Abstract
Purpose
To perform an extensive exploratory analysis to build a deeper insight into clinically relevant molecular biomarkers in Papillary, Follicular, and Anaplastic thyroid carcinomas (PTC, FTC, ATC).
Methods
Thirteen Thyroid Cancer (THCA) datasets incorporating PTC, FTC, and ATC were derived from the Gene Expression Omnibus. Genes differentially expressed (DEGs) between THCA and normal were identified and subjected to GO and KEGG analyses. Multiple topological properties were harnessed and protein-protein interaction (PPI) networks were constructed to identify the hub genes followed by survival analysis and validation.
Results
There were 70, 87, and 377 DEGs, and 23, 27, and 53 hub genes for PTC, FTC, and ATC samples, respectively. Survival analysis detected 39 overall and 49 relapse-free survival-relevant hub genes. Six hub genes, BCL2, FN1, ITPR1, LYVE1, NTRK2, TBC1D4, were found common to more than one THCA type. The most significant hub genes found in the study were: BCL2, CD44, DCN, FN1, IRS1, ITPR1, MFAP4, MKI67, NTRK2, PCLO, TGFA. The most enriched and significant GO terms were Melanocyte differentiation for PTC, Extracellular region for FTC, and Extracellular exosome for ATC. Prostate cancer for PTC was the most significantly enriched KEGG pathway. The results were validated using TCGA data.
Conclusions
The findings unravel potential biomarkers and therapeutic targets of thyroid carcinomas.




Similar content being viewed by others
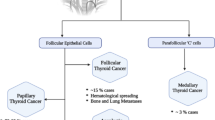
Data availability
Raw files of the datasets used in the study can be found in GEO database (https://www.ncbi.nlm.nih.gov/geo/query/acc.cgi) by using the corresponding accession numbers: GSE3467, GSE3678, GSE5364, GSE9115, GSE27155, GSE29265, GSE33630, GSE53072, GSE53157, GSE58545, GSE60542, GSE65144, GSE171011. The dataset corresponding to the TCGA-THCA project can be found at https://portal.gdc.cancer.gov/projects/TCGA-THCA. We accessed the datasets on 30 October 2023. The result files and the code scripts can be downloaded from https://github.com/code-rishav/bioinformatics.
References
C.C. Juhlin, O. Mete, Z.W. Baloch, The 2022 who classification of thyroid tumors: novel concepts in nomenclature and grading. Endocrine-Related Cancer 30, e220293 (2023).
S. Wang, J. Wu, C. Guo et al. Identification and validation of novel genes in anaplastic thyroid carcinoma via bioinformatics analysis. Cancer Manag. Res. 9787–9799 (2020)
Y. Shen, S. Dong, J. Liu et al. Identification of potential biomarkers for thyroid cancer using bioinformatics strategy: a study based on geo datasets. BioMed. Res. Int. 2020 (2020)
L. Liu, C. He, Q. Zhou et al. Identification of key genes and pathways of thyroid cancer by integrated bioinformatics analysis. J. Cellular Physiol. 234, 23647–23657 (2019)
J. Tang, D. Kong, Q. Cui et al. Bioinformatic analysis and identification of potential prognostic micrornas and mrnas in thyroid cancer. PeerJ 6, e4674 (2018)
R. Edgar, M. Domrachev, A.E. Lash, Gene expression omnibus: Ncbi gene expression and hybridization array data repository. Nucleic Acids Res. 30, 207–210 (2002)
Oliveros, J. C. Venny. an interactive tool for comparing lists with venn diagrams. http://bioinfogp.cnb.csic.es/tools/venny/index.html (2007)
A. Franceschini, D. Szklarczyk, S. Frankild et al. String v9. 1: protein-protein interaction networks, with increased coverage and integration. Nucleic Acids Res. 41, D808–D815 (2012)
