Abstract
In this paper, we propose a new filtering algorithm, the variational Bayesian-based adaptive maximum correntropy generalized high-degree cubature Kalman filter, which is designed to improve filtering accuracy under conditions of unknown measurement noise covariance and measurement outliers. Considering that the generalized high-degree cubature rule can solve the high dimensional nonlinear problem well, based on the generalized high-degree cubature Kalman filter, the variational Bayesian method is utilized to approximate the measurement noise covariance, and the maximum correntropy criterion is used to reduce the influence of the measurement outliers on the state estimation. Additionally, we introduce a resistance factor to correct measurement values and optimize the kernel bandwidth for different types of noise. Simulation experiments on target tracking and integrated navigation demonstrate that our proposed algorithm effectively suppresses unknown time-varying noise and non-Gaussian mutation noise, outperforming existing filtering algorithms in terms of estimation accuracy, robustness, and adaptability.











Similar content being viewed by others
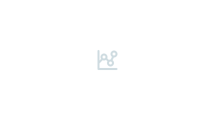
Data Availability
Data will be made available on reasonable request.
References
F. Albu, L. T. T. Tran, S. Nordholm, The hybrid simplified Kalman filter for adaptive feedback cancellation, in 2018 International Conference on Communications (COMM), Bucharest, Romania (2018), pp. 45–50. https://doi.org/10.1109/ICComm.2018.8484823
M. Ali, C.W. Ahn, M. Pant et al., An image watermarking scheme in wavelet domain with optimized compensation of singular value decomposition via artificial bee colony. Inf. Sci. 301, 44–60 (2016). https://doi.org/10.1016/j.ins.2014.12.042
I. Arasaratnam, S. Haykin, Cubature Kalman filters. IEEE Trans. Autom. Control 6(54), 1254–1269 (2009). https://doi.org/10.1109/TAC.2009.2019800
A. Assa, F. Janabi-Sharifi, A Kalman filter-based framework for enhanced sensor fusion. IEEE Sens. J. 6(15), 3281–3292 (2015). https://doi.org/10.1109/JSEN.2014.2388153
J. Bin, X. Ming, C.Y. Cheng, High-degree cubature Kalman filters. Automatica 4(49), 510–518 (2009). https://doi.org/10.1016/j.automatica.2012.11.014
G.T. Cinar, J.C. Príncipe, Hidden state estimation using the correntropy filter with fixed point update and adaptive kernel size, in Proceedings of 2012 IEEE International Joint Conference on Neural Networks, IJCNN, July. (2012), pp. 1–6. https://doi.org/10.1109/IJCNN.2012.6252730
N. Davari, A. Gholami, An asynchronous adaptive direct Kalman filter algorithm to improve underwater navigation system performance. IEEE Sens. J. 4(17), 1061–1068 (2016). https://doi.org/10.1109/JSEN.2016.2637402
P. Dong, Z. Jing, H. Leung, K. Shen, Variational Bayesian adaptive cubature information filter based on Wishart distribution. IEEE Trans. Autom. Control 62(11), 6051–6057 (2017). https://doi.org/10.1109/TAC.2017.2704442
S. Fakoorian, R. Izanloo, A. Shamshirgaran, D. Simon, Maximum correntropy criterion Kalman filter with adaptive kernel size, in 2019 IEEE National Aerospace and Electronics Conference, NAECON, Dayton, OH, USA (2019), pp. 581–584. https://doi.org/10.1109/NAECON46414.2019.9057886
Y. Guo, B. Xu, L. Wang, A robust SINS/USBL integrated navigation algorithm based on earth frame and right group error definition. IEEE Trans. Instrum. Meas. 71, 1–16 (2022). https://doi.org/10.1109/TIM.2022.3196425
Y. Hao, A. Xu, X. Sui, Y. Wang, A modified extended kalman filter for a two-antenna GPS/INS vehicular navigation system. Sensors 18(11), 3809 (2018). https://doi.org/10.3390/s18113809
J. He, C. Sun, B. Zhang, P. Wang, Maximum correntropy square-root cubature Kaman filter for non-gaussian measurement noise. IEEE Access 8, 70162–70170 (2020). https://doi.org/10.1109/ACCESS.2020.2986022
B. Hou, Z. He, X. Zhou et al., Maximum correntropy criterion Kalman filter for-Jerk tracking model with non-Gaussian noise. Entropy 12(19), 648 (2017). https://doi.org/10.3390/e19120648
