Abstract
Atmospheric thermodynamic data are gathered by high technology remote instruments such as radiosondes, giving rise to profiles that are usually modelled as functions depending only on height. The radiosonde balloons, however, drift away in the atmosphere resulting in not necessarily vertical but three-dimensional trajectories. To model this kind of functional data, we introduce a “point based” formulation of an heteroskedastic functional regression model that includes a trivariate smooth function and results to be an extension of a previously introduced unidimensional model. Functional coefficients of both the conditional mean and variance are estimated by reformulating the model as a standard generalized additive model and subsequently as a mixed model. This reformulation leads to a double mixed model whose parameters are fitted by using an iterative algorithm that allows to adjust for heteroskedasticity. The proposed modelling approach is applied to describe collocation mismatch when we deal with couples of balloons launched at two different locations. In particular, we model collocation error of atmospheric pressure in terms of meteorological covariates and space and time mismatch. Results show that model fitting is improved once heteroskedasticity is taken into account.











Similar content being viewed by others
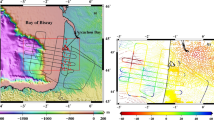
References
Berberan-Santos MN, Bodunov EN, Pogliani L (2010) On the barometric formula inside the earth. J Math Chem 47(3):990–1004
Caballero W, Giraldo R, Mateu J (2013) A universal kriging approach for spatial functional data. Stoch Environ Res Risk Assess 27(7):1553–1563
Cuevas A (2014) A partial overview of the theory of statistics with functional data. J Stat Plan Inference 147:1–23
Eilers PHC, Marx BD (2003) Multivariate calibration with temperature interaction using two-dimensional penalized signal regression. Chemom Intell Lab Syst 66:159–174
Fassò A, Ignaccolo R, Madonna F, Demoz B (2013) Statistical modelling of atmospheric vertical profiles and the collocation problem. Atmos Meas Tech Discuss 6:7505–7533. doi:10.5194/amtd-6-7505-2013
Escabias M, Valderrama J, Aguilera AM, Santofimia ME, Aguilera-Morillo MC (2013) Stepwise selection of functional covariates in forecasting peak levels of olive pollen. Stoch Environ Res Risk Assess 27(2):367–376
Ferraty F, Vieu P (2006) Nonparametric functional data analysis: theory and practice. Springer, New York
Gijbels I, Prosdocimi I, Claeskens G (2010) Nonparametric estimation of mean and dispersion functions in extended generalized linear models. Test 19:580–608
Guo W (2004) Functional data analysis in longitudinal settings using smoothing splines. Stat Methods Med Res 13:49–62
Harezlak J, Coull BA, Laird NM, Magari SR, Christiani DC (2007) Penalized solutions to functional regression problems. Comput Stat Data Anal 99:4911–4925
Hastie T, Tibshirani R (1993) Varying-coefficient models. J R Stat Soc B 55:757–796
Horvàth L, Kokoszka P (2012) Inference for functional data with applications. Springer, New York
Ignaccolo R, Mateu J, Giraldo R (2013) Kriging with external drift for functional data for air quality monitoring. Stoch Environ Res Risk Assess. doi:10.1007/s00477-013-0806-y
Immler FJ, Dykema J, Gardiner T, Whiteman DN, Thorne PW, Vomel H (2010) Reference quality upper-air measurements: guidance for developing GRUAN data products. Atmos Meas Tech 3:1217–1231
Ivanescu AE, Staicu AM, Greven S, Scheipl F, Crainiceanu CM (2012) Penalized function-on-function regression (April 2012). Johns Hopkins University, Dept. of Biostatistics Working Papers. Working Paper 240
Karlis D, Vasdekis VGS, Banti M (2009) Heteroscedastic semiparametric models for domestic water consumption aggregated data. Environ Ecol Stat 16:355–367
Nash J, Oakley T, Vömel H, LI Wei (2010) WMO Intercomparison of high Quality Radiosonde Systems Yangjiang, China, 12 July–3 August 2010; WMO report reference number IOM 107 (TD 1580). available at: http://www.wmo.int/pages/prog/www/IMOP/publications-IOM-series.html
Ngo L, Wand MP (2004) Smoothing with mixed model software. J Stat Softw 71(9):1–54
Nott DJ (2006) Semiparametric estimation of mean and variance functions for non-Gaussian data. Comput Stat 21:603–620
Ramsay JO, Silverman BW (2005) Functional data analysis. Springer, New York
R Core Team (2013) R: A language and environment for statistical computing. R Foundation for Statistical Computing, Vienna, Austria. URL http://www.R-project.org/
Reiss PT, Ogden RT (2009) Smoothing parameter selection for a class of semiparametric linear models. J R Stati Soc B 71:50517523
Robinson GK (1991) That BLUP is a good thing: the estimation of random effects. Stati Sci 6:15–32
Ruiz-Medina MD, Espejo RM (2012) Spatial autoregressive functional plug-in prediction of ocean surface temperature. Stoch Environ Res Risk Assess 26:335–344
Ruppert D, Wand MP, Carroll RJ (2003) Semiparametric regression. Cambridge University Press, New York
Seidel DJ, Sun B, Pettey M, Reale A (2011), Global radiosonde balloon drift statistics. J Geophys Res 116:D7
Speed T (1991) Comment on paper by Robinson. Stati Sci 6:421744
Thorne PW, V\(\ddot{\rm o}\)mel H, Bodeker G et al (2013) GCOS reference upper air network (GRUAN): Steps towards assuring future climate records. AIP Conference Proceedings 1552:1042–1047. doi:http://dx.doi.org/10.1063/1.4821421
Wahba G (1990) Spline models for observational data. SIAM, Philadelphia
Wand MP (2003) Smoothing and mixed models. Comput Stat 18:223–249
Wang H, Akritas MG (2010) Inference from heteroscedastic functional data. J Nonparametric Stat 22(2):149–168
Wood SN (2004) Stable and efficient multiple smoothing parameter estimation for generalized additive models. J Am Stat Assoc 99:673–686
Wood AN (2006) Generalized additive models: an introduction with R. Chapman & Hall/CRC, Boca Raton
Wood SN (2011) Fast stable restricted maximum likelihood and marginal likelihood estimation of semiparametric generalized linear models. J R Stat Soc B 73(1):3–36
Wood SN (2012) mgcv: Mixed GAM computation vehicle with GCV/AIC/REML smoothness estimation, R package version 1.7–22
Wood SN (2013) On p values for smooth components of an extended generalized additive model. Biometrika 100(1):221–228
Zhang JT (2013) Analysis of variance for functional data. Chapman & Hall/CRC, Boca Raton
Acknowledgments
The authors would like to thank Belay Demoz and Fabio Madonna for useful discussions and comments and two anonymous referees whose comments and suggestions improved the reading and quality of the manuscript.
Author information
Authors and Affiliations
Corresponding author
Additional information
Work partially supported by FIRB 2012 grant (project no. RBFR12URQJ) provided by the Italian Ministry of Education, Universities and Research.
Rights and permissions
About this article
Cite this article
Ignaccolo, R., Franco-Villoria, M. & Fassò, A. Modelling collocation uncertainty of 3D atmospheric profiles. Stoch Environ Res Risk Assess 29, 417–429 (2015). https://doi.org/10.1007/s00477-014-0890-7
Published:
Issue Date:
DOI: https://doi.org/10.1007/s00477-014-0890-7