P. Shannon, A. Markiel, O. Ozier et al. Cytoscape: a software environment for integrated models of biomolecular interaction networks. Genome Res. 13, 2498–2504 (2003)
C.-H. Chin, S.H. Chen, H.H. Wu et al. cytohubba: identifying hub objects and sub-networks from complex interactome. BMC Syst. Biol. 8, 1–7 (2014)
B.T. Sherman, M. Hao, J. Qiu et al. David: a web server for functional enrichment analysis and functional annotation of gene lists (2021 update). Nucleic Acids Res. 50, W216–W221 (2022)
D. Tang, M. Chen, X. Huang et al. Srplot: A free online platform for data visualization and graphing. PLoS One 18, e0294236 (2023)
Á. Nagy, G. Munkácsy, B. Győrffy, Pancancer survival analysis of cancer hallmark genes. Sci. Rep. 11, 6047 (2021)
N. Agrawal, R. Akbani, B.A. Aksoy et al. Integrated genomic characterization of papillary thyroid carcinoma. Cell 159, 676–690 (2014)
Á. Bartha, B. Győrffy, Tnmplot. com: a web tool for the comparison of gene expression in normal, tumor and metastatic tissues. Int. J. Mol. Sci. 22, 2622 (2021)
T. Knight, D. Luedtke, H. Edwards et al. A delicate balance–the bcl-2 family and its role in apoptosis, oncogenesis, and cancer therapeutics. Biochem. Pharmacol. 162, 250–261 (2019)
V.K. Anagnostou, F.J. Lowery, V. Zolota et al. High expression of bcl-2 predicts favorable outcome in non-small cell lung cancer patients with non squamous histology. BMC Cancer 10, 1–11 (2010)
S. Wu, F. Wu, Z. Jiang, Identification of hub genes, key mirnas and potential molecular mechanisms of colorectal cancer. Oncol. Rep. 38, 2043–2050 (2017)
W. Liang, F. Sun, Identification of key genes of papillary thyroid cancer using integrated bioinformatics analysis. J. Endocrinol. Investig. 41, 1237–1245 (2018)
Y. Liu, S. Gao, Y. Jin et al. Bioinformatics analysis to screen key genes in papillary thyroid carcinoma. Oncol. Lett. 19, 195–204 (2020)
X. Li, J. He, M. Zhou et al. Identification and validation of core genes involved in the development of papillary thyroid carcinoma via bioinformatics analysis. Int. J. Genomics 2019 (2019).
A. Almansoori, P. M. Bhamidimarri, R. Bendardaf et al. Identifying diagnostic and prognostic targets for papillary thyroid carcinoma through mining gene expression big datasets using adaptive filtering and advanced bioinformatics algorithms. In 2021 14th International Conference on Developments in eSystems Engineering (DeSE), 358–363 (IEEE, 2021).
Y.-X. Wang, L. Zhao, X.-Y. Wang et al. Role of caspase 8, caspase 9 and bcl-2 polymorphisms in papillary thyroid carcinoma risk in Han Chinese population. Med. Oncol. 29, 2445–2451 (2012)
A.R. Jordan, R.R. Racine, M.J. Hennig et al. The role of cd44 in disease pathophysiology and targeted treatment. Front. Immunol. 6, 182 (2015)
K. Xie, A biomarker and molecular mechanism investigation for thyroid cancer. Central Eur. J. Immunol. 48, 203–218 (2023)
H.-N. Li, H.M. Zhang, X.R. Li et al. Mir-205-5p/ggct attenuates growth and metastasis of papillary thyroid cancer by regulating cd44. Endocrinology 163, bqac022 (2022)
T. Kawai, K. Iwata, Y. Shinotsuka et al. Cd44v8-10 and cd44s are age-dependently expressed in primary cultured papillary thyroid carcinoma cells and are associated with cell proliferation. Kobe J. Med. Sci. 65, E1 (2019)
T. Okada, T. Nakamura, T. Watanabe et al. Coexpression of epcam, cd44 variant isoforms and claudin-7 in anaplastic thyroid carcinoma. PLoS One 9, e94487 (2014)
