Abstract
Objectives
To develop and validate a combined model based on Gd–BOPTA-enhanced MRI to identify advanced liver fibrosis.
Methods
A total of 102 patients with chronic HBV infection were divided into a training cohort (n = 80) and a time-independent testing cohort 1 (n = 22). In the training cohort, radiomics signatures were extracted from the hepatobiliary phase. Model 1 was constructed with clinic-radiological factors using multivariable logistic regression to predict advanced liver fibrosis, and model 2 incorporated radiomics signatures based on model 1. The diagnostic performances were compared with serum fibrosis tests and FibroScan tests using area under curve (AUC) in testing cohort 1. Another 45 patients with other causes were collected in testing cohort 2 for further validation.
Results
Model 1 showed age (OR = 1.079) and periportal space widening (OR = 7.838) were the independent factors for predicting advanced fibrosis. After integrating radiomics signatures, model 2 enabled more accurately than model 1 in training cohort (0.940 vs. 0.802, p = 0.003). In testing cohort 1, model 2 demonstrated a superior AUC compared with model 1 (0.900 vs. 0.813,p = 0.131), FibroScan test (0.900 vs. 0.733, p = 0.193), and serum fibrosis tests (APRI and Fib-4 was 0.667 and 0.791). In testing cohort 2, model 2 incorporating radiomics signatures showed satisfactory performance (0.874 vs. 0.757,p = 0.010) compared with model 1.
Conclusions
Radiomics signatures derived from Gd-BOPTA–enhanced HBP images may offer complementary information to the clinic-radiological model for predicting advanced liver fibrosis.
Key Points
• Linear or reticular hyperintensity on T2WI, periportal space widening, and diffuse periportal enhancement on HBP can be useful for predicting advanced liver fibrosis.
• Clinic-radiological features such as patient age and periportal space widening are the two independent factors predicting advanced fibrosis.
• Radiomics signatures derived from Gd-BOPTA-enhanced HBP images offer complementary information to the clinic-radiological model for predicting advanced liver fibrosis.






Similar content being viewed by others
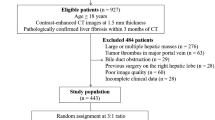
Abbreviations
- ALT:
-
Alanine aminotransferase
- APRI:
-
Aspartate transaminase–to-platelet ratio index
- AST:
-
Aspartate transaminase
- AUC:
-
Area under the curve
- DWI:
-
Diffusion-weighted imaging
- Fib-4:
-
Fibrosis-4 index
- FOV:
-
Field of view
- Gd-BOPTA:
-
Gadobenate dimeglumine
- Gd-EOB-DTPA:
-
Gadolinium-ethoxybenzyl-diethylenetriamine
- HBP:
-
Hepatobiliary phase
- HBV:
-
Hepatitis B virus
- ICC:
-
Inter-correlation coefficient
- MAFLD:
-
Metabolic-associated fatty liver disease
- MRE:
-
MR elastography
- MRI:
-
Magnetic resonance imaging
- PT:
-
Prothrombin time
- PVP:
-
Portal venous phase
- ROI:
-
Regions of interest
- TBIL:
-
Total bilirubin
- TE:
-
Echo time
- TR:
-
Repetition time
- UE:
-
Ultrasound-based elastography
References
Lee HW, Lee JS, Ahn SH (2020) Hepatitis B virus cure: targets and future therapies. Int J Mol Sci 22:213
Seitz HK, Bataller R, Cortez-Pinto H et al (2018) Alcoholic liver disease. Nat Rev Dis Primers 4:16
Kuchay MS, Choudhary NS, Mishra SK (2020) Pathophysiological mechanisms underlying MAFLD. Diabetes Metab Syndr 14:1875–1887
Borssén ÅD, Palmqvist R, Kechagias S et al (2017) Histological improvement of liver fibrosis in well-treated patients with autoimmune hepatitis: a cohort study. Medicine (Baltimore) 96:e7708
Robotin MC (2011) Hepatitis B prevention and control: lessons from the East and the West. World J Hepatol 3:31–37
Roehlen N, Crouchet E, Baumert TF (2020) Liver fibrosis: mechanistic concepts and therapeutic perspectives. Cells 9:875
Kim SU, Park JY, Kim DY et al (2010) Non-invasive assessment of changes in liver fibrosis via liver stiffness measurement in patients with chronic hepatitis B: impact of antiviral treatment on fibrosis regression. Hepatol Int 4:673–680
