Abstract
Objectives
Hippocampal radiomic features (HRFs) can serve as biomarkers in Alzheimer’s disease (AD). However, how different hippocampal segmentation methods affect HRFs in AD is still unknown. The aim of the study was to investigate how different segmentation methods affect HRF accuracy in AD analysis.
Methods
A total of 1650 subjects were identified from the Alzheimer’s Disease Neuroimaging Initiative database (ADNI). The mini-mental state examination (MMSE) and Alzheimer’s disease assessment scale (ADAS-cog13) were also adopted. After calculating the HRFs of intensity, shape, and textural features from each side of the hippocampus in structural magnetic resonance imaging (sMRI), the consistency of HRFs calculated from 7 different hippocampal segmentation methods was validated, and the performance of machine learning–based classification of AD vs. normal control (NC) adopting the different HRFs was also examined. Additional 571 subjects from the European DTI Study on Dementia database (EDSD) were to validate the consistency of results.
Results
Between different segmentations, HRFs showed a high measurement consistency (R > 0.7), a high significant consistency between NC, mild cognitive impairment (MCI), and AD (T-value plot, R > 0.8), and consistent significant correlations between HRFs and MMSE/ADAS-cog13 (p < 0.05). The best NC vs. AD classification was obtained when the hippocampus was sufficiently segmented by primitive majority voting (threshold = 0.2). High consistent results were reproduced from independent EDSD cohort.
Conclusions
HRFs exhibited high consistency across different hippocampal segmentation methods, and the best performance in AD classification was obtained when HRFs were extracted by the naïve majority voting method with a more sufficient segmentation and relatively low hippocampus segmentation accuracy.
Key Points
• The hippocampal radiomic features exhibited high measurement/statistical/clinical consistency across different hippocampal segmentation methods.
• The best performance in AD classification was obtained when hippocampal radiomics were extracted by the naïve majority voting method with a more sufficient segmentation and relatively low hippocampus segmentation accuracy.






Similar content being viewed by others
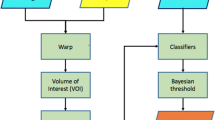
Abbreviations
- ACC:
-
Accuracy
- AD:
-
Alzheimer’s disease
- ADAS-cog13:
-
Alzheimer’s disease assessment scale
- ADNI:
-
The Alzheimer’s Disease Neuroimaging Initiative database
- ANTs:
-
The Advanced Normalization Tools
- AUC:
-
The area under the ROC curve
- EDSD:
-
The European DTI Study on Dementia database
- HRFs:
-
Hippocampal radiomic features
- ICC:
-
Intraclass correlation coefficient
- LLL:
-
Local label learning
- MAIS:
-
Multi-atlas image segmentation
- MCI:
-
Mild cognitive impairment
- ML:
-
Metric learning
- MMSE:
-
Mini-mental state examination
- MV:
-
Majority voting
- NC:
-
Normal control
- NLP:
-
Nonlocal patch
- RF:
-
Random forest
- RF-SSLP:
-
Random forest-semi-supervised label propagation
- RLBP:
-
Random local binary pattern
- ROC:
-
Receiver operating characteristic
- SEN:
-
Sensitivity
- SPE:
-
Specificity
- SVM:
-
Support vector machine
References
Handels RL, Wolfs CA, Aalten P, Joore MA, Verhey FR, Severens JL (2014) Diagnosing Alzheimer’s disease: a systematic review of economic evaluations. Alzheimers Dement 10:225–237
Jack CR Jr, Albert MS, Knopman DS et al (2011) Introduction to the recommendations from the National Institute on Aging-Alzheimer’s Association workgroups on diagnostic guidelines for Alzheimer’s disease. Alzheimers Dement 7:257–262
Querfurth HW, LaFerla FM (2010) Alzheimer’s disease. N Engl J Med 362:329–344
Petersen RC (2016) Mild cognitive impairment. Continuum (Minneap Minn) 22:404–418
Petersen RC, Roberts RO, Knopman DS et al (2009) Mild cognitive impairment: ten years later. Arch Neurol 66:1447–1455
Aerts HJ, Velazquez ER, Leijenaar RT et al (2014) Decoding tumour phenotype by noninvasive imaging using a quantitative radiomics approach. Nat Commun 5:4006
Lambin P, Rios-Velazquez E, Leijenaar R et al (2012) Radiomics: extracting more information from medical images using advanced feature analysis. Eur J Cancer 48:441–446
Chaddad A, Desrosiers C, Niazi T (2018) Deep radiomic analysis of MRI related to Alzheimer’s disease. IEEE Access 6:58213–58221
