Abstract
Background
Interleukin-10 (IL-10) is an anti-inflammatory cytokine that affects different immune cells. It is also associated with the stimulation of the T and B cells for the production of antibodies. Several genetic polymorphisms in the IL-10 gene have been reported to cause or aggravate certain diseases like inflammatory bowel disease, rheumatoid arthritis, systemic sclerosis, asthma, etc. However, the disease susceptibility and abnormal function of the mutated IL-10 variants remain obscure.
Results
In this study, we used seven bioinformatics tools (SIFT, PROVEAN, PMut, PANTHER, PolyPhen-2, PHD-SNP, and SNPs&GO) to predict the disease susceptible non-synonymous SNPs (nsSNPs) of IL-10. Nine nsSNPs of IL-10 were predicted to be potentially deleterious: R42G, R45Q, F48L, E72G, M95T, A98D, R125S, Y155C, and I168T. Except two, all of the putative deleterious mutations are found in the highly conserved region of IL-10 protein structure, thus affecting the protein's stability. The 3-D structure of mutant proteins was modeled by project HOPE, and the protein–protein interactions were assessed with STRING. The predicted nsSNPs: R42Q, R45Q, F48L, E72G, and I168T are situated in the binding site region of the IL-10R1 receptor. Disruption of binding affinity with its receptor leads to deregulation of the JAK-STAT pathway and results in enhanced inflammation that imbalance in cellular signaling. Finally, Kaplan–Meier Plotter analysis displayed that deregulation of IL-10 expression affects gastric and ovarian cancer patients' survival rate. Thus, IL-10 could be useful as a potential prognostic marker gene for some cancers.
Conclusion
This study has determined the deleterious nsSNPs of IL-10 that might contribute to the malfunction of IL-10 protein and ultimately lead to the IL-10 associated diseases.
Similar content being viewed by others
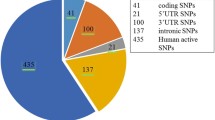
Background
The immune system is constituted of various immune cells, which are responsible for monitoring and getting rid of unfamiliar agents or invading microorganisms. The immune cells can act directly by themselves or by synthesizing molecules capable of inducing B cells, NK cells, T cells, and other immune cells [1, 2]. Activation and differentiation of the immune cells are largely dependent on different types of interleukins (ILs). Interleukins are the subsets of a large group of naturally occurring cytokines that are primarily released from specific immune cells in response to endotoxic threat, stress, heat, or inflammation. They act as cellular messengers by binding to high-affinity receptors on the cell surface [3, 4]. ILs play essential roles in innate and adaptive immune systems and modulate cell behavior [4, 5]. Interleukin 10 (IL-10) is one of the most crucial anti-inflammatory cytokines with the most diverse immune cells' effects [6]. It is produced by activated immune cells, particularly monocytes /macrophages and T cell subsets, including Tr1, Treg, and Th1 cells. IL-10 can down-regulate the expression of pathogenic Th17 cytokines, MHC class II antigens, and co-stimulatory molecules on macrophages [4, 7]. In addition to the immune suppression role, the IL-10 can also play an immune stimulatory role for B and T cells [6]. This dimeric cytokine's pleiotropic activities are mediated by its interaction with the tetrameric cell surface receptor complex, consisting of two IL-10R1 and two IL-10R2 [8, 9]. The receptor complex assembles sequentially: first, IL-10 binds with high-affinity IL-10R1 cell surface receptor and form IL-10/IL-10R1 complex [10, 11]. This IL-10/IL-10R1 intermediate complex is subsequently recognized through the low-affinity IL-10R2 receptor, resulting in an active signaling complex that induces the intracellular JAK-STAT pathway [12]. Recent studies showed that immune-related genes like IL-10 are highly polymorphic and associated with various types of diseases [13,14,15]. The most frequently occurred polymorphism is single nucleotide polymorphism (SNP) and can be identified once in every 100–300 base pairs of the human genome. It has been estimated that nearly 10 million SNPs are present in the human genome where 0.5 million SNPs are located in the coding region of different genes [16,17,18,19]. A recent report on Trans Omics for Precision Medicine (TOPMed) program suggested an average of 3.78 million genetic variants are present in each genome. Among the all genetic variants, a total of 23,916 variants or SNPs are coding variation [20]. The SNPs that may alter amino acid residue in the protein sequence are known as Non-synonymous SNPs (nsSNPs). They are particularly important as they may affect the protein function by destabilizing protein structure or altering its physicochemical properties [21]. Non-coding SNPs are also important as they may influence mRNA splicing, binding of the transcription factor to cis-regulatory elements, differential expression of genes, degradation of mRNA, and alternation in the sequences of noncoding RNAs [22]. In the recent era, hundreds and thousands of SNPs were associated with hundreds of disease studies [23, 24]. In various studies, several polymorphisms are identified in the coding and noncoding regions of IL-10. Some of the SNPs of IL-10 have already been characterized and found to significantly influence the immune response toward pathogenic challenges and disease outcome [25]. These polymorphisms cause functional changes of the IL-10 protein that are associated with various inflammatory and autoimmune diseases, such as inflammatory bowel disease: Crohn’s disease and ulcerative colitis, chronic hepatitis B and C, allergy and autoimmunity [25,26,27]. Blockage of IL-10 signaling may lead to enhanced inflammation and an increased number of Tregs (Regulatory T-cells) and MDSCs (Myeloid Derived Suppressor Cells), which inhibit tumor immunity, allowing tumors to grow [28]. Individuals with Chronic inflammatory bowel disease are predisposed to colon cancer, and individuals with chronic hepatitis are more prone to develop hepatocellular carcinoma [29, 30]. Considering the importance of IL-10 in multiple diseases, our present study investigates the disease-causing nsSNPs in the IL-10 gene and determining their deleterious effects on the protein. High-risk deleterious SNPs were further analyzed computationally to predict their structural and functional impact on IL-10 protein, which provides new insights for further genetic association studies.
