Abstract
Rare diseases are characterized by low prevalence and are often chronically debilitating or life-threatening. Imaging-based classification of rare diseases is challenging due to the severe shortage in training examples. Few-shot learning (FSL) methods tackle this challenge by extracting generalizable prior knowledge from a large base dataset of common diseases and normal controls, and transferring the knowledge to rare diseases. Yet, most existing methods require the base dataset to be labeled and do not make full use of the precious examples of the rare diseases. To this end, we propose in this work a novel hybrid approach to rare disease classification, featuring two key novelties targeted at the above drawbacks. First, we adopt the unsupervised representation learning (URL) based on self-supervising contrastive loss, whereby to eliminate the overhead in labeling the base dataset. Second, we integrate the URL with pseudo-label supervised classification for effective self-distillation of the knowledge about the rare diseases, composing a hybrid approach taking advantages of both unsupervised and (pseudo-) supervised learning on the base dataset. Experimental results on classification of rare skin lesions show that our hybrid approach substantially outperforms existing FSL methods (including those using fully supervised base dataset) for rare disease classification via effective integration of the URL and pseudo-label driven self-distillation, thus establishing a new state of the art.
J. Sun and D. Wei—Contributed equally; J. Sun contributed to this work during an internship at Tencent.
Access this chapter
Tax calculation will be finalised at checkout
Purchases are for personal use only
Similar content being viewed by others
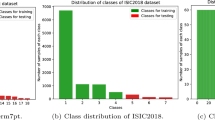
References
Chen, T., Kornblith, S., Norouzi, M., Hinton, G.: A simple framework for contrastive learning of visual representations. In: International Conference on Machine Learning, pp. 1597–1607. PMLR (2020)
Chen, W.Y., Liu, Y.C., Kira, Z., Wang, Y.C.F., Huang, J.B.: A closer look at few-shot classification. In: International Conference on Learning Representations (2019)
Chen, X., Fan, H., Girshick, R., He, K.: Improved baselines with momentum contrastive learning. arXiv preprint arXiv:2003.04297 (2020)
Codella, N., et al.: Skin Lesion Analysis Toward Melanoma Detection 2018: A Challenge hosted by the International Skin Imaging Collaboration (ISIC). arXiv preprint arXiv:1902.03368 (2019)
Finn, C., Abbeel, P., Levine, S.: Model-agnostic meta-learning for fast adaptation of deep networks. In: International Conference on Machine Learning, pp. 1126–1135. PMLR (2017)
Furlanello, T., Lipton, Z., Tschannen, M., Itti, L., Anandkumar, A.: Born again neural networks. In: International Conference on Machine Learning, pp. 1607–1616. PMLR (2018)
He, K., Fan, H., Wu, Y., Xie, S., Girshick, R.: Momentum contrast for unsupervised visual representation learning. In: Proceedings of the IEEE/CVF Conference on Computer Vision and Pattern Recognition, pp. 9729–9738 (2020)
Hinton, G., Vinyals, O., Dean, J.: Distilling the knowledge in a neural network. In: NIPS Deep Learning and Representation Learning Workshop (2015)
Hsu, K., Levine, S., Finn, C.: Unsupervised learning via meta-learning. In: International Conference on Learning Representations (2018)
Huang, G., Liu, Z., van der Maaten, L., Weinberger, K.Q.: Densely connected convolutional networks. In: Proceedings of the IEEE Conference on Computer Vision and Pattern Recognition (2017)
Huang, L., Zhang, C., Zhang, H.: Self-adaptive training: Beyond empirical risk minimization. arXiv preprint arXiv:2002.10319 (2020)
Jiang, X., Ding, L., Havaei, M., Jesson, A., Matwin, S.: Task adaptive metric space for medium-shot medical image classification. In: Shen, D., et al. (eds.) MICCAI 2019. LNCS, vol. 11764, pp. 147–155. Springer, Cham (2019). https://doi.org/10.1007/978-3-030-32239-7_17
Ker, J., Wang, L., Rao, J., Lim, T.: Deep learning applications in medical image analysis. IEEE Access 6, 9375–9389 (2018)
Khodadadeh, S., Boloni, L., Shah, M.: Unsupervised meta-learning for few-shot image classification. In: Advances in Neural Information Processing Systems, vol. 32. Curran Associates, Inc. (2019)
Kim, K., Ji, B., Yoon, D., Hwang, S.: Self-knowledge distillation: A simple way for better generalization. arXiv preprint arXiv:2006.12000 (2020)
Lee, K., Maji, S., Ravichandran, A., Soatto, S.: Meta-learning with differentiable convex optimization. In: Proceedings of the IEEE/CVF Conference on Computer Vision and Pattern Recognition, pp. 10657–10665 (2019)
