Abstract
Facing the challenges of the European Water Framework Directive and competing demands requires a sound knowledge of the hydrological system. This is a major challenge in regions like Northeast Germany. The landscape has been massively reshaped during repeated advances and retreats of glaciation during the Pleistocene. This resulted in a complex setting of unconsolidated sediments with high textural heterogeneity and with layered aquifer systems, partly confined, but usually of unknown number and extent of single aquifers. The Institute of Landscape Hydrology aims both at a better understanding of hydrological processes and at providing a basis for sustainable water resources management in this region. That would require sound information about the respective regions of interest that are rarely available at sufficient degree of detail. Thus, there is urgent need for alternative approaches. For example, time series of groundwater head, lake water level and stream runoff do not only depend on (unknown) geological structures, but in turn can reveal information about major geological features. To that end, different approaches have been developed and successfully applied at different scales, based both on advanced time series analysis and dimension reduction approaches and on well-known and rather simple methods. This approach has been coined “forensic hydrology”: Like in a crime story, numerous pieces of evidence are combined in a systematic way to end up with a consistent conceptual model about the prevailing cause–effect relationships. An example is given for the Quillow catchment in Northeast Germany in a rather complex geological setting.
Similar content being viewed by others
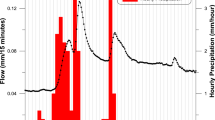
Introduction
Managing water resources in a changing world (Cassardo and Jones 2011), water security (IHP 2012), change in hydrology and society (Montanari et al. 2013) as well as the need for integrated water resources management (EU WFD 2000) are the challenges for hydrological research in the next decades. The water cycle at local, regional and global scales is increasingly under pressure. Climate and land use changes and other global drivers, e.g., population growth and rapid urbanization, will put pressure on water resources with a tremendous impact on the natural environment (IHP 2012). To understand the functioning of the hydrological cycle under the ongoing changing conditions, hydrology has made enormous efforts with regard to small relatively homogeneous systems over a relative short timescale. In the next decade, hydrological research has to focus on the understanding of complex systems over much longer timescales (Wagener et al. 2010; Milly et al. 2008) considering nonlinearities, heterogeneities and highly dynamic processes.
Hydrology in complex settings
Hydrology builds on a wealth of process studies that revealed numerous causal relationships and often rather complex, nonlinear interactions between different processes or influencing factors. Consequently, water resources management has to face the challenge to differentiate between different effects that occur in parallel. Different effects might add to each other in some cases and might compensate each other in others. Often considerable lag times have to be accounted for as well. On the other hand, climate change as well as massive anthropogenic effects, both intended and unintended, and partly being on the way for centuries or even millennia in most parts of the world, needs to be accounted for.
Hydrologists have developed a whole zoo of powerful models. These models can handle many of the challenges even for complex systems. In practice, however, there is a substantial misbalance between the potential of spatially distributed models and the available data to condition and to constrain them. Consequently, models often are massively over-parameterized due to a lack of data and of knowledge of the respective local conditions, leading to substantial uncertainty (Beven 2001). In addition, models combine findings from preceding studies about single causal relationships. However, feedback between different processes is often hard to quantify in empirical studies in complex settings. Consequently, feedbacks in models are often necessarily implemented on experts’ judgement rather than on sound data.
Landscape hydrology approaches
The term “landscape hydrology” has been introduced for a scientific approach to meet the challenges given above. In contrast to, e.g., “catchment hydrology”, the term “landscape hydrology” usually does not only imply a larger spatial scale, but very heterogeneous settings as well (Hyndman et al. 2007), including very different soil types (Terribile et al. 2011), the nexus between groundwater and surface water systems (Hyndman et al. 2007; McLaughlin et al. 2014; Yuan et al. 2015), or large-scale feedbacks like evapotranspiration as a source for rainfall in other regions (Woodward et al. 2014). Thus, landscapes are primarily considered as highly interconnected systems comprising numerous abiotic, biotic and anthropogenic elements. Due to the large number of feedback links between landscape elements, landscapes are far from being random ensembles of elements. Instead, they are highly constrained, that is, the number of effective degrees of freedom is much smaller than one could expect with regard to the number of landscape elements (Lischeid et al. 2015).
Landscape hydrology aims at systematically exploring these constraints and at making efficient use of them. Following that approach has in many cases revealed that although the structure of hydrological systems might be complex, hydrological functioning often is surprisingly simple or low dimensional. The term “functioning” is used for time series of hydrological variables like discharge, groundwater head or soil water content. On the one hand, the effects of small-scale heterogeneities often level off at larger scales (Hohenbrink and Lischeid 2015). On the other hand, among a variety of processes that affect hydrological functioning at the landscape scale usually only a few prevail and need to be considered (Seyfried and Wilcox 1995; Blöschl 2001; Sivakumar 2004).
Taking that seriously implies that hydrological functioning should reflect the effects of the prevailing processes and could in turn be used to infer major features that are relevant for hydrological functioning. For example, time series of groundwater head, lake water level and stream runoff do not only depend on geological structures, but could be used to reveal information about major geological features. This information can then be used, e.g., to reduce the uncertainty of a hydrological model.
This is the basis of the forensic hydrology approach. The term “forensic” came up in the environmental sciences in the 1970s in the context of identifying causes of environmental pollution (Hurst 2007). In the case of hydrology, it usually requires an identification of flowpaths and flow velocities, or residence time, respectively (Hurst 2007). In addition, the term “forensic hydrology” has been used for analysis of major floods and droughts (Loáiciga 2001; Borga et al. 2014; Ramirez and Herrera 2016). It is now used in a wider sense for systematic analysis of cause–effect relationships in complex settings in hydrology, combining a variety of different methods of direct and indirect inference (e.g. Kappel 2014) in a systematic way. Thus, hydrologists act as “geodetectives” (Hurst 2007), similar like detectives in a crime story, although not restricted to court cases. In this study, both well-known and rather simple as well as innovative sophisticated data analysis approaches were used and the results merged in a systematic way for developing a consistent conceptual model of the Quillow study in a very complex geological setting. This is a necessary prerequisite for assessing pathways of subsurface transport of contaminants and nutrients from arable fields and the respective residence time. Key questions are: Are the small lakes that are abundant in that region hydraulically connected to a regional aquifer? Are there major aquitards in the catchment, and how far do they extend? Which area does a given stream drain?
