Abstract
Objectives
Accurate evaluation of bowel fibrosis in patients with Crohn’s disease (CD) remains challenging. Computed tomography enterography (CTE)–based radiomics enables the assessment of bowel fibrosis; however, it has some deficiencies. We aimed to develop and validate a CTE-based deep learning model (DLM) for characterizing bowel fibrosis more efficiently.
Methods
We enrolled 312 bowel segments of 235 CD patients (median age, 33 years old) from three hospitals in this retrospective study. A training cohort and test cohort 1 were recruited from center 1, while test cohort 2 from centers 2 and 3. All patients performed CTE within 3 months before surgery. The histological fibrosis was semi-quantitatively assessed. A DLM was constructed in the training cohort based on a 3D deep convolutional neural network with 10-fold cross-validation, and external independent validation was conducted on the test cohorts. The radiomics model (RM) was developed with 4 selected radiomics features extracted from CTE images by using logistic regression. The evaluation of CTE images was performed by two radiologists. DeLong’s test and a non-inferiority test were used to compare the models’ performance.
Results
DLM distinguished none-mild from moderate-severe bowel fibrosis with an area under the receiver operator characteristic curve (AUC) of 0.828 in the training cohort and 0.811, 0.808, and 0.839 in the total test cohort, test cohorts 1 and 2, respectively. In the total test cohort, DLM achieved better performance than two radiologists (*1 AUC = 0.579, *2 AUC = 0.646; both p < 0.05) and was not inferior to RM (AUC = 0.813, p < 0.05). The total processing time for DLM was much shorter than that of RM (p < 0.001).
Conclusion
DLM is better than radiologists in diagnosing intestinal fibrosis on CTE in patients with CD and not inferior to RM; furthermore, it is more time-saving compared to RM.
Key Points
• Question Could computed tomography enterography (CTE)–based deep learning model (DLM) accurately distinguish intestinal fibrosis severity in patients with Crohn’s disease (CD)?
• Findings In this cross-sectional study that included 235 patients with CD, DLM achieved better performance than that of two radiologists’ interpretation and was not inferior to RM with significant differences and much shorter processing time.
• Meaning This DLM may accurately distinguish the degree of intestinal fibrosis in patients with CD and guide gastroenterologists to formulate individualized treatment strategies for those with bowel strictures.






Similar content being viewed by others
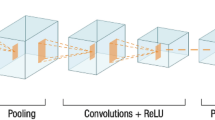
Abbreviations
- AI:
-
Artificial intelligence
- CD:
-
Crohn’s disease
- CTE:
-
CT enterography
- DLM:
-
Deep learning model
- RM:
-
Radiomics model
- VOI:
-
Volume of interest
References
Latella G, Di Gregorio J, Flati V, Rieder F, Lawrance IC (2015) Mechanisms of initiation and progression of intestinal fibrosis in IBD. Scand J Gastroenterol 50:53–65
Bouhnik Y, Carbonnel F, Laharie D et al (2018) Efficacy of adalimumab in patients with Crohn’s disease and symptomatic small bowel stricture: a multicentre, prospective, observational cohort (CREOLE) study. Gut 67:53–60
Rieder F, Fiocchi C, Rogler G (2017) Mechanisms, management, and treatment of fibrosis in patients with inflammatory bowel diseases. Gastroenterology 152:340-350.e346
Li XH, Mao R, Huang SY et al (2018) Characterization of degree of intestinal fibrosis in patients with Crohn disease by using magnetization transfer MR imaging. Radiology 287:494–503
Pellino G, Nicolai E, Catalano OA et al (2016) PET/MR versus PET/CT imaging: impact on the clinical management of small-bowel Crohn’s disease. J Crohns Colitis 10:277–285
Baumgart DC, Müller HP, Grittner U et al (2015) US-based real-time elastography for the detection of fibrotic gut tissue in patients with stricturing Crohn disease. Radiology 275:889–899
