Abstract
The intelligent teaching system is the most important in the field of teaching. It uses artificial intelligence technology to bring a lot of help to learners in terms of knowledge and skill acquisition. In this process, human tutors are not required to participate. The student model is the core of the intelligent teaching system. Using the Bayesian network with strong self-learning ability to construct the intelligent teaching system student model can significantly improve the intelligence level of the intelligent teaching system. Firstly, we discussed the basic concepts of the student model of the intelligent teaching system. Then, from the perspective of students’ ability teaching, combined with the students’ learning status and characteristics, the factors influencing the students’ learning process are analyzed. Finally, an intelligent teaching system student model was built based on Bayesian network. This model can objectively evaluate students’ cognitive ability and can infer students’ next action. In addition, the model is also applicable to the online test system, and the experimental results obtained demonstrate the effectiveness of the modified model.









Similar content being viewed by others
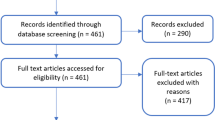
References
Zhou W (2018) Research on zhu jiahua’s thought and practice of education reform [D]. Jiangsu university
Nord WR (1969) Beyond the teaching machine: the neglected area of operant conditioning in the theory and practice of management[J]. Organ Behav Hum Perform 4(4):375–401
Hilles MM, Naser SSA (2017) Knowledge-based intelligent tutoring system for teaching mongo database[J]. Eur Acad Res 4(10):8783–8794
He BX, Zhuang KJ (2017) Research on the intelligent information system for the multimedia teaching equipment management[C] international conference on information system and artificial intelligence. IEEE:129–132
Sharma S, Ghorpade S, Sahni A et al (2012) Survey of intelligent tutoring systems: a review on the development of expert/intelligent tutoring systems, various teaching strategies and expert tutoring system design suggestions[J]. Polym J 44(6):522–530
Sun L (2017) Knowledge representation and fusion method of human resource-related competitive intelligence[C] international conference on Progress in informatics and computing. IEEE:18–22
Wen M-F, Xu Z-L, Yang C-H (2006) Remote open network teaching system based on intelligent agent technology[J]. CET China Educ Technol 1:89–92
HU Shui -xing (2017) Empirical analysis on educational data mining and its instructional application [J]. Modern Distance Education 4:129–132
Paassen B, Mokbel B, Hammer B (2016) Adaptive structure metrics for automated feedback provision in intelligent tutoring systems[J]. Neurocomputing 192:3–13
Mahmoud MH, El-Hamayed SHA (2016) An intelligent tutoring system for teaching the grammar of the Arabic language[J]. J Electr Syst Inf Technol 3(2):282–294
Jing W, Tao Z, Yanhui L (2017) Network intelligent teaching system based on ontology[J]. Agro Food Industry Hi Tech 28(1):1867–1870
Qingjun Z, Liyan D (2017) The reform of teaching paradigm guided by the intelligent teaching and learning system --taking the 7th grade course reform in Shizhuyuan Shool as an example[J]. CET China Educ Technol (10):103–108
Shenglan XUE (2017) Research on the application of smartphones integrating into classroom teaching[J]. E-education Researc 39(1):86–91
Gang Z, Haitao H, Qiang Z (2017) Analysis of (Maple T.A.) role of online assignments and intelligent scoring system in mathematics teaching[J]. Experimental Technol Manage 34(4):136–137
Alhabbash MI, Mahdi AO, Naser SSA (2016) An intelligent tutoring system for teaching grammar English tenses[J]. Eur Acad Res 4(9):1243–1249
Zhang H (2019) Explore teachers' teaching ability and develop and utilize students' multiple intelligence [D]. Hebei normal university
Zhang Y (2019) Nonlinear signal prediction based on wavelet decomposition and neural network [D]. Hebei normal university
Zhang B, Jia J (2017) Evaluating an intelligent tutoring system for personalized math teaching[C]// international symposium on educational technology. IEEE:126–130
