The problems of implementing systems with a voice interface for remote service of the population are examined. The effectiveness of such systems can be enhanced by automatic analysis of the changes of the emotional state of the user during dialogue. In order to do real-time measurements of the index of the dynamics of the emotional state, it is proposed to use the effect of the sound (phonetic) variability of speech of the user at observation intervals that are of small duration (fractions of a minute). Based on an information-theoretic approach, a method was developed for acoustic measurements of the dynamics of the emotional state under conditions of small samples, using a scale-invariant measure of the variations of the speech waveform in the frequency domain. An example of the practical instantiation of this method in real-time conditions is examined. It is shown that in this case the delay in obtaining measurement results does not exceed 10–20 s. The results of experimental studies confirmed the rapid response of the proposed method and its sensitivity to modifications of the dynamics of the emotional state under the effect of external perturbations. The developed method can be used to introduce automated monitoring of the quality of voice samples of users of the unified biometric systems. Also, the method will be useful to enhance security by noncontact detection of potentially dangerous persons with short-term disturbance of the psychoemotional state.




Similar content being viewed by others
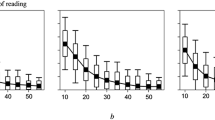
References
S. K. Davis et al., Pers. Indiv. Differ., 160, No. 109938 (2020), https://doi.org/10.1016/j.paid.2020.109938.
V. V. Savchenko and A. V. Savchenko, “A mode of refreshing voice samples in the Unified Biometric System in real time,” Izmer. Tekhn., No. 5, 58–65 (2020), https://doi.org/10.32446/0368-1025it.2020-5-58-65.
V. V. Savchenko and A. V. Savchenko, “A method for measuring the index of acoustic quality of audio recordings prepared for recording and processing in the Unified Biometric System,” Izmer. Tekhn., No. 12, 40–47 (2019), https://doi.org/10.32446/0368-1025it.2019-12-40-46.
E. I. Galyashina, Current problems of the identification of persons using sound records of telephone conversations,” in: Proc. 23rd Int. Sci. Practi. Conf. Activities of Law Enforcement Agencies in Contemporary Conditions, VSI MVD RF, Irkutsk (2018), pp. 141–146, https://istina.msu.ru/publications/article/167326015, acc. 8/14/2020.
E. Falagiarda and O. Collignon, Cortex, 119, 184–194 (2019), https://doi.org/10.1016/j.cortex.2019.04.017.
F. P. Akbulut, H. G. Perros, and M. Shahzad, Comp. Meth. Progr. Biomed., 195, No. 105571 (2020), https://doi.org/10.1016/j.cmpb.2020.105571.
F. A. Shaqra, R. Duwairi, and M. Al-Ayyoub, Proced. Comp. Sci., 151, 37–44 (2019), https://doi.org/10.1016/j.procs.2019.04.009.
J. M. Arana et al., Comp. Hum. Behav., 104, No. 106156 (2020), https://doi.org/10.1016/j.chb.2019.106156.
M. Bourguignon et al., Neurolmage, 216, No. 116788 (2020), https://doi.org/10.1016/j.neuroimage.2020.116788.
Z. Liu et al., Brain Lang., 203, No. 104755 (2020), https://doi.org/10.1016/j.bandl.2020.104755.
B. Schuller, “Voice and speech analysis in search of states and traits,” in: A. A. Salah and T. Gevers (eds.), Computer Analysis of Human Behavior, Springer, Heidelberg (2011), https://doi.org/10.1007/978-0-85729-994-9_9.
D. Cardona et al., Neurocomputing, 265, 78–90 (2017), https://doi.org/10.1016/j.neucom.2016.09.140.
D. Yu and L. Deng, Automatic Speech Recognition: A Deep Learning Approach, Springer, (2014), https://doi.org/10.1007/978-1-4471-5779-3.
M. Schuster, Lect. Notes Comp. Sci., 6230, 8–10 (2010), https://doi.org/10.1007/978-3-642-15246-7_3.
R. Rammohan et al., J. Allergy Clin. Immunol., 139, Iss. 2, No. ab250 (2017), https://doi.org/10.1016/j.jaci.2016.12.804.
N. A. Volodin, T. V. Ermolenko, and V. V. Semenyuk, “A study of the effectiveness of the application of neural networks for recognition of human emotions through the voice,” in: Donetsk Readings 2019: Education, Science, Innovations, Culture, and the Calls to Modernity. Proc. 4th Int. Sci. Conf. (2019), pp. 221–223, https://elibrary.ru/ download/elibrary_41422521_75290048.pdf, acc. Aug. 14, 2020.
A. M. Grachev, D. I. Ignatov, and A. V. Savchenko, Appl. Soft Comput., 79, 354–362 (2019), https://doi.org/10.1016/j.asoc.2019.03.057.
R. A. Ustinov, “Features of modern protection systems for speech information,” Bezopasn. Inform. Tekhn. (electronic journal), 24, No. 4 (2017), https://doi.org/10.26583/bit.2017.4.08.
