Abstract
Objectives
To investigate the value of R2* mapping–based radiomics nomograms in staging liver fibrosis in patients with chronic hepatitis B.
Methods
Between January 2020 and December 2020, 151 patients with chronic hepatitis B were randomly divided into training (n = 103) and validation (n = 48) cohorts. From January to February 2021, 58 patients were included in a test cohort. Radiomics features were selected using the interclass correlation coefficient and least absolute shrinkage and selection operator method. Three radiomics nomograms, combining the radiomics score (Radscore) derived from R2* mapping and clinical variables, were used for staging significant and advanced fibrosis, and cirrhosis. Performance of the model was evaluated using the AUC. The utility and clinical benefits were evaluated using the continuous net reclassification index (NRI), integrated discrimination improvement (IDI), and decision curve analysis (DCA).
Results
The Radscore calculated by 12 radiomics features and independent factors (laminin and platelet) of advanced fibrosis were used to construct the radiomics nomograms. In the test cohort, the AUCs of the radiomics nomograms for staging significant fibrosis, advanced fibrosis, and cirrhosis were 0.738 (95% confidence interval [CI]: 0.604–0.872), 0.879 (95% CI: 0.779–0.98), and 0.952 (95% CI: 0.878–1), respectively. NRI, IDI, and DCA confirmed that radiomics nomograms demonstrated varying degrees of clinical benefit and improvement for advanced fibrosis and cirrhosis, but not for significant fibrosis.
Conclusions
Radiomics nomograms combined with R2* mapping–based Radscore, laminin, and platelet have value in staging advanced fibrosis and cirrhosis but limited value for staging significant fibrosis.
Key Points
• Laminin and platelets were independent predictors of advanced fibrosis.
• Radiomics analysis based on R2* mapping was beneficial for evaluating advanced fibrosis and cirrhosis.
• It was difficult to distinguish significant fibrosis using a radiomics nomogram, which is possibly due to the complex pathological microenvironment of chronic liver diseases.






Similar content being viewed by others
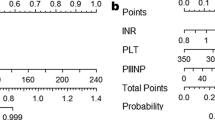
Abbreviations
- AIC:
-
Akaike’s information criterion
- ALB:
-
Albumin
- ALP:
-
Alkaline phosphatase
- ALT:
-
Alanine aminotransferase
- APRI:
-
Aminotransferase-to-platelet ratio index
- AST:
-
Aspartate aminotransferase
- CHE:
-
Cholinesterase
- CI:
-
Confidence interval
- CIV:
-
Type IV collagen
- CLD:
-
Chronic liver diseases
- DB:
-
Direct bilirubin
- DCA:
-
Decision curve analysis
- FIB-4:
-
Fibrosis-4 index
- GGT:
-
γ-Glutamyl transpeptidase
- GLO:
-
Globulin
- HA:
-
Hyaluronic acid
- HGB:
-
Hemoglobin
- ICC:
-
interclass correlation coefficient
- ID:
-
Indirect bilirubin
- IDEAL-IQ:
-
Iterative decomposition of water and fat with echo asymmetry and least squares estimation
- IDI:
-
Integrated discrimination improvement
- IVIM:
-
Intravoxel incoherent motion
- LASSO:
-
Least absolute shrinkage and selection operator
- LDH:
-
Lactate dehydrogenase
- LN:
-
Laminin
- LYM:
-
Lymphocytes
- MAO:
-
Monoamine oxidase
- NEUT:
-
Neutrophil count
- NRI:
-
Net reclassification index
- PA:
-
Prealbumin
- PDFF:
-
Proton density fat fraction
- PIIIP:
-
Type III procollagen N peptide
- PLT:
-
Platelets
- Radscore:
-
Radiomic score
- RBC:
-
Red blood cells
- ROC:
-
Receiver operator characteristic
- TB:
-
Total bilirubin
- TBA:
-
Total bile acid,
- TP:
-
Total protein
- VIF:
-
Variance inflation factor
- WBC:
-
White blood cell
References
Tsochatzis EA, Bosch J, Burroughs AK (2014) Liver cirrhosis. Lancet 383:1749–1761
Schuppan D, Ashfaq-Khan M, Yang AT, Kim YO (2018) Liver fibrosis: direct antifibrotic agents and targeted therapies. Matrix Biol 68-69:435–451
Buzzetti E, Petta S, Manuguerra R et al (2019) Evaluating the association of serum ferritin and hepatic iron with disease severity in non-alcoholic fatty liver disease. Liver Int 39:1325–1334
Martinelli AL, Filho AB, Franco RF et al (2004) Liver iron deposits in hepatitis B patients: association with severity of liver disease but not with hemochromatosis gene mutations. J Gastroenterol Hepatol 19:1036–1041
Mehta KJ, Farnaud SJ, Sharp PA (2019) Iron and liver fibrosis: mechanistic and clinical aspects. World J Gastroenterol 25:521–538
Obrzut M, Atamaniuk V, Glaser KJ et al (2020) Value of liver iron concentration in healthy volunteers assessed by MRI. Sci Rep 10:17887
Yu H, McKenzie CA, Shimakawa A et al (2007) Multiecho reconstruction for simultaneous water-fat decomposition and T2* estimation. J Magn Reson Imaging 26:1153–1161
