Abstract
Shear strength is a very important parameter in designing of reinforced concrete beams or concrete beams reinforced with fiber-reinforced polymer (FRP) bars. So far, numerous studies and design codes have proposed empirical-based formulas for predicting the shear strength of FRP-concrete beams. However, a difference exists between the proposed formulas and experimental results. This study predicts the shear strength of FRP-concrete beams using the novel hybrid BR-ANN model, which integrates artificial neural network (ANN) and Bayesian regularization (BR). For that, a comprehensive database consisting of 303 experimental results is compiled for developing the BR-ANN models. The performance results of BR-ANN are compared with those of 15 existing empirical formulas, which were proposed in typical design codes and well-known published studies. The predicted outputs are evaluated utilizing indicators, which are goodness of fit (\({R}^{2}\)), root mean squared error (\(\mathrm{RMSE}\)), and mean value of the ratio \({V}_{\mathrm{predict}} /{V}_{\mathrm{test}}\). The results reveal that the BR-ANN model outperforms other empirical formulas with a very high \({R}^{2}\) (0.987), very small \(\mathrm{RMSE}\) (7.3 kN). In addition, the mean value of the ratio \({V}_{\mathrm{predict}} /{V}_{\mathrm{test}}\) is equal to unity. Moreover, effects of input variables on the shear strength are evaluated. Finally, a practical design tool is developed to apply the BR-ANN model in calculating the shear strength of FRP-concrete beams.













Similar content being viewed by others
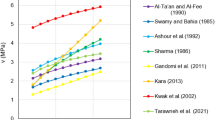
Data availability
The data used to support the findings of this study are included in the article.
References
AASHTO, L. (2009). Bridge design guide specifications for GFRP-reinforced concrete bridge decks and traffic railings. The American Association of State Highway and Transportation Officials.
ACI. (2015). ACI 440.1 R-15: Guide for the design & construction of structural concrete reinforced with FRP bars. American Concrete Institute.
Ahmed, A., Elkatatny, S., Ali, A., Mahmoud, M., & Abdulraheem, A. (2019). New model for pore pressure prediction while drilling using artificial neural networks. Arabian Journal for Science and Engineering, 44, 6079–6088. https://doi.org/10.1007/s13369-018-3574-7
Askar, M. K., Hassan, A. F., & Al-Kamaki, Y. S. (2022). Flexural and shear strengthening of reinforced concrete beams using FRP composites: A state of the art. Case Studies in Construction Materials, 17, e01189.
Asteris, P. G., and Mokos, V. G. (2019). Concrete compressive strength using artificial neural networks. Neural Computing and Applications, 32(15), 11807–11826.
Bentz, E. C., Massam, L., & Collins, M. P. (2010). Shear strength of large concrete members with FRP reinforcement. Journal of Composites for Construction, 14, 637–646.
BISE. (1999). Interim guidance on the design of reinforced concrete structures using fiber composite reinforcement. British Institution of Structural Engineers Seto Ltd.
Burden, F., & Winkler, D. (2009). Bayesian regularization of neural networks. In: D. J. Livingstone (Ed.), Artificial neural networks. Methods in molecular biology™, vol 458. Humana Press. https://doi.org/10.1007/978-1-60327-101-1_3
CNR-DT203. (2007). Guide for the design and construction of concrete structures reinforced with fiber-reinforced polymer bars. Advisory Committee on Technical Recommendations for Construction.
CSA. (2012). S806–12: Design & construction of building structures with fibre reinforced polymers. Canadian Standards Association.
Deitz, D., Harik, I., & Gesund, H. (1999). One-way slabs reinforced with glass fiber reinforced polymer reinforcing bars. Special Publication, 188, 279–286.
Eberhart, R., & Kennedy, J. (1995). Particle swarm optimization (pp. 1942–1948). Citeseer.
El-Sayed, A. K., El-Salakawy, E. F., & Benmokrane, B. (2006). Shear strength of FRP-reinforced concrete beams without transverse reinforcement. ACI Materials Journal, 103, 235.
Foresee, F. D., & Hagan, M. T. (1997). Gauss-Newton approximation to Bayesian learning. In: IEEE, pp. 1930–1935.
Hoult, N., Sherwood, E., Bentz, E. C., & Collins, M. P. (2008). Does the use of FRP reinforcement change the one-way shear behavior of reinforced concrete slabs? Journal of Composites for Construction, 12, 125–133.
ISIS-M03. (2007). Reinforcing concrete structures with fiber reinforced polymers. Canadian network of Centers of Excellence on Intelligent Sensing for Innovative Structures, University of Winnipeg.
JSCE. (1997). Recommendation for design and construction of concrete structures using continuous fiber reinforcing materials (Vol. 23). Japan Society of Civil Engineers, Concrete Engineering Series.
Jumaa, G. B., & Yousif, A. R. (2018). Predicting shear capacity of FRP-reinforced concrete beams without stirrups by artificial neural networks, gene expression programming, and regression analysis. Advances in Civil Engineering, 2018, 1–16.
Kara, I. F. (2011). Prediction of shear strength of FRP-reinforced concrete beams without stirrups based on genetic programming. Advances in Engineering Software, 42, 295–304.
Kaveh, A., & Bondarabady, H. R. (2004). Wavefront reduction using graphs, neural networks and genetic algorithm. International Journal for Numerical Methods in Engineering, 60, 1803–1815.
Kaveh, A., Gholipour, Y., & Rahami, H. (2008). Optimal design of transmission towers using genetic algorithm and neural networks. International Journal of Space Structures, 23, 1–19.
Kaveh, A., & Khalegi, A. (1998). Prediction of strength for concrete specimens using artificial neural networks. In B. H. V. Topping (Ed.), Advances in engineering computational technology (pp. 165–171). Edinburgh, UK: Civil-Comp Press, https://doi.org/10.4203/ccp.53.4.3.
Kaveh, A., & Khavaninzadeh, N. (2023). Efficient training of two ANNs using four meta-heuristic algorithms for predicting the FRP strength (pp. 256–272). Elsevier.
Kaveh, A., & Servati, H. (2001). Design of double layer grids using backpropagation neural networks. Computers & Structures, 79, 1561–1568.