Jugo I, Kovačić B, Slavuj V (2016) Increasing the adaptivity of an intelligent tutoring system with educational data mining: a system overview[J]. Int J Emerg Technol Learn:423–425
Graesser AC, Chipman P, Haynes BC, Olney A (2005) AutoTutor: an intelligent tutoring system with mixed-initiative dialogue[J]. IEEE Trans Educ 48(4):612–618
Li-yuan Z, Wang C-l (2012) Siri personal assistant application research for intelligent tutor system[J]. CET China Educ Technol 10:131–135
Rongmei Z, Fuliang L (2008) Study on Internet intelligent tutoring system based on agent[J]. Modern Electronic Technique 31(6):83–86
Li T (2017) Design of intelligent auxiliary teaching system based on knowledge grid[J]. Modem Compute 5:22–24
Bai Y. Design of Intelligent Evaluation System of Physical Education Teaching Based on Artificial Intelligence Expert Decision System[J]. 2016: 362–370
Lu QR, Zhu J, Tang QL (2013) Design of an intelligent lighting and monitoring system of teaching building based on LabVIEW[J]. Adv Mater Res 756-759:584–588
Liang Y-l, Ying-hao L (2012) Speech assessment-based intelligent tutoring system for spoken English learning[J]. Mod Educ Technol 22(11):82–85
Jaques PA, Seffrin H, Rubi G, de Morais F, Ghilardi C, Bittencourt II, Isotani S (2013) Rule-based expert systems to support step-by-step guidance in algebraic problem solving: the case of the tutor PAT2Math[J]. Expert Syst Appl 40(14):5456–5465
Li, Hongmei, Xu, Zhenhua. Case Study on English Learning Supported by Intelligent Tutoring System[J], 2012, (3): 66–72
Wang D-q, Liu Q-b, Ren G-j et al (2012) A problem-solving intelligent tutor system[J]. CET China Educ Technol (8):90–94
Kure Y (2016) Apparatus and method for synchronized transmission of multimedia content over an asynchronous network[J]. Jordan J Pharm Sci 5(1):65–73
Husnaeni (2016) The enhancement of mathematical critical thinking ability of Aliyah madrasas student model using Gorontalo by interactive learning setting cooperative model [J]. J Educ Pract 7
Chavez M (2016) The first language in the foreign language classroom: teacher model and student language use – an exploratory study[J]. Classroom Discourse 7(2):1–33
Cussens J, Haws D (2017) Polyhedral aspects of score equivalence in Bayesian network structure learning[J]. Math Program 164(1–2):1–40
Campos LMD, Fernández-Luna JM, Huete JF (2003) The BNR model: foundations and performance of a Bayesian network-based retrieval model[J]. Int J Approx Reason 34(2):265–285
Yet B, Perkins ZB, Rasmussen TE, Tai NRM, Marsh DWR (2014) Combining data and meta-analysis to build Bayesian networks for clinical decision support[J]. J Biomed Inform 52(C):373–385
Zhou Y, Fenton N, Zhu C (2016) An empirical study of Bayesian network parameter learning with monotonic influence constraints[J]. Decis Support Syst 87(C):69–79
You Y, Li J, Xu N (2017) A constrained parameter evolutionary learning algorithm for Bayesian network under incomplete and small data[C] Chinese control conference, pp 3044–3051
Silander T, Roos T, Myllymäki P (2010) Learning locally minimax optimal Bayesian networks[J]. Int J Approx Reason 51(5):544–557
Hu J-s, Zhang L, Huang H-j (2016) Application of project-driven heuristic teaching mode in software engineering teaching[J]. Comput Eng Sci 38(6):228–231
Funding
This work is supported by Inner Mongolia University for Nationalities scientific research projects (NMDYB15023, NMDYB15096) and College Scientific Research Project of Inner Mongolia Autonomous Region (NJZY19156).
Author information
Authors and Affiliations
Corresponding author
Additional information
Publisher’s note
Springer Nature remains neutral with regard to jurisdictional claims in published maps and institutional affiliations.
Rights and permissions
About this article
Cite this article
Wu, L. Student model construction of intelligent teaching system based on Bayesian network. Pers Ubiquit Comput 24, 419–428 (2020). https://doi.org/10.1007/s00779-019-01311-3
Received:
Accepted:
Published:
Issue Date:
DOI: https://doi.org/10.1007/s00779-019-01311-3