated vehicle routing problem. In E. G. Talbi (Ed.), Hybrid metaheuristics. Studies in computational intelligence (Vol. 434, pp. 237–269). Berlin: Springer.
Duhamel, C., Lacomme, P., & Prodhon, C. (2011). Efficient frameworks for greedy split and new depth first search split procedures for routing problems. Computers & Operations Research, 38(4), 723–739.
Gendreau, M., Laporte, G., Musaraganyi, C., & Taillard, E. D. (1999). A tabu search heuristic for the heterogeneous fleet vehicle routing problem. Computers & Operations Research, 26, 1153–1173.
Goel, A. (2010). A column generation heuristic for the general vehicle routing problem. In C. Blum & R. Battiti (Eds.), Learning and intelligent optimization. Lecture notes in computer science (Vol. 6073, pp. 1–9). Berlin: Springer.
Goel, A., & Gruhn, V. (2008). A general vehicle routing problem. European Journal of Operational Research, 191(3), 650–660.
Golden, B. L., Assad, A. A., Levy, L., & Gheysens, F. G. (1984). The fleet size and mix vehicle routing problem. Computers & Operations Research, 11, 49–66.
Hansen, P., Mladenović, N., & Pérez, J. M. (2010). Variable neighbourhood search: Methods and applications. Annals of Operations Research, 175, 367–407.
Hoff, A., Andersson, H., Christiansen, M., Hasle, G., & Løkketangen, A. (2010). Industrial aspects and literature survey: Fleet composition and routing. Computers & Operations Research, 37, 2041–2061.
Imran, A., Salhi, S., & Wassan, N. A. (2009). A variable neighborhood-based heuristic for the heterogeneous fleet vehicle routing problem. European Journal of Operational Research, 197, 509–518.
Irnich, S., Schneider, M., & Vigo, D. (2014) Vehicle routing: Problems, methods, and applications, chap Four variants of the vehicle routing problem (pp. 241–271). MOS-SIAM series on optimization.
Koç, Ç., Bektaş, T., Jabali, O., & Laporte, G. (2015). A hybrid evolutionary algorithm for heterogeneous fleet vehicle routing problems with time windows. Computers & Operations Research, 64, 11–27.
Koç, Ç., Bektaş, T., Jabali, O., & Laporte, G. (2016). Thirty years of heterogeneous vehicle routing. European Journal of Operational Research, 249(1), 1–21.
Lahyani, R., Khemakhem, M., & Semet, F. (2015). Rich vehicle routing problems: From a taxonomy to a definition. European Journal of Operational Research, 241(1), 1–14.
Lee, Y., Kim, J., Kang, K., & Kim, K. (2008). A heuristic for vehicle fleet mix problem using tabu search and set partitioning. Journal of the Operational Research Society, 59, 833–841.
Li, F., Golden, B., & Wasil, E. (2007). A record-to-record travel algorithm for solving the heterogeneous fleet vehicle routing problem. Computers & Operations Research, 34, 2734–2742.
Li, X., Leung, S. C., & Tian, P. (2012). A multistart adaptive memory-based tabu search algorithm for the heterogeneous fixed fleet open vehicle routing problem. Expert Systems with Applications, 39, 365–374.
Li, X., Tian, P., & Aneja, Y. (2010). An adaptive memory programming metaheuristic for the heterogeneous fixed fleet vehicle routing problem. Transportation Research Part E: Logistics and Transportation Review, 46(6), 1111–1127.
Lima, C. M. R. R., Goldbarg, M. C., & Goldbarg, E. F. G. (2004). A memetic algorithm for the heterogeneous fleet vehicle routing problem. Electronic Notes in Discrete Mathematics, 18, 171–176.
Liu, F. H., & Shen, S. Y. (1999). The fleet size and mix vehicle routing problem with time windows. The Journal of the Operational Research Society, 50(7), 721–732.
Liu, S., Huang, W., & Ma, H. (2009). An effective genetic algorithm for the fleet size and mix vehicle routing problems. Transportation Research Part E, 45, 434–445.
Lourenço, H. R., Martin, O. C., & Stützle, T. (2010). Iterated local search: Framework and applications. In M. Gendreau & J. Y. Potvin (Eds.), Handbook of metaheuristics. International series in operations research & management science (Vol. 146, pp. 363–397). New York: Springer.
Mancini, S. (2016). A real-life multi depot multi period vehicle routing problem with a heterogeneous fleet: Formulation and adaptive large neighborhood search based matheuristic. Transportation Research Part C: Emerging Technologies, 70, 100–112.