Mai, S. H., Tran, V.-L., Nguyen, D.-D., Nguyen, V. T., & Thai, D.-K. (2022). Patch loading resistance prediction of steel plate girders using a deep artificial neural network and an interior-point algorith. Steel and Composite Structures, 45, 159.
Marani, A., & Nehdi, M. L. (2022). Predicting shear strength of FRP-reinforced concrete beams using novel synthetic data driven deep learning. Engineering Structures, 257, 114083.
Michaluk, C. R., Rizkalla, S. H., Tadros, G., & Benmokrane, B. (1998). Flexural behavior of one-way concrete slabs reinforced by fiber reinforced plastic reinforcements. Structural Journal, 95, 353–365.
Naderpour, H., Poursaeidi, O., & Ahmadi, M. (2018). Shear resistance prediction of concrete beams reinforced by FRP bars using artificial neural networks. Measurement, 126, 299–308.
Nehdi, M., El Chabib, H., & Saïd, A. A. (2007). Proposed shear design equations for FRP-reinforced concrete beams based on genetic algorithms approach. Journal of Materials in Civil Engineering, 19, 1033–1042.
Nguyen, D.-D., Tran, V.-L., Ha, D.-H., Nguyen, V.-Q., & Lee, T.-H. (2021a). A machine learning-based formulation for predicting shear capacity of squat flanged RC walls (pp. 1734–1747). Elsevier.
Nguyen, T.-H., Tran, N.-L., & Nguyen, D.-D. (2021b). Prediction of axial compression capacity of cold-formed steel oval hollow section columns using ANN and ANFIS models. International Journal of Steel Structures. https://doi.org/10.1007/s13296-021-00557-z
Nguyen, T.-H., Tran, N.-L., & Nguyen, D.-D. (2021c). Prediction of critical buckling load of web tapered I-section steel columns using artificial neural networks. International Journal of Steel Structures, 21(4), 1159–1181.
Nguyen, T.-H., Tran, N.-L., Phan, V.-T., & Nguyen, D.-D. (2023a). Improving axial load-carrying capacity prediction of concrete columns reinforced with longitudinal FRP bars using hybrid GA-ANN model. Asian Journal of Civil Engineering, 1–11. https://doi.org/10.1007/s42107-023-00695-1
Nguyen, T. -H., Tran, N. -L., Phan, V. -T., & Nguyen, D.-D. (2023b). Prediction of shear capacity of RC beams strengthened with FRCM composite using hybrid ANN-PSO model. Case Studies in Construction Materials, 18, e02183.
Nguyen, V.-Q., Tran, V.-L., Nguyen, D.-D., Sadiq, S., & Park, D. (2022). Novel hybrid MFO-XGBoost model for predicting the racking ratio of the rectangular tunnels subjected to seismic loading. Transportation Geotechnics, 37, 100878.
Nikoo, M., Aminnejad, B., & Lork, A. (2021). Predicting shear strength in FRP-reinforced concrete beams using bat algorithm-based artificial neural network. Advances in Materials Science and Engineering, 2021, 1–13.
Peng, F., Xue, W., & Xue, W. (2020). Database evaluation of shear strength of slender fiber-reinforced polymer-reinforced concrete members. ACI Structural Journal, 117, 273–281.
Rönnholm, M., Arve, K., Eränen, K., Klingstedt, F., Salmi, T., & Saxén, H. (2005). ANN modeling applied to NO X reduction with octane. Ann future in personal vehicles. In B. Ribeiro, R. F. Albrecht, A. Dobnikar, D. W. Pearson, & N. C. Steele (Eds.), Adaptive and Natural Computing Algorithms (pp. 100–103). Springer. https://doi.org/10.1007/3-211-27389-1_24
Selvan, S. S., Pandian, P. S., Subathira, A., & Saravanan, S. (2018). Comparison of response surface methodology (RSM) and artificial neural network (ANN) in optimization of aegle marmelos oil extraction for biodiesel production. Arabian Journal for Science and Engineering, 43, 6119–6131. https://doi.org/10.1007/s13369-018-3272-5
Shehata, E. F. (1999). Fibre-reinforced polymer (FRP) for shear reinforcement in concrete structures. Ph.D. dissertation (University of Manitoba, Winnipeg, Canada).
Simon, D. (2013). Evolutionary optimization algorithms. Wiley.
Sivanandam, S., Deepa, S., Sivanandam, S., & Deepa, S. (2008). Genetic algorithms. Springer.
Tottori, S., & Wakui, H. (1993). Shear capacity of RC and PC beams using FRP reinforcement. Special Publication, 138, 615–632.
Tran, N.-L., Nguyen, D.-D., & Nguyen, T.-H. (2022). Prediction of speed limit of cars moving on corroded steel girder bridges using artificial neural networks. Sādhanā, 47, 1–14.
Tran, V.-L., & Kim, S.-E. (2020). Efficiency of three advanced data-driven models for predicting axial compression capacity of CFDST columns. Thin-Walled Structures, 152, 106744. https://doi.org/10.1016/j.tws.2020.106744
Tran, V.-L., & Nguyen, D.-D. (2022). Novel hybrid WOA-GBM model for patch loading resistance prediction of longitudinally stiffened steel plate girders. Thin-Walled Structures, 177, 109424.
Tureyen, A. K., & Frosch, R. J. (2002). Shear tests of FRP-reinforced concrete beams without stirrups. Structural Journal, 99, 427–434.
Vakhshouri, B., & Nejadi, S. (2018). Prediction of compressive strength of self-compacting concrete by ANFIS models. Neurocomputing, 280, 13–22. https://doi.org/10.1016/j.neucom.2017.09.099
Wakjira, T. G., Al-Hamrani, A., Ebead, U., & Alnahhal, W. (2022). Shear capacity prediction of FRP-RC beams using single and ensenble ExPlainable Machine learning models. Composite Structures, 287, 115381.
Xue, W., Peng, F., & Zheng, Q. (2016). Design equations for flexural capacity of concrete beams reinforced with glass fiber–reinforced polymer bars. Journal of Composites for Construction, 20, 04015069.
Funding
No funding was used in this study.