P.J. Huber, Robust estimation of a location parameter, in Breakthroughs in Statistics: Methodology and Distribution (1992), pp. 492–518. https://doi.org/10.1007/978-1-4612-4380-9_35
R. Izanloo, S. A. Fakoorian, H. S. Yazdi, D. Simon, Kalman filtering based on the maximum correntropy criterion in the presence of non-Gaussian noise, in Proceedings of 50th Annual Conference on Information Sciences and Systems (Princeton, USA, 2016), pp. 500–505. https://doi.org/10.1109/CISS.2016.7460553
J. Lan, X.R. Li, Multiple conversions of measurements for nonlinear estimation. IEEE Trans. Signal Process. 65(18), 4956–4970 (2017). https://doi.org/10.1109/TSP.2017.2716901
Y. Li, Y. Wang, F. Albu et al., A general zero attraction proportionate normalized maximum correntropy criterion algorithm for sparse system identification. Symmetry 9(10), 229–232 (2017). https://doi.org/10.3390/sym9100229
D. Liu, X. Chen, Y. Xu et al., Maximum correntropy generalized high-degree cubature Kalman filter with application to the attitude determination system of missile. Aerosp. Sci. Technol. 95, 105–114 (2019). https://doi.org/10.1016/j.ast.2019.105441
H. Ren, R. Lu, J. Xiong, Y. Wu, P. Shi, Optimal filtered and smoothed estimators for discrete-time linear systems with multiple packet dropouts under Markovian communication constraints. IEEE Trans. Cybern. 50(9), 4169–4181 (2020). https://doi.org/10.1109/TCYB.2019.2924485
H. Ren, R. Lu, J. Xiong, Y. Xu, Optimal estimation for discrete-time linear system with communication constraints and measurement quantization. IEEE Trans. Syst. Man Cybern. Syst. 50(5), 1932–1942 (2020). https://doi.org/10.1109/TSMC.2018.2792009
S. Särkkä, J. Hartikainen, Non-linear noise adaptive Kalman filtering via variational Bayes, in Proceedings of 2013 IEEE International Workshop on Machine Learning for Signal Processing (Southampton, UK, 2013), pp. 1–6. https://doi.org/10.1109/MLSP.2013.6661935
B. Wang, Q. Ren, Z. Deng et al., A self-calibration method for northogonal angles between gimbals of rotational inertial navigation system. IEEE Trans. Ind. Electron. 4(62), 2353–2362 (2014). https://doi.org/10.1109/TIE.2014.2361671
G. Wang, N. Li, Y. Zhang, Maximum correntropy unscented Kalman and information filters for non-Gaussian measurement noise. J. Frankl. Inst. 354(18), 8659–8677 (2017). https://doi.org/10.1016/j.jfranklin.2017.10.023
G. Wang, Z. Gao, Y. Zhang, B. Ma, Adaptive maximum correntropy Gaussian filter based on variational Bayes. Sensors 18(6), 1960 (2018). https://doi.org/10.3390/s18061960
S. Wang, J. Feng, K.T. Chi, Spherical simplex-radial cubature Kalman filter. IEEE Signal Process. Lett. 1(21), 43–46 (2013). https://doi.org/10.1109/LSP.2013.2290381
X.C. Zhang, Cubature information filters using high-degree and embedded cubature rules. Circuits System Signal Process 3(33), 1799–1818 (2014)
Funding
This research was supported by funds from the National Natural Science Foundation of China under Grant Numbers 41906005, 41705081, National Key Research and Development Project of China under Grant Numbers 2017YFB0202701, and National Basic Research Program of China under Grant Number 2019-JCJQ-ZD-149-00.
Author information
Authors and Affiliations
Corresponding author
Ethics declarations
Conflict of interest
The authors declare no conflict of interest.
Additional information
Publisher's Note
Springer Nature remains neutral with regard to jurisdictional claims in published maps and institutional affiliations.
Rights and permissions
Springer Nature or its licensor (e.g. a society or other partner) holds exclusive rights to this article under a publishing agreement with the author(s) or other rightsholder(s); author self-archiving of the accepted manuscript version of this article is solely governed by the terms of such publishing agreement and applicable law.
About this article
Cite this article
Liu, B., Zhang, X., Jia, S. et al. Variational Bayesian-Based Adaptive Maximum Correntropy Generalized High-Degree Cubature Kalman Filter. Circuits Syst Signal Process 42, 7073–7098 (2023). https://doi.org/10.1007/s00034-023-02436-w
Received:
Revised:
Accepted:
Published:
Issue Date:
DOI: https://doi.org/10.1007/s00034-023-02436-w