Mar-Ortiz, J., González-Velarde, J., & Adenso-Díaz, B. (2013). Designing routes for weee collection: The vehicle routing problem with split loads and date windows. Journal of Heuristics, 19(2), 103–127.
McGinnis, L. F. (1983). Implementation and testing of a primal–dual algorithm for the assignment problem. Operations Research, 31(2), 277–291.
Nagata, Y., Bräysy, O., & Dullaert, W. (2010). A penalty-based edge assembly memetic algorithm for the vehicle routing problem with time windows. Computers & Operations Research, 37(4), 724–737.
Ochi, L., Vianna, D., Drummond, L. M. A., & Victor, A. (1998a). An evolutionary hybrid metaheuristic for solving the vehicle routing problem with heterogeneous fleet. Lecture Notes in Computer Science, 1391, 187–195.
Ochi, L., Vianna, D., Drummond, L. M. A., & Victor, A. (1998b). A parallel evolutionary algorithm for the vehicle routing problem with heterogeneous fleet. Future Generation Computer Systems, 14, 285–292.
Ozfirat, P. M., & Ozkarahan, I. (2010). A constraint programming heuristic for a heterogeneous vehicle routing problem with split deliveries. Applied Artificial Intelligence, 24(4), 277–294.
Paraskevopoulos, D., Repoussis, P., Tarantilis, C., Ioannou, G., & Prastacos, G. (2008). A reactive variable neighborhood tabu search for the heterogeneous fleet vehicle routing problem with time windows. Journal of Heuristics, 14, 425–455.
Pellegrini, P., Favaretto, D., & Moretti, E. (2007). Multiple ant colony optimization for a rich vehicle routing problem: A case study. In B. Apolloni, R. Howlett, & L. Jain (Eds.), Knowledge-based intelligent information and engineering systems. Lecture notes in computer science (Vol. 4693, pp. 627–634). Berlin: Springer.
Penna, P. H. V., Subramanian, A., & Ochi, L. S. (2013). An iterated local search heuristic for the heterogeneous fleet vehicle routing problem. Journal of Heuristics, 19(2), 201–232.
Pessoa, A., Uchoa, E., & de Aragão, M. P. (2009). A robust branch-cut-and-price algorithm for the heterogeneous fleet vehicle routing problem. Networks, 54(4), 167–177.
Pisinger, D., & Røpke, S. (2007). A general heuristic for vehicle routing problems. Computers & Operations Research, 34(8), 2403–2435.
Prins, C. (2002). Efficient heuristics for the heterogeneous fleet multitrip VRP with application to a large-scale real case. Journal of Mathematical Modelling and Algorithms, 1, 135–150.
Prins, C. (2009). Two memetic algorithms for heterogeneous fleet vehicle routing problems. Engineering Applications of Artificial Intelligence, 22(6), 916–928.
Prins, C., Lacomme, P., & Prodhon, C. (2014). Order-first split-second methods for vehicle routing problems: A review. Transportation Research Part C, 40, 179–200.
Repoussis, P., & Tarantilis, C. (2010). Solving the fleet size and mix vehicle routing problem with time windows via adaptive memory programming. Transportation Research Part C: Emerging Technologies, 18(5), 695–712.
Reyes, L. C., Barbosa, J. G., Vargas, D. R., Huacuja, H. F., Valdez, N. R., Ortiz, J. H., Cruz, B. A., & Orta, J. D. (2007) A distributed metaheuristic for solving a real-world scheduling–routing–loading problem. In I. Stojmenovic, R. Thulasiram, L. Yang, W. Jia, M. Guo, R. de Mello (Eds.), Parallel and distributed processing and applications. Lecture notes in computer science (Vol. 4742, pp. 68 – 77).
Rieck, J., & Zimmermann, J. (2010). A new mixed integer linear model for a rich vehicle routing problem with docking constraints. Annals of Operations Research, 181(1), 337–358.
Rochat, Y., & Taillard, R. D. (1995). Probabilistic diversification and intensification in local search for vehicle routing. Journal of Heuristics, 1, 147–167.
Røpke, S., & Pisinger, D. (2006). A unified heuristic for a large class of vehicle routing problems with backhauls. European Journal of Operational Research, 171(3), 750–775.
Salhi, S., Imran, A., & Wassan, N. A. (2014). The multi-depot vehicle routing problem with heterogeneous vehicle fleet: Formulation and a variable neighborhood search implementation. Computers & Operations Research, 52, 315–325.