Sorensen L, Igel C, Liv Hansen N et al (2016) Early detection of Alzheimer’s disease using MRI hippocampal texture. Hum Brain Mapp 37:1148–1161
Zhao K, Ding Y, Han Y et al (2020) Independent and reproducible hippocampal radiomic biomarkers for multisite Alzheimer’s disease: diagnosis, longitudinal progress and biological basis. Sci Bull 65:1103–1113
Feng F, Wang P, Zhao K et al (2018) Radiomic features of hippocampal subregions in Alzheimer’s disease and amnestic mild cognitive impairment. Front Aging Neurosci 10:290
Rohlfing T, Brandt R, Menzel R, Maurer CR Jr (2004) Evaluation of atlas selection strategies for atlas-based image segmentation with application to confocal microscopy images of bee brains. Neuroimage 21:1428–1442
Coupe P, Manjon JV, Fonov V, Pruessner J, Robles M, Collins DL (2011) Patch-based segmentation using expert priors: application to hippocampus and ventricle segmentation. Neuroimage 54:940–954
Zhu H, Tang Z, Cheng H, Wu Y, Fan Y (2019) Multi-atlas label fusion with random local binary pattern features: application to hippocampus segmentation. Sci Rep 9:16839
Zhu H, Cheng H, Yang X, Fan Y, Alzheimer’s Disease Neuroimaging I (2017) Metric learning for multi-atlas based segmentation of hippocampus. Neuroinformatics 15:41–50
Hao Y, Wang T, Zhang X et al (2014) Local label learning (LLL) for subcortical structure segmentation: application to hippocampus segmentation. Hum Brain Mapp 35:2674–2697
Han X (2013) Learning-boosted label fusion for multi-atlas auto-segmentation. International Workshop on Machine Learning in Medical Imaging. Springer, pp 17–24
Zheng Q, Wu Y, Fan Y (2018) Integrating semi-supervised and supervised learning methods for label fusion in multi-atlas based image segmentation. Front Neuroinform 12:69
Mohs RC, Rosen WG, Davis KL (1983) The Alzheimer’s disease assessment scale: an instrument for assessing treatment efficacy. Psychopharmacol Bull 19:448–450
Jack CR Jr, Bernstein MA, Fox NC et al (2008) The Alzheimer’s Disease Neuroimaging Initiative (ADNI): MRI methods. J Magn Reson Imaging 27:685–691
Shrout PE, Fleiss JL (1979) Intraclass correlations: uses in assessing rater reliability. Psychol Bull 86:420
Zhao K, Zheng Q, Che T et al (2020) Regional radiomics similarity networks (R2SNs) in the human brain: reproducibility, small-world properties and a biological basis. Netw Neurosci:1–30
Kumar V, Gu Y, Basu S et al (2012) Radiomics: the process and the challenges. Magn Reson Imaging 30:1234–1248
Gillies RJ, Kinahan PE, Hricak H (2016) Radiomics: images are more than pictures, they are data. Radiology 278:563–577
Lambin P, Leijenaar RTH, Deist TM et al (2017) Radiomics: the bridge between medical imaging and personalized medicine. Nat Rev Clin Oncol 14:749–762
Coroller TP, Grossmann P, Hou Y et al (2015) CT-based radiomic signature predicts distant metastasis in lung adenocarcinoma. Radiother Oncol 114:345–350
Huang X, Cheng Z, Huang Y et al (2018) CT-based radiomics signature to discriminate high-grade from low-grade colorectal adenocarcinoma. Acad Radiol 25:1285–1297
Feng Q, Ding Z (2020) MRI radiomics classification and prediction in Alzheimer’s disease and mild cognitive impairment: a review. Curr Alzheimer Res 17:297–309
Freedman LP, Venugopalan G, Wisman R (2017) Reproducibility2020: progress and priorities. F1000Res 6:604
Velasco-Annis C, Akhondi-Asl A, Stamm A, Warfield SK (2018) Reproducibility of brain MRI segmentation algorithms: empirical comparison of Local MAP PSTAPLE, FreeSurfer, and FSL-FIRST. J Neuroimaging 28:162–172
Jin D, Wang P, Zalesky A et al (2020) Grab-AD: generalizability and reproducibility of altered brain activity and diagnostic classification in Alzheimer’s disease. Hum Brain Mapp 41:3379–3391
Jin D, Zhou B, Han Y et al (2020) Generalizable, reproducible, and neuroscientifically interpretable imaging biomarkers for Alzheimer’s disease. Adv Sci (Weinh) 7:2000675
Li H, Habes M, Wolk DA et al (2019) A deep learning model for early prediction of Alzheimer’s disease dementia based on hippocampal magnetic resonance imaging data. Alzheimers Dement 15:1059–1070
Rathore S, Habes M, Iftikhar MA, Shacklett A, Davatzikos C (2017) A review on neuroimaging-based classification studies and associated feature extraction methods for Alzheimer’s disease and its prodromal stages. Neuroimage 155:530–548
Beheshti I, Demirel H, Alzheimer’s Disease Neuroimaging I (2016) Feature-ranking-based Alzheimer’s disease classification from structural MRI. Magn Reson Imaging 34:252–263
