Abstract
Aiming at the lack of functional versatility in current engineering attachments, an innovative clamp–shear–grab integrated attachment (CSGI attachment), which combines clamping, shearing, and grabbing functions, is proposed based on the author’s US patent. The variable frame four-bar mechanism and separate frame design ideas are adopted in mechanism design, and the attachment’s motion scheme and virtual prototype are presented. In optimization, the optimization objectives of the CSGI attachment are chosen as the gripper opening size, the transmission angle of the clamp–shear mechanism, and the weight, so a multi-objective optimization model with mechanism dimension and topological structure is established. Next, an improved nondominated sorting genetic algorithm (WINSGA-II) is proposed by improving the crossover and search strategies, while its effectiveness is tested using standard indicators and data sets. Testing results show that the WINSGA-II algorithm has better convergence, population diversity, and uniformity than the nondominated sorting genetic algorithm (NSGA-II). Subsequently, the WINSGA-II algorithm is used to obtain the optimal results for the CSGI attachment. Finally, the engineering prototype is made and tested with clamping, shearing, and grabbing. The results show that the CSGI attachment can achieve the intended function. Regarding optimization, the gripper opening size of the CSGI attachment is expanded by 24.2%, and the weight is reduced by 10.1%. The HV and Spacing indicators of the WINSGA-II algorithm are 2.7% higher and 29.6% lower than those of the NSGA-II algorithm for this problem.













Similar content being viewed by others
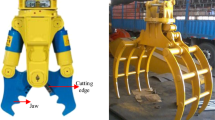
Abbreviations
- \(L_{iqb}\) :
-
The length of the link \(i\) in the state of the clamp–shear mode jaw is closed
- \(L_{iqk}\) :
-
The length of the link \(i\) in the state of the clamp–shear mode jaw is fully open
- \(L_{ibb}\) :
-
The length of the link \(i\) in the state of the grabbing mode gripper is closed
- \(L_{ibk}\) :
-
The length of the link \(i\) in the state of the grabbing mode gripper is fully open
- \(\theta_{iqb}\) :
-
The angle of angle \(i\) in the state of the clamp–shear mode jaw is closed
- \(\theta_{iqk}\) :
-
The angle of angle \(i\) in the state of the clamp–shear mode jaw is fully open
- \(\theta_{ibb}\) :
-
The angle of angle \(i\) in the state of the grabbing mode gripper is closed
- \(\theta_{ibk}\) :
-
The angle of angle \(i\) in the state of the grabbing mode gripper is fully open
- \(L_{iq}\) :
-
The length of the link \(i\) in two states of the clamp–shear mode
- \(L_{ib}\) :
-
The length of the link \(i\) in two states of the grabbing mode
- \(\theta_{iq}\) :
-
The angle of angle \(i\) in two states of the clamp–shear mode
- \(\theta_{ib}\) :
-
The angle of angle \(i\) in two states of the grabbing mode
- \(L_{i}\) :
-
The length of the link \(i\) in two states of in two modes
- \(L_{6b}\) :
-
The length of link 6 when the power hydraulic cylinder protrudes to the limited position
- \(L_{6k}\) :
-
The length of link 6 when the power hydraulic cylinder retracts to the limited position
- \(L_{wj}\) :
-
The length between point \(w\) and point \(j\)
- \(X_{j}\) :
-
The horizontal coordinate of \(j\)
- \(\theta_{{{\text{BG}}}}\) :
-
The angle BCG
- \(F_{2}\) :
-
The thrust of the power hydraulic cylinder
- \(F_{c}\) :
-
The shear force
- \(L_{c}\) :
-
The length of cutting-edge
- \(L_{51}\) :
-
The length in the horizontal direction between point A and point B
- \(L_{52}\) :
-
The length in the vertical direction between points A and B
- \(m_{\min }\) :
-
The minimum mass of each topology-optimized component after topological structure optimization
- \(M\) :
-
Original weight of each the topology-optimized component
- \(f\left( x \right)_{g\min }\) :
-
