Abstract
Purpose
The objective of this study was to investigate whether computed tomography texture analysis can be used to differentiate papillary renal cell carcinoma (PRCC) subtypes.
Method
Sixty-two PRCC tumors were retrospectively evaluated, with 30 type 1 tumors and 32 type 2 tumors. Texture parameters quantified from three-phase contrast-enhanced CT images were compared with least absolute shrinkage and selection operator (LASSO) regression. Receiver operating characteristic (ROC) analysis was performed, and the area under the ROC curve (AUC) was calculated for each parameter. The selected texture parameters of each phase were used to generate support vector machine (SVM) classifiers. Decision curve analysis (DCA) of the classification was performed.
Results
The two texture parameters with the top two AUC values were – 333–7 Correlation (AUC = 0.772) and 45–7 Entropy (AUC = 0.753) in the corticomedullary phase, 333–4 Correlation (AUC = 0.832) and 45–7 Entropy (AUC = 0.841) in the nephrographic phase, and 135–7 Entropy (AUC = 0.858) and – 333–1 InformationMeasureCorr2 (AUC = 0.849) in the excretory phase. Entropy and Correlation have a high correlation with the two types of PRCC and are increased in type 2 PRCC. A model incorporating the texture parameters with the top two AUC values in each phase produced an AUC of 0.922 with an accuracy of 84% (sensitivity = 89% and specificity = 80%). The nephrographic-phase model and the model combining the texture parameters of the three phases can differentiate the two types with the largest net benefit.
Conclusions
Computed tomography texture analysis can be used to distinguish type 2 PRCC from type 1 with high accuracy, which may be clinically important.






Similar content being viewed by others
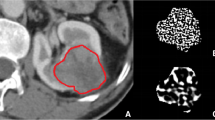
Abbreviations
- AUC:
-
Area under curve
- CT:
-
Computed tomography
- DCA:
-
Decision curve analysis
- ICC:
-
Intraclass correlation coefficient
- LASSO:
-
Least absolute shrinkage and selection operator
- MRI:
-
Magnetic resonance imaging
- PRCC:
-
Papillary renal cell carcinoma
- RCC:
-
Renal cell carcinoma
- ROC:
-
Receiver operating characteristic
- ROI:
-
Region of interest
- SVM:
-
Support vector machine
- TA:
-
Texture analysis
References
Moch H, Cubilla AL, Humphrey PA, Reuter VE, Ulbright TM (2016) The 2016 WHO classification of tumours of the urinary system and male genital organs-part a: renal, penile, and testicular tumours. Eur Urol 70:93–105
K. Sasaguri, N. Takahashi, CT and MR imaging for solid renal mass characterization, Eur. J. Radiol. 99 (2018) 40–54
Doshi AM, Ream JM, Kierans AS, et al. Use of MRI in differentiation of papillary renal cell carcinoma subtypes: qualitative and quantitative analysis. AJR 2016; 206:566–572
Egbert ND, Caoili EM, Cohan RH, et al. Differentiation of papillary renal cell carcinoma subtypes on CT and MRI. AJR 2013; 201:347–355
Rosenkrantz AB, Sekhar A, Genega EM, et al. Prognostic implications of the magnetic resonance imaging appearance in papillary renal cell carcinoma. Eur Radiol 2013; 23:579–587
Gurel S, Narra V, Elsayes KM, Siegel CL, Chen ZE, Brown JJ. Subtypes of renal cell carcinoma:MRI and pathological features. Diagn Interv Radiol 2013; 19:304–311
Vikram R, Ng CS, Tamboli P, et al. Papillary renal cell carcinoma: radiologic-pathologic correlation and spectrum of disease. Radio Graphics 2009; 29:741–754; discussion, 755–747
Yoshimitsu K, Kakihara D, Irie H, et al. Papillary renal carcinoma: diagnostic approach by chemical shift gradient-echo and echo-planar MR imaging.J Magn Reson Imaging 2006; 23:339–344
Beck SD, Patel MI, Snyder ME, et al. Effect of papillary and chromophobe cell type on disease free survival after nephrectomy for renal cell carcinoma. Ann Surg Oncol 2004; 11:71–77
Yamashita S, Ioritani N, Oikawa K, Aizawa M, Endoh M, Arai Y. Morphological subtyping of papillary renal cell carcinoma: clinicopathological characteristics and prognosis. Int J Urol 2007;14:679–683
