Abstract
Online-to-offline (O2O) commerce, e.g., the internet celebrity economy, provides a seamless service experience between online commerce and offline bricks-and-mortar commerce. This type of commerce model is closely related to location-based social networks (LBSNs), which incorporate mobility patterns and human social ties. Personalized point-of-interest (POI) recommendations are crucial for O2O commerce in LBSNs; such recommendations not only help users explore new venues but also enable many location-based services, e.g., the targeting of mobile advertisements to users. However, producing personalized POI recommendations for O2O commerce is highly challenging, since LBSNs involve heterogeneous types of data and the user-POI matrix is very sparse. LBSNs have substantially altered how people interact by sharing a wide range of user information, such as the products and services that users use and the places and events that users visit. To address these challenges in O2O commerce LBSNs, we analyze users’ check-in behaviors in detail and introduce the concept of a heterogeneous information network (HIN). Then, we propose a HIN-based POI recommendation system, which consists of two components: an improved singular value decomposition (SVD++) and factorization machines (FMs). The results of experiments on two real-world O2O commerce websites, namely, Gowalla and Foursquare, demonstrate that our method is more accurate than baseline methods. Additionally, a case study of the bricks-and-mortar brand of internet celebrity indicates that our proposed POI recommendation system can be used to conduct online promotion and purchasing to drive offline marketing and consumption.





Similar content being viewed by others
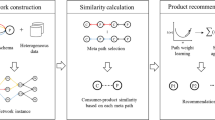
References
Adomavicius, G., & Tuzhilin, A. (2005). Toward the next generation of recommender systems: A survey of the state-of-the-art and possible extensions. IEEE Transactions on Knowledge & Data Engineering, 6, 734–749.
Allen-Zhu, Z., & Yuan, Y. (2016). Improved svrg for non-strongly-convex or sum-of-non-convex objectives. In International Conference on Machine Learning (pp. 1080–1089).
Alperstein, N. M. (2019). The new new sensibility: Selling celebrity/celebrities selling on digital media. In Celebrity and Mediated Social Connections (pp. 95–127). Palgrave Macmillan, Cham.
Baker, J., Parasuraman, A., Grewal, D., & Voss, G. B. (2002). The influence of multiple store environment cues on perceived merchandise value and patronage intentions. Journal of Marketing, 66(2), 120–141.
Bao, J., Zheng, Y., &Mokbel, M. F. (2012). Location-based and preference-aware recommendation using sparse geo-social networking data. In Proceedings of the 20th international conference on advances in geographic information systems (pp. 199–208). ACM.
Bao, J., Zheng, Y., Wilkie, D., & Mokbel, M. (2015). Recommendations in location-based social networks: A survey. GeoInformatica, 19(3), 525–565.
Brockmann, D., Hufnagel, L., & Geisel, T. (2006). The scaling laws of human travel. Nature, 439(7075), 462–465.
CBNData Report. (2018). 2018 Shanghai Restaurant Temperament Study. http://www.cbndata.com/report/1259/detail?isReading=report&isreading=report&page=10&readway=stand.
Chang, E. C., & Woo, T. (2019). The influence of internet celebrities (Wanghongs) on social media users in China. In CERC (pp. 373–379).
Chang, J. R., Chen, M. Y., Chen, L. S., & Chien, W. T. (2019). Recognizing important factors of influencing trust in O2O models: An example of OpenTable. Soft Computing, 1–17.
Cho, E., Myers, S. A., &Leskovec, J. (2011). Friendship and mobility: User movement in location-based social networks. In Proceedings of the 17th ACM SIGKDD international conference on Knowledge discovery and data mining (pp. 1082–1090). ACM.
Duan, R., Jiang, C., Jain, H. K., Ding, Y., & Shu, D. (2019). Integrating geographical and temporal influences into location recommendation: a method based on check-ins. Information Technology and Management, 1–18.
Eirinaki, M., Gao, J., Varlamis, I., & Tserpes, K. (2018). Recommender systems for large-scale social networks: A review of challenges and solutions. Future Generation Computer Systems, 78, 413–418.
Floh, A., & Madlberger, M. (2013). The role of atmospheric cues in online impulse-buying behavior. Electronic Commerce Research and Applications, 12(6), 425–439.
