Abstract
A key issue for effective management and operating of dam reservoirs is predicting the water inflow values into dam reservoir. To address this subject, here, genetic programming (GP) is used by proposing two cases. In the first case, water inflow values are predicted separately for each month. However, in the second case, these values are predicted simultaneously for all months. Furthermore, for each case, two approaches are proposed here. In the first approach, the hybrid method, called the ANN-NGSA-II method, is proposed to find proper input data sets. However, in the second approach, the useful input data sets are found automatically using the GP method. For comparison purpose, the ANN and SARIMA models are also used, to predict the water inflow values. As a case study, in this research, the Zayandehroud dam reservoir is selected. The results indicate that the ANN model outperforms both results of the GP and SARIMA methods. In other words, correlation coefficient (R2), Nash Sutcliffe (NS), and root means square error (RMSE) values of ANN are 0.97, (0.88), 0.954 (0.87), and 17.19 (30.54) million cubic meters, respectively, for training (test) data set.
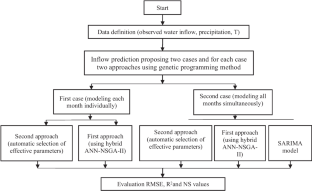











Similar content being viewed by others
Availability of Data and Materials
The datasets generated during and/or analyzed during the current study are available from the corresponding author upon reasonable request.
References
Abdollahi S, Raeisi J, Khalilianpour M, Ahmadi F, Kisi O (2017) Daily mean streamflow prediction in perennial and non-perennial rivers using four data driven techniques. Water Resour Manag 31(15):4855–4874
Ahmadi F, Mehdizadeh S, Nourani V (2022) Improving the performance of random forest for estimating monthly reservoir inflow via complete ensemble empirical mode decomposition and wavelet analysis. Stoch Env Res Risk Assess 36(9):2753–2768
Babaei M, Moeini R, Ehsanzadeh E (2019) Artificial neural network and support vector machine models for inflow prediction of dam reservoir (case study: Zayandehroud dam reservoir). Water Resour Manag 33(6):2203–2218
Bai Y, Chen Z, Xie J, Li C (2016) Daily reservoir inflow forecasting using multiscale deep feature learning with hybrid models. J Hydrol 532:193–206
Bhavita K, Swathi D, Manideep J, Sandeep DS, Rathinasamy M (2019) Regime-wise genetic programming model for improved streamflow forecasting. Water Resour Environ Eng I, Singapore, p. 195–201
Box GE, Jenkins GM, Reinsel GC, Ljung GM (2015) Time series analysis: forecasting and control. John Wiley & Sons
Budu K (2014) Comparison of wavelet-based ANN and regression models for reservoir inflow forecasting. J Hydrol Eng 19(7):1385–1400
Chang FJ, Chen YC (2001) A counter propagation fuzzy-neural network modeling approach to real time stream flow prediction. J Hydrol 245(1–4):153–164
Chaplot B, Birbal P (2022) Development of stage-discharge rating curve using ANN. Int J Hydrol Sci Technol 14(1):75–79
Coulibaly P, Anctil F, Bobee B (2000) Daily reservoir inflow forecasting using artificial neural networks with stopped training approach. J Hydrol 230(3–4):244–257
Coulibaly P, Haché M, Fortin V, Bobee B (2005) Improving daily reservoir inflow forecasts with model combination. J Hydrol Eng 10(2):91–99
Cryer JD, Chan K-S, Chan K-S (2008) Time series analysis: with applications in R (Vol. 2). Springer
Danandeh Mehr A, Kahya D, Olyaie E (2013) Streamflow prediction using linear genetic programming in comparison with a neuro-wavelet technique. J Hydrol 505:240–249
Fallah Mehdipour F, Bozorg Haddad O, Mariño MA (2012) Real-time operation of reservoir system by genetic programming. Water Resour Manag 26(14):4091–4103
French MN, Krajewski WF, Cuykendall RR (1992) Rainfall forecasting in space and time using a neural network. J Hydrol 137(1–4):1–31
