Abstract
With the rapid development of the Internet of Things and smart wearable devices, there is an increasing demand for motion capture and image recognition technology. This study aims to develop an optical motion capture system based on wearable devices of the Internet of Things to achieve accurate recognition and dynamic visual simulation of dance movements. This paper uses a motion capture technique based on optical principle. First, multiple optical sensors were installed on the wearable device to capture the dancers' movements. Then through the image recognition algorithm, the image is processed and analyzed to extract the dancers' posture and movement information. Finally, through the simulation algorithm, the captured dancers' movements are transformed into dynamic visualizations in real time. The experimental results show that the system can accurately capture and identify the movements of different dancers and provide high-quality dynamic visualizations. Through the system, dancers can observe their movements in real time, check and improve dance skills, and improve the quality of dance performance.





Similar content being viewed by others
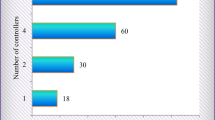
Data availability
The data will be available upon request.
References
Deng, L., Leung, H., Gu, N., Yang, Y.: Real-time mocap dance recognition for an interactive dancing game. Comput Anim. Virtual Worlds 22(2–3), 229–237 (2011)
Devi, M., Saharia, S., Bhattacharyya, D.K.: Dance gesture recognition: a survey. Int. J. Comput. Appl. 122(5), 19–26 (2015)
Fujimoto, M., Fujita, N., Takegawa, Y., Terada, T., Tsukamoto, M.: A motion recognition method for a wearable dancing musical instrument. In 2009 International symposium on wearable computers, pp. 11–18. IEEE, (2009)
Heryadi, Y., Fanany, M. I., & Arymurthy, A. M.: Stochastic regular grammar-based learning for basic dance motion recognition. In: 2013 international conference on advanced computer science and information systems (ICACSIS), pp. 419–424. IEEE, (2013)
Iqbal, J., Sidhu, M.S.: Acceptance of dance training system based on augmented reality and technology acceptance model (TAM). Virtual Real. 26(1), 33–54 (2022)
Iqbal, S.M., Mahgoub, I., Du, E., Leavitt, M.A., Asghar, W.: Advances in healthcare wearable devices. NPJ Flex Electron 5(1), 289–294 (2021)
Seneviratne, S., Hu, Y., Nguyen, T., Lan, G., Khalifa, S., Thilakarathna, K., Hassan, M., Seneviratne, A.: A survey of wearable devices and challenges. IEEE Commun. Surv. Tutor. 19(4), 2573–2620 (2017)
Shen, D., Jiang, X., Teng, L.: Residual network based on convolution attention model and feature fusion for dance motion recognition. EAI Endors Trans. Scalable Inform. Syst. 9(4), 56–63 (2021)
Shi, Y.: Stage performance characteristics of minority dance based on human motion recognition. Mob. Inform. Syst. (2022). https://doi.org/10.1155/2022/1940218
Tran, D., Bourdev, L., Fergus, R., Torresani, L., Paluri, M.: Learning spatiotemporal features with 3d convolutional networks. In: Proceedings of the IEEE international conference on computer vision, pp. 4489–4497, (2015).
Tsuchida, S., Fukayama, S., Hamasaki, M., Goto, M.: AIST dance video database: multi-genre, multi-dancer, and multi-camera database for dance information processing. In ISMIR, Vol. 1, No. 5, p. 6, (2019)
Wu, H.: Design of embedded dance teaching control system based on FPGA and motion recognition processing. Microprocess. Microsyst. 83, 102–107 (2021)
Zhai, X.: Dance movement recognition based on feature expression and attribute mining. Complexity 2021, 1–12 (2021)
Zhang, S.: An intelligent and fast dance action recognition model using two-dimensional convolution network method. J. Environ. Public Health 2022, 4713643–4713643 (2022)
Zhang, H.B., Zhang, Y.X., Zhong, B., Lei, Q., Yang, L., Du, J.X., Chen, D.S.: A comprehensive survey of vision-based human action recognition methods. Sensors 19(5), 1005–1013 (2019)
Funding
The authors have not disclosed any funding.
Author information
Authors and Affiliations
Contributions
YZ has done the first version, ZW has done the simulations. All authors have contributed to the paper’s analysis, discussion, writing, and revision.
Corresponding author
Ethics declarations
Conflict of interest
The authors declare that they have no competing interests.
Additional information
Publisher's Note
Springer Nature remains neutral with regard to jurisdictional claims in published maps and institutional affiliations.
Rights and permissions
Springer Nature or its licensor (e.g. a society or other partner) holds exclusive rights to this article under a publishing agreement with the author(s) or other rightsholder(s); author self-archiving of the accepted manuscript version of this article is solely governed by the terms of such publishing agreement and applicable law.
About this article
Cite this article
Zhang, Y., Wang, Z. Dynamic visualization simulation of light motion capture in dance image recognition based on IoT wearable devices. Opt Quant Electron 56, 141 (2024). https://doi.org/10.1007/s11082-023-05734-4
Received:
Accepted:
Published:
DOI: https://doi.org/10.1007/s11082-023-05734-4