Gao, H., Tang, J., Hu, X., & Liu, H. (2013). Exploring temporal effects for location recommendation on location-based social networks. In Proceedings of the 7th ACM conference on Recommender systems (pp. 93–100). ACM.
Gorgoglione, M., Panniello, U., & Tuzhilin, A. (2019). Recommendation strategies in personalization applications. Information & Management, 56(6), 103143.
Hang, M., Pytlarz, I., & Neville, J. (2018). Exploring student check-in behavior for improved point-of-interest prediction. In Proceedings of the 24th ACM SIGKDD International Conference on Knowledge Discovery & Data Mining (pp. 321–330). ACM.
Hu, Q. Y., Huang, L., Wang, C. D., & Chao, H. Y. (2019). Item orientated recommendation by multi-view intact space learning with overlapping. Knowledge-Based Systems, 164, 358–370.
Huo, H., Liu, X., Zheng, D., Wu, Z., Yu, S., & Liu, L. (2017). Collaborative filtering fusing label features based on SDAE. In Industrial Conference on Data Mining (pp. 223–236). Springer, Cham.
Interdonato, R., Atzmueller, M., Gaito, S., Kanawati, R., Largeron, C., & Sala, A. (2019). Feature-rich networks: Going beyond complex network topologies. Applied Network Science, 4(1), 4.
Jordan, P. W. (2003). Designing pleasurable products: An introduction to the new human factors. CRC press.
Kang, W. C., Wan, M., & McAuley, J. (2018). Recommendation through mixtures of heterogeneous item relationships. In Proceedings of the 27th ACM International Conference on Information and Knowledge Management (pp. 1143–1152). ACM.
Ke, C. K., Wu, M. Y., Ho, W. C., Lai, S. C., & Huang, L. T. (2018). Intelligent point-of-interest recommendation for tourism planning via density-based clustering and genetic algorithm.
Koren, Y. (2008). Factorization meets the neighborhood: A multifaceted collaborative filtering model. In Proceedings of the 14th ACM SIGKDD international conference on Knowledge discovery and data mining (pp. 426–434). ACM.
Kostyra, D. S., Reiner, J., Natter, M., & Klapper, D. (2016). Decomposing the effects of online customer reviews on brand, price, and product attributes. International Journal of Research in Marketing, 33(1), 11–26.
Li, R. (2018). The secret of internet celebrities: A qualitative study of online opinion leaders on Weibo. In 51st Hawaii International Conference on System Sciences (HICSS-51) (pp. 533–542).
Li, H., Hong, R., Zhu, S., & Ge, Y. (2015). Point-of-interest recommender systems: A separate-space perspective. In 2015 IEEE International Conference on Data Mining (pp. 231–240). IEEE.
Liu, B., Fu, Y., Yao, Z., &Xiong, H. (2013). Learning geographical preferences for point-of-interest recommendation. In Proceedings of the 19th ACM SIGKDD international conference on Knowledge discovery and data mining (pp. 1043–1051). ACM.
Meier, L., Van De Geer, S., & Bühlmann, P. (2008). The group lasso for logistic regression. Journal of the Royal Statistical Society: Series B (Statistical Methodology), 70(1), 53–71.
Mnih, A., &Salakhutdinov, R. R. (2008). Probabilistic matrix factorization. In Advances in neural information processing systems (pp. 1257–1264).
Noulas, A., Scellato, S., Mascolo, C., & Pontil, M. (2011). An empirical study of geographic user activity patterns in foursquare. In Fifth international AAAI conference on weblogs and social media.
Pan, Y., Wu, D., & Olson, D. L. (2017). Online to offline (O2O) service recommendation method based on multi-dimensional similarity measurement. Decision Support Systems, 103, 1–8.
Pan, Y., Wu, D., Luo, C., & Dolgui, A. (2019). User activity measurement in rating-based online-to-offline (O2O) service recommendation. Information Sciences, 479, 180–196.
Park, J., & Kim, R. B. (2018). A new approach to segmenting multichannel shoppers in Korea and the US. Journal of Retailing and Consumer Services, 45, 163–178.
Qiao, S., Han, N., Zhou, J., Li, R. H., Jin, C., & Gutierrez, L. A. (2018). SocialMix: A familiarity-based and preference-aware location suggestion approach. Engineering Applications of Artificial Intelligence, 68, 192–204.
