Abstract
The sudden and harmful nature of rockbursts in tunnels necessitates an accurate and applicable method for automatically recognizing rock fracture signals during rockburst microseismic (MS) monitoring. In this paper, the performance and applicability of recognizing MS waveforms using an artificial neural network (ANN) and a deep neural network (DNN) were studied in tunnels excavated by different methods. The results show that ANN performs very well in recognizing rock fracturing waveforms with a signal-to-noise ratio (SNR) ≥ 3 but has a low accuracy for those with an SNR < 3. The DNN also performs well for waveforms with SNR ≥ 3, and has a relatively high accuracy for waveforms with SNR < 3. The ANN model can be used in tunnels excavated by drilling and blasting (D&B) since there are fewer “small” rock fracturing events. The DNN model is applicable in tunnels excavated by the tunnel boring machine (TBM), recognizing more “small” events. In addition, the ANN model is a better choice, with fewer training samples at the initial stage of monitoring working. With continuous monitoring, the DNN model can be used to ensure and improve the accuracy. These results lay a foundation for automatic rockburst MS monitoring techniques in tunnel engineering.
Similar content being viewed by others
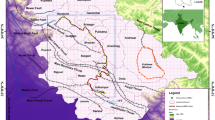
References
Bi L, Xie W, Zhao JJ (2019) Automatic recognition and classification of multi-channel microseismic waveform based on DCNN and SVM. Computers and Geosciences 123:111–120, DOI: https://doi.org/10.1016/j.cageo.2018.10.008
Bradley AE (1997) The use of the area under the ROC curve in the evaluation of machine learning algorithms. Pattern Recognition 30(7):1145–1159, DOI: https://doi.org/10.1016/S0031-3203(96)00142-2
Chen LX, Guo WY, Zhang DX, Zhao TB (2022) Experimental study on the influence of prefabricated fissure size on the directional propagation law of rock type-I crack. International Journal of Rock Mechanics and Mining Sciences 160:105274, DOI: https://doi.org/10.1016/j.ijrmms.2022.105274
Dai LY, Dong HL, Li XG (2019) Review of miceoseismic data denoising dethods. Journal of Jilin University(Earth Science Edition) 49(4): 1145–1159, DOI: https://doi.org/10.13278/j.cnki.jjuese.20180128
Del PE, Esposito A, Giudicepietro F, Marinaro M, Martini M, Scarpetta S (2003) Discrimination of earthquakes and underwater explosions using neural networks. Bulletin of the Seismological Society of America 93(1):215–223, DOI: https://doi.org/10.1785/0120020005
Dong LJ, Wesseloo J, Potvin Y, Li XB (2016a) Discriminant models of blasts and seismic events in mine seismology. International Journal of Rock Mechanics and Mining Sciences 86:282–291, DOI: https://doi.org/10.1016/j.ijrmms.2016.04.021
Dong LJ, Wesseloo J, Potvin Y, Li XB (2016b) Discrimination of mine seismic events and blasts using the fisher classifier, Naive Bayesian classifier and logistic regression. Rock Mechanics and Rock Engineering 49(1):183–211, DOI: https://doi.org/10.1007/s00603-015-0733-y
Feng XT (2018) Rockburst mechanisms, monitoring, warning, and mitigation, Butterworth-Heinemann, DOI: https://doi.org/10.1016/C2015-0-01493-9
Feng GL, Chen BR, Xiao YX, Jiang Q, Li PX, Zheng H, Zhang W (2022a) Microseismic characteristics of rockburst development in deep TBM tunnels with alternating soft–hard strata and application to rockburst warning: A case study of the Neelum–Jhelum hydropower project. Tunnelling and Underground Space Technology 122:104398, DOI: https://doi.org/10.1016/j.tust.2022.104398
Feng GL, Feng XT, Chen BR, Xiao YX, Yu Y (2014) A microseismic method for dynamic warning of eockburst development processes in tunnels. Rock Mechanics and Rock Engineering 48(5):2061–2076, DOI: https://doi.org/10.1007/s00603-014-0689-3
