Abstract
Tea tree is an economically important crop. The rapid and efficient mapping of the distribution and dynamic changes in tea plantations informs decision making for government departments. It also plays an important role in rational tea planting and environmental governance. This study used the 10 m Sentinel-2 image data to map the spatial distribution of tea plantations in Yingde City, China. In this article, we analyzed the differences in vegetation and texture characteristics between the new and mature tea plantations. We found that the texture features of the new and mature tea plantations were significantly different in contrast, which can be used as an index for tea plantation extraction. Moreover, we selected machine learning classifiers, including support vector machine (SVM) and random forests (RF) method, which were utilized to extract the preliminary classification to complete spatial distribution mapping of tea plantations. The overall accuracy of SVM and RF was 90.79% and 89.42%, respectively, and the kappa coefficient was 0.88 and 0.86, respectively. SVM had the highest overall accuracy in terms of tea plantation distribution at the regional scale. These results demonstrate that: (1) separating tea plantations into mature and new tea plantations, taking into account vegetation and texture features such as soil brightness index and contrast, will help improve the accuracy of tea plantation classification and (2) using multi-period images combined with machine learning classification methods can improve the efficiency and accuracy of tea plantation identification.



Similar content being viewed by others
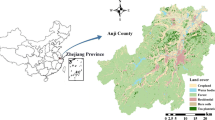
Data availability
The datasets generated during the current study are available from the corresponding author on reasonable request.
References
Ashourloo, D., Shahrabi, H., Azadbakht, M., Rad, A., Aghighi, H., & Radiom, S. (2020). A novel method for automatic potato mapping using time series of Sentinel-2 images. Computers and Electronics in Agriculture, 175, 105583. https://doi.org/10.1016/j.compag.2020.105583
Chander, G., & Markham, B. (2009). Summary of current radiometric calibration coefficients for Landsat MSS, TM, ETM+, and EO-1 ALI sensors. Remote Sensing of Environment, 113, 893–903. https://doi.org/10.1016/j.rse.2009.01.007
Chen, X., Wang, D., Li, J., Xu, T., Lai, K., Ding, Q., & Lin, M. (2020). A spectroscopic approach to detect and quantify phosmet residues in Oolong tea by surface-enhanced Raman scattering and silver nanoparticle substrate. Food Chemistry, 312, 126016. https://doi.org/10.1016/j.foodchem.2019.126016
Di, S., Zong, M., Li, S., Li, H., Duan, C., Peng, C., & Wang, D. (2020). The effects of the soil environment on soil organic carbon in tea plantations in Xishuangbanna, Southwestern China. Agriculture, Ecosystems and Environment, 297, 106951. https://doi.org/10.1016/j.agee.2020.106951
Dutta, R., Stein, A., & Bhagat, R. M. (2011). Integrating satellite images and spectroscopy to measuring green and black tea quality. Food Chemistry, 127(2), 866–874. https://doi.org/10.1016/j.foodchem.2010.12.160
Dutta, R., Stein, A., Smaling, E., Bhagat, R., & Hazarika, M. (2010). Effects of plant age and environmental and management factors on tea yield in Northeast India. Agronomy Journal - AGRON J. https://doi.org/10.2134/agronj2010.0091
Huang, J., Luo, Q., Liu, X., & Zhang, J. (2016). Winter wheat yield forecasting based on time series of MODIS NDVI. Transactions of the Chinese Society for Agricultural Machinery, 47(2), 295–301. https://doi.org/10.6041/j.issn.1000-1298.2016.02.039
Hunt, M., Blackburn, G., Carrasco, L., Redhead, J., & Rowland, C. (2019). High resolution wheat yield mapping using Sentinel-2. Remote Sensing of Environment, 233, 111410. https://doi.org/10.1016/j.rse.2019.111410
Immitzer, M., Vuolo, F., & Atzberger, C. (2016). First experience with sentinel-2 data for crop and tree species classifications in central Europe. Remote Sensing, 8, 166. https://doi.org/10.3390/rs8030166
Kalita, R. M., Das, A. K., & Nath, A. J. (2015). Allometric equations for estimating above- and belowground biomass in Tea (Camellia sinensis (L.) O. Kuntze) agroforestry system of Barak Valley, Assam, northeast India. Biomass and Bioenergy, 83, 42–49. https://doi.org/10.1016/j.biombioe.2015.08.017