Czaja AJ (2019) Review article: iron disturbances in chronic liver diseases other than haemochromatosis - pathogenic, prognostic, and therapeutic implications. Aliment Pharmacol Ther 49:681–701
Chow AM, Gao DS, Fan SJ et al (2012) Liver fibrosis: an intravoxel incoherent motion (IVIM) study. J Magn Reson Imaging 36:159–167
Tosun M, Onal T, Uslu H, Alparslan B, Çetin Akhan S (2020) Intravoxel incoherent motion imaging for diagnosing and staging the liver fibrosis and inflammation. Abdom Radiol (NY) 45:15–23
Liang J, Song X, Xiao Z, Chen H, Shi C, Luo L (2018) Using IVIM-MRI and R2* mapping to differentiate early stage liver fibrosis in a rat model of radiation-induced liver fibrosis. Biomed Res Int 2018:4673814
Jafari R, Hectors SJ, Koehne de González AK et al (2021) Integrated quantitative susceptibility and R2 * mapping for evaluation of liver fibrosis: an ex vivo feasibility study. NMR Biomed 34:e4412
Yoshikawa M, Kudo K, Harada T et al (2021) Quantitative susceptibility mapping versus R2*-based histogram analysis for evaluating liver fibrosis: preliminary results. Magn Reson Med Sci. https://doi.org/10.2463/mrms.mp.2020-0175
Pan Z, Li Z, Meng F, Hu Y, Zhang X, Chen Y (2022) Fat- and iron-corrected ADC to assess liver fibrosis in patients with chronic hepatitis B. Diagn Interv Radiol 28:5–11
Hu F, Yang R, Huang Z et al (2019) 3D Multi-Echo Dixon technique for simultaneous assessment of liver steatosis and iron overload in patients with chronic liver diseases: a feasibility study. Quant Imaging Med Surg 9:1014–1024
Lambin P, Rios-Velazquez E, Leijenaar R et al (2012) Radiomics: extracting more information from medical images using advanced feature analysis. Eur J Cancer 48:441–446
Wang JC, Fu R, Tao XW et al (2020) A radiomics-based model on non-contrast CT for predicting cirrhosis: make the most of image data. Biomark Res 8:47
Ni M, Wang L, Yu H et al (2021) Radiomics approaches for predicting liver fibrosis with nonenhanced T1 -weighted imaging: comparison of different radiomics models. J Magn Reson Imaging 53:1080–1089
Qiu QT, Zhang J, Duan JH, Wu SZ, Ding JL, Yin Y (2020) Development and validation of radiomics model built by incorporating machine learning for identifying liver fibrosis and early-stage cirrhosis. Chin Med J (Engl) 133:2653–2659
Trovillion EM, Schubert L, Dietz AC (2018) Iron overload in survivors of childhood cancer. J Pediatr Hematol Oncol 40:396–400
Wai CT, Greenson JK, Fontana RJ et al (2003) A simple noninvasive index can predict both significant fibrosis and cirrhosis in patients with chronic hepatitis C. Hepatology 38:518–526
Sterling RK, Lissen E, Clumeck N et al (2006) Development of a simple noninvasive index to predict significant fibrosis in patients with HIV/HCV coinfection. Hepatology 43:1317–1325
Sun Y, Zhou J, Wang L et al (2017) New classification of liver biopsy assessment for fibrosis in chronic hepatitis B patients before and after treatment. Hepatology 65:1438–1450
Scheuer PJ (1991) Classification of chronic viral hepatitis: a need for reassessment. J Hepatol 13:372–374
Hu P, Hu X, Lin Y et al (2021) A combination model of radiomics features and clinical biomarkers as a nomogram to differentiate nonadvanced from advanced liver fibrosis: a retrospective study. Acad Radiol 28(Suppl 1):S45–S54
Schawkat K, Ciritsis A, von Ulmenstein S et al (2020) Diagnostic accuracy of texture analysis and machine learning for quantification of liver fibrosis in MRI: correlation with MR elastography and histopathology. Eur Radiol 30:4675–4685
Yokoo T, Wolfson T, Iwaisako K et al (2015) Evaluation of liver fibrosis using texture analysis on combined-contrast-enhanced magnetic resonance images at 3.0T. Biomed Res Int 2015:387653
Yeung J, Ganeshan B, Endozo R et al (2020) Equilibrium CT texture analysis for the evaluation of hepatic fibrosis: preliminary evaluation against histopathology and extracellular volume fraction. J Pers Med 10:46
Zhang X, Gao X, Liu BJ et al (2015) Effective staging of fibrosis by the selected texture features of liver: which one is better, CT or MR imaging? Comput Med Imaging Graph 46(Pt 2):227–236
Homayounieh F, Saini S, Mostafavi L et al (2020) Accuracy of radiomics for differentiating diffuse liver diseases on non-contrast CT. Int J Comput Assist Radiol Surg 15:1727–1736
Park HJ, Lee SS, Park B et al (2019) Radiomics analysis of gadoxetic acid-enhanced MRI for staging liver fibrosis. Radiology 290:380–387
Wu S, Li H, Dong A et al (2021) Differences in radiomics signatures between patients with early and advanced T-stage nasopharyngeal carcinoma facilitate prognostication. J Magn Reson Imaging 54:854–865