Study site and data
Study site
The study area comprises the Quillow catchment in Northeast Germany, about 100 km north of Berlin, and its immediate surroundings. The landscape of Northeast Germany has been massively reshaped during repeated advances and retreats of glaciation during the Pleistocene. This resulted in a complex setting of unconsolidated sediments with high textural heterogeneity and with layered aquifer systems, partly confined, but usually of unknown number and extent of single aquifers. Topography and the related stream network are still far from maturity. Besides some large lakes, numerous small lakes and wetlands developed in drainless depressions. These small lakes (<1 ha area each) are called kettle holes (Kalettka and Rudat 2006). In the following the terms, “lakes” and “kettle holes” will be used synonymously. They typically undergo frequent wet–dry cycles, and therefore, the area of water surface is highly variable. The natural drainage network was massively extended by man to enable crop production in formerly waterlogged areas.
The Quillow catchment has an area of about 160 km2 upstream of the confluence with the Strom stream which drains the adjacent catchment in the south (Fig. 1). Area had been determined based on topography and groundwater head data of the uppermost main aquifer and might differ from that of the true groundwater catchment of the hydrogeological setting which is not known with sufficient detail. In addition, groundwater flow direction might differ in the overlying shallow aquifer or in deeper aquifers.
The topography of this till-dominated region is characterized by gently rolling hills, a so-called hummocky landscape (Sommer et al. 2008). Topographic altitude decreases from 110 m a.s.l. in the western part of the catchment, which is dominated by terminal moraines, to 20 m a.s.l. in the east, that is, the glacial valley of the receiving Ucker River. Groundwater flow direction in the uppermost main aquifer follows the topographical gradient, approximately parallel to the main stream (Merz and Steidl 2015).
The unconsolidated sediments form a series of layered Pleistocene and Tertiary aquifers of about 100–150 m thickness with a 50-m-thick Oligocene marine clay layer as a lower confining bed. The complete series consists of permeable marine dominated sediments of upper Oligocene and the lower Miocene with a complex interplay between glacial deposits of the Pleistocene with a vertical extent of more than 100 m. These deposits, dominated by sediments from the main glaciations (Elster, Saalian and Weichselian), can be divided into different aquifers separated by till layers. Interlayering of clayey till layers results in a system of layered aquifers of unknown number, lateral extent and of hydraulic links in between. This is a major challenge for groundwater studies and models.
Soils are mainly loamy and sandy. Wetlands in the riparian zone and in small depressions comprise about 5% of the catchment area. Partly due to the heterogeneity of the Pleistocenic deposits, partly due to erosion processes, soils exhibit substantial heterogeneity even at small scales (Sommer et al. 2008; Gerke et al. 2016). Land use is dominated by agriculture which covers about 74% of the catchment area where arable fields prevail. Grassland is found mainly close to the Quillow stream and in the eastern lowland parts of the catchment. In addition, small forest patches are located mainly in the western and southwestern part of the catchment. There are no major settlements in the catchment except for some small villages and single houses. Both settlement density and intensity of agricultural production have been rather stable over many decades. Tile drains are very common in the riparian zone and in small depressions. In contrast, there is no evidence that surface runoff might play a major role for runoff generation.
Meteorological data are available from the Dedelow Research Station in the northern part of the catchment. Mean values for the 1992–2013 periods were 8.6 °C for air temperature, and for precipitation 563.8 mm per year, corrected for wind and evaporation error according to Richter (1995). Potential evapotranspiration determined according to Allen et al. (1998) was 635.2 mm per year, yielding a negative value of the climatic water balance of about 71.4 mm per year. Long-term mean discharge 1972–1990 of the Quillow stream after confluence with the Strom stream from the adjacent catchment amounted 143.6 mm per year (B. Stein and B. Hölzel, pers. comm. 1994). However, there are substantial uncertainties with respect to identifying the catchment boundaries as stated above, as well as the connectivity between the hydrological subsystems.
Data
Digital elevation model
For the Quillow catchment, except for the outermost western part, digital elevation data at high spatial resolution (1 m) were provided by Landesvermessung und Geobasisinformation Brandenburg (State Survey and Geospatial Basic Information Brandenburg; LGB) acting on behalf of the Landesamt für Umwelt, Gesundheit und Verbraucherschutz Brandenburg (State Office of Environment, Health and Consumer Protection Brandenburg; LUGV). The data are based on an airborne laser survey in spring 2011. They were used with kind permission of LGB Brandenburg, ©Geobasis-DE/LGB 2012.
For open water bodies, the digital elevation data approximate the water level during the time of the survey. Due to aberration of the laser beam, the error of water level determination might be in the order of 0.1 m or even more, especially for large water bodies. Water level in the streams was determined at 100-m intervals along the streams, resulting in 1273 elevation points.
In addition, water level in 1176 small lakes (kettle holes) was determined using the same data set. They were identified first manually on the basis of different digital maps including aerial views, biotope types and topographical maps, scale 1:10,000. Then, the elevation of the water level was determined at these points based on the digital elevation model. We did not make full use of the high spatial resolution of elevation data for streams in order to roughly balance the number of stream and lake water level data points and to achieve a more homogeneous coverage of the area.
Soil hydrological data
Soil water content had been measured automatically at 1-h intervals using TDR probes or FDR probes (site Kraatz) in 20–300 cm depth at seven different locations within the catchment (Table 1; Schindler et al. 2008). In addition, the same measurements were taken in a series of lysimeters located at the Dedelow research station (Fig. 1). Lysimeters were filled with homogenized substrates excavated at different sites in the catchment. Surface area is 1 m2, depth 2 m. Lysimeters were vegetated with different crops. Table 1 gives an overview over site properties and measurement depths.