Feng GL, Feng XT, Xiao YX, Yao ZB, Hu L, Niu WJ, Li T (2019) Characteristic microseismicity during the development process of intermittent rockburst in a deep railway tunnel. International Journal of Rock Mechanics and Mining Sciences 124:104135, DOI: https://doi.org/10.1016/j.ijrmms.2019.104135
Feng XT, Yang CX, Kong R, Zhao J, Zhou YY, Yao ZB, Hu L (2022b) Excavation-induced deep hard rock fracturing: Methodology and applications. Journal of Rock Mechanics and Geotechnical Engineering 14:1–34, DOI: https://doi.org/10.1016/j.jrmge.2021.12.003
Gu ST, Zhang W, Jiang BY, Hu CC (2018) Case of rock burst danger and its prediction and prevention in tunneling and mining period at an irregular coal face. Geotechnical and Geological Engineering 37(4):2545–2564, DOI: https://doi.org/10.1007/s10706-018-00776-y
Hu L, Feng XT, Xiao YX, Feng GL, Li SJ, Pan PZ, Yao ZB (2019) Characteristics of the microseismicity resulting from the construction of a deeply-buried shaft. Tunnelling and Underground Space Technology 85:114–127, DOI: https://doi.org/10.1016/j.tust.2018.12.016
Huang LQ, Li J, Hao H, Li XB (2018) Micro-seismic event detection and location in underground mines by using Convolutional Neural Networks (CNN) and deep learning. Tunnelling and Underground Space Technology 81:265–276, DOI: https://doi.org/10.1016/j.tust.2018.07.006
Jozefowicz R, Zaremba W, Sutskever I (2015) An empirical exploration of recurrent network architectures. International Conference on International Conference on Machine Learning
Li PX, Feng XT, Feng GL, Xiao YX, Chen BR (2019) Rockburst and microseismic characteristics around lithological interfaces under different excavation directions in deep tunnels. Engineering Geology 260:105209, DOI: https://doi.org/10.1016/j.enggeo.2019.105209
Li B, Li N, Wang E, Li X, Niu Y, Zhang X (2017) Characteristics of coal mining microseismic and blasting signals at Qianqiu coal mine. Environmental Earth Sciences 76:722, DOI: https://doi.org/10.1007/s12665-017-7070-2
Li B, Wang E, Li Z, Niu Y, Li N, Li X (2021) Discrimination of different blasting and mine microseismic waveforms using FFT, SPWVD and multifractal method. Environmental Earth Sciences 80:36, DOI: https://doi.org/10.1007/s12665-020-09330-7
Liu B, Wang R, Zhao G Guo X, Wang Y, Li J, Wang S (2020) Prediction of rock mass parameters in the TBM tunnel based on BP neural network integrated simulated annealing algorithm. Tunnelling and Underground Space Technology 95:103103, DOI: https://doi.org/10.1016/j.tust.2019.103103
Liu Q, Zhang F, Liu M, Shen W (2016) A fault prediction method based on modified genetic algorithm using BP neural network algorithm. Paper presented at the 2016 IEEE International Conference on Systems, Man, and Cybernetics (SMC)
Malovichko D (2012) Discrimination of blasts in mine seismology. Deep and High Stress Mining, 161–172, DOI: https://doi.org/10.36487/ACG_rep/120111_malovichko
Musil M, Plesinger A (1996) Discrimination between local microearthquakes and quarry blasts by multi-layer perceptrons and Kohonen maps. Bulletin of the Seismological Society of America 86(84):1077–1090, DOI: https://doi.org/10.1785/BSSA0860041077
Paul BQ, Pierre G Yoann C, Munkhuu U (2015) Detection and classification of seismic events with progressive multi-channel correlation and hidden Markov models. Computers and Geosciences 83:110–119, DOI: https://doi.org/10.1016/j.cageo.2015.07.002
Peng PA, Wang LG, Pei AL (2019) Non-parametric automatic microseismic data denoising via PD method and its application. Chinese Journal of Rock Mechanics and Engineering 38(s1):3061–3069, DOI: https://doi.org/10.13722/j.cnki.jrme.2017.1151
Perola T, Gharbib ME, Denolle MA (2018) Convolutional neural network for earthquake detection and location. Science Advances 4(2): E1700578, DOI: https://doi.org/10.1126/sciadv.1700578