Materials and methods
Retrieval of IL-10 nsSNPs (dataset)
The entire reported SNPs of the IL-10 gene and its protein sequence (Uniprot ID P22301) were retrieved from NCBI dbSNP (http://www.ncbi.nlm.nih.gov/snp) and Uniprot Knowledgebase database (http://www.uniprot.org/), respectively. A total of 1800 SNPs of different functional classes (Fig. 1) were mapped in IL-10 gene sequence. Out of 1800 SNPs, 91 are non-synonymous SNPs (nsSNPs) found in the coding region that may lead to missense or nonsense mutations, subsequently affecting the protein's structure and function. Our investigation accounted for the nsSNPs in the coding region of IL-10 Protein.
Prediction of the deleterious nsSNPs
We employed seven different tools to predict the deleterious effects of nsSNPs: SIFT-Sorting Intolerant From Tolerant (SIFT; http://sift.bii.a-star.edu.sg/) [31], Protein Variation Effect Analyzer (PROVEAN; http://provean.jcvi.org/index.php) [32] Predictor of human Deleterious Single Nucleotide Polymorphisms (PhD-SNP; http://snps.biofold.org/phd-snp/phd-snp.html) [33] PMut (http://mmb.irbbarcelona.org/PMut), Polymorphism Phenotyping v2 (PolyPhen-2; http://genetics.bwh.harvard.edu/pph/) [34], Protein analysis through evolutionary relationship (PANTHER; http://www.pantherdb.org/tools/csnpScoreForm.jsp) [35] and SNPs&GO (http://snps.biofold.org/snps-and-go/snps-and-go.html) [31,32,33,34,35,36]. SIFT predicts an amino acid substitution's effects on the function of a protein based on sequence homology and the substituted amino acids' physical characteristics [37]. PolyPhen-2 tool predicts the effects of substituted amino acids on structure and function of a protein based on physical and comparative properties [34]. PROVEAN is a support vector machine-based (SVM) server, which predicts whether a substituted amino acid has an impact on the function of a given protein or not. The tools like PANTHER, SNPs&GO, PHD- SNP, and PMut were used for the prediction of whether a single nucleotide polymorphism is likely to be involved in the insurgence of diseases using functional annotation of protein [35, 38,39,40]. The nsSNPs predicted deleterious by at least six of the above mentioned in silico tools were considered as high risk nsSNPs and selected for the further analysis.
Analyzing protein stability due to mutations
I-Mutant 3.0 (http://gpcr2.biocomp.unibo.it/cgi/predictors/I-Mutant3.0/I-Mutant3.0.cgi), Mupro (http://mupro.proteomics.ics.uci.edu) and INPS-MD (http://inpsmd.biocomp.unibo.it) tools were used to evaluate the stability changes of IL-10 protein upon point mutations. I-Mutant 3.0 is a support vector machine (SVM) based predictor that determines the degree of protein destabilization and measures the ΔΔG value (kcal/mol). The ΔΔG (delta delta G) value is the difference between Gibbs free energy values of mutated protein from the Gibbs free energy value of wild type protein. A ΔΔG value less than ‘0’ indicates that the variants cause the decreased stability of the protein whether ΔΔG value greater than ‘0’ means increased stability of that protein [41].
On the other hand, MUpro uses a large number of mutation datasets which actually based on both SVM and neural networks machine learning methods. The other third tools INPS-MD (Impact of Non-synonymous mutations on Protein Stability-Multi Dimension) based on sequence descriptor that uses Support Vector Regression (SVR) to calculate ΔΔG value. Both the MUpro and INPS-MD measure the ΔΔG for estimation of protein stability and the cut-off value of ΔΔG is also same to I-Mutant 3.0 [42, 43].
The IL-10 protein sequence along with wild type and substitute amino acids at their corresponding position was used as input in the aforementioned tools to predict the mutational effect on protein stability [41].
Identification of mutational impacts on structural and functional properties of proteins
For sorting out disease associated or neutral amino acid substitutions in protein sequence, the commonly predicted mutations were further examined by MutPred2 web server (http://mutpred.mutdb.org). It is a machine learning based tool that integrates genetic and molecular data for prediction of the pathogenicity of substituted amino acid. It also predicts molecular cause of the disease [44].