Groundwater data
For this study, groundwater head data from two different data sets were used. Details are given in Table 2. The authors’ group has been operating four groundwater wells (198, 201, 203, 204) that are located in the central part of the catchment (Fig. 1). They have been equipped with automatic ventilated pressure transducers, recording groundwater head at daily intervals. For more details, the reader is referred to Merz and Steidl (2015).
In addition, groundwater head data were provided by the State Office of Environment, Health and Consumer Protection Brandenburg (LUGV). These wells were located within or close to the Quillow catchment (Fig. 1). Groundwater head had been determined by pressure transducers (daily intervals) or manually (once or four times per month).
Stream discharge
Water level of the Quillow stream has been measured at hourly intervals close to the Dedelow research station (Fig. 1) by an automatic ventilated pressure transducer. That site is located underneath a road bridge where the stream exhibits a clearly defined cross section. Thus, water level data could be converted to discharge based on a rating curve that has been regularly calibrated by current meter measurements.
In addition, differential stream gauging was performed at different measurement points in the main stream of the catchment during baseflow conditions. A current meter was used to determine flow velocity at different positions in cross sections of the stream. The measured values of flow velocity were integrated over the respective cross-sectional area. As this approach was meant to yield a quick first insight into spatial patterns along the stream, no sound error analysis was performed. Based on experience with that approach in preceding studies, an uncertainty range of about 10 to 20% is assumed.
Methods
The methods used in this study are only briefly described here. Please refer to the cited papers for methodological details. All analyses and graphs presented in this study were performed using the R software (R Core Team 2014).
Principal component analysis of hydrological time series
Hydrological time series usually exhibit substantial spatial heterogeneity. That might be due to the spatial heterogeneity of rainfall, interception, evapotranspiration, soil and aquifer properties as well as anthropogenic impacts. Principal component analysis (PCA) of hydrological time series aims at exploiting the observed heterogeneity as a source of information for quantitative assessments of the respective effects. In other disciplines, terms like Empirical Orthogonal Functions or Karhunen–Loève transformation are used instead of PCA.
In mathematical terms, a principal component analysis performs an eigenvalue decomposition of a covariance matrix of a set of variables into a set of independent principal components. When time series are subjected to a PCA, the resulting principal components constitute time series as well. Any time series of the input data set can then be represented as a linear combination of the principal components without any loss of information. The respective weighting factors reflect the bivariate correlation (called “loadings”) between observed time series and principal components as well as the eigenvalues of the principal components. The sum of the eigenvalues of selected principal components over the sum of the eigenvalues of all components is equal to the fraction of variance of the total data set explained by the respective components. Principal components usually are sorted by decreasing eigenvalues, that is, the most important components are listed first.
Principal component analysis has been used in hydrology for many decades but has only rarely been applied to time series, in contrast to other disciplines like climatology. However, it has proven to be a powerful tool to identify and to quantify the impact of river water stage on riparian groundwater head (Lehr et al. 2015), of groundwater production wells on adjacent lakes (Böttcher et al. 2014), of different crops and tillage practices on soil water content (Hohenbrink et al. 2016), or of climatic gradients on stream discharge (Thomas et al. 2012). Application of PCA on a data set comprising soil matrix potential, groundwater head and stream discharge nicely illustrated the hydrological continuum within a catchment (Lischeid et al. 2017).
When PCA is applied to time series, the first component usually is similar to a time series consisting of the spatial averages of normalized observed values per time step. Subsequent components reflect then different effects that cause deviation from that mean behaviour each (Hohenbrink et al. 2016). One of these processes that is prominent in groundwater head and soil hydrological data sets is increasing transformation of the input signal (e.g. rainfall or snowmelt) with depth in the vadose zone, that is, increasing attenuation of the amplitudes and deceleration of the signal (Hohenbrink and Lischeid 2015; Lischeid et al. 2010). This phenomenon will be called “damping” in the following.
Principal component analysis of time series does not require equidistant time series. However, the data need to be synchronous, that is, measurement dates need to be identical for all observed variables. To ensure equal weighing of all observables, each time series was normalized to unit variance and zero mean prior the analysis. For more detailed information about that approach, the reader is referred to Lischeid et al. (2010), Hohenbrink and Lischeid (2015) and Hohenbrink et al. (2016).
Differential stream gauging
Net inflow of groundwater into a stream, or net loss of stream water due to seepage or discharge to the aquifer within a given stream reach can be assessed by the net difference of discharge at both ends of the respective stream reach. In addition, tracer tests can be used to assess gross inflow (Bencala et al. 2011; Bergstrom et al. 2016). It is recommended to perform these measurements during baseflow (Cey et al. 1998; Ruehl et al. 2006; Kalbus et al. 2006; Kikuchi et al. 2012) to minimize the effect of surface runoff or interflow feeding the stream. In addition, short-term temporal variability should be negligible as discharge measurements are usually taken consecutively.
Applicability of that approach often is limited by the limited precision of discharge measurements. On the other hand, it allows rapid assessment of groundwater—stream interaction at medium to large scale with little effort. Thus, it has been widely used in hydrology. However, there is no uniform nomenclature. The approach has been termed “longitudinal variation in discharge” (Zellweger et al. 1998), “measurements of stream discharge along the reach” (Cey et al. 1998), “seepage runs” (Ruehl et al. 2006), “incremental stream flow” (Kalbus et al. 2006), “differential flow gauging” (McCallum et al. 2012) or “differential discharge measurements” (Kikuchi et al. 2012). Here the term “differential stream gauging” will be used.
Comparison of groundwater head and surface water level
According to Darcy’s law, hydraulic gradients in the subsurface are determined by groundwater flow density and hydraulic conductivity of the respective substrate. Small-scale heterogeneities of the latter are likely to level out at larger scale, resulting in a fairly smooth groundwater head surface (e.g. Fleckenstein et al. 2006). Consequently, major deviations of groundwater head or lake water level at selected sites from the mean groundwater surface provide strong evidence for missing hydraulic links between the respective hydrological systems.
In a first step, comparing the shape and smoothness of the respective surfaces could provide substantial evidence for or against hydraulic connection between surface water systems and groundwater. As a quantitative measure of the smoothness variograms were used. Variograms describe the variance of spatial data as a function of distance between respective data points. Spatial variance is low for data sets with a smooth surface and for small distances, tending to increase with distance. In contrast, for abrasive surfaces spatial variance is close to the maximum already for very short distances.
