Abstract
Traditionally, the homogeneity of available units of the same finished good (FG\(_i\)) to be promised to customers has been assumed. However, contexts with lack of homogeneity in the product (LHP) are characterised by units of the same FG\(_i\), which differ in some characteristics that are relevant for customers and give rise to different subtypes. For instance, in the ceramic industry, tiles are classified based on quality, tone and gage, because of functional and aesthetical reasons related to their joint installation. LHP imposes new constraints in the order promising process because customers need homogeneous units. However, the final amount of the homogeneous units in planned lots is uncertain when promising orders, because they will only be known once produced and classified. In this sense, we introduce homogeneity constraints including fuzzy sets; specifically, we address the interaction among fuzzy homogeneity coefficients that represent the fraction of each homogeneous sublot. Therefore, modelling uncertainty in interdependent technological coefficients in a dynamic context is one of the main novelties of our proposal. Thus, in this paper, in order to reliably meet the homogeneity required by customers, a fuzzy model is proposed to support the promising process in LHP contexts after taking into account uncertainty in planned homogeneous sublots. The fuzzy model is translated into an alpha-parametric equivalent crisp model. Here, it is important to highlight another important novelty of the paper related to the proposed methodology to analyse the suitability of the minimum degree of possibility (the \(\alpha\)-cut), by an adapted TOPSIS-based fuzzy procedure. Finally, the experimental design, which is inspired in the ceramic sector, proves both the validity of the model and a better performance of the fuzzy model compared to the deterministic one, in several executions with forecasts of the real size of homogeneous sublots.


Similar content being viewed by others
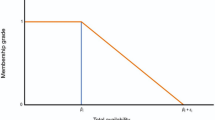
References
Ahumada, O., Villalobos, J.R.: Operational model for planning the harvest and distribution of perishable agricultural products. Int. J. Prod. Econ. 133.2, 677–687 (2011). doi:10.1016/j.ijpe.2011.05.015
Davoli, G. et al.: A stochastic simulation approach for production scheduling and investment planning in the tile industry. Int. J. Eng. Sci. Technol. 2(9) (2010). doi:10.4314/ijest.v2i9.64006.
Grillo, H., Alemany, M.M.E., Ortiz, A.: A review of mathematical models for supporting the order promising process under Lack of Homogeneity in Product and other sources of uncertainty. Comput. Ind. Eng. 91, 239–261 (2016). doi:10.1016/j.cie.2015.11.013
Alemany, M.M.E., et al.: Order promising process for extended collaborative selling chain. Prod. Plann. Control 19.2, 105–131 (2008). doi:10.1080/09537280801896011
Alemany, M.M.E., et al.: A model driven decision support system for reallocation of supply to orders under uncertainty in ceramic companies. Technol. Econ. Dev. Econ. 21.4, 596–625 (2015). doi:10.3846/20294913.2015.1055613
Alarcón, F., Alemany, M.M.E., Ortiz, A.: Conceptual framework for the characterization of the order promising process in a collaborative selling network context. Int. J. Prod. Econ. 120.1, 100–114 (2009). doi:10.1016/j.ijpe.2008.07.031
Bui, T., Sebastian, H.-J.: IEEE. Integration of multi-criteria decision analysis and negotiation in order promising’. In: 43rd Hawaii International Conference on Systems Sciences vol 1–5. Proceedings of the Annual Hawaii International Conference on System Sciences. pp. 1115–1124 (2010). doi:10.1109/HICSS.2010.237
Ball, M.O., Chen, C.-Y., Zhao, Z.-Y.: In: Simchi-Levi, D., Wu, S.D., Shen, Z.-J. (eds.) Handbook of Quantitative Supply Chain Analysis: Modeling in the E-Business Era”. Chap. Available to Promise, pp. 447–483. Springer, Boston (2004). doi:10.1007/978-1-4020-7953-5_11
Alemany, M.M.E., et al.: Available-To-Promise modeling for multi-plant manufacturing characterized by lack of homogeneity in the product: An illustration of a ceramic case. Appl. Math. Model. 37.5, 3380–3398 (2013). doi:10.1016/j.apm.2012.07.022
Jiménez, M., et al.: Linear programming with fuzzy parameters: an interactive method resolution. Eur. J. Oper. Res. 177.3, 1599–1609 (2007). doi:10.1016/j.ejor.2005.10.002
Peidro, D., et al.: A fuzzy linear programming based approach for tactical supply chain planning in an uncertainty environment. Eur. J. Oper. Res. 205.1, 65–80 (2010). doi:10.1016/j.ejor.2009.11.031
Yong, D.: Plant location selection based on fuzzy TOPSIS. Int. J. Adv. Manuf. Technol. 28.7–8, 839–844 (2006). doi:10.1007/s00170-004-2436-5
Chen, C.-T.: A fuzzy approach to select the location of the distribution center. In: Fuzzy Sets and Systems 118.1, pp. 65–73 (2001)
Chen, C.-T.: Extensions of the TOPSIS for group decision-making under fuzzy environment. In: Fuzzy Sets and Systems 114.1, pp. 1–9 (2000).