Author information
Authors and Affiliations
Contributions
T-HN: conceptualization, software, visualization, writing—original draft. X-BN: methodology, data curation, writing—original draft. V-HN: validation; visualization. T-HTN: validation; visualization. D-DN: methodology, formal analysis, writing—original draft, writing—review and editing, supervision.
Corresponding author
Ethics declarations
Conflict of interest
The authors declare that they have no known competing financial interests or personal relationships that could have appeared to influence the work reported in this paper.
Additional information
Publisher's Note
Springer Nature remains neutral with regard to jurisdictional claims in published maps and institutional affiliations.
Appendix. The used database
Appendix. The used database
ID | \(b_{{\text{w}}}\) (mm) | \(d\) (mm) | \(a/d\) | \(a\) (mm) | \(f_{{\text{c}}}{\prime}\) (MPa) | \(\rho_{{\text{l}}}\) (%) | \(E_{{\text{f}}}\) (GPa) | \(E_{{\text{c}}}\) (MPa) | \(V\) (kN) |
---|---|---|---|---|---|---|---|---|---|
1 | 229 | 225 | 4.1 | 914 | 36 | 1.11 | 40.34 | 20 | 39.1 |
2 | 178 | 225 | 4.1 | 914 | 36 | 1.42 | 40.34 | 20 | 32.5 |
3 | 229 | 225 | 4.1 | 914 | 36 | 1.65 | 40.34 | 20 | 45.4 |
4 | 279 | 225 | 4.1 | 914 | 36 | 1.81 | 40.34 | 20 | 46.5 |
5 | 254 | 225 | 4.1 | 914 | 36 | 2.05 | 40.34 | 20 | 46.2 |
6 | 229 | 225 | 4.1 | 914 | 36 | 2.27 | 40.34 | 20 | 43.2 |
7 | 1000 | 165 | 6.06 | 1000 | 40 | 0.39 | 114 | 20 | 143.8 |
8 | 1000 | 165 | 6.06 | 1000 | 40 | 0.78 | 114 | 20 | 170.8 |
9 | 1000 | 161 | 6.21 | 1000 | 40 | 1.17 | 114 | 20 | 193.8 |
10 | 1000 | 162 | 6.17 | 1000 | 40 | 0.86 | 40 | 20 | 116.8 |
11 | 1000 | 159 | 6.29 | 1000 | 40 | 1.7 | 40 | 20 | 145.8 |
12 | 1000 | 162 | 6.17 | 1000 | 40 | 1.71 | 40 | 20 | 166.8 |
13 | 1000 | 159 | 6.29 | 1000 | 40 | 2.44 | 40 | 20 | 166.8 |
14 | 1000 | 154 | 6.49 | 1000 | 40 | 2.63 | 40 | 20 | 171.8 |
15 | 250 | 326 | 3.07 | 1000 | 50 | 0.87 | 128 | 20 | 79.7 |
16 | 250 | 326 | 3.07 | 1000 | 50 | 0.87 | 39 | 20 | 72.7 |
17 | 250 | 326 | 3.07 | 1000 | 45 | 1.24 | 134 | 20 | 106.2 |
18 | 250 | 326 | 3.07 | 1000 | 45 | 1.22 | 42 | 20 | 62.2 |
19 | 250 | 326 | 3.07 | 1000 | 44 | 1.72 | 134 | 20 | 126.7 |
20 | 250 | 326 | 3.07 | 1000 | 44 | 1.71 | 42 | 20 | 79.7 |
21 | 250 | 326 | 3.07 | 1000 | 63 | 1.71 | 135 | 20 | 132.2 |
22 | 250 | 326 | 3.07 | 1000 | 63 | 1.71 | 42 | 20 | 89.2 |
23 | 250 | 326 | 3.07 | 1000 | 63 | 2.2 | 135 | 20 | 176.2 |
24 | 250 | 326 | 3.07 | 1000 | 63 | 2.2 | 42 | 20 | 117.7 |
25 | 600 | 262 | 6.68 | 1750 | 68 | 0.77 | 48 | 20 | 89.2 |
26 | 600 | 262 | 6.68 | 1750 | 68 | 1.53 | 48 | 20 | 116.2 |
27 | 150 | 180 | 3.7 | 667 | 28 | 0.45 | 38 | 20 | 13 |
28 | 150 | 220 | 3.03 | 667 | 28 | 0.71 | 32 | 20 | 18.1 |
29 | 150 | 240 | 2.78 | 667 | 28 | 0.86 | 32 | 20 | 25.8 |
30 | 150 | 180 | 3.7 | 667 | 49 | 1.39 | 32 | 20 | 18 |
31 | 150 | 220 | 3.03 | 667 | 49 | 1.06 | 32 | 20 | 28.1 |
32 | 150 | 240 | 2.78 | 667 | 49 | 1.15 | 32 | 20 | 30.8 |
33 | 457 | 360 | 3.4 | 1219 | 35 | 0.96 | 40.54 | 20 | 108.1 |
34 | 457 | 360 | 3.4 | 1219 | 35 | 0.96 | 37.88 | 20 | 94.7 |
35 | 457 | 360 | 3.4 | 1219 | 35 | 0.96 | 47.1 | 20 | 114.8 |
36 | 457 | 360 | 3.4 | 1219 | 35 | 1.92 | 40.54 | 20 | 137 |
37 | 457 | 360 | 3.4 | 1219 | 35 | 1.92 | 37.88 | 20 | 152.6 |
38 | 457 | 360 | 3.4 | 1219 | 35 | 1.92 | 47.1 | 20 | 177 |