Ghorbani MA, Khatibi R, Mehr AD, Asadi H (2018) Chaos-based multigene genetic programming: A new hybrid strategy for river flow forecasting. J Hydrol 562:455–467
Hadi SJ, Tombul M (2018) Monthly stream flow forecasting using continuous wavelet and multi-gene genetic programming combination. J Hydrol 561:674–687
Hadiyan PP, Moeini R, Ehsanzadeh E (2020) Application of static and dynamic artificial neural networks for forecasting inflow discharges, case study: Sefidroud Dam reservoir. Sustain Comput: Inform Syst 27:100401
Jain SK, Das A, Srivastava DK (1999) Application of ANN for reservoir inflow prediction and operation. J Water Resour Plan Manag 125(5):263–271
Johari A, Habibagahi G, Ghahramani A (2006) Prediction of soil–water characteristic curve using genetic programming. J Geotech Geoenviron Eng 132(5):664–665
Khorram S, Jehbez N (2023) A hybrid CNN-LSTM approach for monthly reservoir inflow forecasting. Water Resour Manag 37(10):4097–4121
Kim T, Shin J-Y, Kim H, Kim S, Heo J-H (2019) The use of large-scale climate indices in monthly reservoir inflow forecasting and its application on time series and artificial intelligence models. Water 11(2):374
Kumar S, Tiwari MK, Chatterjee C, Mishra A (2015) Reservoir inflow forecasting using ensemble models based on neural networks, wavelet analysis and bootstrap method article. Water Resour Manag 29(13):4863–4883
Latif SD, Ahmed AN (2023) Streamflow prediction utilizing deep learning and machine learning algorithms for sustainable water supply management. Water Resour Manage 37(8):3227–3241
Li F, Ma G, Chen S, Huang W (2021) An ensemble modeling approach to forecast daily reservoir inflow using bidirectional long- and short-term memory (Bi-LSTM), variational mode decomposition (VMD), and energy entropy method. Water Resour Manag 35(9):2941–2963
Lin GF, Chen GR, Huang PY, Chou YC (2009) Support vector machine-based models for hourly reservoir inflow forecasting during typhoon-warning periods. J Hydrol 372(1–4):17–29
Lin GF, Wu MC (2011) An RBF network with a two-step learning algorithm for developing a reservoir inflow forecasting model. J Hydrol 405(3–4):439–450
Livingstone DJ, Manallack DT, Tetko IV (1997) Data modelling with neural networks: advantages and limitations. J Comput Aided Mol Des 11:135–142
Mishra AK, Desai VR (2006) Drought forecasting using feed-forward recursive neural network. Ecol Model 198(1–2):127–138
Mijwel MM (2021) Artificial neural networks advantages and disadvantages. Mesop J Big Data 2021:29–31
Muluye GY, Coulibaly P (2007) Seasonal reservoir inflow forecasting with low-frequency climatic indices: A comparison of data-driven methods. Hydrol Sci J 52(3):508–522
Nasseri M, Moeini A, Tabesh M (2011) Forecasting monthly urban water demand using Extended Kalman Filter and Genetic Programming. Expert Syst Appl 38(6):7387–7395
Nayak PC, Sudheer KP (2008) Fuzzy model identification based on cluster estimation for reservoir inflow forecasting. Hydrol Process: Int J 22(6):827–841
Ni Q, Wang L, Ye R, Yang F, Sivakumar M (2010) Evolutionary modeling for streamflow forecasting with minimal datasets: a case study in the West Malian River, China. Environ Eng Sci 27(5):377–385
O’Neill M, Vanneschi L, Gustafson S, Banzhaf W (2010) Open issues in genetic programming. Genet Program Evolvable Mach 11:339–363
Partal T, Kişi Ö (2007) Wavelet and neuro-fuzzy conjunction model for precipitation forecasting. J Hydrol 342(1–2):199–212
Poorsepahy-Samian H, Espanmanesh V, Zahraie B (2016) Improved inflow modeling in stochastic dual dynamic programming. J Water Resour Plan Manag 142(12):04016065
Rajesh M, Anishka S, Viksit PS, Arohi S, Rehana S (2023) Improving short-range reservoir inflow forecasts with machine learning model combination. Water Resour Manag 37(1):75–90
