Abstract
Separating volcanic rocks into groups based on field observations or geochemical data is important for understanding their origins. None of the conventional plotting methods for classification of different rock types are designed to do this. Isotope data may partly improve such classifications, but higher costs are involved. To address this issue, we applied principal component analysis (PCA) and a support vector machine (SVM) to geochemical data in order to separate volcanic rock samples into different groups. The first step used total alkalis–silica classification and Harker diagrams with an SVM to separate samples based on differences in rock type. We then used PCA and SVM together to verify the existence of a particular group of volcanic rocks and its relationship to geological processes. For the studied example, the results quantitatively verify the existence of a volcanic rock group and reveal a possible relationship between ore-forming processes and the recognized volcanic rocks. This study also demonstrates the feasibility of improvement based on some classical plots for quantitative classification of igneous rocks.






Similar content being viewed by others
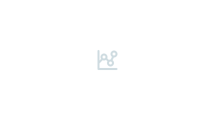
References
Aitchison J (1988) The statistical analysis of compositional data. Technometrics 30(1):120–121
Aitchison J, Greenacre M (2002) Biplots of compositional data. J R Stat Soc 51(4):375–392
An Y, Yang J, Chen WF, Wang JR, Zhang QC, Pan ZX, Jiao S (2017) The revelation of big data: differences between N⁃MORB, E⁃MORB and OIB and their possible causes. Chin J Geol 52(3):16 (in Chinese with English abstract)
Chen CX (1999) Application of data discovery to data processing of geochemistry. J Gl Inst Tech 19(3):230–234 (in Chinese with English abstract)
Cristianini N, Shawe-Taylor J (2000) An introduction to support vector machines: and other kernel-based learning methods. Cambridge University Press, Cambridge, pp 687–689
Cui FH, Zheng CQ, Xu XC, Yao WG, Shi L, Li J, Xu JL (2013) Late carboniferous magmatic activities in the Quanshenglinchang Area, Great Xing’an Range: constrains on the timing of amalgamation between Xing’an and Songnen Massifs. Acta Geol Sin 87(9):1247–1263 (in Chinese with English abstract)
Cui XW, Jiang SH, Li HB, Chang YH (2015) Integrated prospecting model of Erdaohe silver-lead-zinc deposit in the Zhalantun area, Inner Mongolia. Miner Explor 6(6):667–678 (in Chinese with English abstract)
Deng NY, Tian YJ (2004) Support vector machine-a new method of data mining. China Science Publishing and Media Ltd, Beijing, pp 1–408 (in Chinese)
Di PF, Chen WF, Zhang Q, Wang JR, Tang QY, Jiao ST (2018) Comparison of global N-MORB and E-MORB classification schemes. Acta Petrol Sin 34(2):264–274 (in Chinese with English abstract)
Diao H, Zhang D, Di YJ, Wang Z, Wang HR, Xiong GQ (2011) The extraction of alteration anomalies from ASTER data based on principal component analysis and fractal model. Remote Sens Land Resour 23(2):75–80 (in Chinese with English abstract)
Ding SF, Qi BJ, Tan HY (2011) An overview on theory and algorithm of support vector machines. J Univ Elec Sci Tech Chin 40(1):2–10 (in Chinese with English abstract)
Du DW, Yuan YL, Meng XW (2000) Solutions of composition translation in composition data analysis. Geol Rev 46(Z4):364–366 (in Chinese with English abstract)
Garreta R, Moncecchi G (2013) Learning scikit-learn: Machine Learning in Python. Packt Publishing, Birmingham, pp 1–118
Geladi P, Kowalski BR (1985) Partial least-squares regression: a tutorial. Anal Chim Acta 185(86):1–17
Greenacre M (2011) Measuring subcompositional incoherence. Math Geosci 43(6):681–693
Gui Z, Chen JG, Wang CB (2017) Classification of imbalance geological data based on PCA-SMOTE algorithm and random forest: a case study of geochemical data from the eastern Tianshan of China. J Gl Univ Tech 37(4):587–593 (in Chinese with English abstract)
Hunter JD (2007) Matplotlib: a 2D graphics environment. Comput Sci Eng 9(3):90–95
Iwamori H, Yoshida K, Nakamura H, Kuwatani T, Hamada M, Haraguchi S, Ueki K (2017) Classification of geochemical data based on multivariate statistical analyses: complementary roles of cluster, principal component, and independent component analyses. Geochem Geophys Geosyst 18(3):994–1012
Jahn BM (2004) The Central Asian Orogenic Belt and growth of the continental crust in the Phanerozoic. Geol Soc, London, Special Publications 226:73–100