39 | 200 | 225 | 2.67 | 600 | 41 | 0.25 | 145 | 20 | 37 |
40 | 200 | 225 | 2.67 | 600 | 49 | 0.5 | 145 | 20 | 47.9 |
41 | 200 | 225 | 2.67 | 600 | 41 | 0.63 | 145 | 20 | 48.1 |
42 | 200 | 225 | 2.67 | 600 | 41 | 0.88 | 145 | 20 | 43.6 |
43 | 200 | 225 | 3.56 | 800 | 41 | 0.5 | 145 | 20 | 47.8 |
44 | 200 | 225 | 4.5 | 950 | 41 | 0.5 | 145 | 20 | 39.2 |
45 | 150 | 250 | 3 | 750 | 34 | 1.51 | 105 | 20 | 45.6 |
46 | 150 | 250 | 3 | 750 | 34 | 3.02 | 105 | 20 | 46.6 |
47 | 150 | 250 | 3 | 750 | 34 | 2.27 | 105 | 20 | 41.1 |
48 | 178 | 279 | 2.69 | 750 | 24 | 2.3 | 40 | 20 | 54 |
49 | 178 | 287 | 2.61 | 750 | 24 | 0.77 | 40 | 20 | 36.6 |
50 | 178 | 287 | 2.61 | 750 | 24 | 1.34 | 40 | 20 | 40.6 |
51 | 160 | 346 | 2.75 | 952 | 37 | 0.72 | 42 | 20 | 60.3 |
52 | 160 | 346 | 3.32 | 1149 | 43 | 1.1 | 42 | 20 | 45.1 |
53 | 160 | 325 | 3.54 | 1151 | 34 | 1.54 | 42 | 20 | 47.8 |
54 | 130 | 310 | 3.06 | 949 | 37 | 0.72 | 120 | 20 | 48.5 |
55 | 130 | 310 | 3.71 | 1150 | 43 | 1.1 | 120 | 20 | 51 |
56 | 130 | 310 | 3.71 | 1150 | 34 | 1.54 | 120 | 20 | 57.9 |
57 | 305 | 158 | 4.5 | 710 | 29 | 0.73 | 40 | 20 | 27.8 |
58 | 305 | 158 | 5.8 | 913 | 30 | 0.73 | 40 | 20 | 29.6 |
59 | 305 | 158 | 5.8 | 913 | 27 | 0.73 | 40 | 20 | 30.5 |
60 | 150 | 210 | 3.65 | 767 | 38 | 1.31 | 45 | 20 | 27.2 |
61 | 150 | 210 | 3.65 | 767 | 33 | 1.36 | 45 | 20 | 22.7 |
62 | 450 | 937 | 3.26 | 3050 | 46 | 0.51 | 37 | 20 | 142.3 |
63 | 450 | 438 | 3.48 | 1525 | 35 | 0.55 | 37 | 20 | 87.8 |
64 | 450 | 194 | 3.93 | 762 | 35 | 0.66 | 37 | 20 | 55.1 |
65 | 450 | 857 | 3.56 | 3050 | 36 | 2.23 | 37 | 20 | 240.3 |
66 | 450 | 405 | 3.77 | 1525 | 35 | 2.36 | 37 | 20 | 140.3 |
67 | 450 | 188 | 4.05 | 762 | 35 | 2.54 | 37 | 20 | 74.6 |
68 | 200 | 220 | 2.5 | 550 | 30 | 0.32 | 146.2 | 20 | 35.4 |
69 | 150 | 220 | 2.5 | 550 | 30 | 0.43 | 146.2 | 20 | 25 |
70 | 150 | 220 | 2.5 | 550 | 30 | 0.77 | 147.9 | 20 | 26.1 |
71 | 200 | 220 | 3.5 | 770 | 30 | 0.32 | 146.2 | 20 | 29.4 |
72 | 150 | 220 | 3.5 | 770 | 30 | 0.43 | 146.2 | 20 | 26.9 |
73 | 150 | 220 | 3.5 | 770 | 30 | 0.77 | 147.9 | 20 | 29.6 |
74 | 200 | 220 | 4.5 | 990 | 30 | 0.32 | 146.2 | 20 | 26.6 |
75 | 150 | 220 | 4.5 | 990 | 30 | 0.43 | 146.2 | 20 | 24.6 |
76 | 150 | 220 | 4.5 | 990 | 30 | 0.77 | 147.9 | 20 | 28.1 |
77 | 200 | 220 | 2.5 | 550 | 30 | 0.32 | 48.2 | 20 | 25.7 |
78 | 150 | 220 | 2.5 | 550 | 30 | 0.43 | 48.2 | 20 | 24.4 |
79 | 150 | 220 | 2.5 | 550 | 30 | 0.77 | 49.1 | 20 | 27.3 |
80 | 200 | 220 | 3.5 | 770 | 30 | 0.32 | 48.2 | 20 | 27.2 |
81 | 150 | 220 | 3.5 | 770 | 30 | 0.43 | 48.2 | 20 | 21.1 |
82 | 150 | 220 | 3.5 | 770 | 30 | 0.77 | 49.1 | 20 | 19.5 |
83 | 200 | 220 | 4.5 | 990 | 30 | 0.32 | 48.2 | 20 | 20 |
84 | 150 | 220 | 4.5 | 990 | 30 | 0.43 | 48.2 | 20 | 17.2 |
85 | 150 | 220 | 4.5 | 990 | 30 | 0.77 | 49.1 | 20 | 20.5 |
86 | 200 | 220 | 3 | 660 | 34 | 0.32 | 146.2 | 20 | 26.2 |
87 | 150 | 220 | 3 | 660 | 34 | 0.43 | 146.2 | 20 | 19.2 |
88 | 200 | 220 | 3 | 660 | 40 | 0.32 | 146.2 | 20 | 23.6 |
89 | 150 | 220 | 3 | 660 | 40 | 0.43 | 146.2 | 20 | 21.4 |
90 | 150 | 220 | 3 | 660 | 40 | 0.77 | 147.9 | 20 | 26.5 |
91 | 200 | 220 | 3 | 660 | 34 | 0.32 | 48.2 | 20 | 21.1 |
92 | 150 | 220 | 3 | 660 | 34 | 0.43 | 48.2 | 20 | 18.9 |
93 | 200 | 220 | 3 | 660 | 40 | 0.32 | 48.2 | 20 | 20.8 |
94 | 150 | 220 | 3 | 660 | 40 | 0.43 | 48.2 | 20 | 20.3 |
95 | 150 | 220 | 3 | 660 | 40 | 0.77 | 49.1 | 20 | 21.8 |