Conservation profile of high-risk nsSNPs
nsSNPs, which are positioned at highly conserved regions tend to be more deleterious than the nsSNPs those are situated at non-conserved sites. By using empirical Bayesian inference, ConSurf web server predicts putative structural and functional amino acid residues and estimates evolutionary conservation based on the phylogenetic relations between homologous sequences [45]. To further investigate the potential effects of the high-risk nsSNPs, we calculated the degree of evolutionary conservation at all amino acid sites in the IL-10 protein using the ConSurf web server.
Predicting the molecular effects of high risk nsSNPs on protein structure
Project HOPE is an automatic mutant analysis server that helps to analyze the structural and biochemical effects of a point mutation in a protein sequence [46]. We submitted the nine SNPs (rs ID) with the primary structure of IL-10 protein from Protein Data Bank (http://www.rcsb.org/pdb/) into HOPE. HOPE predicts the 3D structure of the mutated protein by collecting structural information from a series of sources and gives the explanation of such a change (in both structure and function of the protein).
Protein–protein interaction prediction
Protein–protein interactions are studied to unveil and annotate all functional interactions among cell proteins. The online database STRING (STRING; http://string-db.org/) was used to predict protein- protein interactions [47].
Kaplan–Meier plotter analysis
Kaplan–Meier plotter database (http://kmplot.com/analysis) uses Gene Expression Omnibus (GEO), European Genome Phenome Atlas (EGA), and the Cancer Genome Atlas (TCGA) datasets for the relapse free and overall survival (OS) information that offers meta-analysis-based discovery and biomarker assessment in cancer patients. The aim of this analysis is to estimate the time to death, an event will eventually occur in everyone that may have significant implications when using these estimates to inform clinical decisions, health care policies, and resource allocation [48]. In this algorithm, the potential effects of 54,675 genes (mRNA, miRNA, protein) can be examined on survival of 13,316 cancer patients (comprising 6234 breast, 3452 lungs, 1,440 gastric, and 2190 ovarian cancer) through microarray gene expression data of 21 types of cancers [49]. By using 207,433 at Affymetrix ID of IL-10 gene, the overall survival analysis was performed on total 13,316 cancers patients. The hazard ratio (HR) with 95% confidence intervals and log rank P-value were enumerated and displayed on the plot.
Results
nsSNPs retrieved from dbSNP database
In the dbSNP database showed the human IL-10 gene consists a total of 1800 SNPs, of which 91 were nsSNPs/missense leading to amino acid substitution (5%), 1336 were intronic SNPs (74%), 48 were synonymous SNPs (3%), and the rests were of other types (Fig. 1). We selected only the nsSNPs for our investigation (Additional file 1: Table S1).
Prediction and analysis of deleterious nsSNPs
The functional impact of nsSNPs was assessed by evaluating the importance of amino acids they alter. A dataset of a total of 91 polymorphic inputs was used for analysis. Structural and functional effects of deleterious SNPs on the IL-10 protein were screened by various computational tools. A graphical representation of the deleterious nsSNPs predicted by seven different computational tools is illustrated in Fig. 2.
First, 91 nsSNPs of IL-10 were submitted to SIFT algorithm. According to SIFT result, out of 91 nsSNPs, 39 nsSNPs were predicted intolerant whose TI scoring was ≤ 0.05. 11 SNPs showed a highly deleterious effect with a tolerant index (TI) score of 0.00; 13 SNPs showed 0.01 TI score, and the remaining 15 SNPs TI score was 0.02–0.04 (Additional file 1: Table S2).
Among the other tools, PROVEAN predicted 23 nsSNPs (out of 91 nsSNPs) as “Deleterious”, similarly, PhD-SNP and PMut tools proposed 28 and 29 nsSNPs, respectively, as “Disease” (Additional file 1: Tables S3, S4, and S5). Additionally, PolyPhen-2 predicted 13 nsSNPs as “Possibly damaging” and 42 nsSNPs as “Probably damaging” (Additional file 1: Table S6). Moreover, PANTHER_PSEP foretold 41 SNPs as “Deleterious”. Among them, 28 SNPs were identified as possibly damaging, and the remaining 13 SNPs were identified as “Probably damaging” (Additional file 1: Table S7). In SNPs&GO tools, five nsSNPs were predicted to be associated with various types of diseases, and the rest of the 86 SNPs were not predicted to have any effects (Additional file 1: Table S8). Finally, mutations predicted deleterious/ damaging /disease-related effects by at least six of the analyzed in silico tools were considered for further investigation (Table 1).
Computational analysis by the seven mentioned tools exhibited nine highly damaging nsSNPs in the IL-10 gene. Out of nine nsSNPs, three of them (e.g., rs550164520 R45Q, rs1421978042 A98D, and rs1022828778 Y155C) were predicted deleterious unanimously by all the employed tools, and other six nsSNPs (rs1274280163 R42G, rs745923816 F48L, rs545228684 E72G, rs1354773439 M95T, and rs1310781150 R125S) were predicted deleterious by the at least six computational tools. Except for the R42G, the remaining eight of them are novel SNPs.