Hosny A, Parmar C, Quackenbush J, Schwartz LH, Aerts HJWL (2018) Artificial intelligence in radiology. Nat Rev Cancer 18:500–510
Esteva A, Kuprel B, Novoa RA et al (2017) Dermatologist-level classification of skin cancer with deep neural networks. Nature 542:115–118
Eweje FR, Bao B, Wu J et al (2021) Deep learning for classification of bone lesions on routine MRI. EBioMedicine 68:103402
Luo H, Xu G, Li C et al (2019) Real-time artificial intelligence for detection of upper gastrointestinal cancer by endoscopy: a multicentre, case-control, diagnostic study. Lancet Oncol 20:1645–1654
Yang X, Wang H, Dong Q et al (2021) An artificial intelligence system for distinguishing between gastrointestinal stromal tumors and leiomyomas using endoscopic ultrasonography. Endoscopy. https://doi.org/10.1055/a-1476-8931
Lamash Y, Kurugol S, Freiman M et al (2019) Curved planar reformatting and convolutional neural network-based segmentation of the small bowel for visualization and quantitative assessment of pediatric Crohn’s disease from MRI. J Magn Reson Imaging 49:1565–1576
Mahapatra D, Vos FM, Buhmann JM (2016) Active learning based segmentation of Crohns disease from abdominal MRI. Comput Methods Programs Biomed 128:75–85
Morilla I (2020) A deep learning approach to evaluate intestinal fibrosis in magnetic resonance imaging models. Neural Comput Appl 32:14865–14874
Li X, Liang D, Meng J et al (2021) Development and validation of a novel computed-tomography enterography radiomic approach for characterization of intestinal fibrosis in Crohn’s disease. Gastroenterology. https://doi.org/10.1053/j.gastro.2021.02.027
Esteva A, Robicquet A, Ramsundar B et al (2019) A guide to deep learning in healthcare. Nat Med 25:24–29
Rimola J, Planell N, Rodríguez S et al (2015) Characterization of inflammation and fibrosis in Crohn’s disease lesions by magnetic resonance imaging. Am J Gastroenterol 110:432–440
Zhang MC, Li XH, Huang SY et al (2019) IVIM with fractional perfusion as a novel biomarker for detecting and grading intestinal fibrosis in Crohn’s disease. Eur Radiol 29:3069–3078
Adler J, Punglia DR, Dillman JR et al (2012) Computed tomography enterography findings correlate with tissue inflammation, not fibrosis in resected small bowel Crohn’s disease. Inflamm Bowel Dis 18:849–856
Viera AJ, Garrett JM (2005) Understanding interobserver agreement: the kappa statistic. Fam Med 37:360–363
Li XH, Feng ST, Cao QH et al (2021) Degree of creeping fat assessed by CT enterography is associated with intestinal fibrotic stricture in patients with Crohn’s disease: a potentially novel mesenteric creeping fat index. J Crohns Colitis. https://doi.org/10.1093/ecco-jcc/jjab005
He K, Zhang X, Ren S, Sun J (2016) Deep residual learning for image recognition. 2016 IEEE Conference on Computer Vision and Pattern Recognition 90:9
Lin TY, Goyal P, Girshick R, He K, Dollar P (2020) Focal loss for dense object detection. IEEE Trans Pattern Anal Mach Intell 42:318–327
Kingma DP. Ba J (2015) ADAM: a method for stochastic optimization. The International Conference on Learning Representations:15
Selvaraju RR, Cogswell M, Das A et al (2017) Grad-CAM: visual explanations from deep networks via gradient-based localization. IEEE International Conference on Computer Vision:9
Van Der Maaten L, Hinton G (2008) Visualizing Ddata using t-SNE. J Mach Learn Res 9:27
Obuchowski NA (2005) ROC analysis. AJR Am J Roentgenol 184:364–372
Dercle L, Fronheiser M, Lu L et al (2020) Identification of non-small cell lung cancer sensitive to systemic cancer therapies using radiomics. Clin Cancer Res 26:2151–2162
Liu JP, Ma MC, Wu CY, Tai JY (2006) Tests of equivalence and non-inferiority for diagnostic accuracy based on the paired areas under ROC curves. Stat Med 25:1219–1238
Zhou XH, Obuchowski NA, McClish DK (2002) Statistical methods in diagnostic medicine. Wiley, New York, pp 188–192
Vickers AJ, Elkin EB (2006) Decision curve analysis: a novel method for evaluating prediction models. Med Decis Making 26:565–574