Rampell, A. (2010). Why Online2Offline commerce is a trillion dollar opportunity. Techcrunch, August 7.
Rendle, S. (2012). Factorization machines with libfm. ACM Transactions on Intelligent Systems and Technology (TIST), 3(3), 57.
Shen, C. W., Chen, M., & Wang, C. C. (2019). Analyzing the trend of O2O commerce by bilingual text mining on social media. Computers in Human Behavior, 101, 474–483.
Shi, C., Li, Y., Zhang, J., Sun, Y., & Philip, S. Y. (2016). A survey of heterogeneous information network analysis. IEEE Transactions on Knowledge and Data Engineering, 29(1), 17–37.
Shi, C., Hu, B., Zhao, W. X., & Philip, S. Y. (2018). Heterogeneous information network embedding for recommendation. IEEE Transactions on Knowledge and Data Engineering, 31(2), 357–370.
Shi, C., Zhang, Z., Ji, Y., Wang, W., Philip, S. Y., & Shi, Z. (2019). SemRec: a personalized semantic recommendation method based on weighted heterogeneous information networks. World Wide Web, 22(1), 153–184.
Sun, Y., & Han, J. (2013). Mining heterogeneous information networks: A structural analysis approach. AcmSigkdd Explorations Newsletter, 14(2), 20–28.
Sun, Y., Han, J., Yan, X., Yu, P. S., & Wu, T. (2011). Pathsim: meta path-based top-k similarity search in heterogeneous information networks. Proceedings of the VLDB Endowment, 4(11), 992–1003.
Symeonidis, P., Ntempos, D., & Manolopoulos, Y. (2014). Location-based social networks. In Recommender systems for location-based social networks (pp. 35–48). Springer, New York, NY.
Tang, M., & Zhu, J. (2019). Research of O2O website based consumer purchase decision-making model. Journal of Industrial and Production Engineering, 36(6), 371–384.
Tang, L., Cai, D., Duan, Z., Ma, J., Han, M., & Wang, H. (2019). Discovering Travel Community for POI Recommendation on Location-Based Social Networks. Complexity, 2019.
Wang, L., & Yi, B. (2019). Research on O2O take-away restaurant recommendation system: Taking ele. Me APP as an example. Cluster Computing, 22(3), 6069–6077.
Wollenburg, J., Holzapfel, A., Hübner, A., & Kuhn, H. (2018). Configuring retail fulfillment processes for omni-channel customer steering. International Journal of Electronic Commerce, 22(4), 540–575.
Xiao, S., & Dong, M. (2015). Hidden semi-Markov model-based reputation management system for online to offline (O2O) e-commerce markets. Decision Support Systems, 77, 87–99.
Xing, S., Liu, F., Zhao, X., & Li, T. (2018). Points-of-interest recommendation based on convolution matrix factorization. Applied Intelligence, 48(8), 2458–2469.
Xu, X., & Pratt, S. (2018). Social media influencers as endorsers to promote travel destinations: An application of self-congruence theory to the Chinese generation Y. Journal of Travel & Tourism Marketing, 35(7), 958–972.
Xue, X., Hongfang, H., Wang, S., & Qin, C. (2016). Computational experiment-based evaluation on context-aware O2O service recommendation. IEEE Transactions on Services Computing.
Ye, M., Yin, P., & Lee, W. C. (2010). Location recommendation for location-based social networks. In Proceedings of the 18th SIGSPATIAL international conference on advances in geographic information systems (pp. 458–461). ACM.
Yu, L. (2018). A novel E-commerce model and system based on O2O sports community. Information Systems and e-Business Management, 1–21.
Yu, Y., & Chen, X. (2015). A survey of point-of-interest recommendation in location-based social networks. In Workshops at the Twenty-Ninth AAAI Conference on Artificial Intelligence.
Zhang, J. D., & Chow, C. Y. (2015). Geosoca: Exploiting geographical, social and categorical correlations for point-of-interest recommendations. In Proceedings of the 38th International ACM SIGIR Conference on Research and Development in Information Retrieval (pp. 443–452). ACM.
Author information
Authors and Affiliations
Corresponding author
Additional information
Responsible Editor: Ravi S. Sharma
Publisher’s note
Springer Nature remains neutral with regard to jurisdictional claims in published maps and institutional affiliations.