Raman H, Sunilkumar N (1995) Multivariate modelling of water resources time series using artificial neural networks. Hydrol Sci J 40(2):145–163
Saadat M, Asghari K (2017) Reliability improved stochastic dynamic programming for reservoir operation optimization. Water Resour Manag 31(6):1795–1807
Safavi HR, Golmohammadi MH, Sandoval-Solis S (2015) Expert knowledgebased modeling for integrated water resources planning and management in the Zayandehrud River Basin. J Hydrol 528:773–789
Sattari MT, Yurekli K, Pal M (2012) Performance evaluation of artificial neural network approaches in forecasting reservoir inflow. Appl Math Model 36(6):2649–2657
Sepahvand R, Safavi HR, Rezaei F (2019) Multi-objective planning for conjunctive use of surface and ground water resources using genetic programming. Water Resour Manag 33(6):2123–2137
Shelke M, Londhe SN, Dixit PR, Kolhe P (2023) Reservoir inflow prediction: a comparison between semi distributed numerical and artificial neural network modelling. Water Resour Manag 37(15):6127–6143
Shiri J, Kişi Ö (2011) Comparison of genetic programming with neuro-fuzzy systems for predicting short-term water table depth fluctuation. Comput Geosci 37(10):1692–1701
Tadesse KB, Dinka MO (2017) Application of SARIMA model to forecasting monthly flows in Waterval River, South Africa. J Water Land Dev 35(1):229
Verma S, Pant M, Snasel V (2021) A comprehensive review on NSGA-II for multi-objective combinatorial optimization problems. IEEE Access 9:57757–57791
Wang J, Du Y, Zhang X (2008) Theory and application with seasonal time series. Nankai University Press, China
Wang S, Li C, Lim A (2019) Why are the ARIMA and SARIMA not sufficient. arXiv preprint arXiv:1904.07632
Xu W, Zhang C, Peng Y, Fu G, Zhou H (2014) A two stage Bayesian stochastic optimization model for cascaded hydropower systems considering varying uncertainty of flow forecasts. Water Resour Res 50(12):9267–9286
Funding
This research did not receive any specific grant from funding agencies in the public, commercial, or not-for-profit sector.
Author information
Authors and Affiliations
Contributions
Ramtin Moeini contributed to the study's conception and design. Material preparation, data collection, and analysis were performed by Kamran Nasiri. Finally, the SARIMA model is calibrated and used by Seyed Hossein Hosseini. The manuscript was written by Ramtin Moeini. All authors read and approved the final manuscript.
Corresponding author
Ethics declarations
Ethical Approval
This article does not contain any studies with human participants or animals performed by any of the authors.
Consent to Participate
Informed consent was obtained from all individual participants included in the study.
Financial Interest
Ramtin Moeini, Kamran Nasiri, and Seyed Hossein Hosseini declare they have no financial interests.
Additional information
Publisher's Note
Springer Nature remains neutral with regard to jurisdictional claims in published maps and institutional affiliations.
Rights and permissions
Springer Nature or its licensor (e.g. a society or other partner) holds exclusive rights to this article under a publishing agreement with the author(s) or other rightsholder(s); author self-archiving of the accepted manuscript version of this article is solely governed by the terms of such publishing agreement and applicable law.
About this article
Cite this article
Moeini, R., Nasiri, K. & Hosseini, S.H. Predicting the Water Inflow Into the Dam Reservoir Using the Hybrid Intelligent GP-ANN- NSGA-II Method. Water Resour Manage (2024). https://doi.org/10.1007/s11269-024-03856-2
Received:
Accepted:
Published:
DOI: https://doi.org/10.1007/s11269-024-03856-2