Identification of functional and structural modifications of IL-10 predicted by MutPred2
The shortlisted nine nsSNPs predicted as deleterious from the previous steps were submitted to the MutPred2 web server. The resulting probability scores, g-value, and p-value are shown in Table 2. It helps to predict the reason for molecular alternations potentially affecting the phenotype. The structural and functional alterations predicted include- loss of sulfation, acetylation, allosteric site; alerted transmembrane protein, coiled-coil, disordered interface, metal-binding; and gain of solvent accessibility, intrinsic disorder, loop, B-factor, catalytic site. The output of MutPred2 tool consists of a general score (g) that represents the average score from all neural networks in MutPred2. The threshold value of ‘g’ score is 0.50. A ‘g-score’ value greater than 0.50 (g > 0.50) for a certain mutation suggest the pathogenicity. [44]. Scores with g-value > 0.5 and p-value < 0.05 are referred to as actionable hypotheses, whereas the scores with g-value > 0.75 and p-value < 0.05 are referred to as confident hypotheses. In MutPred2 prediction, the F48L, M95T, A98D, R125S, Y155C, and I168T substitution showed g-values greater than 0.5 and p-values lower than 0.05 (Table 2).These predicted data provide compelling evidence that the several nsSNPs could play a potential role in the structural and functional modifications of IL-10 protein.
The impact of predicted deleterious mutations on IL-10 protein stability
The nine predicted nsSNPs were further subjected to I-Mutant 3.0, INPS- MD and Mupro tools for protein stability analysis through comparing free energies. Seven of the nine nsSNPs (R42G, R45Q, F48L, E72G, M95T, A98D, and I68T) showed a decrease in structure stability unanimously with all the three analyzed tools. The four variants R42G, R45Q, F48L, and I168T unanimously showed ΔΔG (delta delta G) values less than -1 kcal/mol. The others three variants E72G, M95T, and A98D unanimously showed the ΔΔG values less than zero, which would be predicted to alter the structure and function of the protein by decreasing its stability (Table 3).
Analysis of conservation
Evolutionary conserved amino acids of a protein across species are functionally and biologically very important. Mutation in the conserved region of a protein are often deleterious and may affect the function of the protein. Our ConSurf analysis showed that there are a total of 72 conserved amino acid in the IL-10 protein with scores between 7 and 9. Out of the nine selected high-risk SNPs in IL-10 protein, seven nsSNPs (R45Q, F48L, M95T, A98D, R125S, Y155C, and I168T) were located in the highly conserved region with conservation value ranging 7–9 (Fig. 3). The other two nsSNPs (R42G and E72G) were situated in the variable region. Taken together, our data strongly suggest that the selected nsSNPs are deleterious to IL-10 structure and/or function.
Protein structure analysis
The 3D model structures of the nine mutated IL-10 proteins were generated by Project HOPE (http://www.cmbi.ru.nl/hope/) (Fig. 4). Project HOPE simulates the structural characteristics of amino acid residues substitutions on native protein. Besides, project HOPE showed the physicochemical properties such as size, charge, hydrophobicity values differed between wild and mutant type amino acids, as shown in Table 4. All the nine predicted nsSNPs caused changes in the size of amino acids. Apart from the A98D mutation, the size of the remaining eight mutant amino acids become smaller than the wild type variant. Out of nine nsSNPs, five nsSNPs (R42G, R45Q, E72G, A98D, and R125S) found to alter the amino acid charges in the mutant variant. Also, seven of the nsSNPs (R42G, E72G, M95T, A98D, R125S, Y155C, and I168T) caused changes in hydrophobicity of amino acids (Table 4).
Further analysis with HOPE project found that out of the nine mutations, four (R42G, R45Q, F48L, and R125S) were situated in the domain region. Besides, four mutations (R42G, E72G, R125S, and Y155C) were found to cause the loss of hydrogen bond interaction and three (M95T, A98D, and I168T) caused the loss of hydrophobic interaction. Interestingly, all of the nine mutations were found to locate in the conserved region that might affect the structure and function of IL-10 protein (Table 5).
Protein–protein interaction analysis
The STRING server result showed that Interleukin-10 protein interacts with ten proteins including, interleukin-10 receptor alpha subunit (IL-10RA), interleukin-6 (IL6), tumor necrosis factor (TNF), interleukin-1 beta (IL1B), Interleukin-8 (CXCL8), C–C motif chemokine-2 (CCL2), signal transducer and activator of transcription-3 (STAT 3), Granulocyte–macrophage colony stimulating factor (CSF2), C–C motif chemokine-5 (CCL5), and T-lymphocyte activation antigen CD80 (CD80) (Fig. 5).
