Abstract
Energy efficiency is considered the prominent metric in data communication systems and has a greater impact on designing Millimetre-wave (mm-wave) multiple input multiple output (MIMO) systems. As the recent mm-wave system relies upon the number of radiofrequency (RF) chains, effective RF chains are preferred to maximize overall system performance, energy and spectral efficiency. Degraded system efficiency and increased path loss are the major limitations faced in MIMO systems. This harms the data rate transmission between transmitter and receiver antennas. Hence, an effectual mm-wave with a massive MIMO model is presented in this research by adopting standard techniques. The chief objective of this research is to maximize energy and spectral efficiency with an appropriate selection of RF chains. The optimal RF chains are selected through a hybrid optimization model called the differential evolution firefly selection model (DEA + FOA). After selecting optimal RF chains, an appropriate precoding scheme is used to route the transmitted data over the preferred direction by overcoming the path-loss issues. In the precoding step, the successive interference cancellation based technique is employed to optimize the RF chain's sub-capacity and increase the system efficiency. The performance of a proposed model is analyzed by evaluating the energy and spectral efficiency by varying the number of RF chains at low and high signal-to-noise ratio rates. The proposed optimization and precoding techniques are compared with existing approaches to analyze the efficacy of a proposed MIMO system. The proposed model has proven extremely effective in transforming data from transmitter to receiver with increased system efficiency.












Similar content being viewed by others
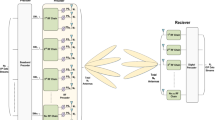
Data Availability
Data sharing does not apply to this article.
References
Nouri, M., Behroozi, H., Bastami, H., Moradikia, M., Jafarieh, A., Abdelhadi, A., & Han, Z. (2023). Hybrid precoding based on active learning for mmWave massive MIMO communication systems. IEEE Transactions on Communications., 71, 3043–3058.
Khalid, S., Abbas, W. B., Kim, H. S., & Niaz, M. T. (2020). Evolutionary algorithm based capacity maximization of 5G/B5G hybrid precoding systems. Sensors, 20(18), 5338.
Suneetha, N., & Satyanarayana, P. (2023). Intelligent channel estimation in millimeter wave massive MIMO communication system using hybrid deep learning with heuristic improvement. International Journal of Communication Systems, 36(5), e5400.
Ravikumar, C. V. (2023). Developing novel channel estimation and hybrid precoding in millimeter-wave communication system using heuristic-based deep learning. Energy, 268, 126600.
Vlachos, E., & Thompson, J. (2020). Energy-efficiency maximization of hybrid massive MIMO precoding with random-resolution DACs via RF selection. IEEE Transactions on Wireless Communications, 20(2), 1093–1104.
Khalid, S., Mehmood, R., Abbas, W., Khalid, F., & Naeem, M. (2021). Probabilistic distribution learning algorithm based transmit antenna selection and precoding for millimeter wave massive MIMO systems. Telecommunication Systems, 76(3), 449–460.
Li, X., Huang, Y., Heng, W., & Wu, J. (2021). Machine learning-inspired hybrid precoding for mmWave MU-MIMO systems with domestic switch network. Sensors, 21(9), 3019.
Sharifi, S., Shahbazpanahi, S., & Dong, M. (2021). A POMDP-based antenna selection for massive MIMO communication. IEEE Transactions on Communications, 70(3), 2025–2041.
Kumar, S., Mahapatra, R., & Singh, A. (2023). Multi-user mmWave massive-MIMO hybrid beamforming: A quantize deep learning approach. In 2023 National Conference on Communications (NCC) (pp. 1–6). IEEE.
Yang, X., Jin, S., Li, G. Y., & Li, X. (2021). Asymmetrical uplink and downlink transceivers in massive MIMO systems. IEEE Transactions on Vehicular Technology, 70(11), 11632–11647.
Yetis, C. M., Björnson, E., & Giselsson, P. (2021). Joint analog beam selection and digital beamforming in millimeter wave cell-free massive mimo systems. IEEE Open Journal of the Communications Society, 2, 1647–1662.
Salh, A., Shah, N.S.M., Audah, L., Abdullah, Q., Abdullah, N., Hamzah, S.A., & Saif, A. (2021). Trade-off energy and spectral efficiency in 5G massive MIMO system. arXiv preprint arXiv:2105.10722.
Liu, P., Li, Y., Cheng, W., Gao, X., & Huang, X. (2021). Intelligent reflecting surface aided NOMA for millimeter-wave massive MIMO with lens antenna array. IEEE Transactions on Vehicular Technology, 70(5), 4419–4434.
Byreddy, A. R., & Logashanmugam, E. (2023). Energy and spectral efficiency improvement using improved shark smell-coyote optimization for massive MIMO system. International Journal of Communication Systems, 36, e5381.
He, Y., & M.S., Zeng, F., Zheng, H., Wang, R., Zhang, M. and Liu, X. (2021). Energy efficient power allocation for cell-free mmWave massive MIMO with hybrid precoder. IEEE Communications Letters, 26(2), 394–398.
Zhang, Y., Cheng, Y., Zhou, M., Yang, L., & Zhu, H. (2020). Analysis of uplink cell-free massive MIMO system with mixed-ADC/DAC receiver. IEEE Systems Journal, 15, 5162–5173.
