Abstract
This study provides evidence supporting the use of the update strategies for the support vector regression (SVR) model. Firstly, the fitting and interpolation method (FIM) is presented to select SVR parameters, and three infill strategies are adopted to search for update points. Secondly, the infill strategy and parameter selection method are illustrated by test functions that illustrate their dependability. The distribution of update points, the sample density and the proportion of update points are discussed. Finally, the adaptive SVR surrogate model is applied to optimize the protective effect of railway wind barriers. The result shows that the parameter selection method has high stability. On the whole, the accuracy of the adaptive SVR model using a suitable infill strategy will be improved with an increasing proportion of update points if the final number of training points is identical. The optimization result shows an optimal porosity of 0.117 when the height of the railway wind barrier is 2.05 m (full scale).











Similar content being viewed by others
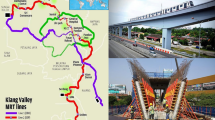
References
Aich U, Banerjee S (2014) Modeling of EDM responses by support vector machine regression with parameters selected by particle swarm optimization. Appl Math Model 38:2800–2818
Basudhar A (2012) Selection of anisotropic kernel parameters using multiple surrogate information. 12th AIAA Aviation Technology, Integration, and Operations (ATIO) Conference and 14th AIAA/ISSM, Indianapolis, Indiana
Ben-Israel A, Greville T (2003) Generalized inverses, 2nd edn. Springer, New York
Bourdin P, Wilson JD (2008) Windbreak aerodynamics: is computational fluid dynamics reliable? Bound-Layer Meteorol 126(2):181–208
Chapelle O, Vapnik V, Bousquet O, Mukherjee S (2002) Choosing multiple parameters for support vector machines. Mach Learn 46(1):131–159
Charuvisit S, Kimura K, Fujino Y (2004) Effects of wind barrier on a vehicle passing in the wake of a bridge tower in cross wind and its response. J Wind Eng Ind Aerodyn 92(7–8):609–639
Chu C, Chang C, Huang C et al (2013) Windbreak protection for road vehicles against crosswind. J Wind Eng Ind Aerodyn 116:61–69
Coleman SA, Baker CJ (1992) Reduction of accident risk for high sided road vehicles in cross winds. J Wind Eng Ind Aerodyn 44–44:2685–2695
Cristianini N, Shawe-Taylor J (2000) An introduction to support vector machines and other kernel-based learning methods. Cambridge University Press, Cambridge
Dixon L and Szegö G (1978) Towards global optimization 2. North Holland, Amsterdam, the Netherlands
Dong ZB, Luo WY, Qian GQ et al (2007) A wind tunnel simulation of the mean velocity fields behind upright porous fences. Agric For Meteorol 146(1–2):82–93
Fan HY, Dulikravich GS, Han ZX (2005) Aerodynamic data modeling using support vector machines. Inverse Prob Sci Eng 13(3):261–278
Forrester A, Keane A (2009) Recent advances in surrogate-based optimization. Prog Aerosp Sci 45(1):50–79
Forrester A, Sóbester A and Keane A (2008) Engineering design via surrogate modelling: a practical guide. A John Wiley and Sons, Ltd
Goel T, Haftka R, Shyy W, Queipo N (2007) Ensemble of surrogates. Struct Multidiscip Optim 33:199–216
Green DW and Perry RH (2008) Perry’s chemical engineers handbook (8th edn.). McGraw-Hill
Gunn SR (1998) Support vector machines for classification and regression. Image speech Intelligent Systems Group
Guo WW, Wang YJ, Xia H, Lu S (2015) Wind tunnel test on aerodynamic effect of wind barriers on train-bridge system. Sci China Technol Sci 58(2):219–225
He XH, Zhou YF, Wang HF, Han Y, Shi K (2014) Aerodynamic characteristics of a trailing rail vehicles on viaduct based on still wind tunnel experiments. J Wind Eng Ind Aerodyn 135:22–33
Hsu CW, Chang CC and Lin CJ (2003) A practical guide to support vector classification. Technical report, University of National Taiwan, Department of Computer Science and Information Engineering, 1–12
Huang CL, Wang CJ (2006) A GA-based feature selection and parameters optimization for support vector machines. Expert Syst Appl 31:231–240
Jie HX, Wu YZ, Ding JW (2014) An adaptive metamodel-based global optimization algorithm for black-box type problems. Eng Optim 46:1–24
Jones D (2001) A taxonomy of global optimization methods based on response surfaces. J Glob Optim 21:345–383
Jones D, Schonlau M, Welch W (1998) Efficient global optimization of expensive black-box functions. J Glob Optim 13:455–492
Keerthi S, Lin C (2003) Asymptotic behaviors of support vector machines with Gaussian Kernel. Neural Comput 15(7):1667–1689