Ji B (2017) Synthetic information mineral prediction research of polymetallic deposit in Haobugao distinct, Inner Mongolia. Hefei University of Technology, Hefei, pp 1–173 (in Chinese with English abstract)
Kramer O (2016) Scikit-learn: machine learning for evolution strategies. Springer International Publishing, Berlin, pp 45–53
Kuwatani T, Nagata K, Okada M, Watanabe T, Ogawa Y, Komai T, Tsuchiya N (2014) Geochemical identification of the tsunami deposit using machine learning machine learning techniques. Japan Geoscience Union meeting
Kuwatani T, Nagata K, Okada M, Watanabe T, Ogawa Y, Komai T, Tsuchiya N (2015) Machine-learning techniques for geochemical discrimination of 2011 Tohoku tsunami deposits. Sci Rep 4(1):7077–7077
Kwiatkowska EJ, Fargion GS (2003) Application of machine-learning techniques toward the creation of a consistent and calibrated global chlorophyll concentration baseline dataset using remotely sensed ocean color data. IEEE Trans Geosci Remote Sens 41(12):2844–2860
Lark RM (2010) Compositional data analysis in the geosciences: from theory to practice. J R Stat Soc 171(1):313–314
Li SG, Wang SG (1983) On applicability of principal component analysis to dividing of geochemical process stages. Sci Geol Sin 2:181–187 (in Chinese with English abstract)
Liu JW (2014a) Research on personal recommendation algorithm based on clustering ensemble of high-dimensional data. South China University of Technology, Guangzhou, p 70 (in Chinese with English abstract)
Liu YJ (2014b) Geological characteristics and peripheral predictions of Erdaohe lead-zinc-silver deposit in Zhalantun area. Inner Mongolia. Jilin University, Changchun, p 84 (in Chinese with English abstract)
Liu Y (2015) The study of regional geochemistry data analysis and metallogenic information fusion models-take the Nanling Metallogenic Belt as an example. China University of Geosciences, Wuhan, p 123 (in Chinese with English abstract)
Liu BJ, Liu TD (2001) Some problems of geology. Adv Earth Sci 16(5):607–616 (in Chinese with English abstract)
Liu JT, Cheng QM, Wang JG (2012) Application of structural equation modeling in geochemical data analysis. Earth Sci J Chin Univ Geosci 37(6):1191–1198 (in Chinese with English abstract)
Liu YJ, Chen YJ, Wu TT, Zhang YF, Zhao HG, Wang CB, Zhu HL, Wu GX (2013) Prediction of concealed ore bodies in periphery of Erdaohe lead-zinc-silver deposit in Chaihe area, Inner Mongolia. Glob Geol 32(3):9
Lv ZC, Hao LB, Duan GZ, Li DC, Lian CY (2000) Geochemical research on Mesozoic volcanic rocks in the Manzhouli-E'erguna region. Acta Mineral Sin 20(4):406–414 (in Chinese with English abstract)
Ma Z, Zhang CL, Gao SC (2017) Lithology identification based on principal component analysis and fuzzy recognition. Lith Res 29(5):127–133 (in Chinese with English abstract)
Mao AQ, Sun DY, Guo J, Li X, Yang AX, Yang DG (2016) Geochemical characteristics and petrogenesis of volcanic rocks from Tamulangou Formation in Erguna region, northern Da Hinggan Mountain. Glob Geol 35(3):641–652
Müller KR, Mika S, Rätsch G, Tsuda K, Schölkopf B (2001) An introduction to kernel-based learning algorithms. IEEE Trans Neural Netw 12(2):181–201
Nie FJ, Liu Y, Liu YF, Jiang SH, Zhang K, Liu Y (2011) Ore-forming processes of silver-polymetallic deposits occurring within Tsav-Jiawula region along China-Mongolian border. J Jl Univ (Earth Sci Ed) 41(6):1715–1725 (in Chinese with English abstract)
Pawlowsky GV, Olea RA (2004) Geostatistical analysis of compositional data. Oxford University Press, Oxford, pp 1–177
Pawlowsky GV, Egozcue JJ, Tolosana DR (2015) Modelling and analysis of compositional data. John Wiley & Sons Ltd, New Jersey, pp 1–252
Pearson K (1895) Note on Regression and inheritance in the case of two parents. Proc R Soc Lond 58:240–242
Pedregosa F, Varoquaux G, Gramfort A, Michel V, Louppe G (2013) Scikit-learn: machine learning in Python. J Mach Learn Res 12(10):2825–2830
Qi HN (2004) Support vector machines and application research overview. Comput Eng 30(10):6–9 (in Chinese with English abstract)
Scull P, Schaetzl RJ (2011) Using PCA to characterize and differentiate loess deposits in Wisconsin and Upper Michigan, USA. Geomorphology 127(3):143–155
Shu Q, Chang Z, Lai Y, Zhou Y, Sun Y, Yan C (2016) Regional metallogeny of Mo-bearing deposits in northeastern China, with new Re–Os dates of porphyry Mo deposits in the northern Xilamulun district. Econ Geol 111:1783–1798
Tang FM, Wang ZD, Chen JY (2005) On multiclass classification methods for support vector machines. Decis Control 20(7):746–749