Z. Wang, Q. Wang, Q. Wang et al. Prognostic significance of cd24 and cd44 in breast cancer: a meta-analysis. Int. J. Biol. Mark. 32, 75–82 (2017)
S. Goldoni, R.V. Iozzo, Tumor microenvironment: modulation by decorin and related molecules harboring leucine-rich tandem motifs. Int. J. Cancer 123, 2473–2479 (2008)
L. Arnaldi, R. Borra, R. Maciel et al. Gene expression profiles reveal that dcn, dio1, and dio2 are underexpressed in benign and malignant thyroid tumors. Thyroid 15, 210–221 (2005)
Y. Wan, X. Zhang, H. Leng et al. Identifying hub genes of papillary thyroid carcinoma in the tcga and geo database using bioinformatics analysis. PeerJ 8, e9120 (2020)
R.I. Lieverse, D. Marcus, A.M. van der Wiel et al. Human fibronectin extra domain b as a biomarker for targeted therapy in cancer. Mol. Oncol. 14, 1555–1568 (2020)
T. Qu, Y.-P. Li, X.-H. Li et al. Identification of potential biomarkers and drugs for papillary thyroid cancer based on gene expression profile analysis. Mol. Med. Rep. 14, 5041–5048 (2016)
Q.-S. Geng, T. Huang, L.F. Li et al. Over-expression and prognostic significance of fn1, correlating with immune infiltrates in thyroid cancer. Front. Med. 8, 812278 (2022)
J. Han, M. Chen, Y. Wang et al. Identification of biomarkers based on differentially expressed genes in papillary thyroid carcinoma. Sci. Rep. 8, 9912 (2018)
G. Xue, X. Lin, J.F. Wu et al. Identification of key genes of papillary thyroid carcinoma by integrated bioinformatics analysis. Biosci. Rep. 40, BSR20201555 (2020)
R. Fan, L. Dong, P. Li et al. Integrated bioinformatics analysis and screening of hub genes in papillary thyroid carcinoma. Plos One 16, e0251962 (2021)
Z. Li, C. Xiong, J. Wei et al. Identification of potential key genes in anaplastic thyroid cancer using bioinformatics analysis. Blood&Genomics 6, 53–61 (2022)
J. Wang, L. Wu, W. Lu et al. Identification of key pathways and genes downstream of insulin-like growth factor 1 in thyroid carcinoma. Genetic Testing Mol. Biomarkers 26, 522–531 (2022)
F. Yu, D. Huang, Y. Kuang, et al. Irs1 promotes thyroid cancer metastasis through emt and pi3k/akt pathways. Clin. Endocrinol. 100, 284–293 (2024).
Q. Xu, A. Song, Q. Xie, The integrated analyses of driver genes identify key biomarkers in thyroid cancer. Technol. Cancer Res. Treat. 19, 1533033820940440 (2020)
G. Hu, H.-f Feng, H. Zhan, Identification of an autophagy-related signature predicting overall survival for papillary thyroid carcinoma. Dose-Response 18, 1559325819899265 (2020)
D. Peng, W. Li, B. Zhang et al. Overexpression of lncrna slc26a4-as1 inhibits papillary thyroid carcinoma progression through recruiting ets1 to promote itpr1-mediated autophagy. J. Cell. Mol. Med. 25, 8148–8158 (2021)
Y. Hu, N. Zhou, R. Wang, Apatinib strengthens the anti-tumor effect of cisplatin in thyroid carcinoma through downregulating vegfr2. J BUON 26, 613–9 (2021)
M.-L. Tsai, C.H. Lee, L.C. Huang et al. Crispr-mediated knockout of vegfr2/kdr inhibits cell growth in a squamous thyroid cancer cell line. FEBS Open Bio. 12, 993–1005 (2022)
M. Wu, H. Yuan, X. Li et al. Identification of a five-gene signature and establishment of a prognostic nomogram to predict progression-free interval of papillary thyroid carcinoma. Front. Endocrinol. 10, 790 (2019)
L.K. Dalkilic, S. Dalkilic, Identification of key genes involved in papillary thyroid cancer by bioinformatics tools. Progr. Nutr. 33, e2020014 (2020).
