Abstract
Spontaneous reporting of suspected adverse drug reactions (ADRs) has long been a cornerstone of pharmacovigilance. With the increasingly large volume of ADRs, regulatory agencies, scientific/academic organizations and marketing authorization holders have applied statistical tools to assist in signal detection by identifying disproportionate reporting relationships in spontaneous reporting databases. These tools have generated large numbers of signals defined as drug-ADR reporting associations that meet specified statistical criteria.
The challenge is to identify which signals are most likely to be medically important and therefore warrant priority for further investigation. Decisions related to signal triage are often complex and are based on a combination of clinical, epidemiological, pharmacological and regulatory criteria. There are no specific regulations, guidelines or standards that provide an objective basis for these decisions.
This paper describes preliminary work to identify and quantify the specific factors that contribute to a decision to prioritize a specific drug-ADR combination for further in-depth review. We applied a tool from the discipline of decision analysis to systematically assess the important attributes of spontaneously reported ADRs. A model was created that integrates these assessments and produces rankings for the signals generated from quantitative signalling methods. Although more research is necessary to evaluate the performance of this model fully, preliminary results suggest that the use of formal decision analysis approaches to support signal triage can provide potential benefit and will help meet an important need.








Similar content being viewed by others
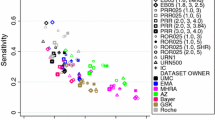
References
Bate A, Linquist M, Edwards IR, et al. A Bayesian neural network method for adverse drug reaction signal generation. Eur J Clin Pharmacol 1998; 54: 315–21
Evans SJW, Waller PC, Davis S. Use of proportional reporting ratios (PRRs) for signal generation from spontaneous adverse drug reaction reports. Pharmacoepidemiol Drug Saf 2001; 10: 483–6
Szarfman A, Machado SG, O’Neill RT. Use of screening algorithms and computer systems to efficiently signal higher-than-expected combinations of drugs and events in the US FDA’s spontaneous reports database. Drug Saf 2002; 25(6): 381–92
Eudravigilance Expert Working Group. Draft Guideline on the use of statistical signal detection methods in the Eudravigilance data analysis system. London: European Medicines Agency; 2006 Nov 16. EMEA/106464/2006
Purcell P, Barty S. Statistical techniques for signal generation: the Australian experience. Drug Saf 2002; 25(6): 415–21
Almenoff J, Tonning A, Gould L, et al. Perspectives on the use of data mining in pharmacovigilance. Drug Saf 2005; 28(11): 981–1007
Stahl M, Lindquist M, Edwards IR, et al. Introducing triage logic as a new strategy for the detection of signals in the WHO drug monitoring database. Pharmacoepidemiol Drug Saf 2004; 13: 355–63
Waller P, Lee E. Responding to drug safety issues. Pharmacoepidemiol Drug Saf 1999; 8(7): 535–52
Clark JA. Algorithms. In: Mann RD, Andrews EB, editors. Pharmacovigilance. 1st ed. Chichester: John Wiley & Sons, 2002: 229–46
Holden WL, Juhaeri J, Dai W. Benefit-risk analysis: a proposal using quantitative methods. Pharmacoepidemiol Drug Saf 2003; 12: 611–6
Holden WL, Juhaeri J, Dai W. Benefit-risk analysis: examples using quantitative methods. Pharmacoepidemiol Drug Saf 2003; 12: 693–7
Waller P, Heeley E, Moseley J. Impact analysis of signals detected from spontaneous adverse drug reaction reporting data. Drug Saf 2005; 28(1): 843–50
Heeley E, Waller P, Moseley J. Testing and implementing signal impact analysis in a regulatory setting: results of a pilot study. Drug Saf 2005; 28(10): 901–6
Kirkwood CE. Strategic decision making: multiobjective decision analysis with spreadsheets. Belmont (CA): Duxbury Press, 1996
Keeney RL. Value-focused thinking: a path to creative decisionmaking. Cambridge (MA): Harvard University Press, 1996
Keeney RL, Raiffa H. Decisions with multiple objectives: preferences and value tradeoffs. Cambridge: Cambridge University Press, 1993
Yee CL, Klincewicz SL, Knight JF, et al. Practical considerations in developing an automated signaling program within a pharmacovigilance department. Drug Inf J 2004; 38: 293–300
Safety reporting requirements for human drug and biological products: proposed rule. Federal Register 2003 Mar 14; 67 (50) [online]. Available from URL: http://www.fda.gov/Cber/rules/safereport.htm [Accessed 2008 Jul 8]
Hogg R, Craig A. Introduction to mathematical statistics. 5th ed. Englewood Cliffs (NJ): Prentice Hall, 1994
Kendall MG. Rank correlation methods. 4th ed. London: Griffin, 1970
Siegel S, Castellan N. Nonparametric statistics for the behavioral sciences. 2nd ed. New York: McGraw-Hill, 1988
Landis J, Koch G. The measurement of observer agreement for categorical data. Biometrics 1977; (33): 159–74
Landrum MB, Normand ST. Applying Bayesian ideas to the development of medical guidelines. StatMed 1999; 18:117–37
Acknowledgements
All authors were full-time employees of Johnson & Johnson at the time of the completed work. No sources of funding were used in the preparation of this article. The authors have no conflicts of interest that are directly relevant to the content of this article. We would like to acknowledge the important contributions of other project team members to this work: Karen Kaplan, Rezaul Karim, Kenneth Kwong, Anders Lindholm, Aparna Mohan, Stanley Music, Mary Panaccio and Saurabh Raman.
Author information
Authors and Affiliations
Corresponding author
Electronic supplementary material
Rights and permissions
About this article
Cite this article
Levitan, B., Yee, C.L., Russo, L. et al. A Model for Decision Support in Signal Triage. Drug-Safety 31, 727–735 (2008). https://doi.org/10.2165/00002018-200831090-00001
Published:
Issue Date:
DOI: https://doi.org/10.2165/00002018-200831090-00001