Variogram values \(\upgamma\) as a function of distance \(h\) are calculated as mean squared distances between two points x i , x j
usually using a rather small number of distance classes with \({N\left(h\right)}\) data points each for pairwise comparison.
The data of the variogram of the observed values were fitted to an exponential model given by
where \(h\) denotes distance, c denotes the sill, and a denotes the range. For large data sets and large distances, the sill approaches the variance of the data. For the exponential model, the range is a measure of how rapidly spatial variance increases with distance.
As low-frequency patterns and trends would mask the patterns of spatial variance as a function of distance, spatial data were detrended prior the analysis by linear regression with the northing and easting coordinates.
The total number of data points of small lake water level was 1176, that is, some orders of magnitude smaller compared to the number of data points of the digital elevation model. Thus, 1000 data points of the latter were selected randomly in ten different realizations to be analysed via variogram and to be compared with that of the lake water level data.
Data of deep groundwater wells were too sparse to be interpolated. Here a different approach was used. For each of the wells, mean groundwater level was compared to linear interpolation between lake and stream water level points in up to 1 km distance. The difference between these respective two approaches was compared to the square root of the error variance of the linear interpolation, performed without the groundwater head data.
Results
Principal component analysis of hydrological time series
Topsoil data
The first data set that was subjected to a principal component analysis consisted of time series of discharge of the Quillow stream, of groundwater head at four wells (198, 201, 203, 204) and of 47 time series of soil water content, measured at different sites within the catchment and in six lysimeters at the Dedelow Research Station (Fig. 1; Table 1). Daily values were available from the catchment runoff, and noon values from groundwater wells and soil water content probes were used. In total, the data set covered the April 19, 2002–December 10, 2004, period although with some extended data gaps (221 days with missing data out of 967 days).
Out of the principal components, only the first six will be considered with eigenvalues exceeding 1 (from 26.9 down to 1.5). The first principal component explained 52% of the total data set, the second another 19%, and the third up to the sixth component another 20%.
Following the approach presented by Hohenbrink et al. (2016), loadings on the first six principal components were investigated with respect to clear differences between sites. Because of the low number of replicates (2–5 per sites), no significance test was applied. Instead, mean and ranges of loadings per sites were studied. For the first, second and sixth principal components, the ranges of loadings widely overlap between different sites (not shown). In contrast, some lysimeter sites clearly stand out with respect to components 3–5 (Fig. 2). Especially the two lysimeters filled with coarse sand (Lys30 and Lys31) exhibit much lower loadings on the fifth component compared to all other sites. In contrast, mean values for field sites did not differ much. This holds for comparison of forest (CH-K, Sk-B, Sk-M) and arable field sites as well. In general, within-site variability was much higher for field sites compared to the lysimeters that had been filled with homogenized soil material (Fig. 2).
The first two components covered 71% of the total variance of the data set. Comparably to other studies (Lischeid et al. 2010; Hohenbrink et al. 2016), the scores of the first component were very similar to a time series of spatial averages of normalized values of the measured data (not shown). Loadings on the first and second principal components of all time series are shown in Fig. 3. The closer the single symbols plot to the unit circle, the higher the fraction of variance of the respective time series that is explained by the first two components. Time series of soil water content measured at shallow depth tend to plot in the upper half of the graph, time series from probes at greater depth and all groundwater head data plot in the lower half, and stream discharge right in between. Similarly as has been shown by Lischeid et al. (2010) and Hohenbrink and Lischeid (2015), that sequence in clockwise direction reflects an increasing degree of transformation of the input signal with depth, that is, increasing damping of the signal imposed by infiltrating rainfall or snowmelt (not shown).
Groundwater data
For some of the groundwater wells, only monthly values of groundwater head were available but covering a longer time period (15.03.2002–15.02.2008) than the soil water content data. Thus, a separate principal component analysis was performed on these time series of ten observations wells. The first two components explained 93% of the variance. Correspondingly, the time series represented as single symbols plotted close to the unit circle, similarly as in Fig. 3 (not shown). As has been shown above, location of the time series represented by single symbols in that figure reflects the different degrees of damping of the respective time series. The degree of damping can be quantified by the angle between the x-axis and a line connecting single symbols and the origin of the coordinate system (Lischeid et al. 2010; Hohenbrink and Lischeid 2015) where negative values indicate weak, and positive values strong damping. Plotting the damping coefficient versus depth (Fig. 4) reveals two different groups. Five wells, coloured in light blue, plot close to a common regression line (r 2 = 0.957). This is a typical phenomenon often found: Damping usually clearly increases with depth for soil hydrological time series (Hohenbrink and Lischeid 2015; Lischeid et al. 2017), and correspondingly damping of time series of groundwater head increases with thickness of the overlying vadose zone in unconfined aquifers (Lischeid et al. 2010; Böttcher et al. 2014).
In contrast, the remaining five wells (marked in red) clearly plot above that regression line. As has been shown by Lischeid et al. (2010), this is a strong indication for a confined aquifer. These wells exhibit much more damped behaviour than one would expect with regard to the respective thickness of the vadose zone (Fig. 4). For example, damping at well 26486011 is much more pronounced than at well 26490030 although depth to groundwater is approximately the same. Well 26486011 is located very close to the main stream (cf. Fig. 1). Mean head in this well is even above soil surface and stream water level, thus exhibiting an upward head gradient towards the stream and indicating an artesian well. Damping of this well is in the same range as that for well 26480022 (Fig. 4) where groundwater head is roughly 6.70 m below surface. Groundwater that discharges at the artesian well must have been recharged elsewhere where the upper confining bed was missing or thinned out. In addition, it can be concluded that the thickness of the vadose zone in that recharge area must have been about 6.7 m as well.
Correspondingly, Fig. 4 strongly suggests confined conditions at the screening depth of wells 204, 198, 201 and 203 as well. The latter three wells exhibit roughly the same damping coefficient which would suggest mean depth to groundwater of about 13 m (vertical projection onto the regression line) in the respective zones of groundwater recharge.