H.S. Kim, D.H. Kim, J.Y. Kim et al. Microarray analysis of papillary thyroid cancers in korean. Kor. J. Internal Med. 25, 399 (2010)
J. Li, J. Wang, Z. Liu et al. Tumor-suppressive role of microfibrillar associated protein 4 and its clinical significance as prognostic factor and diagnostic biomarker in hepatocellular carcinoma. J. Cancer Res. Ther. 18, 1919–1925 (2022)
R. Yerushalmi, R. Woods, P.M. Ravdin et al. Ki67 in breast cancer: prognostic and predictive potential. The Lancet Oncol. 11, 174–183 (2010)
S. Agarwal, A. Bychkov, C.-K. Jung, Emerging biomarkers in thyroid practice and research. Cancers 14, 204 (2021)
Y. Han, K. Xia, T. Su, Exploration of the important role of microfibril-associated protein 4 gene in oral squamous cell carcinoma. Med. Sci. Monit.: Int. Med. J. Exp. Clin. Res. 27, e931238–1 (2021)
M.Y. Ahmed, B.A. Chioza, A. Rajab et al. Loss of pclo function underlies pontocerebellar hypoplasia type iii. Neurology 84, 1745–1750 (2015)
L. Shi, Y. Huang, X. Huang et al. Analyzing the key gene expression and prognostics values for acute myeloid leukemia. Transl. Cancer Res. 9, 7284 (2020)
Y. Zhang, Z. Qiu, L. Wei et al. Integrated analysis of mutation data from various sources identifies key genes and signaling pathways in hepatocellular carcinoma. PloS One 9, e100854 (2014)
M. Bernhardt, H.-M. Behrens, S. Krüger et al. Exploration of the tumor biological significance of pclo in gastric cancer-results from a large central european cohort. Pathobiol. J. Immunopathol. Mol. Cellular Biol. 1–9 (2023) (Online ahead of print).
R. Sun, Y. Yang, W. Lü et al. Single-cell transcriptomic analysis of normal and pathological tissues from the same patient uncovers colon cancer progression. Cell Biosci. 13, 1–15 (2023)
O.L. Griffith, A. Melck, S.J. Jones et al. Meta-analysis and meta-review of thyroid cancer gene expression profiling studies identifies important diagnostic biomarkers. J. Clin. Oncol. 24, 5043–5051 (2006)
D. Degl’Innocenti, C. Alberti, G. Castellano et al. Integrated ligand-receptor bioinformatic and in vitro functional analysis identifies active tgfa/egfr signaling loop in papillary thyroid carcinomas. PLoS One 5, e12701 (2010)
E. Molinaro, C. Romei, A. Biagini et al. Anaplastic thyroid carcinoma: from clinicopathology to genetics and advanced therapies. Nat. Rev. Endocrinol. 13, 644–660 (2017)
Author contributions
S.A. and S.G. contributed to the study conception and design. Material preparation, data collection, and analysis were performed by all the authors. The first draft of the manuscript was written by S.G. and R.R. and all authors commented on previous versions of the manuscript. All authors read and approved the final manuscript.
Author information
Authors and Affiliations
Corresponding author
Ethics declarations
Conflict of interest
The authors declare no competing interests.
Additional information
Publisher’s note Springer Nature remains neutral with regard to jurisdictional claims in published maps and institutional affiliations.
Supplementary information
Rights and permissions
Springer Nature or its licensor (e.g. a society or other partner) holds exclusive rights to this article under a publishing agreement with the author(s) or other rightsholder(s); author self-archiving of the accepted manuscript version of this article is solely governed by the terms of such publishing agreement and applicable law.
About this article
Cite this article
Agarwal, S., Gupta, S. & Raj, R. Identification of potential targetable genes in papillary, follicular, and anaplastic thyroid carcinoma using bioinformatics analysis. Endocrine (2024). https://doi.org/10.1007/s12020-024-03836-x
Received:
Accepted:
Published:
DOI: https://doi.org/10.1007/s12020-024-03836-x