Wang, Y.-M., Elhag, T.M.: Fuzzy TOPSIS method based on alpha level sets with an application to bridge risk assessment. In: Expert Systems with Applications 31.2, pp. 309–319 (2006)
Wang, T.-C., Chang, T.-H.: Application of TOPSIS in evaluating initial training aircraft under a fuzzy environment. In: Expert Systems with Applications 33.4, pp. 870–880 (2007)
Gupta, A., Maranas, C.D.: Managing demand uncertainty in supply chain planning. In: 2nd Pan American Workshop in Process Systems Engineering 27.8–9, pp. 1219–1227 (Sept. 2003). doi:10.1016/S0098-1354(03)00048-6
Lababidi, H.M.S., et al.: Optimizing the supply chain of a petrochemical company under uncertain operating and economic conditions. Ind. Eng. Chem. Res. 43.1, 63–73 (2004). doi:10.1021/ie030555d
Santoso, T., et al.: A stochastic programming approach for supply chain network design under uncertainty. Eur. J. Oper. Res. 167.1, 96–115 (2005). doi:10.1016/j.ejor.2004.01.046
Sodhi, M.S.: Managing demand risk in tactical supply chain planning for a global consumer electronics company. Prod. Oper. Manag. 14.1, 69–79 (2009). doi:10.1111/j.1937-5956.2005.tb00010.x
Mula, J., Peidro, D., Poler, R.: The effectiveness of a fuzzy mathematical programming approach for supply chain production planning with fuzzy demand. Integr. Global Supply Chain 128.1, 136–143 (2010). doi:10.1016/j.ijpe.2010.06.007
Wang, J., Shu, Y.-F.: Fuzzy decision modeling for supply chain management. Fuzzy Sets Syst. 150.1, 107–127 (2005). doi:10.1016/j.fss.2004.07.005
Bellman, R.E., Zadeh, L.A.: Decision-making in a fuzzy environment. In: Management Science 17.4 (Dec. 1970). doi:10.1287/mnsc.17.4.B141
Dubois, D., Prade, H.: Possibility Theory: An Approach to Computerized Processing of Uncertainty. Springer Science & Business Media, New York (2012)
Dubois, D., Fargier, H., Fortemps, P.: Fuzzy scheduling: modelling flexible constraints vs. coping with incomplete knowledge. Fuzzy Sets Sched. Plann. 147.2, 231–252 (2003). doi:10.1016/S0377-2217(02)00558-1
Alemany, M.M.E., et al.: A fuzzy model for shortage planning under uncertainty due to lack of homogeneity in planned production lots. Appl. Math. Model. 39.15, 4463–4481 (2015). doi:10.1016/j.apm.2014.12.057
Gen, M., Tsujimura, Y., Ida, K.: Method for solving multiobjective aggregate production planning problem with fuzzy parameters. Comput. Ind. Eng. 23.1–4, 117–120 (1992). doi:10.1016/0360-8352(92)90077-W
Peidro, D., Vasant, P.: Transportation planning with modified S-curve membership functions using an interactive fuzzy multi-objective approach. Appl. Soft Comput. 11.2, 2656–2663 (2011). doi:10.1016/j.asoc.2010.10.014
Cadenas, J., Verdegay, J.: Using fuzzy numbers in linear programming. In: IEEE Transactions on Systems, Man and Cybernetics, Part B (Cybernetics) 27.6, pp. 1016–1022 (Dec. 1997). doi:10.1109/3477.650062
Peidro, D., et al.: Fuzzy optimization for supply chain planning under supply, demand and process uncertainties. Fuzzy Sets Syst. 160.18, 2640–2657 (2009). doi:10.1016/j.fss.2009.02.021