Salhi, S., & Sari, M. (1997). A multi-level composite heuristic for the multi-depot vehicle fleet mix problem. European Journal of Operational Research, 103(1), 95–112.
Salhi, S., Wassan, N., & Hajarat, M. (2013). The fleet size and mix vehicle routing problem with backhauls: Formulation and set partitioning-based heuristics. Transportation Research Part E: Logistics and Transportation Review, 56, 22–35.
Silva, M. M., Subramanian, A., & Ochi, L. S. (2015). An iterated local search heuristic for the split delivery vehicle routing problem. Computers & Operations Research, 53, 234–249.
Subramanian, A., Penna, P. H. V., Uchoa, E., & Ochi, L. S. (2012). A hybrid algorithm for the heterogenous fleet vehicle routing problem. European Journal of Operational Research, 221, 285–295.
Subramanian, A., Uchoa, E., & Ochi, L. S. (2013). A hybrid algorithm for a class of vehicle routing problems. Computers & Operations Research, 40(10), 2519–2531.
Taillard, E. D. (1999). A heuristic column generation method for heterogeneous fleet. RAIRO (Recherche opérationnelle), 33, 1–14.
Tarantilis, C. D., Kiranoudis, C. T., & Vassiliadis, V. S. (2003). A list based threshold accepting metaheuristic for the heterogeneous fixed fleet vehicle routing problem. Journal of the Operational Research Society, 54, 65–71.
Tarantilis, C. D., Kiranoudis, C. T., & Vassiliadis, V. S. (2004). A threshold accepting metaheuristic for the heterogeneous fixed fleet vehicle routing problem. European Journal of Operational Research, 152, 148–158.
Tavakkoli-Moghaddam, R., Safaei, N., Kah, M., & Rabbani, M. (2007). A new capacitated vehicle routing problem with split service for minimizing fleet cost by simulated annealing. Journal of the Franklin Institute, 344(5), 406–425. (Modeling, simulation and applied optimization Part II).
Tütüncü, G. Y. (2010). An interactive gramps algorithm for the heterogeneous fixed fleet vehicle routing problem with and without backhauls. European Journal of Operational Research, 201, 593–600.
Vidal, T., Crainic, T. G., Gendreau, M., & Prins, C. (2013a). Heuristics for multi-attribute vehicle routing problems: A survey and synthesis. European Journal of Operational Research, 231(1), 1–21.
Vidal, T., Crainic, T. G., Gendreau, M., & Prins, C. (2013b). A hybrid genetic algorithm with adaptive diversity management for a large class of vehicle routing problems with time-windows. Computers & Operations Research, 40(1), 475–489.
Vidal, T., Crainic, T. G., Gendreau, M., & Prins, C. (2014). A unified solution framework for multi-attribute vehicle routing problems. European Journal of Operational Research, 234(3), 658–673.
Vidal, T., Crainic, T., Gendreau, M., & Prins, C. (2015). Time-window relaxations in vehicle routing heuristics. Journal of Heuristics, 21(3), 329–358.
Wassan, N. A., & Osman, I. H. (2002). Tabu search variants for the mix fleet vehicle routing problem. Journal of the Operational Research Society, 53, 768–782.
Yao, B., Yu, B., Hu, P., Gao, J., & Zhang, M. (2016). An improved particle swarm optimization for carton heterogeneous vehicle routing problem with a collection depot. Annals of Operations Research, 242(2), 303–320.
Yousefikhoshbakht, M., Didehvar, F., & Rahmati, F. (2014). Solving the heterogeneous fixed fleet open vehicle routing problem by a combined metaheuristic algorithm. International Journal of Production Research, 52(9), 2565–2575.
Acknowledgements
The authors would like to thank the anonymous reviewers for their valuable comments. This research was partially supported by the Conselho Nacional de Desenvolvimento Científico e Tecnológico (CNPq), under Grants: 446683/2014-0 (first author); 305223/2015-1, 428549/2016-0 (second author); 308498/2015-1 (fourth author); 400722/2013-5 (third and fifth authors).
Author information
Authors and Affiliations
Corresponding author
Appendix: Detailed results
Appendix: Detailed results
1.1 HFFVRP-V
Detailed results obtained for the HFFVRP-V instances of: (i) (Brandão 2011, B11), compared with the TSA of (Brandão 2011, B11) and the ILS-RVND-SP of (Subramanian et al. 2012, SPUO12) (Table 7); and (ii) (Li et al. 2007, LGW07), compared with (Brandão 2011, B11) and (Subramanian et al. 2012, SPUO12) (Table 8).