Afdhal NH, Nunes D (2004) Evaluation of liver fibrosis: a concise review. Am J Gastroenterol 99:1160–1174
Martínez SM, Crespo G, Navasa M, Forns X (2011) Noninvasive assessment of liver fibrosis. Hepatology 53:325–335
Chin JL, Pavlides M, Moolla A, Ryan JD (2016) Non-invasive markers of liver fibrosis: adjuncts or alternatives to liver biopsy? Front Pharmacol 7:159
Yin M, Glaser KJ, Talwalkar JA, Chen J, Manduca A, Ehman RL (2016) Hepatic MR elastography: clinical performance in a series of 1377 consecutive examinations. Radiology 278:114–124
Tang A, Cloutier G, Szeverenyi NM, Sirlin CB (2015) Ultrasound elastography and MR elastography for assessing liver fibrosis: Part 2, diagnostic performance, confounders, and future directions. AJR Am J Roentgenol 205:33–40
Foucher J, Chanteloup E, Vergniol J et al (2006) Diagnosis of cirrhosis by transient elastography (FibroScan): a prospective study. Gut 55:403–408
Hsu C, Caussy C, Imajo K et al (2019) Magnetic resonance vs transient elastography analysis of patients with nonalcoholic fatty liver disease: a systematic review and pooled analysis of individual participants. Clin Gastroenterol Hepatol 17:630–637.e638
Li J, Liu H, Zhang C et al (2020) Native T1 mapping compared to ultrasound elastography for staging and monitoring liver fibrosis: an animal study of repeatability, reproducibility, and accuracy. Eur Radiol 30:337–345
Catania R, Furlan A, Smith AD, Behari J, Tublin ME, Borhani AA (2021) Diagnostic value of MRI-derived liver surface nodularity score for the non-invasive quantification of hepatic fibrosis in non-alcoholic fatty liver disease. Eur Radiol 31:256–263
European Association for the Study of the Liver. Electronic address eee, Clinical Practice Guideline P, Chair, representative EGB, Panel m (2021) EASL Clinical Practice Guidelines on non-invasive tests for evaluation of liver disease severity and prognosis - 2021 update. J Hepatol 75:659–689
Puigvehí M, Broquetas T, Coll S et al (2017) Impact of anthropometric features on the applicability and accuracy of FibroScan(®) (M and XL) in overweight/obese patients. J Gastroenterol Hepatol 32:1746–1753
Wong GL, Wong VW, Chim AM et al (2011) Factors associated with unreliable liver stiffness measurement and its failure with transient elastography in the Chinese population. J Gastroenterol Hepatol 26:300–305
Wagner M, Corcuera-Solano I, Lo G et al (2017) Technical failure of MR elastography examinations of the liver: experience from a large single-center study. Radiology 284:401–412
Pickhardt PJ, Graffy PM, Said A et al (2019) Multiparametric CT for noninvasive staging of hepatitis C virus-related liver fibrosis: correlation with the histopathologic fibrosis score. AJR Am J Roentgenol 212:547–553
Ludwig DR, Fraum TJ, Ballard DH, Narra VR, Shetty AS (2021) Imaging biomarkers of hepatic fibrosis: reliability and accuracy of hepatic periportal space widening and other morphologic features on MRI. AJR Am J Roentgenol 216:1229–1239
Hako R, Kristian P, Jarcuska P et al (2019) Noninvasive assessment of liver fibrosis in patients with chronic hepatitis B or C by contrast-enhanced magnetic resonance imaging. Can J Gastroenterol Hepatol 2019:3024630
Shu J, Zhao JN, Han FG, Tang GC, Chen X (2017) Chronic hepatitis B: correlation of abnormal features on T2-weighted imaging and dynamic contrast-enhanced imaging with hepatic histopathology. Radiol Med 122:807–813
Venkatesh SK, Yin M, Takahashi N, Glockner JF, Talwalkar JA, Ehman RL (2015) Non-invasive detection of liver fibrosis: MR imaging features vs. MR elastography. Abdom Imaging 40:766–775
Park HJ, Lee SS, Park B et al (2019) Radiomics analysis of gadoxetic acid-enhanced MRI for staging liver fibrosis. Radiology 290:380–387
Yasaka K, Akai H, Kunimatsu A, Abe O, Kiryu S (2018) Liver fibrosis: deep convolutional neural network for staging by using gadoxetic acid-enhanced hepatobiliary phase MR images. Radiology 287:146–155