96 | 150 | 223 | 3.3 | 750 | 40 | 1.1 | 45 | 20 | 27.9 |
97 | 457 | 883 | 3.1 | 2743 | 30 | 0.6 | 41 | 20 | 179.6 |
98 | 457 | 883 | 3.1 | 2743 | 30 | 0.6 | 41 | 20 | 176.9 |
99 | 114 | 292 | 3.1 | 914 | 32 | 0.6 | 43.2 | 20 | 19.8 |
100 | 114 | 292 | 3.1 | 914 | 32 | 0.6 | 43.2 | 20 | 18.5 |
101 | 229 | 146 | 3.1 | 457 | 60 | 0.6 | 43.2 | 20 | 29 |
102 | 229 | 146 | 3.1 | 457 | 32 | 0.6 | 43.2 | 20 | 37.3 |
103 | 229 | 146 | 3.1 | 457 | 32 | 0.6 | 43.2 | 20 | 26.7 |
104 | 457 | 880 | 3.1 | 2743 | 30 | 1.2 | 41 | 20 | 246.2 |
105 | 457 | 880 | 3.1 | 2743 | 31 | 1.2 | 41 | 20 | 238.2 |
106 | 114 | 292 | 3.1 | 914 | 41 | 1.21 | 48.2 | 20 | 22.6 |
107 | 114 | 292 | 3.1 | 914 | 41 | 1.21 | 48.2 | 20 | 21.2 |
108 | 229 | 146 | 3.1 | 457 | 41 | 1.2 | 48.2 | 20 | 33.4 |
109 | 229 | 146 | 3.1 | 457 | 41 | 1.2 | 48.2 | 20 | 32.9 |
110 | 150 | 180 | 5.6 | 1000 | 20 | 0.87 | 115 | 20 | 19.1 |
111 | 150 | 180 | 5.6 | 1000 | 20 | 1.46 | 115 | 20 | 24.4 |
112 | 150 | 180 | 5.6 | 1000 | 27 | 0.87 | 115 | 20 | 26.2 |
113 | 150 | 180 | 5.6 | 1000 | 27 | 1.46 | 115 | 20 | 27.5 |
114 | 250 | 305 | 2.5 | 763 | 39 | 0.84 | 48 | 20 | 62.8 |
115 | 250 | 305 | 3.5 | 1068 | 39 | 0.84 | 48 | 20 | 45.9 |
116 | 250 | 310 | 2.5 | 775 | 33 | 0.42 | 144 | 20 | 79 |
117 | 250 | 310 | 3.5 | 1085 | 33 | 0.42 | 144 | 20 | 61.1 |
118 | 250 | 440 | 2.5 | 1100 | 43 | 0.89 | 48 | 20 | 132.5 |
119 | 300 | 584 | 2.5 | 1460 | 36 | 0.91 | 48 | 20 | 118.1 |
120 | 250 | 442 | 2.5 | 1105 | 72 | 1.46 | 48.2 | 20 | 119.2 |
121 | 300 | 578 | 2.5 | 1445 | 72 | 1.51 | 48.2 | 20 | 160.5 |
122 | 250 | 460 | 2.5 | 1150 | 41 | 0.44 | 144 | 20 | 67.6 |
123 | 300 | 594 | 2.5 | 1485 | 36 | 0.43 | 144 | 20 | 143.7 |
124 | 250 | 449 | 2.5 | 1123 | 72 | 0.82 | 144 | 20 | 103.5 |
125 | 300 | 594 | 2.5 | 1485 | 72 | 0.73 | 144 | 20 | 151.3 |
126 | 250 | 296 | 2.5 | 740 | 36 | 1.41 | 46.3 | 20 | 67.3 |
127 | 250 | 296 | 2.5 | 740 | 36 | 1.41 | 46.3 | 20 | 72.7 |
128 | 250 | 455 | 2.5 | 1138 | 41 | 0.35 | 46.3 | 20 | 71.1 |
129 | 250 | 434 | 2.5 | 1085 | 41 | 1.46 | 46.3 | 20 | 95.3 |
130 | 250 | 310 | 2.5 | 775 | 41 | 0.18 | 144 | 20 | 60.5 |
131 | 250 | 310 | 2.5 | 775 | 41 | 0.66 | 144 | 20 | 74.3 |
132 | 250 | 460 | 2.5 | 1150 | 41 | 0.22 | 144 | 20 | 73.3 |
133 | 250 | 439 | 2.5 | 1098 | 41 | 0.65 | 144 | 20 | 85.6 |
134 | 250 | 291 | 2.5 | 728 | 63 | 0.87 | 46.3 | 20 | 77.4 |
135 | 250 | 291 | 2.5 | 728 | 85 | 0.87 | 46.3 | 20 | 82 |
136 | 250 | 310 | 2.5 | 775 | 63 | 0.42 | 144 | 20 | 73.4 |
137 | 300 | 150 | 4 | 600 | 23 | 1.34 | 29 | 20 | 33.5 |
138 | 300 | 150 | 4 | 600 | 28 | 1.79 | 29 | 20 | 36.5 |
139 | 154 | 222 | 3.15 | 699 | 39 | 1.55 | 34 | 20 | 39.7 |
140 | 635 | 202 | 6.04 | 1220 | 72 | 0.94 | 43.3 | 20 | 138.4 |
141 | 635 | 202 | 6.04 | 1220 | 87 | 0.94 | 43.3 | 20 | 136.6 |
142 | 635 | 202 | 6.04 | 1220 | 60 | 0.94 | 43.3 | 20 | 125 |
143 | 635 | 202 | 6.04 | 1220 | 63 | 0.94 | 43.3 | 20 | 113.9 |
144 | 635 | 202 | 6.04 | 1220 | 75 | 0.94 | 43.3 | 20 | 104.1 |
145 | 635 | 202 | 6.04 | 1220 | 60 | 0.94 | 43.3 | 20 | 104.6 |
146 | 635 | 227 | 4.47 | 1015 | 68 | 0.94 | 43.3 | 20 | 124 |
147 | 635 | 240 | 6.04 | 1450 | 79 | 0.79 | 43.3 | 20 | 109 |
148 | 635 | 240 | 6.04 | 1450 | 61 | 0.79 | 43.3 | 20 | 126.4 |
149 | 635 | 240 | 6.04 | 1450 | 63 | 0.79 | 43.3 | 20 | 101 |
150 | 635 | 240 | 6.04 | 1450 | 67 | 0.79 | 43.3 | 20 | 106.8 |