Sun Q, Lin X, Zhao Y et al (2020) Deep learning vs. radiomics for predicting axillary lymph node metastasis of breast cancer using ultrasound images: don’'t forget the peritumoral region. Front Oncol 10:53
Truhn D, Schrading S, Haarburger C, Schneider H, Merhof D, Kuhl C (2019) Radiomic versus convolutional neural networks analysis for classification of contrast-enhancing lesions at multiparametric breast MRI. Radiology 290:290–297
Luo Y, Chen X, Chen J et al (2020) Preoperative prediction of pancreatic neuroendocrine neoplasms grading based on enhanced computed tomography imaging: validation of deep learning with a convolutional neural network. Neuroendocrinology 110:338–350
Kato H, Kanematsu M, Zhang XJ et al (2007) Computer-aided diagnosis of hepatic fibrosis: pPreliminary evaluation of MRI texture analysis using the finite difference method and an artificial neural network. AJR Am J Roentgenol 189:117–122
Gordon IO, Bettenworth D, Bokemeyer A et al (2022) International consensus to standardise histopathological scoring for small bowel strictures in Crohn’s disease. Gut 71:479–486
Chiorean MV, Sandrasegaran K, Saxena R, Maglinte DD, Nakeeb A, Johnson CS (2007) Correlation of CT enteroclysis with surgical pathology in Crohn’'s disease. Am J Gastroenterol 102:2541–2550
Li JN, Mao R, Kurada S et al (2019) Pathogenesis of fibrostenosing Crohn’'s disease. Transl Res 209:39–54
Byers P (2020) There can be no other reason for this behavior: issues in the ascription of knowledge to humans and AI. Integr Psychol Behav Sci. https://doi.org/10.1007/s12124-020-09531-6
Acknowledgements
The authors thank Xinning Liu (a pathologist from The First Affiliated Hospital of Sun Yat-sen University) for her contribution to the histopathologic score for the test of the inter-pathologist consistency.
Funding
This study was supported by the National Natural Science Foundation of China (82070680, 82072002, 81600508, 81770654, 81771908, 81870451, 81802431, and 81972516), Natural Science Foundation of Guangdong Province (2018A030313050 and 2021A1515012448), Guangdong Basic and Applied Basic Research Foundation (2020A1515010571), and Shenzhen-Hong Kong Institute of Brain Science-Shenzhen Fundamental Research Institutions (2019SHIBS0003). The funders of the study had no role in study design, data collection, data analysis, data interpretation, or writing of the report.
Author information
Authors and Affiliations
Corresponding authors
Ethics declarations
Guarantor
The scientific guarantor of this publication is Dr. Xuehua Li.
Conflict of interest
The authors declare no competing interests.
Statistics and biometry
No complex statistical methods were necessary for this paper.
Informed consent
Written informed consent was waived by the Institutional Review Board.
Ethical approval
Approved by the institutional ethics review board of The First Affiliated Hospital of Sun Yat-sen University.
Study subjects or cohorts overlap
One hundred fourteen patients with 159 samples in the training cohort and 53 patients with 53 samples in test cohort 2 in this study came from our previous radiomics study Development and Validation of a Novel Computed-Tomography Enterography Radiomic Approach for Characterization of Intestinal Fibrosis in Crohn’s Disease.
Methodology
• retrospective
• cross sectional study
• multi-center study
Additional information
Publisher’s note
Springer Nature remains neutral with regard to jurisdictional claims in published maps and institutional affiliations.
Supplementary information
ESM 1
(DOCX 1.28 mb)
Rights and permissions
About this article
Cite this article
Meng, J., Luo, Z., Chen, Z. et al. Intestinal fibrosis classification in patients with Crohn’s disease using CT enterography–based deep learning: comparisons with radiomics and radiologists. Eur Radiol 32, 8692–8705 (2022). https://doi.org/10.1007/s00330-022-08842-z
Received:
Revised:
Accepted:
Published:
Issue Date:
DOI: https://doi.org/10.1007/s00330-022-08842-z