Kleijnen J (2009) Kriging metamodeling in simulation: a review. Eur J Oper Res 192:707–716
Kozmar H, Procino L, Borsani L, Bartoli G (2012) Sheltering efficiency of wind barriers on bridges. J Wind Eng Ind Aerodyn 107–108:274–284
Kwon SD, Kim DH, Lee SH et al (2011) Design criteria of wind barriers for traffic. Part 1: wind barrier performance. Wind Struct 14(1):55–70
Lee SJ, Park KC, Park CW (2002) Wind tunnel observations about the shelter effect of porous fences on the sand particle movements. Atmos Environ 36:1453–1463
Lin SW, Ying KC, Chen SC (2008) Particle swarm optimization for parameter determination and feature selection of support vector machines. Expert Syst Appl 35:1817–1824
Liu YL, Chen WL, Ding LP et al (2013) Response surface methodology based on support vector regression for polygon blank shape optimization design. Int J Adv Manuf Technol 66:1391–1405
McKay M, Conover W, Beckman R (1979) A comparison of three methods for selecting values of input variables in the analysis of output from a computer code. Technometrics 21(2):239–245
Mullur A, Messac A (2005) Extended radial basis functions: more flexible and effective metamodeling. AIAA J 43(6):1306–1315
Nakayama H, Arakawa M, Washino K (2003) Using support vector machines in optimization for black-box objective functions. IEEE Proc Int Joint Conf 2:1617–1622
Pan F, Zhu P, Zhang Y (2010) Metamodel-based lightweight design of B-pillar with TWB structure via support vector regression. Comput Struct 88:36–44
Richard B, Cremona C, Adelaide L (2012) A response surface method based on support vector machines trained with an adaptive experimental design. Struct Saf 39:14–21
Richards PJ, Norris SE (2011) Appropriate boundary conditions for computational wind engineering models revisited. J Wind Eng Ind Aerodyn 99(4):257–266
Sack J, Welch WJ, Mitchell TJ et al (1989) Design and analysis of computer experiments (with discussion). Stat Sci 4:409–435
Smola AJ (1998) Learning with kernels. PhD Thesis, Technische Unverisity Berlin
Smola AJ, Schölkopf B (2004) A tutorial on support vector regression. Stat Comput 14:199–222
Sóbester A, Leary S, Keane A (2005) On the design of optimization strategies based on global response surface approximation models. J Glob Optim 33:31–59
Song H, Choi K, Lee I et al (2013) Adaptive virtual support vector machine for reliability analysis of high-dimensional problems. Struct Multidiscip Optim 47:479–491
Tan XH, Bi WH, Hou XL et al (2011) Reliability analysis using radial basis function networks and support vector machines. Comput Geotech 2011(38):178–186
Vapnik V, Golowich S, Smola A (1997) Support method for prediction approximation regression estimation, and signal processing. Advance in neural information processing system 9. MIT Press, Cambridge, MA
Wan XT, Pekny J, Reklaitis G (2005) Simulation-based optimization with surrogate models-application to supply chain management. Comput Chem Eng 29:1317–1328
Wang C, Duan QY, Gong W, Ye AZ, Di ZH, Miao CY (2014) An evaluation of adaptive surrogate modeling based optimization with two benchmark problems. Environ Model Softw 60:167–179
Wu K, Wang S (2009) Choosing the kernel parameters for support vector machines by the inter-cluster distance in the feature space. Pattern Recogn 42:710–717
Xiang HY, Li YL, Chen B et al (2014) Protection effect of railway wind barrier on running safety of train under cross winds. Adv Struct Eng 17(8):1176–1187
Xiang HY, Li YL, Wang B, Liao HL (2015) Numerical simulation of the protective effect of railway wind barriers under crosswinds. Int J Rail Transp 3(3):151–163
Zhang J, Chouwdhury S, Messac A (2012) An adaptive hybrid surrogate model. Struct Multidiscip Optim 46:233–238
Zhu P, Pan F, Chen W et al (2012) Use of support vector regression in structural optimization: application to vehicle crashworthiness design. Math Comput Simul 86:21–31
Acknowledgements
The writers are grateful for the financial support from the National Natural Science Foundation of China (51408503, U1334201, 51525804), the Fundamental Research Funds for the Central Universities (2682014BR049) and the Sichuan Province Youth Science and Technology Innovation Team (15CXTD0004).
Author information
Authors and Affiliations
Corresponding author
Rights and permissions
About this article
Cite this article
Xiang, H., Li, Y., Liao, H. et al. An adaptive surrogate model based on support vector regression and its application to the optimization of railway wind barriers. Struct Multidisc Optim 55, 701–713 (2017). https://doi.org/10.1007/s00158-016-1528-9
Received:
Revised:
Accepted:
Published:
Issue Date:
DOI: https://doi.org/10.1007/s00158-016-1528-9