Li, F.F., Fergus, R., Perona, P.: One-shot learning of object categories. IEEE Trans. Pattern Anal. Mach. Intell. 28(4), 594–611 (2006)
Li, X., Yu, L., Jin, Y., Fu, C.-W., Xing, L., Heng, P.-A.: Difficulty-Aware Meta-learning for Rare Disease Diagnosis. In: Martel, A.L., et al. (eds.) MICCAI 2020. LNCS, vol. 12261, pp. 357–366. Springer, Cham (2020). https://doi.org/10.1007/978-3-030-59710-8_35
Litjens, G., et al.: A survey on deep learning in medical image analysis. Med. Image Anal. 42, 60–88 (2017)
Oord, A.v.d., Li, Y., Vinyals, O.: Representation learning with contrastive predictive coding. arXiv preprint arXiv:1807.03748 (2018)
Paul, A., Tang, Y.X., Shen, T.C., Summers, R.M.: Discriminative ensemble learning for few-shot chest X-ray diagnosis. Med. Image Anal. 68, 101911 (2021)
Ravichandran, A., Bhotika, R., Soatto, S.: Few-shot learning with embedded class models and shot-free meta training. In: Proceedings of the IEEE/CVF International Conference on Computer Vision, pp. 331–339 (2019)
Sandler, M., Howard, A., Zhu, M., Zhmoginov, A., Chen, L.C.: MobileNetV2: Inverted residuals and linear bottlenecks. In: Proceedings of the IEEE Conference on Computer Vision and Pattern Recognition (2018)
Shen, D., Wu, G., Suk, H.I.: Deep learning in medical image analysis. Annu. Rev. Biomed. Eng. 19(1), 221–248 (2017)
Snell, J., Swersky, K., Zemel, R.: Prototypical networks for few-shot learning. In: Advances in Neural Information Processing Systems, pp. 4080–4090 (2017)
Steiner, B., et al.: PyTorch: an imperative style, high-performance deep learning library. Adv. Neural Inf. Process. Syst. 32, 8026–8037 (2019)
Stolk, P., Willemen, M.J., Leufkens, H.G.: Rare essentials: drugs for rare diseases as essential medicines. Bull. World Health Organ. 84, 745–751 (2006)
Sung, F., Yang, Y., Zhang, L., Xiang, T., Torr, P.H., Hospedales, T.M.: Learning to compare: relation network for few-shot learning. In: Proceedings of the IEEE Conference on Computer Vision and Pattern Recognition (June 2018)
Tian, Y., Wang, Y., Krishnan, D., Tenenbaum, J.B., Isola, P.: Rethinking few-shot image classification: a good embedding is all you need? In: Proceedings of the European Conference on Computer Vision (2020)
Tschandl, P., Rosendahl, C., Kittler, H.: The HAM10000 dataset, a large collection of multi-source dermatoscopic images of common pigmented skin lesions. Sci. Data 5(1), 1–9 (2018)
Vinyals, O., Blundell, C., Lillicrap, T., Kavukcuoglu, K., Wierstra, D.: Matching networks for one shot learning. In: Advances in Neural Information Processing Systems, pp. 3637–3645 (2016)
Zhang, L., Song, J., Gao, A., Chen, J., Bao, C., Ma, K.: Be your own teacher: improve the performance of convolutional neural networks via self distillation. In: Proceedings of the IEEE/CVF International Conference on Computer Vision, pp. 3713–3722 (2019)
Zhang, X., Zhou, X., Lin, M., Sun, J.: ShuffleNet: an extremely efficient convolutional neural network for mobile devices. In: Proceedings of the IEEE Conference on Computer Vision and Pattern Recognition (2018)
Zhu, W., Liao, H., Li, W., Li, W., Luo, J.: Alleviating the incompatibility between cross entropy loss and episode training for few-shot skin disease classification. In: Martel, A.L., et al. (eds.) MICCAI 2020. LNCS, vol. 12266, pp. 330–339. Springer, Cham (2020). https://doi.org/10.1007/978-3-030-59725-2_32
Acknowledgments
This work was supported by the Fundamental Research Funds for the Central Universities (Grant No. 20720190012), Key-Area Research and Development Program of Guangdong Province, China (No. 2018B010111001), and Scientific and Technical Innovation 2030 - “New Generation Artificial Intelligence” Project (No. 2020AAA0104100).
Author information
Authors and Affiliations
Corresponding author
Editor information
Editors and Affiliations
1 Electronic supplementary material
Below is the link to the electronic supplementary material.
Rights and permissions
Copyright information
© 2021 Springer Nature Switzerland AG
About this paper
Cite this paper
Sun, J., Wei, D., Ma, K., Wang, L., Zheng, Y. (2021). Unsupervised Representation Learning Meets Pseudo-Label Supervised Self-Distillation: A New Approach to Rare Disease Classification. In: de Bruijne, M., et al. Medical Image Computing and Computer Assisted Intervention – MICCAI 2021. MICCAI 2021. Lecture Notes in Computer Science(), vol 12905. Springer, Cham. https://doi.org/10.1007/978-3-030-87240-3_50
Download citation
DOI: https://doi.org/10.1007/978-3-030-87240-3_50
Published:
Publisher Name: Springer, Cham
Print ISBN: 978-3-030-87239-7
Online ISBN: 978-3-030-87240-3
eBook Packages: Computer ScienceComputer Science (R0)