Wai CT, Greenson JK, Fontana RJ et al (2003) A simple noninvasive index can predict both significant fibrosis and cirrhosis in patients with chronic hepatitis C. Hepatology 38:518–526
Sterling RK, Lissen E, Clumeck N et al (2006) Development of a simple noninvasive index to predict significant fibrosis in patients with HIV/HCV coinfection. Hepatology 43:1317–1325
Batts KP, Ludwig J (1995) Chronic hepatitis. An update on terminology and reporting. Am J Surg Pathol 19:1409–1417
Curovic Rotbain E, Lund Hansen D, Schaffalitzky de Muckadell O, Wibrand F, Meldgaard Lund A, Frederiksen H (2017) Splenomegaly - diagnostic validity, work-up, and underlying causes. PLoS One 12:e0186674
Bezerra AS, D'Ippolito G, Faintuch S, Szejnfeld J, Ahmed M (2005) Determination of splenomegaly by CT: is there a place for a single measurement? AJR Am J Roentgenol 184:1510–1513
Filippone A, Blakeborough A, Breuer J et al (2010) Enhancement of liver parenchyma after injection of hepatocyte-specific MRI contrast media: a comparison of gadoxetic acid and gadobenate dimeglumine. J Magn Reson Imaging 31:356–364
Wu Z, Osamu M, Azusa K et al (2015) Hepatitis C related chronic liver cirrhosis: feasibility of texture analysis of MR images for classification of fibrosis stage and necroinflammatory activity grade. PLoS ONE 10:e0118297
Guo X, Schwartz LH, Zhao B (2019) Automatic liver segmentation by integrating fully convolutional networks into active contour models. Med Phys 46:4455–4469
Ren J, Yuan Y, Qi M, Tao X (2020) Machine learning-based CT texture analysis to predict HPV status in oropharyngeal squamous cell carcinoma: comparison of 2D and 3D segmentation. Eur Radiol 30:6858–6866
Elkilany A, Fehrenbach U, Auer TA et al (2021) A radiomics-based model to classify the etiology of liver cirrhosis using gadoxetic acid-enhanced MRI. Sci Rep 11:10778
Scalco E, Rizzo G (2017) Texture analysis of medical images for radiotherapy applications. Br J Radiol 90:20160642
Terrault NA, Lok ASF, McMahon BJ et al (2018) Update on prevention, diagnosis, and treatment of chronic hepatitis B: AASLD 2018 hepatitis B guidance. Hepatology 67:1560–1599
Jiang K, Zhang L, Li J et al (2022) Diagnostic efficacy of FibroScan for liver inflammation in patients with chronic hepatitis B: a single-center study with 1185 liver biopsies as controls. BMC Gastroenterol 22:37
Onishi H, Theisen D, Zachoval R, Reiser MF, Zech CJ (2019) Intrahepatic diffuse periportal enhancement patterns on hepatobiliary phase gadoxetate disodium-enhanced liver MR images. Medicine (Baltimore) 98:e14784
Funding
This study has received funding from the Scientific Research Project from the Education Department of Fujian Province (JAT200167)
Author information
Authors and Affiliations
Corresponding author
Ethics declarations
Guarantor
The scientific guarantor of this publication is Prof. Dairong Cao, MD, The First Affiliated Hospital of Fujian Medical University.
Conflict of interest
The authors of this manuscript declare no relationships with any companies, whose products or services may be related to the subject matter of the article.
Statistics and biometry
No complex statistical methods were necessary for this paper.
Informed consent
Written informed consent was waived by the Institutional Review Board.
Ethical approval
Institutional Review Board approval was obtained.
Methodology
• retrospective
• diagnostic or prognostic study
• performed at one institution
Additional information
Publisher’s note
Springer Nature remains neutral with regard to jurisdictional claims in published maps and institutional affiliations.
Rights and permissions
About this article
Cite this article
Zheng, W., Guo, W., Xiong, M. et al. Clinic-radiological features and radiomics signatures based on Gd-BOPTA-enhanced MRI for predicting advanced liver fibrosis. Eur Radiol 33, 633–644 (2023). https://doi.org/10.1007/s00330-022-08992-0
Received:
Revised:
Accepted:
Published:
Issue Date:
DOI: https://doi.org/10.1007/s00330-022-08992-0