S. Cui, E. Li, and X. Kang, “Autoregressive model based smoothing forensics of very short speech clips,” 2020 IEEE Int. Conf. on Multimedia and Expo (ICME), London, United Kingdom (2020), pp. 1–6, https://doi.org/10.1109/ICME46284.2020.9102765.
V. V. Savchenko, Radioelectr. Commun. Syst., 63, No. 1, 42–54 (2020), https://doi.org/10.3103/S0735272720010045.
V. V. Savchenko and A. V. Savchenko, “The criterion of a guaranteed level of signifi cance in the problem of automatic segmentation of a speech waveform,” Radiotekhn. Elektron., 65, No. 11, 1060–1066 (2020), https://doi.org/10.31857/S0033849420110157.
R. G. Hautamäki et al., Speech Commun., 95, 1–15 (2017), https://doi.org/10.1016/j.specom.2017.10.002.
N. N. Lebedev and E. D. Karimov, “Acoustic characteristics of a speech waveform as an indicator of the functional state of the person,” Usp. Fiziol. Nauk, 45, No. 1, 57–95 (2014), http://naukarus.com/akusticheskieharakteristiki-rechevogo-signala-kak-pokazatel-funktsionalnogo sostoyaniya-cheloveka, acc. Aug. 14, 2020.
V. V. Savchenko, J. Commun. Technol. Electr., 63, No. 1, 53–57 (2018), https://doi.org/10.1134/S1064226918010126.
A. V. Savchenko and V. V. Savchenko, J. Commun. Technol. Electr., 61, No. 4, 430–435 (2016), https://doi.org/10.1134/S1064226916040112.
V. V. Savchenko, “A method of measuring the index of acoustic voice quality based on an information-theoretic approach,” Izmer. Tekhn., No. 1, 60–64 (2018), https://doi.org/10.32446/0368-1025it.2018-1-60-64.
V. V. Savchenko and L. V. Savchenko, “ A method of measuring the index of intelligibility of speech signals in the Kullback–Leibler informational metric,” Izmer. Tekhn., No. 9, 59–64 (2019), https://doi.org/10.32446/0368-1025it.2019-9-59-64.
L. V. Savchenko and A. V. Savchenko, J. Commun. Technol. Electr., 64, No. 3, 238–244 (2019), https://doi.org/10.1134/S1064226919030173.
A. V. Savchenko and V. V. Savchenko, “A method of measuring the frequency of the fundamental component of a speech waveform for systems of the acoustic analysis of speech,” Izmer. Tekhn., No. 3, 59–63 (2019), https://doi.org/10.32446/0368-1025it.2019-3-59-63.
A. V. Savchenko, “Three-Way decisions in efficient classification of piecewise stationary speech waveforms,” in Polkowski L. et al. (eds.), Rough Sets. IJCRS 2017. Lecture Notes in Computer Science, Springer, Cham (2017), Vol. 10314, https://doi.org/10.1007/978-3-319-60840-2_19.
S. Kullback, Information Theory and Statistics, Dover Publications, N.Y. (1997), https://www.amazon.com/dp/0486696847, acc. Aug. 14, 2020.
R. M. Gray et al., IEEE T. Signal Proces., 28, No. 4, 367–377 (1980), https://doi.org/10.1109/TASSP.1980.1163421.
A. V. Savchenko, V. V. Savchenko, and L. V. Savchenko, “Optimization of Gain in Symmetrized Itakura–Saito Discrimination for Pronunciation Learning,” in: A. Kononov et al. (eds), Mathematical Optimization Theory and Operations Research. MOTOR 2020. Lecture Notes in Computer Science, Springer, Cham (2020), Vol. 12095, https://doi.org/10.1007/978-3-030-49988-4_30.
V. Vestman et al., Speech Commun., 99, 62–79 (2018), https://doi.org/10.1016/j.specom.2018.02.009.
Q. Candan, Signal Process., 166, No. 107256 (2020), 10.1016/j.sigpro.2019.107256.
K. S. Tuncel and M. G. Baydogan, Pattern Recogn., 73, 202–215 (2018), https://doi.org/10.1016/j.patcog.2017.08.016.
V. V. Savchenko and A. V. Savchenko, Radioelectron. Commun. Syst., 62, 276–286 (2019), https://doi.org/10.3103/S0735272719050042.
S. L. Marple, Digital Spectral Analysis with Applications, Dover Publications, Mineola, New York (2019), 2nd ed., https://www.goodreads.com/book/show/19484239, acc. Aug. 14, 2020.
This study was performed with the support of the Russian Science Foundation (Project No. 20-71-10010).
Author information
Authors and Affiliations
Corresponding author
Additional information
Translated from Izmeritel’naya Tekhnika, No. 4, pp. 49–57, April, 2021.
Rights and permissions
About this article
Cite this article
Savchenko, L.V., Savchenko, A.V. A Method of Real-Time Dynamic Measurement of a Speaker’s Emotional State from a Speech Waveform. Meas Tech 64, 319–327 (2021). https://doi.org/10.1007/s11018-021-01935-z
Received:
Accepted:
Published:
Issue Date:
DOI: https://doi.org/10.1007/s11018-021-01935-z