151 | 1854 | 202 | 6.04 | 1220 | 84 | 0.96 | 43.3 | 20 | 396.3 |
152 | 1854 | 202 | 6.04 | 1220 | 59 | 0.96 | 43.3 | 20 | 330 |
153 | 1854 | 202 | 6.04 | 1220 | 63 | 0.96 | 43.3 | 20 | 279.3 |
154 | 1854 | 202 | 6.04 | 1220 | 63 | 0.96 | 43.3 | 20 | 294.9 |
155 | 1854 | 202 | 6.04 | 1220 | 57 | 0.96 | 43.3 | 20 | 303.8 |
156 | 1854 | 202 | 6.04 | 1220 | 56 | 0.96 | 43.3 | 20 | 307.3 |
157 | 1854 | 202 | 6.04 | 1220 | 84 | 0.54 | 43.3 | 20 | 282.9 |
158 | 1854 | 202 | 6.04 | 1220 | 63 | 0.54 | 43.3 | 20 | 254 |
159 | 1854 | 202 | 6.04 | 1220 | 56 | 0.54 | 43.3 | 20 | 225.9 |
160 | 300 | 200 | 3.5 | 700 | 52 | 0.35 | 114 | 20 | 64.6 |
161 | 300 | 300 | 3.5 | 1050 | 52 | 0.32 | 114 | 20 | 62.3 |
162 | 300 | 400 | 3.5 | 1400 | 52 | 0.3 | 114 | 20 | 57.3 |
163 | 300 | 500 | 3.5 | 1750 | 52 | 0.28 | 114 | 20 | 71.5 |
164 | 300 | 400 | 6.5 | 2600 | 52 | 0.3 | 114 | 20 | 55.2 |
165 | 300 | 400 | 6 | 2400 | 52 | 0.3 | 114 | 20 | 65.9 |
166 | 150 | 280 | 2.5 | 700 | 45 | 0.11 | 148 | 20 | 23.2 |
167 | 150 | 280 | 5 | 1400 | 49 | 0.11 | 148 | 20 | 13.6 |
168 | 150 | 280 | 2.5 | 700 | 46 | 0.21 | 148 | 20 | 28.2 |
169 | 150 | 280 | 2.5 | 700 | 24 | 0.11 | 148 | 20 | 23.2 |
170 | 400 | 250 | 3 | 750 | 51 | 0.57 | 47.5 | 20 | 57.1 |
171 | 400 | 250 | 3 | 750 | 49 | 0.86 | 47.5 | 20 | 75.1 |
172 | 400 | 250 | 3 | 750 | 51 | 1.14 | 47.5 | 20 | 86.1 |
173 | 400 | 250 | 3 | 750 | 51 | 1.71 | 47.5 | 20 | 109.1 |
174 | 400 | 250 | 3 | 750 | 52 | 2.28 | 47.5 | 20 | 116.1 |
175 | 400 | 250 | 3 | 750 | 50 | 0.86 | 47.5 | 20 | 79.1 |
176 | 400 | 250 | 4 | 1000 | 51 | 1.14 | 47.5 | 20 | 90.4 |
177 | 400 | 250 | 6 | 1500 | 50 | 1.71 | 47.5 | 20 | 91.1 |
178 | 400 | 250 | 8 | 2000 | 48 | 2.28 | 47.5 | 20 | 84.8 |
179 | 400 | 250 | 3 | 750 | 51 | 4.05 | 51.9 | 20 | 136.1 |
180 | 400 | 250 | 8 | 2000 | 48 | 4.05 | 51.9 | 20 | 99.8 |
181 | 200 | 250 | 4 | 1000 | 31 | 0.68 | 41.3 | 20 | 24.5 |
182 | 200 | 250 | 4 | 1000 | 31 | 0.79 | 41.3 | 20 | 26.6 |
183 | 200 | 250 | 4 | 1000 | 31 | 1.08 | 41.3 | 20 | 33.4 |
184 | 200 | 250 | 3 | 750 | 35 | 0.51 | 41.3 | 20 | 30.5 |
185 | 200 | 250 | 3 | 750 | 35 | 0.76 | 41.3 | 20 | 39.9 |
186 | 200 | 250 | 3 | 750 | 35 | 1.01 | 41.3 | 20 | 37.8 |
187 | 200 | 370 | 2.7 | 1000 | 22 | 0.12 | 141 | 20 | 34.7 |
188 | 200 | 370 | 2.7 | 1000 | 22 | 0.24 | 141 | 20 | 37.9 |
189 | 200 | 270 | 3.6 | 1000 | 29 | 0.16 | 141 | 20 | 34.2 |
190 | 200 | 270 | 3.6 | 1000 | 29 | 0.33 | 141 | 20 | 34.2 |
191 | 200 | 170 | 5.9 | 1000 | 24 | 0.26 | 141 | 20 | 18.5 |
192 | 200 | 170 | 5.9 | 1000 | 24 | 0.52 | 141 | 20 | 21.7 |
193 | 1000 | 140 | 6.07 | 850 | 48 | 1.01 | 41 | 20 | 100.6 |
194 | 1000 | 140 | 6.07 | 850 | 48 | 0.79 | 47.6 | 20 | 125.1 |
195 | 1000 | 140 | 6.07 | 850 | 48 | 1.01 | 47.6 | 20 | 112.1 |
196 | 1000 | 140 | 6.07 | 850 | 48 | 1.22 | 47.6 | 20 | 125.1 |
197 | 1000 | 140 | 6.07 | 850 | 50 | 1.42 | 47.6 | 20 | 170 |
198 | 1000 | 137.5 | 6.18 | 850 | 48 | 1.85 | 51.9 | 20 | 151.8 |
199 | 1000 | 140 | 6.07 | 850 | 43 | 1.01 | 69.5 | 20 | 174.6 |
200 | 1000 | 140 | 6.07 | 850 | 49 | 1.21 | 69.5 | 20 | 128.1 |
201 | 1000 | 140 | 6.07 | 850 | 49 | 2.43 | 69.5 | 20 | 126.6 |
202 | 1000 | 137.5 | 6.18 | 850 | 50 | 2.55 | 69.5 | 20 | 127.6 |
203 | 1000 | 140 | 6.07 | 850 | 77 | 1.01 | 69.5 | 20 | 161.3 |
204 | 1000 | 140 | 6.07 | 850 | 83 | 1.03 | 69.5 | 20 | 143.7 |