Differential stream gauging
Whereas the upper reaches of the stream network often fell dry during the growing season, the lower reaches of the Quillow stream were perennial, indicating a sufficiently large groundwater store that gradually drains to the stream. Differential stream gauging along the stream during dry periods can then give some evidence for major subsurface structures like, e.g., thinning out of a confining bed. Figure 5 presents the results of the measurement campaigns and a map of the location of the measurement points. In addition, size of the respective subcatchments is indicated for comparison. If groundwater discharge to the stream would occur homogeneously along the stream, discharge should increase proportionally to the latter.
Differential stream gauging was performed in the main stream in May and September 2014, and in October 2015. Sampling started at the outlet of the Parmen Lake (0 km). The reaches of the stream downstream of the Parmen lake, that is, sampling points 15–21, were dry during the last two measurement campaigns (Fig. 5). Correspondingly, discharge increased only slightly in May 2014 along the uppermost 10 km of the stream in spite of a substantial increase of the size of the respective subcatchments down to measurement point 12. In contrast, there were two segments of stepwise increase in discharge in May 2014, that is, at about 10 and 15 km distance from the outflow of the Parmen lake, close to the artesian well 26486011 (cf. Fig. 1). The second steep increase in discharge at 15 km was found in autumn 2014 and 2015 as well.
Groundwater head and surface water level
Figure 6 presents the location and elevation of stream water level points, kettle holes and groundwater head in deep wells in the Quillow catchment and its immediate surroundings. The same colour coding is used throughout to facilitate comparison. In total, water level data of 1176 kettle holes and of 1273 stream points were used.
Water level in streams and kettle holes exhibits a fairly smooth pattern, roughly following the inclination of topography from west to east. Kettle hole water level tended to be slightly higher compared to that in the adjacent streams.
Groundwater head in the deep observation wells is roughly equal to that of adjacent kettle holes and streams in the eastern half of the catchment. In contrast, in the western half of the catchment, groundwater head in deep wells is substantially lower, indicating a second deeper aquifer that is hydraulically isolated from the uppermost shallow aquifer.
The mean difference between groundwater head and surface water level was studied in detail. A multivariate regression of water level in kettle holes and stream water level points at up to 1000 m distance from the respective well was performed, and the elevation difference between temporally averaged groundwater head and its vertical projection onto that plane was determined. Minimum number of surface water level points was 12, and maximum 82 for single wells. That analysis could be performed for 20 out of 34 wells. Results are given in Fig. 7.
Mean groundwater head is more than 10 m below surface water level in the western part of the catchment, tending to approach surface water level towards the eastern part of the catchment. East of well 204 that difference is close to zero. Five wells clearly stand out due to extraordinary low groundwater head compared to surface water level, marked by white bars in Fig. 7. They are all located close to the southern or northern catchment boundary, respectively (cf. Fig. 1). Only at well 26486011, groundwater head exceeds that of surface water level, indicating an artesian well. This well is located very close to the stream (cf. Fig 1).
The smoothness of the surface spanned by surface water level data in kettle holes and streams was compared to that of topography in a more systematic approach. The variograms of the ten different random selections of the digital elevation model data were very similar to each other (Fig. 8). On the other hand, they clearly differed from that of the kettle hole water level data. Sill values, that is, the variance for rather large distances, ranged between 80.9 and 113.3 m2 for topographical data, and were only 36.3 m2 for the kettle hole water level data. The range as a measure of the slope of increase of variance with distance varied between 2649 and 4442 m for the topographical data, whereas it was only 1713 m for kettle hole water level data. Consequently, the variogram of the latter approximately reached a plateau at about 7 km distance, whereas the variograms of digital elevation data continued to increase far beyond that distance.
Discussion: Resulting conceptual model and implications for modelling
According to the results of the principal component analysis, the time series of soil water content exhibited substantial spatial heterogeneity. Although in general the damping of the input signal tended to increase with depth, soil water content at one site in 185 cm depth behaved like that at 60 cm depth at another site (Fig. 3). Much of that heterogeneity can be ascribed to the enormous heterogeneity of unconsolidated sediments (Merz et al. 2009) and soils in this Pleistocenic landscape, ranging from coarse sandy soils to clayey loam soils. In addition, erosion processes resulted in substantial spatial heterogeneity at the range of a few 10 m as well (Sommer et al. 2008). Consequently, soil hydrological and chemical processes exhibit enormous spatial heterogeneity (Rieckh et al. 2012; Gerke et al. 2016) in this region.
However, except for some of the lysimeters, there was no evidence for systematic differences between different sites, even between arable field and forest sites (Fig. 2). This could have been masked by substantial within-site heterogeneity. Only two of the lysimeters that were filled with coarse sand clearly stood out, indicating that only extremes of soil texture needed to be taken into account. Applying the same approach to another soil hydrological data set, Hohenbrink et al. (2016) found clear differences between two crop rotation schemes. However, that difference accounted only for 3.6% of the total variance. It is very likely that a difference of that magnitude would not been detectable in the Quillow data set given the enormous spatial variability. However, these differences of soil hydrological dynamics must not be mismatched with differences of the total sum of deep seepage or groundwater recharge. In fact, Schindler et al. (2008) found clear differences of deep seepage rates between forest sites and arable fields as well as between different texture classes in a data set that comprised among others the Quillow data used for this study.
Thomas et al. (2012) used the same approach and found clear evidence for a climatic gradient reflected by respective components when analysing hydrographs from all over the Federal State of Brandenburg. Thus, although the annual sum of precipitation is known to decrease from west to east in the catchment (Sommer et al. 2008), the temporal patterns obviously are the same.
In addition, time series of groundwater head and of discharge plot right in between those of soil water content time series in Fig. 3, illustrating a hydrological continuity between vadose zone, aquifer and stream. According to Fig. 3, the hydrograph is substantially less damped compared to the latters. Damping of the hydrograph corresponds more to that of soil water content at approximately 1.5 m depth. It can be concluded that runoff generation to a large degree occurs at rather shallow soil depth. Here tile drains might play an important role.