This article is part of the Topical Collection on Recommendation Systems (RS) in Electronic Markets
Appendix
Appendix
Weighted meta path-based similarity
As described in related works, the temporal characteristics of user behavior in O2O commerce LBSNs contain two aspects: periodicity and preference variance. To capture the temporal cyclic pattern, a time-indexing scheme that encodes a standard time stamp to a specified time slot was devised. We consider the preference variance in a two-hierarchy category: time of day and day of the week. Therefore, as shown in Table 6, a week is divided into weekdays and the weekend, while a day is divided into four sessions. Hence, in total, there are 8 time slots, which can represent both weekly and daily preference variances.
Many real-world networks, especially O2O commerce LBSNs, contain attribute values on links. For instance, to represent covisitation events, the time slot is used as the weight for the user-POI link. However, few conventional HINs deal with attribute values on links. In this paper, we employ the concept of WHIN to handle this issue (Shi et al. 2019).
Definition 1.
Weighted heterogeneous information network. A weighted heterogeneous information network is defined as a directed graph G = (V, E, W) with schema \( S=\left(\mathcal{A},\mathcal{R},\mathcal{W}\right) \), where V is the node set, E is the link set, W is the attribute value (weight) set, \( \mathcal{A}=\left\{A\right\} \) is the node type set, \( \mathcal{R}=\left\{R\right\} \) is the link type set, and \( \mathcal{W}=\left\{W\right\} \) is the attribute value type set. Each object v ∈ V maps to an object type \( \varphi (v)\in \mathcal{A} \) by function \( \varphi :V\longrightarrow \mathcal{A} \), each link e ∈ E maps to a relation \( \psi (e)\in \mathcal{R} \) by function \( \varphi :E\longrightarrow \mathcal{R} \), and each attribute value w ∈ W maps to an attribute value type \( \theta (w)\in \mathcal{W} \) via a function \( \uptheta :W\longrightarrow \mathcal{W} \).
With the concept of WHIN, an intuitive strategy is to extend the conventional meta path to deal with attribute values on relations, namely, to a weighted meta path.
Definition 2.
Weighted meta path. A weighted meta path is a meta path that is based on an attribute value constraint on relations; this constraint is denoted as \( {A}_1\overset{\delta_1\left({R}_1\right)}{\to }{A}_2\overset{\delta_2\left({R}_2\right)}{\to}\cdots \overset{\delta_l\left({R}_l\right)}{\to }{A}_{l+1}\mid \mathcal{C} \). The attribute value function δ(R) is a set of values from the attribute value range of relation R. \( {A}_i\overset{\delta_i\left({R}_i\right)}{\to }{A}_{i+1} \) represents the relation Ri between Ai and Ai + 1 that is based on the attribute values δi(Ri). The constraint \( \mathcal{C} \) is a set of correlation constraints among attribute value functions.
Most similarity measures on meta paths are based on matrix multiplication, e.g., counting the probability of a random walk or the number of meta path instances. However, these similarity measures fail to handle the information of attribute value constraints on multiple relations of O2O commerce. To address this problem, we introduce the concept of an atomic meta path and use an ingenious solution to ensure that the existing path-based similarity measure remains available in the weighted meta path.
Definition 3
Atomic meta path. Given a weighted meta path \( \mathcal{P} \), the atomic meta path is a subset of the weighted meta path \( \mathcal{P} \) in which all attribute value functions δ(R) take a specified value. Namely, a weighted meta path is a complete set of atomic meta paths that satisfy the constraint \( \mathcal{C} \).
Given the above definitions, an intuitive idea is to estimate the similarities between the source and the target nodes. In this paper, we employ PathSim (Sun et al. 2011) as the similarity measure, which can identify peer objects in the network. PathSim can be expressed as:
where \( {\mathcal{P}}_{\mathcal{C}} \) is a weighted meta path with attribute value constraint \( \mathcal{C} \), \( {\mathcal{P}}_{\alpha } \) is an atomic meta path of \( {\mathcal{P}}_{\mathcal{C}} \), and \( {p}_{x\rightsquigarrow y}\in {\mathcal{P}}_{\alpha } \)indicates a path instance connecting node x and y along atomic meta path \( {\mathcal{P}}_{\alpha } \). As PathSim counts the number of path instances along the meta path with a normalized term, all users are treated identically. In particular, we should consider the effect of the normalized term in PathSim.
