Abstract
This paper proposes an integrated intelligent system that builds a fault diagnosis inference model based on the advantage of rough set theory and genetic algorithms (GAs). Rough set theory is a novel data mining approach that deals with vagueness and can be used to find hidden patterns in data sets. Based on this approach, minimal condition variable subsets and induction rules are established and illustrated using an application for motherboard electromagnetic interference (EMI) test fault diagnosis. This integrated system successfully integrated the rough set theory for handling uncertainty with a robust search engine, GA. The result shows that the proposed method can reduce the number of conditional attributes used in motherboard EMI fault diagnosis and maintain acceptable classification accuracy. The average diagnostic accuracy of 80% shows that this hybrid model is a promising approach to EMI diagnostic support systems .
Similar content being viewed by others
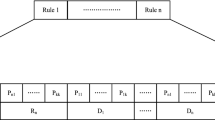
References
Archambeault B, Connor S (2002) Measurements and simulations for ground-to-ground plane noise for SDRAM and DDR RAM daughter cards and motherboards for EMI emissions. Proceedings of the IEEE International Symposium on Electromagnetic Compatibility, Minneapolis, Minnesota, USA, 2002, pp 105–108
Morgan D (1994) A handbook for EMC testing and measurement (IEEE Electrical Measurement Series 8). Peter Peregrinus, London
Raza I (2000) Containing emissions from a microprocessor module. Proceedings of the IEEE International Symposium on Electromagnetic Compatibility, Washington, DC, USA, 2000, 2:871–876
Mills JP (1993) Electromagnetic interference. Prentice-Hall, New York
Lankford M, Davis K, Johnson RW, Baginski ME, Hontgas H, Slattery K, Newberry R, Evans J (1997) Investigation of design and performance of multichip modules including electromagnetic compatibility. Proceedings of the 6th International Conference on Multichip Modules, Denver, CO, USA, 1997, pp 187–192
Kusiak A (2001) Rough set theory: a data mining tool for semiconductor manufacturing. IEEE Trans Electron Packag Manuf 24(1):44–50
Han B, Wu TJ (2001) Data mining in multisensor system base on rough set theory. Proceedings of the American Control Conference, Arlington, VA, USA, 2001, 6:4427–4431
Han J, Micheline K (2001) Data mining: concepts and techniques. Kaufmann, San Francisco, CA
Gardner R, Bieker J (2000) Solving tough semiconductor manufacturing problems using data mining. IEEE/SEMI Advanced Semiconductor Manufacturing Conference, Boston, MA, USA, pp 46–55, 2000
Abidi SSR, Hoe KM (2002) Symbolic exposition of medical data-sets: a data mining workbench to inductively derive data-defining symbolic rules. Proceedings of the 15th IEEE Symposium on Computer-based Medical Systems, Maribor, Slovenia, 2002, pp 123–128
Bayardo RJ, Rakesh A, Dimitrios G (1999) Constraint-based rule mining in large, dense databases. Proceedings of the 15th International Conference on Data Engineering, Sydney, Australia, pp 188–197
Zhai LY, Khoo LP (2001) A prototype genetic algorithm-enhanced rough set-based rule induction system. Comput Ind 46(1):95–106
Ahn BS, Cho SS, Kim CY (2000) The integrated methodology of rough set theory and artificial neural network for business prediction. Expert Syst Appl 18(2):65–74
Zhai LY, Khoo LP, Fok SC (2002) Feature extraction using rough set theory and genetic algorithms – an application for the simplification of product quality evaluation. Comput Ind Eng 43(4):661–676
Goldberg DE (1989) Genetic algorithms in search, optimization and machine learning. Addison-Wesley, Boston
Pawlak Z (1982) Rough sets. Int J Inf Comput Sci 11(5):341–356
Pawlak Z (1984) Rough classification. Int J Man Mach Stud 20:469–483
Pawlak Z (2002) Rough set approach to knowledge-based decision support. Eur J Oper Res 99(1):48–57
Pawlak Z (1999) Rough set theory for intelligent industrial application. Proceedings of the Second International Conference on Intelligent Processing and Manufacturing of Materials, Honululu, Hawaii, USA, 1:37–44
Shen L, Tay FEH, Qu LS, Shen Y (2000) Fault diagnosis using rough sets theory. Comput Ind 43(1):61–72
Wu Z (2001) Research on remote sensing image classification using neural network based on rough sets. Proceedings of 2001 International Conferences on Info-tech and Info-net, Oct 2001, Beijing, China, pp 279–284
Wang B, Chen S, Lin T (2002) Rough set based knowledge acquiring method in intelligent detecting system for lack of weld. Proceedings of the 4th World Congress on Intelligent Control and Automation, Shanghai, China, 2002, pp 2887–2891
Questier F, Arnaut-rollier I, Walczak B, Massart DL (2002) Application to rough set theory to feature selection for unsupervised clustering. Chemometrics Intell Lab Syst 63(2):155–167
Khoo LP, Tor SB, Zhai LY (1999) A rough-set based approach for classification and rule induction. Int J Adv Manuf Technol 15:438–444
Slowinski R, Stefanowski J (1994) Rough classification with valued closeness relation. In: Diday E, Lechevallier Y, Schader M, Bertrand P, Burtschy B (eds) New approaches in classification and data analysis. Springer, Berlin Heidelberg New York
Walczak B, Massart DL (1999) Rough sets theory. Chemometrics Intell Lab Syst 47(1):1–16
Norwegian University of Science and Technology (2001) ROSETTA version 1.4.41. http://www.idi.ntnu.no/∼aleks/rosetta/. Cited 5 Dec 2001
Vinterbo S, Ohrn A (2000) Minimal approximate hitting sets and rule templates. Int J Approximate Reasoning 25(2):123–143
Bruha I (1997) Quality of decision rules: definitions and classification schemes for multiple rules. In: Nakhaeizadeh G, Taylor CC (eds) Machine learning and statistics: the interface. Wiley, New York, pp 107–131
Author information
Authors and Affiliations
Corresponding author
Rights and permissions
About this article
Cite this article
Huang, C., Li, T. & Peng, T. A hybrid approach of rough set theory and genetic algorithm for fault diagnosis. Int J Adv Manuf Technol 27, 119–127 (2005). https://doi.org/10.1007/s00170-004-2142-3
Received:
Accepted:
Published:
Issue Date:
DOI: https://doi.org/10.1007/s00170-004-2142-3