Comparing water levels in kettle holes and streams, there was strong evidence of a common hydrological system (Fig. 6). In addition, the smoothness of the surface spanned by surface water level compared to that of topography supported that inference (Fig. 8). In contrast, groundwater head in wells screened at greater depth were substantially lower except for three wells that were located close to the stream in the eastern lowland part of this and in an adjacent catchment (Figs. 1, 7). On the other hand, deep groundwater wells close to the stream in the more upstream part of the catchment obviously are neither directly hydraulically linked to the stream nor to the kettle holes (Figs. 1, 7).
The line of demarcation of these two parts of the catchment is approximately identical with the upper end of the gaining reaches of the stream between stream measurement points 13 and 12 (Fig. 5). Combining these findings with those of the discrepancy between groundwater heads and surface water level in the upstream parts of the catchment suggests that upstream that demarcation line one or more aquitards separate the uppermost aquifer from a deeper one. That aquitard(s) obviously thin(s) out upstream the demarcation line, and about 2 km downstream an area of high kettle hole density that stretches from North to South, perpendicular to the main stream (Fig. 1). This is an area that is drained by some of the major tributaries of the Quillow stream (Fig. 1). That feature could indicate that the uppermost aquifer thins out here, e.g., due to intersection of topography with an approximately horizontal lower confining bed.
The line of intersection seems to be located close to well 26486011, the only artesian well in the catchment. About 2.5 km downstream of this well, close to stream measurement point 8 (Fig. 5), a fen exists close to the stream from which water discharges to the stream. Substantial efforts to drain that fen by trenching to separate it from the presumed interflow or shallow groundwater from the adjacent hillslope have not been successful. Fairly high electric conductivity of the discharging water from the fen points actually to deep groundwater that discharges here but presumably had recharged in more upstream parts of the catchment.
According to Fig. 4, the degree of damping found at the artesian well 26486011 points to recharge in an area rather far from this site where the thickness of the vadose zone is about 6–7 m. This points to a substantial lateral extent of the upper confining layer. A corresponding mismatch between the degree of damping of groundwater head time series and depth of pressure head below surface has been found at wells 198, 201, 203 and 204 as well. They are all located close to the stream, up to 5 km upstream the artesian well (Fig. 1). The degree of damping found at these wells points to recharge substantially further uphill where the thickness of the vadose zone is up to 13 m approximately (Fig. 4). High electric conductivity and absence of oxygen and nitrate in these wells (Merz and Steidl 2015) are indicative for long groundwater residence time, supporting this inference.
These findings can be summarized as follows. Hydrological processes in the topsoil exhibit substantial spatial heterogeneity, urging for a large number of replicates of soil hydrological data for model calibration. Besides, there is no evidence for clear spatial patterns within the catchment that need to be considered. In general, damping of the input signal increases with depth in the vadose zone and seems to exert first-order control on the dynamics of groundwater head and stream discharge.
Kettle holes and streams are part of a common uppermost shallow hydrological system that is separated from an underlying major aquifer in the western part of the catchment and close to the catchment boundary in the eastern part as well. However, the underlying confining layer must be leaky. Otherwise neither periodical drying-up of stream reaches in this part of the catchment nor recharge of the underlying deeper aquifer would be possible. In contrast, in the central western part of the catchment, there was strong evidence for an extended tight confining layer.
Conclusions
Landscape hydrology considers landscapes as systems being subject to numerous feedback links, that is, as highly constrained systems, and applies modern methods of system analysis to make efficient use of the available data. That approach opens a pathway to handle systems with very complex structure like that of the thick unconsolidated Pleistocenic sediments in North Central Europe. An example was given in this study.
Although we observed substantial small-scale spatial heterogeneity of soil moisture dynamics in the vadose zone, there was no evidence of systematic differences between different plots within the catchment, even not for different land use classes. In addition, measurements on field sites did not differ systematically from those in lysimeters filled with homogenized material, except for lysimeters filled with coarse sand. Discharge dynamics corresponded to that of soil moisture at 1.5 m depth, emphasizing the role of shallow flowpaths including tile drains for runoff generation. Small lakes (kettle holes) that are abundant in the catchment are generally hydraulically connected to the groundwater system. The main aquifer is unconfined in the eastern half of the catchment, but is confined and separated from an overlying shallow aquifer in the western part that is linked to lakes and streams. This information is essential for assessing nutrient and agricultural contaminant transport and turnover in the catchment, including the fate of pesticides. Using this information as a starting point for modelling studies, model uncertainty can be substantially reduced right from the beginning.
Landscape hydrology is not restricted to a single tool, like, e.g., a certain type of model, that could be applied in a schematic way for solving any problem. Rather it aims at systematically determining the constraints of the respective hydrological system currently being under study in order to achieve an internally consistent conceptual model. This is realized by means of a well-balanced set of complementary methods and tools of hydrological systems analysis like demonstrated in the Quillow study. It might not be spectacular at the first sight but is a very promising pathway to follow for the sake of sound understanding of given hydrological systems and for solving real-world problems for the benefit of society and nature.
However, likewise there is urgent need for developing advanced tools for analysis of hydrological behaviour and for determining constraints of the given system in a more sophisticated way. This approach is called “forensic hydrology”. Like in criminalistics, hydrologists need to be well equipped with a variety of powerful diagnostic tools to be able to meet the demands of modern society.