Since a weighted meta path is a combination of corresponding atomic meta paths, we regard the similarity measure that is based on a weighted meta path as the sum of the similarity measures that are based on the corresponding atomic meta paths. Moreover, the PathSim strategy along a weighted meta path can be described as the following steps: Initially, we count the number of path instances along each atomic meta path. Then, we sum the corresponding numbers along every atomic meta path before normalization. Therefore, our solution identifies similar users more accurately because they have the same preferences.
The longer the meta paths are, the less likely they are to generate satisfactory similarity measures because they fail to convey a distinct meaning (Sun et al. 2011). Thus, we employ 7 meaningful meta paths whose lengths are no longer than 4. All the weighted and unweighted meta paths that are used in this paper are listed in Table 7. These meta paths all begin from the source node U (user) and end at the target node P (POI), while intermediate nodes can be interpreted by various latent features in the LBSNs of O2O commerce, e.g., user preferences, temporal effects, and geographical and social influences.
By calculating the similarities between all users and all POIs along the meta paths \( \mathcal{P} \), a user-POI similarity matrix \( \hat{R}\in {\mathbb{R}}^{m\times n} \) is obtained, where \( \hat{R_{ij}} \)represents the similarity between user uiand POIpj, and mand n denote the numbers of users and POIs, respectively. With L meta paths in an O2O commerce LBSN,we can obtain L user-POI similarity matrices that differ in terms of semantics and are denoted by \( {\hat{R}}^1,\cdots, {\hat{R}}^L \).
Latent features in O2O commerce LBSNs
After user-POI similarity matrices have been obtained, we employ matrix factorization (MF) to identify the latent features of users and POIs in O2O commerce. Our MF approach is based on a state-of-the-art model, namely, SVD++, which was proposed by Koren (2008). To alleviate the noise problem and overcome the data sparsity in the user-POI similarity matrices, SVD++ considers user and POI biases and the influence of rated POIs, in addition to the user- and POI-specific vectors, for rating prediction. An SVD++ model associates each user u with a user-factor vector pu ∈ ℝF and each POI j with a POI-factor vectorqj ∈ ℝF. Formally, the rating for user uon POI j is predicted by:
where μ is the global average rating; bu and bj represent the user and POI biases, respectively; Nu is a set of POIs for which user u exhibited an implicit preference; and \( {\mathcal{Y}}_i \)denotes the implicit influence of POIs that have been rated by user u in the past on the ratings of unknown POIs in the future. Thus, the feature vector of user u can also be represented by the set of rated POIs andmodeled as \( \left({p}_u+{\left|{N}_u\right|}^{-\frac{1}{2}}{\sum}_{i\in {N}_u}{\mathcal{Y}}_i\right) \) rather than simply being represented as pu.
Assuming that user preferences in O2O commerce are controlled by few factors, we factor the similarity matrix \( \hat{R} \) into two low-rank matricesby solving the optimizing problem in (5):
where κ is a set of (u, j)pairs for which ruj is known and λupdenotes the hyperparameter that controls the influence of the regularization to avoid overfitting. For L user-POI similarity matrices, we can obtain L groups of latent features of users and POIs; these features are denoted as U(1), ⋯, U(L), P(1), ⋯, P(L).
POI recommendation with factorization machine
With L groups of user and POI latent features in O2O commerce LBSNs, an intuitive strategy is to combine these features linearly to generate ratings. In previous approaches, the rating is predicted by a weighted ensemble of inner products of user-specific and POI-specific vectors from every meta path. However, this conventional approach fails to capture the interactions between and among inter-meta path features, thereby possibly decreasing prediction accuracy.
Therefore, an FM-based method is proposed for generating POI recommendations in O2O commerce LBSNs. First, we concatenate all the user and POI features from L meta paths. The result is denoted by Xn, which represents the feature vector of the n-th sample after concatenation.
where \( {u}_i^l \) and \( {p}_j^l \)represent user and POI features, respectively, that were generated from the l-th meta path. Given all of the latent features in Eq. (6), the formula for the FM is as follows:
where w0is the global bias, Wis the first-order weights for modeling the strength of the latent features, V is the second-order weights for modeling the interactions among latent features, vi represents the i-th variable with K factors, and \( {x}_i^n \)is the i-th feature in Xn.Particularly, d = 2LF denotes the number of latent features that are generated by L meta paths, where F is a constant, namely, the rank that is used to factor every similarity matrix.