Uno H, Tian L, Cai T, Kohane IS, Wei LJ (2013) A unified inference procedure for a class of measures to assess improvement in risk prediction systems with survival data. Stat Med 32:2430–2442
Pencina MJ, D’Agostino RB Sr, Steyerberg EW (2011) Extensions of net reclassification improvement calculations to measure usefulness of new biomarkers. Stat Med 30:11–21
Vickers AJ, Elkin EB (2006) Decision curve analysis: a novel method for evaluating prediction models. Med Decis Making 26:565–574
Song J, Shi J, Dong D et al (2018) A new approach to predict progression-free survival in stage IV EGFR-mutant NSCLC patients with EGFR-TKI therapy. Clin Cancer Res 24:3583–3592
Wu J, Zhang Q, Zhao Y et al (2019) Radiomics analysis of iodine-based material decomposition images with dual-energy computed tomography imaging for preoperatively predicting microsatellite instability status in colorectal cancer. Front Oncol 9:1250
Hu HT, Wang Z, Huang XW et al (2019) Ultrasound-based radiomics score: a potential biomarker for the prediction of microvascular invasion in hepatocellular carcinoma. Eur Radiol 29:2890–2901
Hope TA, Ohliger MA, Qayyum A (2014) MR imaging of diffuse liver disease: from technique to diagnosis. Radiol Clin North Am 52:709–724
Younesi S, Parsian H (2019) Diagnostic accuracy of glycoproteins in the assessment of liver fibrosis: a comparison between laminin, fibronectin, and hyaluronic acid. Turk J Gastroenterol 30:524–531
Körner T, Kropf J, Gressner AM (1996) Serum laminin and hyaluronan in liver cirrhosis: markers of progression with high prognostic value. J Hepatol 25:684–688
Wang K, Lu X, Zhou H et al (2019) Deep learning radiomics of shear wave elastography significantly improved diagnostic performance for assessing liver fibrosis in chronic hepatitis B: a prospective multicentre study. Gut 68:729–741
Corrias G, Erta M, Sini M et al (2021) Comparison of multimaterial decomposition fat fraction with DECT and proton density fat fraction with IDEAL IQ MRI for quantification of liver steatosis in a population exposed to chemotherapy. Dose Response 19:1559325820984938
Eskreis-Winkler S, Corrias G, Monti S et al (2018) IDEAL-IQ in an oncologic population: meeting the challenge of concomitant liver fat and liver iron. Cancer Imaging 18:51
Barash H, Gross E, Matot I et al (2007) Functional MR imaging during hypercapnia and hyperoxia: noninvasive tool for monitoring changes in liver perfusion and hemodynamics in a rat model. Radiology 243:727–735
Hernando D, Kramer JH, Reeder SB (2013) Multipeak fat-corrected complex R2* relaxometry: theory, optimization, and clinical validation. Magn Reson Med 70:1319–1331
Li J, Lin H, Liu T et al (2018) Quantitative susceptibility mapping (QSM) minimizes interference from cellular pathology in R2* estimation of liver iron concentration. J Magn Reson Imaging 48:1069–1079
Kee Y, Sandino CM, Syed AB et al (2021) Free-breathing R2* mapping of hepatic iron overload in children using 3D multi-echo UTE cones MRI. Magn Reson Med 85:2608–2621
Funding
The authors state that this work has not received any funding.
Author information
Authors and Affiliations
Corresponding authors
Ethics declarations
Guarantor
The scientific guarantor of this publication is Chen Zhang.
Conflict of interest
The authors of this manuscript declare no relationships with any companies whose products or services may be related to the subject matter of the article.
Statistics and biometry
No complex statistical methods were necessary for this paper.
Informed consent
Written informed consent was waived by the Institutional Review Board.
Ethical approval
Institutional Review Board approval was obtained.
Methodology
• retrospective
• diagnostic study
• performed at one institution
Additional information
Publisher’s note
Springer Nature remains neutral with regard to jurisdictional claims in published maps and institutional affiliations.
Supplementary information
ESM 1
(DOCX 396 kb)
Rights and permissions
Springer Nature or its licensor holds exclusive rights to this article under a publishing agreement with the author(s) or other rightsholder(s); author self-archiving of the accepted manuscript version of this article is solely governed by the terms of such publishing agreement and applicable law.
About this article
Cite this article
Zhang, D., Cao, Y., Sun, Y. et al. Radiomics nomograms based on R2* mapping and clinical biomarkers for staging of liver fibrosis in patients with chronic hepatitis B: a single-center retrospective study. Eur Radiol 33, 1653–1667 (2023). https://doi.org/10.1007/s00330-022-09137-z
Received:
Revised:
Accepted:
Published:
Issue Date:
DOI: https://doi.org/10.1007/s00330-022-09137-z