Abstract
Artificial intelligence (AI)-based systems are normally data driven applications, where the model is trained to think on its own based on the external circumstances. The power of AI has reached every facet of business and common life and is even being largely explored to be adopted in life sciences and medical domains. It supports the human in decision-making through the cognitive utilities which arises out of self-learning capabilities of a model. With the exponential growth of data, supply chain management and analytics have attracted a large community of researchers to build intelligent systems which can lead to re-invention of data-driven decision systems powered by AI. Systems and literature of the past shows that AI-based technologies are promising in intelligent supply chain management (SCM) and building resilient SCMs. There is a gap in literature which addresses on the framework for decision support systems in SCM and application of AI methods for building a robust supply chain resilience (SCR) leading to more exploration on the topic. In this paper, a decision framework is proposed by incorporating fuzzy logic and recurrent neural networks (RNN) for disclosing the patterns of various AI-enabled techniques for SCRs. The proposed analysis involved data from leading literatures to determine the most adoptable and significant applications of AI in SCRs. The analysis shows that techniques such as fuzzy programing, network based algorithms, and genetic algorithms have large impact on building SCRs. The results help in decision-making by exhibiting an integrated framework which can help the AI practitioners for developing SCRs.
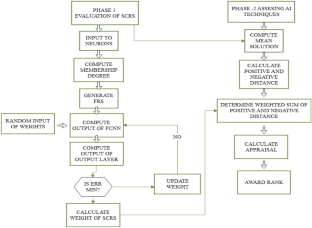


Similar content being viewed by others
Data Availability
The data that support the findings of this study are available on request from the corresponding author.
References
Zhao P, Li Z, Han X, Duan X (2022) Supply chain network resilience by considering disruption propagation: topological and operational perspectives. IEEE Syst J 16(4):5305–5316. https://doi.org/10.1109/JSYST.2022.3161788
Raj R et al (2015) Measuring the resilience of supply chain systems using a survival model. IEEE Syst J 9(2):377–381. https://doi.org/10.1109/JSYST.2014.2339552
Pavlov D, Ivanov A, Dolgui, Sokolov B (2018) Hybrid fuzzy-probabilistic approach to supply chain resilience assessment in IEEE Transactions on Engineering Management. 65(2):303–315.https://doi.org/10.1109/TEM.2017.277357
Hosseini S, Ivanov D, Blackhurst J (2022) Conceptualization and measurement of supply chain resilience in an open-system context. IEEE Trans Eng Manage 69(6):3111–3126. https://doi.org/10.1109/TEM.2020.3026465
Bak O, Shaw S, Colicchia C, Kumar V (2023) A systematic literature review of supply chain resilience in small–medium enterprises (SMEs): a call for further research. IEEE Trans Eng Manage 70(1):328–341. https://doi.org/10.1109/TEM.2020.3016988
Ocicka B, Mierzejewska W, Brzeziński J (2022) Correction: creating supply chain resilience during and post-COVID-19 outbreak: the organizational ambidexterity perspective. Decision 49:361. https://doi.org/10.1007/s40622-022-00322-z
Yin W (2022) Identifying the pathways through digital transformation to achieve supply chain resilience: an fsQCA approach. Environ SciPollut Res. https://doi.org/10.1007/s11356-022-22917-w
Gao Y, Feng Z, Zhang S (2021) Managing supply chain resilience in the era of VUCA. Front Eng Manag 8:465–470. https://doi.org/10.1007/s42524-021-0164-2
Kazemian I, Torabi SA, Zobel CW et al (2022) A multi-attribute supply chain network resilience assessment framework based on SNA-inspired indicators. Oper Res Int J 22:1853–1883. https://doi.org/10.1007/s12351-021-00644-3
Misbauddin SM, Alam MJ, Karmaker CL, Nabi MNU, Hasan MM (2023) Exploring the antecedents of supply chain viability in a pandemic context: an empirical study on the commercial flower supply chain of an emerging economy. Sustainability 15:2146. https://doi.org/10.3390/su15032146
Pajić V, Kilibarda M, Andrejić M (2023) A novel hybrid approach for evaluation of resilient 4PL provider for E-commerce. Mathematics 11:511. https://doi.org/10.3390/math11030511
Belhadi A, Kamble S, Fosso Wamba S, Queiroz MM (2022) Building supply-chain resilience: an artificial intelligence-based technique and decision-making framework. Int J Prod Res 60(14):4487–4507. https://doi.org/10.1080/00207543.2021.1950935
Chen X, Xi Z, Jing P (2017) A unified framework for evaluating supply chain reliability and resilience. IEEE Trans Reliab 66(4):1144–1156. https://doi.org/10.1109/TR.2017.2737822
Tian Y, Shi Y, Shi X, Li M, Zhang M (2021) Research on supply chain network resilience considering the exit and reselection of enterprises. IEEE Access 9:91265–91281. https://doi.org/10.1109/ACCESS.2021.3090332
Zhao K, Kumar A, Harrison TP, Yen J (2011) Analyzing the resilience of complex supply network topologies against random and targeted disruptions. IEEE Syst J 5(1):28–39. https://doi.org/10.1109/JSYST.2010.2100192
Pennisi di Floristella A, Chen X (2022) Building resilient supply chains in uncertain times: a comparative study of EU and ASEAN approaches to supply chain resilience. Asia Eur J 20:457–475. https://doi.org/10.1007/s10308-022-00652-8