Clinical correlation between IL-10 deregulation and the survival rate of patients with different cancer types
In this step, we attempted to associate the deregulation of the IL-10 gene with clinical databases to infer possible functional consequences of IL-10 deregulation in cancer patients. Kaplan–Meier Plotter was used to retrieve the prognostic information of IL-10 gene and analyzed with the survival of patients with gastric, lungs, breast, and ovarian cancer that is shown in Fig. 6. The plot analysis revealed that IL-10 deregulation shows different implications in different cancer types. In the case of gastric and ovarian cancer, the increased level of IL-10 expression predicted decreased number of patients at risk (more survival rate). The HR ratio and P-value for gastric and ovarian cancer were (HR 1.37 [1.14–1.64], P = 0.00078) and (HR 1.22 [1.08–1.39], P = 0.0017) (Fig. 6). In addition, for breast (HR 0.85 [0.75–0.97], P = 0.013) and lung (HR 1.84 [1.52–2.24], P = 0.00000000028) cancer, a low level of IL-10 expressions is associated with a high number of patients at risk (Less survival rate). A control expression of the IL-10 gene appears to be inevitable for a healthy person. An erroneous transcription of the IL-10 gene could lead to the development of different types of cancer. Therefore, the IL-10 gene could be advantageous as a putative prognostic marker for some cancers. Since the nsSNPs have an impact on IL-10 protein’s structure and function, we believe that the nine nsSNPs identified in this study are expected to have almost similar functional consequences in IL-10 deregulation.
Discussion
The human IL-10 gene is located on Chromosome-1 that encodes 178 amino acid long protein. After cleavage of the N-terminal 18 amino acid signal sequence, the mature protein consists of 160 amino acids [8, 50, 51]. Thousands of polymorphisms have already been reported in the both coding and noncoding region of the IL-10 gene. Identification of functionally important SNPs from a pool containing both damaging and neutral SNPs with molecular approaches seems to be expensive and time-consuming. Multiple computational approaches play a great role in predicting and identifying important variants that have damaging effects on proteins structure and function [52,53,54]. However, the present in silico approaches have some weaknesses in prediction of deleterious nsSNPs because every algorithms use different parameters for prediction. Thereby, single algorithms should not be considered for proper prediction of deleterious nsSNPs. In order to predict deleterious nsSNPs precisely requires implementation of different algorithms with different parameters and aspects. A consensus result obtained from the majority of the tools can provide a reliable outcome. In this study, we investigated the genetic variations in IL-10 locus. Nine high-risk missense SNPs were identified by seven different computational tools amid 91 missense SNPs that have been reported to date. The filtered nine nsSNPs were analyzed in I-Mutant 3.0, Mupro and IPNS-MD to investigate their protein stability effects. Seven of the nsSNPs were found to cause a decrease in stability, whereas the two others predicted to increase the rigidity of IL-10 protein (Table 3). We have predicted the conserved amino acid residues in the IL10 protein based on evolutionary conservation using ConSurf. The ConSurf results revealed that most of the high risk nsSNPs position located in a highly conserved region (Fig. 3). The reason of molecular alternation that potentially affects the structure and function of the IL10 protein were examined using MutPred2 web server (Table 2). Alternation of protein’s stability affects the conformational structure and thus governs the function of a protein [55]. The aforementioned nsSNPs affect the proteins stability and might cause maximum damaging effects on its structure and function. Decreased protein stability may change the protein folding mechanism and can cause increased degradation or aberrant aggregation of proteins [56, 57]. Project HOPE software results have provided important information about the possible effects of missense SNPs of IL-10 gene. The polymorphisms (rs1274280163, rs550164520, rs745923816, rs545228684, rs1354773439, rs1421978042, rs771912629, rs1022828778, and rs1310781150) result in R42G, R45Q, F48L, E72G, M95T, A98D, R125S, Y155C, and I168T amino acid substitutions, respectively. Those substituted amino acids have different physiochemical properties that may interrupt the IL-10 protein structure. Due to the polymorphisms, the mutated residues (R42G, E72G, R125S, and Y155C) were more hydrophobic than wild-type residues, which might cause the loss of Hydrogen bond with other molecules and may disrupt correct protein folding. In contrast, the wild-type amino acid residues were more hydrophobic than in A98D and I168T mutation, resulting in loss of hydrophobic interactions with other molecules on the surface of the protein.
