A Machine Learning Method for the Optimization Design of Laser Pulse in Fast Ignition Simulations
Authors (first, second and last of 7)
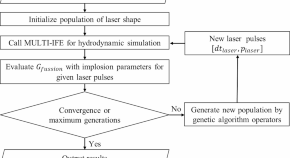
Collection
Dr. Rea, a research scientist and working group leader at MIT Plasma Science and Fusion Center, integrates machine learning algorithms with plasma control systems to address the challenge of disruptive tokamak plasmas. She works with scientists at Alcator C-Mod, DIII-D, EAST, and TCV facilities on machine learning applications for plasma stability. Dr Rea is the PSFC Disruptions Group leader and a key contributor to SPARC design and ITER research. Dr Rea further organizes the PSFC Computational Physics School for Fusion Research, focusing on High Performance Computing, Parallel Programming, Computational Statistics, and Machine Learning.
EPFL / Swiss Plasma Center (PPB-115) Station 13, Lausanne, Switzerland
Princeton Plasma Physics Laboratory, Princeton, NJ, USA