The gth minimization objective function
- \(f\left( x \right)_{g\max }\) :
-
The gth maximization objective function
- \(\sigma_{\max }\) :
-
The maximum stress of each topology-optimized component after topological structure optimization
- \({\text{Nop}}\) :
-
Number of populations
- \({\text{Noi}}\) :
-
Number of iterations
- \({\text{Cp}}\) :
-
Crossover probability
- \({\text{Mp}}\) :
-
Mutation probability
- \({\text{It}}\) :
-
Initial temperature
- \({\text{Tt}}\) :
-
Termination temperature
- \({\text{Cf}}\) :
-
Cooling factor
- \({\text{Noili}}\) :
-
Number of inner loop iterations
- \({\text{Cpf}}\) :
-
Crossover probability factor
- \({\text{Nofp}}\) :
-
Number of reference points
References
R Kapica D Vrublova M Vrubel 2018 The system of tracking the position of the bucket excavator’s wheel for prevention of risk situations Acta Geodyn Geomater 15 3 277 287 https://doi.org/10.13168/Agg.2018.0020
M Iphar 2012 ANN and ANFIS performance prediction models for hydraulic impact hammers Tunn Undergr Space Technol 27 1 23 29 https://doi.org/10.1016/j.tust.2011.06.004
S Han 2022 Snake robot gripper module for search and rescue in narrow spaces IEEE Robot Autom Lett 7 2 1667 1673 https://doi.org/10.1109/Lra.2022.3140812
Shan Y et al (2021) Design and development of multi-arm cooperative rescue robot actuator based on variant scissor mechanism. In: Intelligent robotics and applications, Icira 2021, Pt Iv. 13016, p 215–225. https://doi.org/10.1007/978-3-030-89092-6_20
Soloviev I, Sicoe A (2014) New persistent back-end for the ATLAS online information service. In: 2014 19th IEEE-Npss real time conference (Rt)
F Pastor 2022 Autonomous wristband placement in a moving hand for victims in search and rescue scenarios with a mobile manipulator IEEE Robot Autom Lett 7 4 11871 11878 https://doi.org/10.1109/lra.2022.3208349
K Bo 2023 Optimum design of large-diameter reverse circulation drill bit for drilling rescue wells using orthogonal experimental method and CFD simulation Energies https://doi.org/10.3390/en16093913
CR Wang J Zhao ED Xia 2019 Design and optimization of a novel rescue end-effector Proc Inst Mech Eng Part C J Mech Eng Sci 233 3 1032 1044 https://doi.org/10.1177/0954406218761506
Zhao J et al. Multi-functional engineering attachment for clamping, cutting, and grasping with a single degree of freedom variable topology mechanism
GY Xu HF Ding ZM Feng 2019 Optimal design of hydraulic excavator shovel attachment based on multiobjective evolutionary algorithm IEEE-ASME Trans Mechatron 24 2 808 819 https://doi.org/10.1109/Tmech.2019.2903140
TA Sullivan JD Ven Van de 2014 Multi-objective, multi-domain genetic optimization of a hydraulic rescue spreader Mech Mach Theory 80 35 51 https://doi.org/10.1016/j.mechmachtheory.2014.04.019
W Yan 2019 Lightweight splint design for individualized treatment of distal radius fracture J Med Syst https://doi.org/10.1007/s10916-019-1404-4
XH Wang 2019 Optimal design of lightweight serial robots by integrating topology optimization and parametric system optimization Mech Mach Theory 132 48 65 https://doi.org/10.1016/j.mechmachtheory.2018.10.015
BJ Kim 2016 Topology optimization of industrial robots for system-level stiffness maximization by using part-level metamodels Struct Multidiscip Optim 54 4 1061 1071 https://doi.org/10.1007/s00158-016-1446-x
J Xue B Shen 2022 Dung beetle optimizer: a new meta-heuristic algorithm for global optimization J Supercomput 79 7 7305 7336 https://doi.org/10.1007/s11227-022-04959-6
Y Wang 2020 An improved ant colony optimization algorithm to the periodic vehicle routing problem with time window and service choice Swarm Evolut Comput https://doi.org/10.1016/j.swevo.2020.100675
LP Zhang L Gao XY Li 2013 A hybrid genetic algorithm and tabu search for a multi-objective dynamic job shop scheduling problem Int J Prod Res 51 12 3516 3531 https://doi.org/10.1080/00207543.2012.751509