It is well established that the human IL-10 protein is a tight dimer consisting of two interpenetrating subunits. Each of the subunits of IL-10 protein comprises six alpha-helices named A-F. The dimeric structure of IL-10 is mainly stabilized by the intertwining of helices E (amino acid position 118 to 131) and F (amino acid position 133 to 159) across the subunit interface [50]. Any mutation within the E and F helix regions of IL-10 subunits could affect the dimerization process of IL-10 protein. Interestingly, R125S and Y155C polymorphisms are found to be located in the E and F helices of IL-10 subunits, respectively. From our HOPE project analysis, we observed that R125S and Y155C mutations cause loss of hydrophobic interaction. Additionally, R125S mutation is found to be responsible for amino acid charge alternation. This finding indicates R125S and Y155C mutations could interfere with the intertwining of two subunits and thereby with the dimerization process of IL-10. Furthermore, the polymorphisms R42G, R45Q, F48L, R125S, and Y155C are located in the protein catalytic domain and are crucial for its catalytic function. Mutation of these residues might disrupt the catalytic activity of IL-10. In the wild type IL-10 protein, the amino acids M95 and A98 residues produce an alpha-helix structure (annotated from UniProt). However, the M95T and A98D polymorphisms of IL-10 do not support the alpha-helix as a secondary structure in the respective position. The other two mutations R42G and E72G introduced a glycine residue at these positions. Glycine is very flexible and can disrupt the required rigidity of the protein at this position. The overall results showed that the modeled mutated protein (Fig. 4) is different from wild-type IL-10 protein, resulting in destabilization of the protein and can cause defective binding of IL-10 with its receptor. The mature IL-10 protein harbors three regions, namely region A, B, and C. The region A (LRDLRDAFSRV is in the position no 23 to 33 amino acids), region B (FFQMKDQLDNLLLKESLLE is in 36 to 54 position), and region C (DIFINYIEAYMTMKIRN amino acid positions 144 to 160) are binding site regions that interact with the IL-10R1 receptor [58]. Our findings showed that out of nine high-risk nsSNPs, five are (R42G, R45Q, F48L, E72G, and I168T) mainly situated in the binding site regions. A previous study by Yoon et al. reported previously that the R42G mutation of IL-10 causes an 80% loss of binding affinity with IL-10R1 [59]. Therefore, we can speculate that the mutations in the binding site regions of IL-10 may disrupt their interactions with their respective receptors, ultimately preventing the downstream signaling by IL-10. Blockage of IL-10 signaling may lead to enhanced inflammation and increased number of Tregs and MDSCs, which inhibit tumor immunity, allowing tumors to grow [28]. Long-term enhanced inflammation contributes to tumor initiation and progression [29]. The other predicted four nsSNPs (R45Q, F48L, E72G, and M95T) would be responsible for the loss of protein function, which may result in the deregulation of the JAK-STAT pathway, causing an imbalance in cellular signaling. The mutations caused by those high risk nine nsSNPs may have structural and functional consequences that may lead to enhanced immune response. This observation suggests that the nine nsSNPs could prioritize diseases like Crohn’s disease, allergy, autoimmunity, and other immune-related disorders.
The data obtained from STRING analysis reveal that the IL-10 protein has many vital functions: it inhibits the synthesis of several cytokines, including INF-gamma, IL-2, and IL-3, TNF GN-CSF produced by activated macrophages and by helper T-cells (Fig. 5). It also regulates the growth and differentiation of various cells such as B cells, NK cells, cytotoxic and helper T cells, and other immune cells [2]. Several studies revealed that low level of IL-10 aggravates autoimmunity pathology and disease severity in patients with multiple sclerosis (MS), juvenile onset arthritis, rheumatoid arthritis (RA), severe asthma, and systemic lupus erythematosus (SLE) [25, 60,61,62,63,64]. The abnormal function of IL-10 caused by the identified nsSNPs might enhance the severity of the mentioned diseases.
IL-10 also shows tumor-promoting and tumor-inhibiting properties. Elevated levels of IL-10 are associated with increased tumor growth with poor prognosis and drug resistance. Again, elevated IL-10 expression down regulates class-I and other cytokines that results in control metastasis and inhibits tumorigenesis. Previous studies showed that IL-10 might contribute to gastric cancer pathogenesis [65]. Similarly, a high expression level of IL-10 was reported in ovarian cancer and found to inhibit ovarian cancer cell growth via downregulation of inflammatory cytokine production [66]. The dual effects of IL-10 could be the result of the concentration ranges of this protein. Through this study, elevated IL-10 gene expression has been shown to govern positive significance on overall survival of gastric and ovarian cancer patients (Fig. 6). Any kind of deregulation caused by SNPs in IL-10 gene might create drastic effects on the survival rate of gastric and ovarian cancer patients. It has been found in many studies that a low level of IL-10 expression is associated with the development of human cervical, sporadic colon, and prostate cancer [67,68,69]. It cannot be neglected that a functionally defective version of IL-10 protein could reproduce similar types of phenotype as observed in the lowly expressed IL-10 patients. However, further studies are warranted in order to verify the correlation between defective IL-10 protein and the development of different types of cancers.
Conclusion
In this study we identified nine putative deleterious nsSNPs of IL-10 by using multiple in silico tools. We believe identification of these nsSNPs should aid in the cost-effective and fast screening method to diagnose diseases that are related to IL-10 expression. Additionally, it will greatly ease the approach of experimental designing for future laboratory-based research.
Availability of data and materials
All data analyzed during this study are included in this article.