Yang, J., Zhang, L., Zhu, C., Guo, X., & Zhang, J. (2021). Energy efficiency optimization of massive MIMO systems based on the particle swarm optimization algorithm. Wireless Communications and Mobile Computing, 2021, 1–11.
Bouchibane, F. Z., & Bensebti, M. (2018). Artificial bee colony algorithm for energy efficiency optimization in massive MIMO system. International Journal of Wireless and Mobile Computing, 15(2), 97–104.
Fountoukidis, K. C., Kalialakis, C., Psannis, K. E., Siakavara, K., Goudos, S. K., Sarigiannidis, P., & Obaidat, M. (2018). MIMO antenna selection using biogeography-based optimization with nonlinear migration models. International Journal of Communication Systems, 31(17), e3813.
Ghosh, J., Zhu, H., & Haci, H. (2021). A novel channel model and optimal beam tracking schemes for mobile millimeter-wave massive MIMO communications. IEEE Transactions on Vehicular Technology, 70(7), 7205–7210.
Sheikh, J. A., Mustafa, F., & Sidiq, S. (2022). New hybrid architecture for energy efficient and low complex massive MIMO system. Arabian Journal for Science and Engineering, 47(3), 3141–3149.
Yu, W., Wang, T., & Wang, S. (2021). Multi-label learning based antenna selection in massive MIMO systems. IEEE Transactions on Vehicular Technology, 70(7), 7255–7260.
Chung, M.K., Liu, L., Johansson, A., Gunnarsson, S., Nilsson, M., Ying, Z., Zander, O. et al. (2021). LuMaMi28: Real-time millimeter-wave massive MIMO systems with antenna selection. arXiv preprint arXiv:2109.03273.
Huang, H., Song, Y., Yang, J., Gui, G., & Adachi, F. (2019). Deep-learning-based millimeter-wave massive MIMO for hybrid precoding. IEEE Transactions on Vehicular Technology, 68(3), 3027–3032.
Zhang, X., & Zhao, F. (2021). Hybrid precoding algorithm for millimeter-wave massive MIMO systems with subconnection structures. Wireless Communications and Mobile Computing, 2021, 1–9.
Elbir, A. M. (2020). A deep learning framework for hybrid beamforming without instantaneous CSI feedback. IEEE Transactions on Vehicular Technology, 69(10), 11743–11755.
Ribeiro, L. N., Schwarz, S., Rupp, M., & de Almeida, A. L. F. (2018). Energy efficiency of mmWave massive MIMO precoding with low-resolution DACs. IEEE Journal of Selected Topics in Signal Processing, 12(2), 298–312.
Sennan, S., Somula, R., Luhach, A. K., Deverajan, G. G., Alnumay, W., Jhanjhi, N. Z., Ghosh, U., & Sharma, P. (2021). Energy efficient optimal parent selection based routing protocol for Internet of Things using firefly optimization algorithm. Transactions on Emerging Telecommunications Technologies, 32(8), e4171.
Gao, S., Wang, K., Tao, S., Jin, T., Dai, H., & Cheng, J. (2021). A state-of-the-art differential evolution algorithm for parameter estimation of solar photovoltaic models. Energy Conversion and Management, 230, 113784.
Gao, X., Dai, L., Han, S., Chih-Lin, I., & Heath, R. W. (2016). Energy-efficient hybrid analog and digital precoding for mmWave MIMO systems with large antenna arrays. IEEE Journal on Selected Areas in Communications, 34(4), 998–1009.
Yu, X., Shen, J.-C., Zhang, J., & Letaief, K. B. (2016). Alternating minimization algorithms for hybrid precoding in millimeter wave MIMO systems. IEEE Journal of Selected Topics in Signal Processing, 10(3), 485–500.
Khalid, S., Mehmood, R., Abbas, W. B., Khalid, F., & Naeem, M. (2022). Energy efficiency maximization of massive MIMO systems using RF chain selection and hybrid precoding. Telecommunication Systems, 80(2), 251–261.
Li, L., Ren, H., Li, X., Chen, W., & Han, Z. (2019). Machine learning-based spectrum efficiency hybrid precoding with lens array and low-resolution ADCs. IEEE Access, 7, 117986–117996.
Funding
No funding is provided for the preparation of the manuscript.
Author information
Authors and Affiliations
Contributions
All authors have equal contributions to this work.
Corresponding author
Ethics declarations
Conflict of interest
Authors declare that they have no conflict of interest.
Ethical Approval
This article does not contain any studies with human participants or animals performed by the authors.
Consent to Participate
All the authors involved have agreed to participate in this submitted article.
Consent to Publish
All the authors involved in this manuscript give full consent to publish this submitted article.
Additional information
Publisher's Note
Springer Nature remains neutral with regard to jurisdictional claims in published maps and institutional affiliations.
Rights and permissions
Springer Nature or its licensor (e.g. a society or other partner) holds exclusive rights to this article under a publishing agreement with the author(s) or other rightsholder(s); author self-archiving of the accepted manuscript version of this article is solely governed by the terms of such publishing agreement and applicable law.
About this article
Cite this article
Srinivas, C.V.V.S., Borugadda, S. RF Chain Selection Using Hybrid Optimization with Precoding in mm-Wave Massive MIMO Systems. Wireless Pers Commun 131, 1997–2017 (2023). https://doi.org/10.1007/s11277-023-10529-7
Accepted:
Published:
Issue Date:
DOI: https://doi.org/10.1007/s11277-023-10529-7