Although learning interactions between latent features substantially improves the performance of the recommendation model, the dense feature matrices that are generated by SVD++ increase the computational cost of learning parameters. Simultaneously, several meta paths help improve prediction accuracy only a little because the information that is contained in them can be covered by others. Therefore, it is crucial to select the most discriminating features for high-dimensional data in O2O commerce LBSNs.
To overcome this problem, we employ group lasso regularization for the FM method Meier et al. 2008). The group lasso is an extension of the lasso to variable selection on predefined groups of variables and is especially suitable for high-dimensional problems.
We denote the whole parameter vector by \( \beta ={\left({\beta}_{\upgamma_1}^{\mathrm{T}},\cdots, {\beta}_{\upgamma_{\mathrm{g}}}^{\mathrm{T}},\cdots, {\beta}_{\upgamma_{\mathrm{G}}}^{\mathrm{T}}\right)}^{\mathrm{T}} \). Then, the group lasso regularization is defined as follows:
where ‖∙‖2 is the l2-norm, \( {\beta}_{\gamma_g} \) is the parameter vector that correspondsto the g-th group of variables, and γg is the corresponding index set for g = 1, 2, ⋯G. In this model, the groups are the meta path-based features.
For the first-order parameters Win Eq. (7), we apply the group lasso to wl. Therefore, the regularization can be reformulated as follows:
where wl ∈ ℝF, which models the weights of the latent feature set for the l-th meta path. For the second-order parameters Vin Eq. (7), the corresponding regularizer is
where vl ∈ ℝF × K is the l-th block of V, which corresponds to the features of the l-th meta path; and where ‖∙‖F is the Frobenius norm.
With group lasso regularizations, our model automatically preserves useful and removes redundant features in the unit of groups. Thus, the objective function to minimize is as follows:
where λWand λV are the constants that control the regularization of Wand V, respectively. As the constants increase, the corresponding regularization becomes heavier.
Model optimization
Due to the use of group lasso regularization, the objective function is non-smooth. The objective function is also not convex for V. An improved stochastic variance reduced gradient (SVRG++) can effectively solve non-convex objective functions with a non-smooth ℓ2 regularizer (Allen-Zhu and Yuan 2016). Let us denote the initial vectors by wϕ and vϕ. Then, our algorithm can be divided into S epochs, where the s-th epoch consists of msstochastic gradient steps. Within each epoch, we compute the full gradients \( {\overset{\sim }{\mu}}_{s-1}\longleftarrow \nabla f\left({\overset{\sim }{w}}^{s-1}\right) \) and \( {\overset{\sim }{\mu}}_{s-1}\longleftarrow \nabla f\left({\overset{\sim }{v}}^{s-1}\right) \), where \( {\overset{\sim }{w}}^{s-1} \)and \( {\overset{\sim }{v}}^{s-1} \) are the average points of the previous epochs, consecutively. Moreover, ms doublesevery two consecutive epochs, thereby distinguishing SVRG++ from other variance-reduction-based methods. As shown in line 7, \( {\overset{\sim }{\mu}}_{s-1} \)is used to define the variance-reduced stochastic gradient ξin every stochastic gradient step. Finally, the starting vectors of the next epoch are set as \( {w}_{m_s-1}^{s-1} \)and \( {v}_{m_s-1}^{s-1} \), which is the ending vector of this epoch. SVRG++is presented as Algorithm 1, where η is the step length.

Rights and permissions
About this article
Cite this article
Kang, L., Liu, S., Gong, D. et al. A personalized point-of-interest recommendation system for O2O commerce. Electron Markets 31, 253–267 (2021). https://doi.org/10.1007/s12525-020-00416-5
Received:
Accepted:
Published:
Issue Date:
DOI: https://doi.org/10.1007/s12525-020-00416-5
Keywords
- POI recommendation system
- O2O commerce
- Internet celebrity economy
- Location-based social networks
- Heterogeneous information networks