Kim, H., Kim, W., & Kim, S. (2020). Damage assessment of rice crop after toluene exposure based on the vegetation index (VI) and UAV multispectral imagery. Remote Sensing, 13, 25. https://doi.org/10.3390/rs13010025
Kumar, B., & Dikshit, O. (2015). Integrating spectral and textural features for urban land cover classification with hyperspectral data. Joint Urban Remote Sensing Event,03,30. https://doi.org/10.1109/JURSE.2015.7120517
Kwak, G.-H., & Park, N.-W. (2019). Impact of texture information on crop classification with machine learning and UAV images. Applied Sciences, 9, 643. https://doi.org/10.3390/app9040643
Kwan, C., Gribben, D., Ayhan, B., Li, J., Bernabé, S., & Plaza, A. (2020). An accurate vegetation and non-vegetation differentiation approach based on land cover classification. Remote Sensing, 12, 3880. https://doi.org/10.3390/rs12233880
Li, L., Friedl, M., Xin, Q., Gray, J., Pan, Y., & Frolking, S. (2014). Mapping crop cycles in China using MODIS-EVI time series. Remote Sensing, 6, 2473–2493. https://doi.org/10.3390/rs6032473
Li, S., Wu, X., Xue, H., Gu, B., Cheng, H., Zeng, J., & Chang, J. (2011). Quantifying carbon storage for tea plantations in China. Agriculture, Ecosystems & Environment, 141(3), 390–398. https://doi.org/10.1016/j.agee.2011.04.003
Liu, Z., & Wang, S. (2018). Detecting changes of wheat vegetative growth and their response to climate change over the North China Plain. IEEE Journal of Selected Topics in Applied Earth Observations and Remote Sensing. https://doi.org/10.1109/JSTARS.2018.2870329
LiZhang, N. D. (2019). Mapping the spatial distribution of tea plantations using high-spatiotemporal-resolution imagery in Northern Zhejiang, China. Forests, 10, 856. https://doi.org/10.3390/f10100856
Luo, L., Xiao, X., Qin, Y., Wang, J., Xu, X., Hu, Y., & Qiao, Z. (2020). Mapping cropping intensity in China using time series Landsat and Sentinel-2 images and Google Earth Engine. Remote Sensing of Environment, 239, 111624. https://doi.org/10.1016/j.rse.2019.111624
Luo, Y. (2015). Tea cultivation (5th ed.). China Agriculture Press.
Ma, C., Yang, F., & Wang, X. (2019). Extracting tea plantations in southern hilly and mountainous region based on mesoscale spectrum and temporal phenological features. Remote Sensing for Land and Resources, 31(1), 141–148. https://doi.org/10.6046/gtzyyg.2019.01.19
Maxwell, A., Warner, T., & Fang, F. (2018). Implementation of machine-learning classification in remote sensing: An applied review. International Journal of Remote Sensing, 39, 2784–2817. https://doi.org/10.1080/01431161.2018.1433343
Mountrakis, G., Im, J., & Ogole, C. (2011). Support vector machines in remote sensing: A review. ISPRS Journal of Photogrammetry and Remote Sensing, 66, 247–259. https://doi.org/10.1016/j.isprsjprs.2010.11.001
Mulianga, B., Bégué, A., Simoes, M., & Todoroff, P. (2013). Forecasting regional sugarcane yield based on time integral and spatial aggregation of MODIS NDVI. Remote Sensing, 5(5), 2184–2199. https://doi.org/10.3390/rs5052184
Nguyen, H., Doan, T., Tomppo, E., & McRoberts, R. (2020). Land use/land cover mapping using multitemporal sentinel-2 imagery and four classification methods—a case study from Dak Nong, Vietnam. Remote Sensing, 12, 1367. https://doi.org/10.3390/rs12091367
Pal, M. (2008). Ensemble of support vector machines for land cover classification. International Journal of Remote Sensing, 29, 3043–3049. https://doi.org/10.1080/01431160802007624
Ramadanningrum, D. P., Kamal, M., & Murti, S. H. (2020). Image-based tea yield estimation using Landsat-8 OLI and Sentinel-2B images. Remote Sensing Applications: Society and Environment, 20, 100424. https://doi.org/10.1016/j.rsase.2020.100424
Silvero, N. E. Q., Demattê, J. A. M., Amorim, M. T. A., dos Santos, N. V., Rizzo, R., Safanelli, J. L., & Bonfatti, B. R. (2021). Soil variability and quantification based on Sentinel-2 and Landsat-8 bare soil images: A comparison. Remote Sensing of Environment, 252, 112117. https://doi.org/10.1016/j.rse.2020.112117
Sitokonstantinou, V., Papoutsis, I., Charalabos, K., Arnal, A., Andrés, A., & Zurbano, J. (2018). Scalable parcel-based crop identification scheme using sentinel-2 data time-series for the monitoring of the common agricultural policy. Remote Sensing, 10, 911. https://doi.org/10.3390/rs10060911