Abbreviations
- IL-10:
-
Interleukin-10
- SNP:
-
Single nucleotide polymorphism
- TOPMed:
-
Trans Omics for Precision Medicine
- nsSNP:
-
Non-synonymous SNP
- Tregs:
-
Regulatory T-cells
- MDSCs:
-
Myeloid derived suppressor cells
- NCBI:
-
National Center for Biotechnology Information
- SIFT:
-
Sorting Intolerant from Tolerant
- SVM:
-
Support-vector machine
- PROVEAN:
-
Protein Variation Effect Analyzer
- PhD-SNP:
-
Predictor of human Deleterious Single Nucleotide Polymorphism
- PolyPhen- 2:
-
Polymorphism Phenotyping v2
- PANTHER:
-
Protein analysis through evolutionary relationship
- ΔΔG:
-
Delta Delta G
- INPS-MD:
-
Impact of Non-synonymous mutations on Protein Stability-Multi Dimension
- GEO:
-
Gene Expression Omnibus
- EGA:
-
European Genome Phenome Atlas
- TCGA:
-
Cancer Genome Atlas
- OS:
-
Overall survival
- HR:
-
Hazard ratio
- TI:
-
Tolerant index
References
Gonzalez-Garza MT, Cruz-Vega DE, Maldonado-Bernal C (2020) IL10 as Cancer Biomarker. In: Translational research in cancer. IntechOpen
Piazzon MC, Savelkoul HF, Pietretti D, Wiegertjes GF, Forlenza M (2015) Carp Il10 has anti-inflammatory activities on phagocytes, promotes proliferation of memory T cells, and regulates B cell differentiation and antibody secretion. J Immunol 194(1):187–199. https://doi.org/10.4049/jimmunol.1402093
Paul WE, Seder RA (1994) Lymphocyte responses and cytokines. Cell 76(2):241–251. https://doi.org/10.1016/0092-8674(94)90332-8
Justiz Vaillant AA, Qurie A (2018) Immunodeficiency. StatPearls. StatPearls Publishing LLC, Treasure Island
Rahim M, Gibbon A, Collins M, September AV (2019) Genetics of musculoskeletal soft tissue injuries: Current status, challenges, and future directions. In: Sports, exercise, and nutritional genomics. Academic Press, pp 317–339. https://doi.org/10.1016/B978-0-12-816193-7.00015-4
Moore KW, de Waal MR, Coffman RL, O’Garra A (2001) Interleukin-10 and the interleukin-10 receptor. Annu Rev Immunol 19(1):683–765. https://doi.org/10.1146/ammurev.immunol.19.1.683
Bogdan C, Vodovotz Y, Nathan C (1991) Macrophage deactivation by interleukin 10. J Exp Med 174(6):1549–1555. https://doi.org/10.1084/jem.174.6.1549
Sabat R, Grütz G, Warszawska K, Kirsch S, Witte E, Wolk K, Geginat J (2010) Biology of interleukin-10. Cytokine Growth Factor Rev 21(5):331–344. https://doi.org/10.1016/j.cytogfr.2010.09.002
Asadullah K, Sterry W, Volk HD (2003) Interleukin-10 therapy—review of a new approach. Pharmacol Rev 55(2):241–269. https://doi.org/10.1124/pr.55.2.4
Ding Y, Qin L, Zamarin D, Kotenko SV, Pestka S, Moore KW, Bromberg JS (2001) Differential IL-10R1 expression plays a critical role in IL-10-mediated immune regulation. J Immunol 167(12):6884–6892. https://doi.org/10.4049/jimmunol.167.12.6884
Tan JC, Indelicato SR, Narula SK, Zavodny PJ, Chou CC (1993) Characterization of interleukin-10 receptors on human and mouse cells. J Biol Chem 268(28):21053–21059. https://doi.org/10.1016/S0021-9258(19)36892-9
Riley JK, Takeda K, Akira S, Schreiber RD (1999) Interleukin-10 receptor signaling through the JAK-STAT pathway: requirement for two distinct receptor-derived signals for anti-inflammatory action. J Biol Chem 274(23):16513–16521. https://doi.org/10.1074/jbc.27423.16513
Costa GC, da Costa Rocha MO, Moreira PR, Menezes AS, Silva MR, Gollob KJ, Dutra WO (2009) Functional IL-10 gene polymorphism is associated with Chagas disease cardiomyopathy. J Infect Dis 199(3):451–454. https://doi.org/10.1086/596061
Gallagher PM, Lowe G, Fitzgerald T, Bella A, Greene CM, McElvaney NG, O’Neill SJ (2003) Association of IL-10 polymorphism with severity of illness in community acquired pneumonia. Thorax 58(2):154–156. https://doi.org/10.1136/thorax.58.2.154
Magalhães CA, Carvalho MD, Sousa LP, Caramelli P, Gomes KB (2017) Alzheimer’s disease and cytokine IL-10 gene polymorphisms: is there an association? Arq Neuropsiquiatr 75:649–656. https://doi.org/10.1590/0004-282X20170110
Brown SM (1999) Snapping up SNPs. Biotechniques 26(6):1090
Staley JR, Blackshaw J, Kamat MA, Ellis S, Surendran P, Sun BB, Paul DS, Freitag D, Burgess S, Danesh J, Young R (2016) PhenoScanner: a database of human genotype–phenotype associations. Bioinformatics 32(20):3207–3209. https://doi.org/10.1093/bioinformatics/btw373