References
Allen RG, Pereira LS, Raes D, Smith M (1998) Crop evapotranspiration—Guidelines for computing crop water requirements. FAO Irrigation and drainage paper 56. Rome
Bencala KE, Gooseff MN, Kimball BA (2011) Rethinking hyporheic flow and transient storage to advance understanding of stream-catchment connections. Water Resour Res. Article ID W00H03. doi:10.1029/2010WR010066
Bergstrom A, Jencso K, McGlynn B (2016) Spatiotemporal processes that contribute to hydrologic exchange between hillslopes, valley bottoms, and streams. Water Resour Res 52:4628–4645. doi:10.1002/2015WR017972
Beven K (2001) On fire and rain (or predicting the effects of change). Hydrol Process 15:1397–1399. doi:10.1002/hyp.458
Blöschl G (2001) Scaling in hydrology. Hydrol Process 15:709–711. doi:10.1002/hyp.432
Borga M, Stoffel M, Marchi L, Marra F, Jakob M (2014) Hydrogeomorphic response to extreme rainfall in headwater systems: flash floods and debris flows. J Hydrol 518:194–205. doi:10.1016/j.jhydrol.2014.05.022
Böttcher S, Merz C, Lischeid G, Dannowski R (2014) Using Isomap to differentiate between anthropogenic and natural effects on groundwater dynamics in a complex geological setting. J Hydrol 519(Part B):1634–1641. doi:10.1016/j.jhydrol.2014.09.048
Cassardo C, Jones JAA (2011) Managing water in a changing world. Water 3:618–628. doi:10.3390/w3020618
Cey EE, Rudolph DL, Parkin GW, Aravena R (1998) Quantifying groundwater discharge to a small perennial stream in southern Ontario, Canada. J Hydrol 210(1–4):21–37
EU WFD (2000) Directive 2000/60/EC of the European Parliament and of the Council establishing a framework for the Community action in the field of water policy, 23 Oct 2000
Fleckenstein JH, Niswonger RG, Fogg GE (2006) River-aquifer interactions, geologic heterogeneity, and low flow management. Ground Water 44(6):837–852
Gerke HH, Rieckh H, Sommer M (2016) Interactions between crop, water, and dissolved organic and inorganic carbon in a hummocky landscape with erosion-affected pedogenesis. Soil Tillage Res 156:230–244
Hohenbrink T, Lischeid G (2015) Does textural heterogeneity matter? Quantifying transformation of hydrological signals in soils. J Hydrol 523:725–738. doi:10.1016/j.jhydrol.2015.02.009
Hohenbrink TL, Lischeid G, Schindler U, Hufnagel J (2016) Disentangling land management and soil heterogeneity effects on soil moisture dynamics. Vadose Zone J. doi:10.2136/vzj2015.07.0107
Hurst RW (2007) An overview of forensic hydrology. Southwest Hydrol 6(4):16–17
Hyndman DW, Kendall AD, Welty NRH (2007) Evaluating temporal and spatial variations in recharge and streamflow using the integrated landscape hydrology model (ILHM). In: Hyndman DW, DayLewis FD, Singha K (eds) Subsurface hydrology: data integration for properties and processes, vol 171. American Geophysical Union, pp 121–141
IHP (2012) The international hydrological programme. http://www.unesco.org/new/en/natural-sciences/environment/water/ihp-viii-water-security/. Assessed 26 Jan 2016
Kalbus E, Reinstorf F, Schirmer M (2006) Measuring methods for groundwater—surface water interactions: a review. Hydrol Earth Syst Sci 10:873–887
Kalettka T, Rudat C (2006) Hydrogeomorphic types of glacially created kettle holes in North-East Germany. Limnologica 36:54–64. doi:10.1016/j.limno.2005.11.001
Kappel WM (2014) Dating hydrological and geomorphic change using dendrochronology in Tully Valley, central New York: a summary. Tree-Ring Res 70(2):91–99
Kikuchi CP, Ferré TPA, Welker JM (2012) Spatially telescoping measurements of improved characterization of ground water-surface water interactions. J Hydrol 446–447:1–12. doi:10.1016/j.jhydrol.2012.04.002
Lehr C, Pöschke F, Lewandowski J, Lischeid G (2015) A novel method to evaluate the effect of a stream restoration on the spatial pattern of hydraulic connection of stream and groundwater. J Hydrol 527:394–401. doi:10.1016/j.jhydrol.2015.04.075
Lischeid G, Natkhin M, Steidl J, Dietrich O, Dannowski R, Merz C (2010) Assessing coupling between lakes and layered aquifers in a complex pleistocene landscape based on water level dynamics. Adv Water Resour 33(11):1331–1339. doi:10.1016/j.advwatres.2010.08.002
Lischeid G, Kalettka T, Merz C, Steidl J (2015) Monitoring the phase space of ecosystems: concept and examples from the Quillow catchment. Ecol.Indic, Uckermark. doi:10.1016/j.ecolind.2015.10.067
Lischeid G, Frei S, Huwe B, Bogner C, Lüers J, Babel W, Foken T (2017) Chapter 15: catchment evapotranspiration and runoff. In: Foken T (ed) Energy and matter fluxes of a spruce forest ecosystem. Ecological studies vol 229, Springer International Publishing AG, New York (in press). doi: 10.1007/978-3-319-49389-3
Loáiciga HA (2001) Flood damages in changing flood plains: a forensic-hydrologic case study. J Am Water Resour Assoc 37(2):467–478
McCallum JL, Cook PG, Berhane D, Rumpf C, McMahon GA (2012) Quantifying groundwater flows to streams using differential flow gaugings and water chemistry. J Hydrol 416–417:118–132. doi:10.1016/j.jhydrol.2011.11.040
McLaughlin DL, Kaplan DA, Cohen MJ (2014) A significant nexus: geographically isolated wetlands influence landscape hydrology. Water Resour Res 50(9):7153–7166. doi:10.1002/2013WR015002
Merz C, Steidl J (2015) Data on geochemical and hydraulic properties of a characteristic confined/unconfined aquifer system of the younger Pleistocene in northeast Germany. Earth Syst Sci Data 7(1):109–116. doi:10.5194/essd-7-109-2015
Merz C, Steidl J, Dannowski R (2009) Parameterization and regionalization of redox based denitrification for GIS-embedded nitrate transport modeling in Pleistocene aquifer systems. Environ Geol 58(7):1587–1599. doi:10.1007/s00254-008-1665-6