1.2 HFFVRP-FV
Detailed results obtained for the HFFVRP instances of (Duhamel et al. 2011, DLP11), compared with the sequential version of the GRASP \(\times \) ELS of (Duhamel et al. 2013, DLP13). Column BKS in Tables 9–12 indicates the best results considering all versions of the GRASP \(\times \) ELS (sequential or parallel). The instances were divided into four sets:
-
DLP11–set 1: [20–95] customers (Table 9);
-
DLP11–set 2: [102–147] customers (Table 10);
-
DLP11–set 3: [152–196] customers (Table 11);
-
DLP11–set 4: [203–256] customers (Table 12).
1.3 HFFOVRP
Detailed results obtained for the instances of (Taillard 1999, T99) as considered in (Yousefikhoshbakht et al. 2014, YDR14). The results obtained by HILS-RVRP were compared with those found by the BRMTS heuristic of the referred authors. Moreover, although Yousefikhoshbakht et al. (2014) mentioned that they used fixed and variant vehicle costs, it appears, according to our testing, that they only used variable costs. Table 13 presents the results involving only variable costs, while Table 14 reports the results involving both fixed and variable costs.
1.4 Results for the MDFSM
Detailed results obtained for the MDFSM instances of (Salhi and Sari 1997, SS97), compared with those found by the VNS2 of (Salhi et al. 2014, SIW14) and the HGSADC of (Vidal et al. 2014, VCGP14) (Table 15). Column RD in Table 15 indicates the instances with route duration.
1.5 HFFVRPB
Detailed results obtained for the HFFVRPB instances of (Tütüncü 2010, T10), compared with those found by the GRAMPS and ADVISER heuristics from the referred authors (Table 16 describes the results found). Note that we did not report the results for some instances because, according to the values suggested by the authors, they are infeasible.
1.6 FSMB
Detailed results obtained for the FSMB instances of (Salhi et al. 2013, SWH13), compared with those found by Framework-2 from the same authors (Table 17).
1.7 SDepVRP
Detailed results obtained for the SDepVRP instances of (Cordeau and Laporte 2001, CL01), compared with those found by the ALNS 50k of (Pisinger and Røpke 2007, PR07) and the ITS of (Cordeau and Maischberger 2012, CM12) (Table 18, old instances without route duration and Table 19, new instances with route duration).
1.8 HFFVRPSD
Detailed results obtained for the FSM instances of (Golden et al. 1984, G84) and adapted for the HFFVRPSD by (Ozfirat and Ozkarahan 2010, OO10), compared with those found by the CP heuristic of the same authors (Table 20). As HFFVRPSD allows visiting the customers more than once, the SP procedure was not considered for this variant.
1.9 FSMVRPTW: minimize route duration
Detailed results obtained for the FSMVRPTW instances of (Liu and Shen 1999, LS99), considering the objective of minimizing the sum of the route durations, compared with those found by the UHGS of (Vidal et al. 2014, VCGP14) and the HEA of (Koç et al. 2015, KBJL15) (Tables 21, 22, 23).
1.10 FSMTW: minimize total distance
Detailed results obtained for the FSMVRPTW instances of (Liu and Shen 1999, LS99), considering the objective of minimizing the sum of the total distance, compared with those found by the UHGS of (Vidal et al. 2014, VCGP14) and the HEA of (Koç et al. 2015, KBJL15) (Tables 24, 25, 26).
1.11 HFFVRPMBTW
Detailed results obtained for the HFFVRPMBTW instances of (Belmecheri et al. 2013, BPYA13), compared with the results obtained by the PSO of the same authors and the EBBO of (Berghida and Boukra 2015, BB15) (Tables 27, 28, 29).
1.12 SDepVRPTW
Detailed results obtained for the SDepVRPTW instances of (Cordeau and Laporte 2001, CL01), compared with those found by the ITS1 of (Cordeau and Maischberger 2012, CM12) and by the HGSADC of (Vidal et al. 2013b, VCGP13) (Table 30).
Rights and permissions
About this article
Cite this article
Penna, P.H.V., Subramanian, A., Ochi, L.S. et al. A hybrid heuristic for a broad class of vehicle routing problems with heterogeneous fleet. Ann Oper Res 273, 5–74 (2019). https://doi.org/10.1007/s10479-017-2642-9
Published:
Issue Date:
DOI: https://doi.org/10.1007/s10479-017-2642-9