Waldert M, Haitel A, Marberger M, et al. Comparison of type I and II papillary renal cell carcinoma (RCC) and clear cell RCC. BJU Int 2008;102:1381–1384
Yamada T, Endo M, Tsuboi M, et al. Differentiation of pathologic subtypes of papillary renal cell carcinoma on CT. AJR 2008; 191:1559–1563
Ng F, Kozarski R, Ganeshan B, Goh V. Assessment of tumor heterogeneity by CT texture analysis: can the largest cross-sectional area be used as an alternative to whole tumor analysis? Eur J Radiol 2013; 82:342–348
Miles KA, Ganeshan B, Hayball MP (2013) CT texture analysis using the filtration-histogram method: what do the measurements mean? Cancer Imaging 13:400–406
Ganeshan B,GohV, MandevilleHC,NgQS, HoskinPJ, Miles KA (2013) Non-small cell lung cancer: histopathologic correlates for texture parameters at CT. Radiology 266:326–336
Goh V, Ganeshan B, Nathan P, Juttla JK, Vinayan A, Miles KA (2011) Assessment of response to tyrosine kinase inhibitors in metastatic renal cell cancer: CT texture as a predictive biomarker. Radiology 261:165–171
Yu H, Scalera J, Khalid M et al (2017) Texture analysis as a radiomic marker for differentiating renal tumors. Abdom Radiol (NY)
Raman SP,Chen Y, Schroeder JL, Huang P, Fishman EK(2014)CT texture analysis of renal masses: pilot study using random forest classification for prediction of pathology. Acad Radiol 21:1587–1596
Zhang GM, Shi B, Xue HD,et al. Can quantitative CT texture analysis be used to differentiate subtypes of renal cell carcinoma? Clin Radiol. 2019 Apr;74(4):287-294.
Deng Y, Soule E , Samuel A,et al. CT texture analysis in the differentiation of major renal cell carcinoma subtypes and correlation with Fuhrman grade. Eur Radiol. 2019 Dec;29(12):6922-6929.
Vendrami CL, Velichko YS, Miller FH, et al. Differentiation of Papillary Renal Cell Carcinoma Subtypes on MRI: Qualitative and Texture Analysis. AJR Am J Roentgenol. 2018 Dec;211(6):1234-1245.
Kocak B, Yardimci AH, Bektas CT, et al. Textural differences between renal cell carcinoma subtypes: Machine learning-based quantitative computed tomography texture analysis with independent external validation. Eur J Radiol. 2018 Oct;107:149-157.
Low G, Huang G, Fu W, Moloo Z, Girgis S. Review of renal cell carcinoma and its common subtypes in radiology. World J Radiol 2016; 8:484–500
Yip C, Davnall F, Kozarski R,et al(2015)Assessment of changes in tumor heterogeneity following neoadjuvant chemotherapy in primary esophageal cancer. Dis Esophagus 28:172–179
Leng S, Takahashi N, Gomez Cardona D et al (2017) Subjective and objective heterogeneity scores for differentiating small renal masses using contrast-enhanced CT. Abdom Radiol (NY) 42: 1485–1492
Chen F, Huhdanpaa H, Desai B et al (2015) Whole lesion quantitative CT evaluation of renal cell carcinoma: differentiation of clear cell from papillary renal cell carcinoma. Springer plus 4:66
Lubner MG, Stabo N, Abel EJ, Del Rio AM, Pickhardt PJ (2016) CT textural analysis of large primary renal cell carcinomas: pretreatment tumor heterogeneity correlates with histologic findings and clinical outcomes. AJR Am J Roentgenol 207:96–105
Vickers A, Elkin E: Decision curve analysis: a novel method for evaluating prediction models. Med Decis Making 2006, 26(6):565-574.
Rousson V, Zumbrunn T. Decision curve analysis revisited: overall net benefit, relationships to ROC curve analysis, and application to case-control studies. BMC Med Inform Decis Mak. 2011 Jun 22; 11:45. https://doi.org/10.1186/1472-6947-11-45.
Author information
Authors and Affiliations
Corresponding author
Additional information
Publisher's Note
Springer Nature remains neutral with regard to jurisdictional claims in published maps and institutional affiliations.
Rights and permissions
About this article
Cite this article
Duan, C., Li, N., Niu, L. et al. CT texture analysis for the differentiation of papillary renal cell carcinoma subtypes. Abdom Radiol 45, 3860–3868 (2020). https://doi.org/10.1007/s00261-020-02588-2
Published:
Issue Date:
DOI: https://doi.org/10.1007/s00261-020-02588-2