Su, S., Wan, C., Li, J., Jin, X., Pi, J., Zhang, Q., & Weng, M. (2017). Economic benefit and ecological cost of enlarging tea cultivation in subtropical China: Characterizing the trade-off for policy implications. Land Use Policy, 66, 183–195. https://doi.org/10.1016/j.landusepol.2017.04.044
Tucker, C. (1979). Red and photographic infrared linear combinations for monitoring vegetation. Remote Sensing of Environment. https://doi.org/10.1016/0034-4257(79)90013-0
Vieira, M., Formaggio, A., Rennó, C., Atzberger, C., Aguiar, D., & Mello, M. (2012). Object based image analysis and data mining applied to a remotely sensed Landsat time-series to map sugarcane over large areas. Remote Sensing of Environment, 123, 553–562. https://doi.org/10.1016/j.rse.2012.04.011
Wang, J., Xiao, X., Luo, L., Wu, X., Qin, Y., Steiner, J., & Dong, J. (2020). Mapping sugarcane plantation dynamics in Guangxi, China, by time series Sentinel-1, Sentinel-2 and Landsat images. Remote Sensing of Environment, 247, 111951. https://doi.org/10.1016/j.rse.2020.111951
Wang, M., Liu, Z., Baig, M. H. A., Wang, Y., Yurui, L., & Chen, Y. (2019). Mapping sugarcane in complex landscapes by integrating multi-temporal Sentinel-2 images and machine learning algorithms. Land Use Policy. https://doi.org/10.1016/j.landusepol.2019.104190
Wang, N., Xue, J., Peng, J., Biswas, A., He, Y., & Shi, Z. (2020). Integrating remote sensing and landscape characteristics to estimate soil salinity using machine learning methods: A case study from Southern Xinjiang, China. Remote Sensing, 12, 4118. https://doi.org/10.3390/rs12244118
Wang, S., He, X., & Ye, S. (2020). Soil aggregation and aggregate-associated carbon, nitrogen, and phosphorus under different aged tea (Camellia sinensis L.) plantations in hilly region of southern Guangxi, China. Scientia Horticulturae, 262, 109007. https://doi.org/10.1016/j.scienta.2019.109007
Wangchuk, S., & Bolch, T. (2020). Mapping of glacial lakes using Sentinel-1 and Sentinel-2 data and a random forest classifier: Strengths and challenges. Science of Remote Sensing, 2, 100008. https://doi.org/10.1016/j.srs.2020.100008
Zhang, H., Kang, J., Xu, X., & Zhang, L. (2020). Accessing the temporal and spectral features in crop type mapping using multi-temporal Sentinel-2 imagery: A case study of Yi’an County, Heilongjiang province, China. Computers and Electronics in Agriculture, 176, 105618. https://doi.org/10.1016/j.compag.2020.105618
Zhao, X., Wang, P., Jin, L., Tan, B., Zhao, X., Liu, D.(2020) The application of spectral characteristics of time series Sentinel-2A images in tea land extraction. Science of Surveying and Mapping, 45(6), 80–88. https://doi.org/10.16251/j.cnki.1009-2307.2020.03.012
Zhou, X., Wang, P., Tansey, K., Zhang, S., Li, H., & Tian, H. (2020). Reconstruction of time series leaf area index for improving wheat yield estimates at field scales by fusion of Sentinel-2, -3 and MODIS imagery. Computers and Electronics in Agriculture, 177, 105692. https://doi.org/10.1016/j.compag.2020.105692
Zhu, J., Pan, Z., Wang, H., Huang, P., Sun, J., Qin, F., & Liu, Z. (2019). An improved multi-temporal and multi-feature tea plantation identification method using sentinel-2 imagery. Sensors, 19, 2087. https://doi.org/10.3390/s19092087
Acknowledgements
We are grateful to Yingde City Agriculture and Rural Bureau for the statistical data support. We also greatly appreciated suggestions from anonymous reviewers and editor staff for the improvement of our manuscript.
Funding
This work was supported by Guangdong Province Key Field R&D Program Project (Project No. 2018B020241001).
Author information
Authors and Affiliations
Contributions
All authors prepared the methodological concept of this study, the original draft, as well as the editing of the manuscript. All authors have read and agreed to the published version of the manuscript. All authors have read and agreed to the published version of the manuscript.
Corresponding author
Ethics declarations
Conflicts of interest
The authors declare that they have no conflicts of interests.
Rights and permissions
About this article
Cite this article
Chen, P., Zhao, C., Duan, D. et al. Extracting tea plantations in complex landscapes using Sentinel-2 imagery and machine learning algorithms. COMMUNITY ECOLOGY 23, 163–172 (2022). https://doi.org/10.1007/s42974-022-00077-8
Received:
Accepted:
Published:
Issue Date:
DOI: https://doi.org/10.1007/s42974-022-00077-8