205 | 1000 | 143.5 | 5.92 | 850 | 50 | 0.45 | 144 | 20 | 173.9 |
206 | 1000 | 143.5 | 5.92 | 850 | 50 | 0.54 | 144 | 20 | 147.6 |
207 | 1000 | 143.5 | 5.92 | 850 | 52 | 0.63 | 144 | 20 | 165.6 |
208 | 1000 | 143.5 | 5.92 | 850 | 45 | 0.72 | 144 | 20 | 163.6 |
209 | 1000 | 143.5 | 5.96 | 855 | 46 | 0.84 | 140 | 20 | 179.6 |
210 | 1000 | 143.5 | 5.96 | 855 | 49 | 0.98 | 140 | 20 | 192.6 |
211 | 1000 | 143.5 | 5.96 | 855 | 41 | 1.11 | 140 | 20 | 198.6 |
212 | 1000 | 143.5 | 5.92 | 850 | 76 | 0.63 | 144 | 20 | 174.6 |
213 | 1000 | 143.5 | 5.92 | 850 | 86 | 0.63 | 144 | 20 | 219.4 |
214 | 203 | 225 | 4.06 | 914 | 80 | 1.25 | 40.3 | 20 | 38.9 |
215 | 152 | 225 | 4.06 | 914 | 80 | 1.66 | 40.3 | 20 | 33.2 |
216 | 165 | 225 | 4.06 | 914 | 80 | 2.1 | 40.3 | 20 | 36.5 |
217 | 203 | 224 | 4.06 | 914 | 80 | 2.56 | 40.3 | 20 | 47.3 |
218 | 127 | 143 | 6.36 | 909 | 60 | 0.33 | 139 | 20 | 14.3 |
219 | 159 | 141 | 6.45 | 909 | 62 | 0.58 | 139 | 20 | 20.3 |
220 | 89 | 143 | 6.36 | 909 | 81 | 0.47 | 139 | 20 | 10 |
221 | 121 | 141 | 6.45 | 909 | 81 | 0.76 | 139 | 20 | 15.7 |
222 | 150 | 250 | 3 | 750 | 28 | 0.55 | 94 | 20 | 38.3 |
223 | 150 | 250 | 3 | 750 | 33 | 1.1 | 94 | 20 | 43.8 |
224 | 150 | 250 | 3 | 750 | 31 | 1.39 | 94 | 20 | 48.3 |
225 | 150 | 250 | 3 | 750 | 35 | 2.2 | 94 | 20 | 59.1 |
226 | 150 | 250 | 2.5 | 625 | 34 | 1.04 | 100 | 20 | 38.8 |
227 | 300 | 500 | 2.5 | 1250 | 30 | 1.04 | 100 | 20 | 145.4 |
228 | 150 | 170 | 4.12 | 700 | 24 | 0.92 | 45.8 | 20 | 12.7 |
229 | 150 | 170 | 4.12 | 700 | 24 | 1.54 | 45.8 | 20 | 13.6 |
230 | 150 | 170 | 4.12 | 700 | 31 | 0.92 | 45.8 | 20 | 14.1 |
231 | 150 | 170 | 4.12 | 700 | 31 | 1.54 | 45.8 | 20 | 15.3 |
232 | 300 | 441 | 3.02 | 1330 | 42 | 3.65 | 62.6 | 20 | 145.4 |
233 | 300 | 412 | 3.16 | 1300 | 43 | 3.25 | 44 | 20 | 153.9 |
234 | 300 | 404 | 3.71 | 1500 | 28 | 3.98 | 62.6 | 20 | 107.6 |
235 | 420 | 83 | 3.61 | 300 | 61 | 0.61 | 42 | 20 | 19.9 |
236 | 420 | 82 | 6.1 | 500 | 61 | 1.1 | 42 | 20 | 25.5 |
237 | 420 | 80 | 6.25 | 500 | 61 | 1.77 | 40 | 20 | 32 |
238 | 420 | 78 | 6.41 | 500 | 61 | 2.61 | 40 | 20 | 40.5 |
239 | 420 | 83 | 3.61 | 300 | 74 | 0.61 | 42 | 20 | 24.9 |
240 | 420 | 82 | 6.1 | 500 | 74 | 1.1 | 42 | 20 | 22 |
241 | 420 | 80 | 6.25 | 500 | 74 | 1.77 | 40 | 20 | 32 |
242 | 420 | 78 | 6.41 | 500 | 74 | 2.61 | 40 | 20 | 36.5 |
243 | 420 | 83 | 3.61 | 300 | 93 | 0.61 | 42 | 20 | 35.9 |
244 | 420 | 82 | 6.1 | 500 | 93 | 1.1 | 42 | 20 | 23 |
245 | 420 | 80 | 6.25 | 500 | 93 | 1.77 | 40 | 20 | 32 |
246 | 420 | 78 | 6.41 | 500 | 93 | 2.61 | 40 | 20 | 38.5 |
247 | 420 | 75 | 6 | 450 | 48 | 0.68 | 42 | 20 | 24.8 |
248 | 420 | 73 | 6.16 | 450 | 48 | 0.93 | 42 | 20 | 27.6 |
249 | 420 | 73 | 6.16 | 450 | 48 | 1.16 | 42 | 20 | 29.6 |
250 | 420 | 75 | 6 | 450 | 76 | 0.68 | 42 | 20 | 27.5 |
251 | 420 | 73 | 6.16 | 450 | 76 | 0.93 | 42 | 20 | 26.5 |
252 | 420 | 73 | 6.16 | 450 | 76 | 1.16 | 42 | 20 | 31.4 |
253 | 420 | 75 | 6 | 450 | 92 | 0.68 | 42 | 20 | 23.7 |
254 | 420 | 73 | 6.16 | 450 | 92 | 0.93 | 42 | 20 | 25.7 |
255 | 420 | 73 | 6.16 | 450 | 92 | 1.16 | 42 | 20 | 34.5 |
256 | 150 | 270 | 4.07 | 1100 | 60 | 0.39 | 70 | 20 | 19.8 |
257 | 150 | 270 | 4.07 | 1100 | 60 | 0.51 | 70 | 20 | 23 |
258 | 200 | 270 | 2.5 | 675 | 47 | 1.82 | 64 | 20 | 82.1 |
259 | 200 | 270 | 2.5 | 675 | 47 | 2.23 | 64 | 20 | 75.7 |
260 | 200 | 270 | 2.5 | 675 | 47 | 2.51 | 64 | 20 | 63.1 |