Milly PCD, Betancourt J, Falkenmark M, Hirsch RH, Kundzewicz ZW, Lettenmaier DP, Stouffer RJ (2008) Stationarity is dead: whither water management? Science 319:573–574. doi:10.1126/science.1151915
Montanari GY, Young G, Savenije HHG, Hughes D, Wagener T, Ren LL, Koutsoyiannis D, Cudennec C, Toth E, Grimaldi S, Blöschl G, Sivapalan M, Beven K, Gupta H, Hipsey M, Schaefli B, Arnheimer B, Boegh E, Schymanski SJ, Di Baldassarre G, Yu B, Hubert P, Huang Y, Schumann A, Post DA, Srinivasan V, Harman C, Thompson S, Rogger M, Viglione A, McMillan H, Characklis G, Pang Z, Belyaev V (2013) “Panta Rhei—everything flows”: change in hydrology and society—the IAHS Scientific Decade 2013–2022. Hydrol Sci J 58(6):1256–1275. doi:10.1080/02626667.2013.809088
Ramirez AI, Herrera A (2016) Forensic hydrology. In: Shetty BSK, Padubidri JR (eds) Forensic analysis—from death to justice. Chapter 3, pp 43–64. http://dx.doi.org/10.5772/64616
Richter D (1995) Ergebnisse methodischer Untersuchungen zur Korrektur des systematischen Meßfehlers des Hellmann-Niederschlagmessers. Berichte des Deutschen Wetterdienstes 194, Offenbach, p 93
Rieckh H, Gerke HH, Sommer M (2012) Hydraulic properties of characteristic horizons depending on relief position and structure in a hummocky glacial soil landscape. Soil Tillage Res 125:123–131. doi:10.1016/j.still.2012.07.004
Ruehl C, Fisher AT, Hatch C, Los Huertos ML, Stemler G, Shennan C (2006) Differential gauging and tracer tests resolve seepage fluxes in a strongly losing stream. J Hydrol 330(1–2):235–248. doi:10.1016/j.jhydrol.2006.03.025
Schindler U, Müller L, Eulenstein F, Dannowski R (2008) A long-term hydrological soil study on the effects of soil and land use on deep seepage dynamics in northeast Germany. Arch Agron Soil Sci 54(5):451–463
Seyfried MS, Wilcox BP (1995) Scale and the nature of spatial variability: field examples having implications for hydrologic modelling. Water Resour Res 31(1):173–184
Sivakumar B (2004) Dominant processes concept in hydrology: moving forward. Hydrol Process 18:2349–2353. doi:10.1002/hyp.5606
Sommer M, Gerke HH, Deumlich D (2008) Modelling soil landscape genesis: a “time split” approach for hummocky agricultural landscapes. Geoderma 145(3–4):480–493
R Core Team (2014) R: A language and environment for statistical computing. R Foundation for Statistical Computing, Vienna, Austria. http://www.R-project.org/
Terribile F, Coppola A, Langella G, Martina M, Basile A (2011) Potential and limitations of using soil mapping information to understand landscape hydrology. Hydrol Earth Syst Sci 15:3895–3933. doi:10.5194/hess-15-3895-2011
Thomas B, Lischeid G, Steidl J, Dannowski R (2012) Regional catchment classification with respect to low flow risk in a Pleistocene landscape. J Hydrol 475:392–402. doi:10.1016/j.jhydrol.2012.10.020
Wagener T, Sivapalan M, Troch PA, McGlynn BL, Harman CJ, Gupta HV, Kumar P, Rao PSC, Basu NB, Wilson JS (2010) The future of hydrology: an evolving science for a changing world. Water Resour Res 46:W05301. doi:10.1029/2009WR008906
Woodward C, Shulmeister J, Larsen J, Jacobsen GE, Zawadzki A (2014) Landscape hydrology. The hydrological legacy of deforestation on global wetlands. Science 346(6211):844–847. doi:10.1126/science.1260510
IUSS Working Group WRB (2006) World reference base for soil resources 2006. World soil resources reports no. 103. FAO, Rome. ISBN 2-5-105511-4
Yuan J, Cohen MJ, Kaplan DA, Acharya S, Larsen LG, Nungesser MK (2015) Linking metrics of landscape pattern to hydrological process in a lotic wetland. Landsc Ecol 30:1893–1912. doi:10.1007/s10980-015-0219-z
Zellweger GW, Avanzino RJ, Bencala KE (1998) Comparison of tracer-dilution and current-meter discharge measurements in a small gravel-bed stream, Little Lost Man Creek, California. US Geological Survey Water-Resources Investigations Report 89-4150. Menlo Park, California
Acknowledgements
The authors are deeply indebted to Roswitha Schulz, Dorith Henning, Ralph Tauschke, Joachim Bartelt, Bernd Schwien and numerous students who did most of the field work, partly under very harsh conditions. We highly appreciate the groundwater head and discharge data provided by the Landesamt für Umwelt, Gesundheit und Verbraucherschutz Brandenburg (State Office of Environment, Health and Consumer Protection Brandenburg; LUGV) and the high-resolution digital elevation data by Landesvermessung und Geobasisinformation Brandenburg (State Survey and Geospatial Basic Information Brandenburg; LGB) acting on behalf of the State Office of Environment, Health and Consumer Protection Brandenburg. Part of the data used for this study have been collected within or associated with the LandScales project, financed by the Leibniz Association, which is highly appreciated.
Author information
Authors and Affiliations
Corresponding author
Additional information
This article is part of a Topical Collection in Environmental Earth Sciences on “Water in Germany”, guest-edited by Daniel Karthe, Peter Chifflard, Bernd Cyffka, Lucas Menzel, Heribert Nacken, Uta Raeder, Mario Sommerhäuser and Markus Weiler.
Rights and permissions
Open Access This article is distributed under the terms of the Creative Commons Attribution 4.0 International License (http://creativecommons.org/licenses/by/4.0/), which permits unrestricted use, distribution, and reproduction in any medium, provided you give appropriate credit to the original author(s) and the source, provide a link to the Creative Commons license, and indicate if changes were made.
About this article
Cite this article
Lischeid, G., Balla, D., Dannowski, R. et al. Forensic hydrology: what function tells about structure in complex settings. Environ Earth Sci 76, 40 (2017). https://doi.org/10.1007/s12665-016-6351-5
Received:
Accepted:
Published:
DOI: https://doi.org/10.1007/s12665-016-6351-5