Ross ZE, Meier MA, Hauksson E, Heaton TH (2018) Generalized seismic phase detection with deep learning. Bulletin of the Seismological Society of America 108(5A):2894–2901, DOI: https://doi.org/10.1785/0120180080
Vallejos JA, McKinnon SD (2013) Logistic regression and neural network classification of seismic records. International Journal of Rock Mechanics and Mining Sciences 62:86–95, DOI: https://doi.org/10.1016/j.ijrmms.2013.04.005
Xiao YX, Feng XT, Chen BR, Feng GL (2018) Chapter 10 - Latest developments on analysis of data monitored. In X. T. Feng (Ed.), Rockburst (pp. 317-338): Butterworth-Heinemann
Xiao YX, Feng XT, Hudson JA, Chen BR, Feng GL, Liu JP (2015) ISRM suggested method for in situ microseismic monitoring of the fracturing process in rock masses. Rock Mechanics and Rock Engineering 49(1):343–369, DOI: https://doi.org/10.1007/s00603-015-0859-y
Zhang W, Feng XT, Bi X, Yao ZB, Xiao YX, Hu L, Niu WJ, Feng GL (2021) An arrival time picker for microseismic rock fracturing waveforms and its quality control for automatic localization in tunnels. Computers and Geotechnics 135:104175, DOI: https://doi.org/10.1016/j.compgeo.2021.104175
Zhang W, Feng XT, Xiao YX, Feng GL, Yao ZB, Hu L, Niu WJ (2020) A rockburst intensity criterion based on the Geological Strength Index, experiences learned from a deep tunnel. Bulletin of Engineering Geology and the Environment 79(7):3585–3603, DOI: https://doi.org/10.1007/s10064-020-01774-2
Zhang W, Feng XT, Yao ZB, Hu L, Xiao YX, Feng GL, Niu WJ, Zhang Y (2022) Development and occurrence mechanisms of fault-slip rockburst in a deep tunnel excavated by drilling and blasting: A case study. Rock Mechanics and Rock Engineering 55:5599–5618, DOI: https://doi.org/10.1007/s00603-022-02927-3
Zhang CQ, Feng XT, Zhou H, Qiu SL, Yang YS (2013) Rock mass damage induced by rockbursts occurring on tunnel floors: A case study of two tunnels at the Jinping II Hydropower Station. Environmental Earth Sciences 71(1):441–450, DOI: https://doi.org/10.1007/s12665-013-2451-7
Zhao M, Chen S, Dave Y (2019) Waveform classification and seismic recognition by convolution neural network. Chinese Journal of Geophysics 62(1):374–382, DOI: https://doi.org/10.6038/cjg2019M0151
Zhao TB, Guo WY, Tan YL, Yin YC, Cai LS, Pan JF (2018) Case studies of rock bursts under complicated geological conditions during multi-seam mining at a depth of 800 m. Rock Mechanics and Rock Engineering 51(5):1–26, DOI: https://doi.org/10.1007/s00603-018-1411-7
Zhao JS, Jiang Q, Lu JF, Chen BR, Pei SF, Wang ZL (2022) Rock fracturing observation based on microseismic monitoring and borehole imaging: In situ investigation in a large underground cavern under high geostress. Tunnelling and Underground Space Technology 126: 104549, DOI: https://doi.org/10.1016/j.tust.2022.104549
Zhou GB, Wu JX, Zhang CL, Zhou ZH (2016) Minimal gated unit for recurrent neural networks. International Journal of Automation and Computing 13(3):226–234, DOI: https://doi.org/10.1007/s11633-016-1006-2
Zhu QJ, Jiang FX, Yin YM, Yu ZX, Wen JL (2012) Classification of mine microseismic events based on wavelet-fractal method and pattern recognition. Chinese Journal of Geotechnical Engineering 34(11):2036–2042
Acknowledgments
This research was partially supported by the National Natural Science Foundation of China (grant no. 52109116), and the Fundamental Research Funds for the Central Universities (grant No. N2224004-031, No. N232405-04).
Author information
Authors and Affiliations
Corresponding author
Rights and permissions
About this article
Cite this article
Zhang, W., Bi, X., Hu, L. et al. Performance and Applicability of Recognizing Microseismic Waveforms Using Neural Networks in Tunnels. KSCE J Civ Eng 28, 951–966 (2024). https://doi.org/10.1007/s12205-023-0719-3
Received:
Revised:
Accepted:
Published:
Issue Date:
DOI: https://doi.org/10.1007/s12205-023-0719-3