RC Leou 2006 A new method for unit maintenance scheduling considering reliability and operation expense Int J Electr Power Energy Syst 28 7 471 481 https://doi.org/10.1016/j.ijepes.2006.02.009
K Deb 2002 A fast and elitist multiobjective genetic algorithm: NSGA-II IEEE Trans Evol Comput 6 2 182 197 https://doi.org/10.1109/4235.996017
CR Wang J Zhao ED Xia 2018 Multi-objective optimal design of a novel multi-function rescue attachment based on improved NSGA-II J Braz Soc Mech Sci Eng https://doi.org/10.1007/s40430-018-1263-9
W Deng 2022 An enhanced fast non-dominated solution sorting genetic algorithm for multi-objective problems Inf Sci 585 441 453 https://doi.org/10.1016/j.ins.2021.11.052
M Yuan 2021 Research on intelligent workshop resource scheduling method based on improved NSGA-II algorithm Robot Comput Integr Manuf https://doi.org/10.1016/j.rcim.2021.102141
B Wang 2019 A NSGA-II algorithm hybridizing local simulated-annealing operators for a Bi-criteria robust job-shop scheduling problem under scenarios IEEE Trans Fuzzy Syst 27 5 1075 1084 https://doi.org/10.1109/Tfuzz.2018.2879789
S Bandyopadhyay 2008 A simulated annealing-based multiobjective optimization algorithm: AMOSA IEEE Trans Evol Comput 12 3 269 283 https://doi.org/10.1109/Tevc.2007.900837
Deb K et al (2002) Scalable multi-objective optimization test problems. In: Cec’02: proceedings of the 2002 congress on evolutionary computation, vols 1 and 2, pp 825–830. https://doi.org/10.1109/cec.2002.1007032
Ishibuchi H, Masuda H, Nojima Y (2016) Sensitivity of performance evaluation results by inverted generational distance to reference points. In: 2016 IEEE congress on evolutionary computation (CEC), pp 1107–1114
K Li 2018 Evolutionary many-objective optimization: a comparative study of the state-of-the-art Ieee Access 6 26194 26214 https://doi.org/10.1109/Access.2018.2832181
Z Qingfu L Hui 2007 MOEA/D: a multiobjective evolutionary algorithm based on decomposition IEEE Trans Evol Comput 11 6 712 731 https://doi.org/10.1109/tevc.2007.892759
K Deb H Jain 2014 An evolutionary many-objective optimization algorithm using reference-point-based nondominated sorting approach, Part I: solving problems with box constraints IEEE Trans Evol Comput 18 4 577 601 https://doi.org/10.1109/tevc.2013.2281535
GB Sinclair JR Beisheim AA Kardak 2019 On the detection of stress singularities in finite element analysis J Appl Mech Trans ASME https://doi.org/10.1115/1.4041766
Funding
This work was supported by the National Key Research and Development Program of China(2021YFC3002003).
Author information
Authors and Affiliations
Corresponding author
Ethics declarations
Conflict of interest
The authors declare that they have no competing interests.
Consent for publication
The manuscript has not been published before and is not being considered for publication elsewhere. All authors have contributed to the creation of this manuscript for important intellectual content and approved the final manuscript.
Additional information
Technical Editor: Rogério Sales Gonçalves.
Publisher's Note
Springer Nature remains neutral with regard to jurisdictional claims in published maps and institutional affiliations.
Rights and permissions
Springer Nature or its licensor (e.g. a society or other partner) holds exclusive rights to this article under a publishing agreement with the author(s) or other rightsholder(s); author self-archiving of the accepted manuscript version of this article is solely governed by the terms of such publishing agreement and applicable law.
About this article
Cite this article
Zhao, J., Wang, X., Zhang, D. et al. Design and optimization of clamp–shear–grab integrated attachment. J Braz. Soc. Mech. Sci. Eng. 46, 296 (2024). https://doi.org/10.1007/s40430-024-04715-2
Received:
Accepted:
Published:
DOI: https://doi.org/10.1007/s40430-024-04715-2