Abstract
In this paper, an approach for developing the prediction model for polymer blends using a back-propagation neural network (BPNN) combined with the Taguchi quality method is presented in an attempt to improve the deficiencies in current neural networks associated with the design of network architecture, including the selection of one optimal set of learning parameters to accomplish faster convergence during training and the desired accuracy during the recall step. The objective of the prediction model is to explore the relationships between the control factor levels and surface roughness in the film coating process. In addition, the feasibility of adopting this approach is demonstrated in the study optimizing the learning parameters of the BPNN structure to forecast the target characteristics of the product or process with various control conditions in the manufacturing system.
Similar content being viewed by others
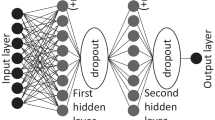
References
Thuenemann AF (2000) Nano-structure materials with low surface energies formed by polyelectrolytes and fluorinated amphiphiles (PEFA). Polym Int 49(7):636–644
Wong CP, Rao Y, Yue J (2002) Material characterization of high dielectric constant polymer-ceramic composite for embedded capacitor to RF application. Active Passive Electron Compon 25(1):123–129
Zhang J, Wilkie CA (2003) Preparation and flammability properties of polyethylene-clay nanocomposites. Polym Degradation Stability 80(1):163–169
Hansma PK, Cleveland JP, Radmacher M, Walters DA, Hillner PE, Bezanilla M, Fritz M, Vie D, Hansma HG (1994) Tapping mode atomic force microscopy in liquids. Appl Phys Lett 64(13):1738–1740
Chen YH, Tam SC, Chen WL, Zheng HY (1996) Application of Taguchi method in the optimization of laser micro-engraving of photomasks. Int J Mater Prod Technol 11(3/4):333–344
Masters I, Khoei AR, Gethin DT (1999) The application of Taguchi method to the aluminium recycling process. In: Proceedings of the 4th ASM international conference on the recycling metals, pp 115–124
Lin YC, Yan BH, Huang FY (2001) Surface improvement using a combination of electrical discgarge machining with ball burnish machining based on the Taguchi method. Int J Adv Manuf Technol 18(9):673–682
Lin ZC, Ho CY (2003) Analysis and application of grey relation and ANOVA in chemical-mechanical polishing process parameters. Int J Adv Manuf Technol 21(1):10–14
de la Maza M (1991) SPLIT net: dynamically adjusting the number of hidden units in a neural network. Artificial Neural Networks 647–651
Lirov Y (1992) Computer aided neural network engineering. Neural Networks 5:711–719
Khaw JFC, Lim BS, Lim LEN (1995) Optimal design of neural networks using the Taguchi method. Neurocomputing 7:225–245
Packianather MS, Drake PR, Rowlands H, et al. (2000) Optimizing the parameters of multilayered feedforward neural networks through Taguchi design of experiments. Qual Reliab Eng Int 16:461–473
Mayer RJ, Benjamin PC (1992) Using the Taguchi paradigm for manufacturing system design using simulation experiments. Comput Ind Eng 22(2):195–209
Phadke MS (1989) Quality engineering using robust design. Prentice-Hall, New York
Taguchi G (1989) Introduction to quality engineering. Asian Productivity Organization, Tokyo
Taguchi G, Yokohama Y, Wu Y (1993) Design of experiments. ASI Press, Tokyo, Japan
Taguchi G, Konishi S (1991) Signal-to-noise ratio for quality evaluation. ASI Press, Tokyo, Japan
Wasserman PD (1989) Neural computing: theory and practice. Van Nostrand Reinhold, New York
Aleksander I, Morton H (1990) An introduction to neural computing. Chapman and Hall, London
Author information
Authors and Affiliations
Corresponding author
Rights and permissions
About this article
Cite this article
Kuo, CF., Wu, YS. Application of a Taguchi-based neural network prediction design of the film coating process for polymer blends. Int J Adv Manuf Technol 27, 455–461 (2006). https://doi.org/10.1007/s00170-004-2215-3
Received:
Accepted:
Published:
Issue Date:
DOI: https://doi.org/10.1007/s00170-004-2215-3