Singh CS, Soni G, Badhotiya GK (2019) Performance indicators for supply chain resilience: review and conceptual framework. J Ind Eng Int 15(Suppl 1):105–117. https://doi.org/10.1007/s40092-019-00322-2
Piprani AZ, Jaafar NI, Ali SM et al (2022) Multi-dimensional supply chain flexibility and supply chain resilience: the role of supply chain risks exposure. OperManag Res 15:307–325. https://doi.org/10.1007/s12063-021-00232-w
Abdullah A, Saraswat S, Talib F (2023) Impact of smart, green, resilient, and lean manufacturing system on SMEs’ performance: a data envelopment analysis (DEA) approach. Sustainability 15:1379. https://doi.org/10.3390/su15021379
Wang Y, Ren J, Zhang L, Liu D (2023) Research on resilience evaluation of green building supply chain based on ANP-fuzzy model. Sustainability 15:285. https://doi.org/10.3390/su15010285
Sengupta T, Narayanamurthy G, Moser R et al (2022) Disruptive technologies for achieving supply chain resilience in COVID-19 era: an implementation case study of satellite imagery and blockchain technologies in fish supply chain. Inf Syst Front 24:1107–1123. https://doi.org/10.1007/s10796-021-10228-3
Dyatkin B (2020) COVID-19 pandemic highlights need for US policies that increase supply chain resilience. MRS Bull 45:794–796. https://doi.org/10.1557/mrs.2020.258
Ayyildiz E (2021) Interval valued intuitionistic fuzzy analytic hierarchy process-based green supply chain resilience evaluation methodology in post COVID-19 era. Environ Sci Pollut Res. https://doi.org/10.1007/s11356-021-16972-y
Rajesh R (2022) A novel advanced grey incidence analysis for investigating the level of resilience in supply chains. Ann Oper Res 308:441–490. https://doi.org/10.1007/s10479-020-03641-5
Mustapha SA, Ali Agha MS, Masood T (2022) The role of collaborative resource sharing in supply chain recovery during disruptions: a systematic literature review. In IEEE Access 10:115603–115623. https://doi.org/10.1109/ACCESS.2022.3217500
Wang J et al (2016) Toward a resilient holistic supply chain network system: concept, review and future direction. IEEE Syst J 10(2):410–421. https://doi.org/10.1109/JSYST.2014.2363161
Schiele H, Hoffmann P, Körber T (2021) Synchronicity management: mitigating supply chain risks by systematically taking demand changes as starting point—a lesson from the COVID-19 crisis. In IEEE Engineering Management Review 49(1):55–62 Firstquarter. https://doi.org/10.1109/EMR.2020.3040016.
Gupta S, Modgil S, Meissonier R, Dwivedi YK (2021) Artificial intelligence and information system resilience to cope with supply chain disruption. In IEEE Transactions on Engineering Management. https://doi.org/10.1109/TEM.2021.3116770
Abdelgaber N, Nikolopoulos C (2021) Calculating the topological resilience of supply chain networks using quantum Hopfield neural networks. 2021 4th International Conference on Artificial Intelligence for Industries (AI4I), Laguna Hills, CA, USA, pp. 61–62. https://doi.org/10.1109/AI4I51902.2021.00023
Das D, Datta A, Kumar P et al (2022) Building supply chain resilience in the era of COVID-19: an AHP-DEMATEL approach. OperManag Res 15:249–267. https://doi.org/10.1007/s12063-021-00200-4
Kazancoglu I, Ozbiltekin-Pala M, Mangla SK et al (2022) Using emerging technologies to improve the sustainability and resilience of supply chains in a fuzzy environment in the context of COVID-19. Ann Oper Res. https://doi.org/10.1007/s10479-022-04775-4
Tsolakis N, Schumacher R, Dora M et al (2022) Artificial intelligence and blockchain implementation in supply chains: a pathway to sustainability and data monetisation? Ann Oper Res. https://doi.org/10.1007/s10479-022-04785-2
Pavlov D, Ivanov A, Dolgui, Sokolov B (2018) Hybrid fuzzy-probabilistic approach to supply chain resilience assessment in IEEE Transactions on Engineering Management. 65(2):303–315.https://doi.org/10.1109/TEM.2017.2773574
Tan WJ, Cai W, Li Z (2016) Adaptive resilient strategies for supply chain networks 2016 IEEE International Conference on Big Data (Big Data), Washington, DC, USA, p 3779–3784. https://doi.org/10.1109/BigData.2016.7841048
Author information
Authors and Affiliations
Contributions
Manikandan Rajagopal has conceived the research idea, prepared the manuscript and carried out the language check. Ramkumar Sivasakthivel has performed the data collection, survey, and model building.
Corresponding author
Ethics declarations
Competing Interests
The authors declare no competing interests.
Additional information
Publisher’s Note
Springer Nature remains neutral with regard to jurisdictional claims in published maps and institutional affiliations.
This article is part of the Topical Collection on Advances and Applications in Supply Chain Management
Rights and permissions
Springer Nature or its licensor (e.g. a society or other partner) holds exclusive rights to this article under a publishing agreement with the author(s) or other rightsholder(s); author self-archiving of the accepted manuscript version of this article is solely governed by the terms of such publishing agreement and applicable law.
About this article
Cite this article
Rajagopal, M., Sivasakthivel, R. An Empirical Framework Using Weighted Feed Forward Neural Network for Supply Chain Resilience (SCR) Strategy Selection. Oper. Res. Forum 5, 33 (2024). https://doi.org/10.1007/s43069-024-00313-z
Received:
Accepted:
Published:
DOI: https://doi.org/10.1007/s43069-024-00313-z