Zheng Q, Wu Y, Fan Y (2018) Integrating semi-supervised and supervised learning methods for label fusion in multi-atlas based image segmentation. Front Neuroinf 12:69
Acknowledgements
Data collection and sharing for this project was funded by the Alzheimer’s Disease Neuroimaging Initiative (ADNI) (National Institutes of Health Grant U01 AG024904) and DOD ADNI (Department of Defense award number W81XWH-12-2-0012). The ADNI was funded by the National Institute on Aging, the National Institute of Biomedical Imaging and Bioengineering, and generous contributions from AbbVie, Alzheimer’s Association; Alzheimer’s Drug Discovery Foundation; Araclon Biotech; BioClinica, Inc.; Biogen; Bristol-Myers Squibb Company; CereSpir, Inc.; Cogstate; Eisai Inc.; Elan Pharmaceuticals, Inc.; Eli Lilly and Company; EuroImmun; F. Hoffmann-La Roche Ltd, and its affiliated company Genentech, Inc.; Fujirebio; GE Healthcare; IXICO Ltd.; Janssen Alzheimer Immunotherapy Research & Development, LLC.; Johnson & Johnson Pharmaceutical Research & Development LLC.; Lumosity; Lundbeck; Merck & Co., Inc.; Meso Scale Diagnostics, LLC.; NeuroRx Research; Neurotrack Technologies; Novartis Pharmaceuticals Corporation; Pfizer Inc.; Piramal Imaging; Servier; Takeda Pharmaceutical Company; and Transition Therapeutics. The Canadian Institutes of Health Research provided funds to support ADNI clinical sites in Canada. Private sector contributions were facilitated by the Foundation for the National Institutes of Health (www.fnih.org). The grantee organization was the Northern California Institute for Research and Education, and the study was coordinated by the Alzheimer’s Therapeutic Research Institute at the University of Southern California. ADNI data were disseminated by the Laboratory for Neuro Imaging at the University of Southern California.
Funding
This study has received funding by the National Natural Science Foundation of China (61802330, 61802331, 61801415).
Author information
Authors and Affiliations
Corresponding author
Ethics declarations
Guarantor
The scientific guarantor of this publication is Qiang Zheng from Yantai University, the lead author of the study.
Conflict of interest
The authors declare no competing interests.
Statistics and biometry
One of the authors (Minhui Ouyang, the last author, Children’s Hospital of Philadelphia) has significant statistical expertise.
Informed consent
Informed written consent was obtained from all subjects (patients) in this study.
Ethics approval
The study was approved by the institutional review boards of all the participating institutions.
Study subjects or cohorts overlap:
It should be noted that 990 of the 1650 subjects in ADNI cohort have been previously reported (Zhao et al, Jin et al). These prior studies focused on whether the hippocampal radiomics feature (Zhao et al) or 3D attention network model (Jin et al) can distinguish AD from NC, whereas the present study aims to test how segmentation methods affect hippocampal radiomic feature accuracy in Alzheimer’s disease analysis.
Zhao K, Ding Y, Han Y, et al Independent and reproducible hippocampal radiomic biomarkers for multisite Alzheimer’s disease: diagnosis, longitudinal progress and biological basis. Science Bulletin. 2020;65(13):1103-13.
Jin D, Wang P, Zalesky A, et al Grab-AD: Generalizability and reproducibility of altered brain activity and diagnostic classification in Alzheimer’s Disease. Hum Brain Mapp. 2020;41(12):3379-91.
Methodology
• retrospective
• case-control study
• multicenter study
Additional information
Publisher’s note
Springer Nature remains neutral with regard to jurisdictional claims in published maps and institutional affiliations.
Supplementary information
ESM 1
(PDF 3499 kb)
Rights and permissions
Springer Nature or its licensor holds exclusive rights to this article under a publishing agreement with the author(s) or other rightsholder(s); author self-archiving of the accepted manuscript version of this article is solely governed by the terms of such publishing agreement and applicable law.
About this article
Cite this article
Zheng, Q., Zhang, Y., Li, H. et al. How segmentation methods affect hippocampal radiomic feature accuracy in Alzheimer’s disease analysis?. Eur Radiol 32, 6965–6976 (2022). https://doi.org/10.1007/s00330-022-09081-y
Received:
Revised:
Accepted:
Published:
Issue Date:
DOI: https://doi.org/10.1007/s00330-022-09081-y