261 | 400 | 575 | 2.92 | 1680 | 32 | 1 | 61.2 | 20 | 159.5 |
262 | 400 | 575 | 2.92 | 1680 | 40 | 1 | 71.2 | 20 | 169 |
263 | 400 | 575 | 2.92 | 1680 | 102 | 1 | 61.2 | 20 | 166 |
264 | 600 | 262 | 6.68 | 1750 | 58 | 0.76 | 55.4 | 20 | 89.2 |
265 | 600 | 262 | 6.68 | 1750 | 58 | 1.51 | 55.4 | 20 | 116.2 |
266 | 1200 | 180 | 5.8 | 1050 | 33 | 0.66 | 44 | 20 | 140.7 |
267 | 1200 | 180 | 5.8 | 1050 | 33 | 0.88 | 44 | 20 | 151.7 |
268 | 1200 | 180 | 5.8 | 1050 | 33 | 1.1 | 44 | 20 | 158.7 |
269 | 1200 | 180 | 5.8 | 1050 | 33 | 0.33 | 50 | 20 | 102.8 |
270 | 1200 | 180 | 5.8 | 1050 | 33 | 0.44 | 50 | 20 | 133.1 |
271 | 1200 | 180 | 5.8 | 1050 | 33 | 0.57 | 50 | 20 | 166.6 |
272 | 152 | 220 | 3.3 | 725 | 49 | 0.3 | 50 | 20 | 17.4 |
273 | 152 | 220 | 3.3 | 725 | 49 | 0.47 | 50 | 20 | 23.5 |
274 | 152 | 220 | 3.3 | 725 | 49 | 0.68 | 50 | 20 | 19 |
275 | 152 | 220 | 3.3 | 725 | 49 | 0.94 | 50 | 20 | 28.4 |
276 | 152 | 220 | 3.3 | 725 | 49 | 1.35 | 50 | 20 | 30.4 |
277 | 152 | 220 | 2.5 | 550 | 49 | 0.3 | 50 | 20 | 20 |
278 | 152 | 220 | 2.5 | 550 | 49 | 0.47 | 50 | 20 | 32.1 |
279 | 152 | 220 | 2.5 | 550 | 49 | 0.68 | 50 | 20 | 27.5 |
280 | 200 | 170 | 5.56 | 945 | 36 | 1.21 | 53 | 20 | 30.9 |
281 | 200 | 170 | 5.56 | 945 | 36 | 2 | 51 | 20 | 40.3 |
282 | 200 | 170 | 5.56 | 945 | 36 | 3.09 | 51 | 20 | 46.8 |
283 | 200 | 170 | 7 | 1190 | 36 | 3.74 | 51 | 20 | 41.6 |
284 | 200 | 165 | 7 | 1155 | 36 | 4.64 | 48 | 20 | 49.8 |
285 | 200 | 165 | 7 | 1155 | 36 | 6.18 | 48 | 20 | 52.9 |
286 | 100 | 180 | 5.56 | 1000 | 41 | 0.74 | 40.8 | 20 | 10.2 |
287 | 100 | 180 | 5.56 | 1000 | 41 | 1.48 | 40.8 | 20 | 12.3 |
288 | 100 | 180 | 5.56 | 1000 | 66 | 0.35 | 124 | 20 | 9.1 |
289 | 100 | 180 | 5.56 | 1000 | 66 | 0.71 | 124 | 20 | 14.2 |
290 | 150 | 379 | 2.9 | 1100 | 31 | 0.99 | 51.5 | 20 | 35.3 |
291 | 150 | 377 | 2.92 | 1100 | 33 | 1.07 | 51.5 | 20 | 32.8 |
292 | 150 | 376 | 2.93 | 1100 | 33 | 1.35 | 51.5 | 20 | 39.6 |
293 | 150 | 377 | 2.92 | 1100 | 31 | 1.42 | 51.5 | 20 | 35.8 |
294 | 150 | 376 | 2.93 | 1100 | 33 | 1.8 | 51.5 | 20 | 39.2 |
295 | 150 | 368 | 2.99 | 1100 | 31 | 1.02 | 51.5 | 20 | 35.8 |
296 | 150 | 367 | 3 | 1100 | 33 | 1.85 | 51.5 | 20 | 48.8 |
297 | 100 | 566 | 3.53 | 2000 | 34 | 8.52 | 59 | 20 | 131.8 |
298 | 100 | 566 | 3.53 | 2000 | 80 | 11.36 | 59 | 20 | 189.8 |
299 | 100 | 572 | 3.5 | 2000 | 43 | 8.43 | 59 | 20 | 141.8 |
300 | 100 | 561 | 3.57 | 2000 | 75 | 11.46 | 62.6 | 20 | 210.8 |
301 | 100 | 572 | 3.5 | 2000 | 37 | 8.43 | 62.6 | 20 | 127.8 |
302 | 110 | 195 | 2.5 | 488 | 35 | 0.66 | 124 | 20 | 19.7 |
303 | 200 | 236.2 | 3.05 | 720 | 35 | 2.1 | 62.6 | 20 | 63.9 |
Rights and permissions
Springer Nature or its licensor (e.g. a society or other partner) holds exclusive rights to this article under a publishing agreement with the author(s) or other rightsholder(s); author self-archiving of the accepted manuscript version of this article is solely governed by the terms of such publishing agreement and applicable law.
About this article
Cite this article
Nguyen, TH., Nguyen, XB., Nguyen, VH. et al. Shear strength prediction of concrete beams reinforced with FRP bars using novel hybrid BR-ANN model. Asian J Civ Eng 25, 1753–1771 (2024). https://doi.org/10.1007/s42107-023-00876-y
Received:
Accepted:
Published:
Issue Date:
DOI: https://doi.org/10.1007/s42107-023-00876-y