Chu, T.-C.: Facility location selection using fuzzy TOPSIS under group decisions. Int. J Uncertain. Fuzziness Knowledgebased Syst. 10.06, 687–701 (2002). doi:10.1142/S0218488502001739
Chamodrakas, I., Alexopoulou, N., Martakos, D.: Customer evaluation for order acceptance using a novel class of fuzzy methods based on TOPSIS. Exp. Syst. Appl. 36.4, 7409–7415 (2009). doi:10.1016/j.eswa.2008.09.050
Nakhaeinejad, M., Nahavandi, N.: An interactive algorithm for multiobjective flow shop scheduling with fuzzy processing time through resolution method and TOPSIS. Int. J. Adv. Manuf. Technol. 66.5–8, 1047–1064 (2013). doi:10.1007/s00170-012-4388-5
Shekarian, E., et al.: A fuzzy reverse logistics inventory system integrating economic order/production quantity models. Int. J. Fuzzy Syst. 18.6, 1141–1161 (2016). doi:10.1007/s40815-015-0129-x
Büyüközkan, G., Parlak, I.B., Tolga, A.C.: Evaluation of knowledge management tools by using an interval type-2 fuzzy TOPSIS method. Int. J. Comput. Intell. Syst. 9.5, 812–826 (2016)
Saradhi, B. Pardha., Shankar, N. R., Suryanarayana, C.: Novel distance measure in fuzzy TOPSIS for supply chain strategy based supplier selection. Math. Probl. Eng. 2016 (2016)
Senvar, O., Turanoglu, E., Kahraman, C.: Usage of metaheuristics in engineering: a literature review. In: Meta–Heuristics Optimization Algorithms in Engineering, Business, Economics, and Finance, pp. 484–528 (2013). doi:10.4018/978-1-4666-2086-5.ch016
Grillo, H., et al.: Application of particle swarm optimisation with backward calculation to solve a fuzzy multi-objective supply chain master planning model. Int. J. Bio-Inspired Comput. 7.3, 157–169 (2015). doi:10.1504/IJBIC.2015.069557
Rajavel, R., Thangarathanam, M.: Adaptive probabilistic behavioural learning system for the effective behavioural decision in cloud trading negotiation market. Futur. Gener. Comput. Syst. 58, 29–41 (2016)
Acknowledgements
This research is partly supported by: The Ministry of Science, Technology and Telecommunications of the of Costa Rica Government (MICITT), through the Programme of Innovation and Human Capital for Competitiveness (PINN)(Contract No. PED-019-2015-1); and the Spanish Ministry of Economy and Competitiveness Projects “Methods and models for operations planning and order management in supply chains characterised by uncertainty in production due to the lack of product uniformity” (PLANGES-FHP) (Ref. DPI2011-23597) and “Operations design and Management of Global Supply Chains” (GLOBOP) (Ref. DPI2012-38061-C02-01).
Author information
Authors and Affiliations
Corresponding author
Rights and permissions
About this article
Cite this article
Grillo, H., Alemany, M.M.E., Ortiz, A. et al. A Fuzzy Order Promising Model With Non-Uniform Finished Goods. Int. J. Fuzzy Syst. 20, 187–208 (2018). https://doi.org/10.1007/s40815-017-0317-y
Received:
Revised:
Accepted:
Published:
Issue Date:
DOI: https://doi.org/10.1007/s40815-017-0317-y