Tian SL, Jin LF, Shuang B (1995) Ore forming relationship between vein silver (lead, zinc) deposit in E’erguna mineralization belt and Tamulangou Formation volcanic rocks. Geol Explor Non-Ferrous Met 4(6):334–340 (in Chinese with English abstract)
Wang Z, Niu Y, Yao D (2012) Discovery and significance of Ag-polymetallic deposit in Inner Mongolia Erdaohe. J Sd Univ Tech (Nat Sci Ed) 26(1):4 (in Chinese with English abstract)
Wang JR, Chen WF, Zhang Q, Jiao ST, Yang J, Pan ZJ, Wang SH (2017) Preliminary research on data mining of N-MORB and E-MORB: discussion on method of the basalt discrimination diagrams and the character of MORB’s mantle source. Acta Petrol Sin 33(3):993–1005 (in Chinese with English abstract)
Wentworth CK (1936) Is geology a science? Science. 83(2162):550–551
Wu F (2014) Abnormal extraction of geochemical data based on Kalman filter and SVM. Chengdu University of Technology, Chengdu, p 50 (in Chinese with English abstract)
Yang FT (2016) Geologic features of Erdaohe silver polymetallic deposit and its ore finding prediction in Middle Daxing’anling Mountains. China University of Geosciences, Beijing, p 99 (in Chinese with English abstract)
Yang J, Wang JR, Zhang Q, Chen WF, Pan ZJ, Jiao ST, Wang SH (2016) Back-arc basin basalt (BABB) data mining: comparison with MORB and IAB. Adv Earth Sci 31(1):66–77 (in Chinese with English abstract)
Yu QY (2019) Analysis of ore-forming information from high-dimensional data: a case study of Erdaohe polymetallic deposit in Inner Mongolia. China University of Geosciences, Beijing, p 149 (in Chinese with English abstract)
Yu QY, Leon B, Yang PH, Zhang D (2018) GeoPyTool: A cross-platform software solution for common geological calculations and plots. Geosci Front 10(4):1437–1447
Yuan Y, Li SK, Zhou AH (2017) Study on the classification method of igneous rocks based on PCA-GA-SVM. Math Pract Theory 47(12):121–128 (in Chinese with English abstract)
Zhang C (2014) Petrogenesis and tectonic setting of volcanic rocks in manketouebo Formation from Ta’erqi area, central Gret Xing’an Range. Jilin University, Changchun, p 77 (in Chinese with English abstract)
Zhang ZT, Wang ZW, Ma YY, Wang M (2010) Application of support vector machine in geochemical exploration. Glob Geol 29(1):78–82
Zhang B, Li JW, Zhang DQ, Zhu GG, She HQ, Dong YJ, Tan G (2011) Geochemic Features of Dongjun Lead-Zinc-Silver Deposit, Hailar Basin, Inner Mongolia. Geol Rev 57(2):253–260 (in Chinese with English abstract)
Zhang J, Shao J, Jiang S, Yang HZ (2017) A study of the stable isotope geochemistry of Erdaohe Pb-Zn ore deposit in Inner Mongolia. Geol Rev 63(s1):225–227 (in Chinese with English abstract)
Zhao PD (2004) Quantitative geoscience: methods and its applications. Higher Education Press, Beijing, pp 140–141 (in Chinese)
Zhao PD, Meng XG (1992) Quantification and geosciences. Earth Sci J Chin Univ Geosci 17(S1):51–56 (in Chinese with English abstract)
Zhou ZH (2016) Machine Learning. Tsinghua University Press, Beijing, pp 247–263 (in Chinese)
Zhou YZ, Chen S, Zhang Q, Xiao F, Wang SG, Liu YP, Jiao ST (2018a) Advances and prospects of big data and a mathematical geoscience. Acta Petrol Sin 34(2):9 (in Chinese with English abstract)
Zhou SG, Zhou KF, Wang JL, Yang GF, Wang SS (2018b) Application of cluster analysis to geochemical compositional data for identifying ore-related geochemical anomalies. Front Earth Sci Chin 12(3):1–15 (in Chinese with English abstract)
Zhu YS (2009) Multivariate statistical analysis of experimental data. Modern physics basic series, Beijing, pp 1–188 (in Chinese)
Acknowledgments
This work was financially supported by the National Natural Science Foundation of China (No. 41772069), the China Geological Survey Program (No. 1212011085490), the Scientific Research Project of Jilin Provincial Education Department( No. JJKH20210689KJ), the Doctoral Initiation Fund from CCIT for Staff (No. 0215073), and the Geological Survey Achievement Transformation Fund of China University of Geosciences (Beijing).
Author information
Authors and Affiliations
Corresponding author
Ethics declarations
Conflict of interest
The author(s) declare that they have no competing interests.
Additional information
Responsible Editor: Biswajeet Pradhan
Supplementary Information
ESM 1
(DOCX 32.1 kb)
Rights and permissions
About this article
Cite this article
Yu, Q., Zhang, X., Hu, B. et al. Separating volcanic rock groups: a novel method based on principal component analysis and a support vector machine. Arab J Geosci 14, 967 (2021). https://doi.org/10.1007/s12517-021-07299-6
Received:
Accepted:
Published:
DOI: https://doi.org/10.1007/s12517-021-07299-6