Cargill M, Altshuler D, Ireland J, Sklar P, Ardlie K, Patil N, Lane CR, Lim EP, Kalyanaraman N, Nemesh J, Ziaugra L (1999) Characterization of single-nucleotide polymorphisms in coding regions of human genes. Nat Genet 22(3):231–238. https://doi.org/10.1038/10290
Rajasekaran R, Doss CG, Sudandiradoss C, Ramanathan K, Rituraj P, Rao S (2008) Computational and structural investigation of deleterious functional SNPs in breast cancer BRCA2 gene. Chin J Biotechnol 24(5):851–856. https://doi.org/10.1016/S1872-2075(08)60042-4
Taliun D, Harris DN, Kessler MD, Carlson J, Szpiech ZA, Torres R, Taliun SA, Corvelo A, Gogarten SM, Kang HM, Pitsillides AN (2021) Sequencing of 53,831 diverse genomes from the NHLBI TOPMed Program. Nature 590(7845):290–299. https://doi.org/10.1038/s41586-021-03205-y
Kucukkal TG, Petukh M, Li L, Alexov E (2015) Structural and physico-chemical effects of disease and non-disease nsSNPs on proteins. Curr Opin Struct Biol 32:18–24. https://doi.org/10.1016/j.sbi.2015.01.003
Kaur T, Thakur K, Singh J, Kamboj SS, Kaur M (2017) Identification of functional SNPs in human LGALS3 gene by in silico analyses. Egypt J Med Hum Genet 18(4):321–328. https://doi.org/10.1016/j.ejmhg.2017.02.001
Welter D, MacArthur J, Morales J, Burdett T, Hall P, Junkins H, Klemm A, Flicek P, Manolio T, Hindorff L, Parkinson H (2014) The NHGRI GWAS Catalog, a curated resource of SNP-trait associations. Nucleic Acids Res 42(D1):D1001–D1006. https://doi.org/10.1093/nar/gkt1229
Wijmenga C, Zhernakova A (2018) The importance of cohort studies in the post-GWAS era. Nat Genet 50(3):322–328
Ng TH, Britton GJ, Hill EV, Verhagen J, Burton BR, Wraith DC (2013) Regulation of adaptive immunity; the role of interleukin-10. Front Immunol 4:129. https://doi.org/10.3389/fimmu.2013.00129
Maloy KJ, Powrie F (2011) Intestinal homeostasis and its breakdown in inflammatory bowel disease. Nature 474(7351):298–306. https://doi.org/10.1038/nature10208
Howes A, Stimpson P, Redford P, Gabrysova L, O’Garra A (2014) Interleukin-10: cytokines in anti-inflammation and tolerance. In: Cytokine frontiers. Springer, Tokyo, pp 327–352. https://doi.org/10.1007/978-4-431-54442-5_13
Merlo P, Cecconi F (2013) XIAP: inhibitor of two worlds. EMBO J 32(16):2187–2188. https://doi.org/10.1038/emboj.2013.152
Balkwill F, Mantovani A (2001) Inflammation and cancer: back to Virchow? The Lancet 357(9255):539–545. https://doi.org/10.1016/S0140-6736(00)04046-0
Coussens LM, Werb Z (2002) Inflammation and cancer. Nature 420(6917):860–867
Kumar P, Henikoff S, Ng PC (2009) Predicting the effects of coding non-synonymous variants on protein function using the SIFT algorithm. Nat Protoc 4(7):1073–1081. https://doi.org/10.1038/nprot.2009.86
Choi Y, Chan AP (2015) PROVEAN web server: a tool to predict the functional effect of amino acid substitutions and indels. Bioinformatics 31(16):2745–2747. https://doi.org/10.1093/bioinformatics/btv195
Capriotti E, Fariselli P (2017) PhD-SNPg: a webserver and lightweight tool for scoring single nucleotide variants. Nucleic Acids Res 45(W1):W247–W252. https://doi.org/10.1093/nar/gkx369
Adzhubei I, Jordan DM, Sunyaev SR (2013) Predicting functional effect of human missense mutations using PolyPhen-2. Curr Protoc Hum Genet 76(1):7–20. https://doi.org/10.1002/0471142905.hg0720s76
Thomas PD, Kejariwal A, Guo N, Mi H, Campbell MJ, Muruganujan A, Lazareva-Ulitsky B (2006) Applications for protein sequence–function evolution data: mRNA/protein expression analysis and coding SNP scoring tools. Nucleic Acids Res 34(2):W645–W650. https://doi.org/10.1093/nar/gkl229
Capriotti E, Calabrese R, Fariselli P, Martelli PL, Altman RB, Casadio R (2013) WS-SNPs&GO: a web server for predicting the deleterious effect of human protein variants using functional annotation. BMC Genomics 14(3):1–7
Ng PC, Henikoff S (2006) Predicting the effects of amino acid substitutions on protein function. Annu Rev Genomics Hum Genet 7:61–80. https://doi.org/10.1146/annurev.genom.7.080505.115630
Capriotti E, Calabrese R, Casadio R (2006) Predicting the insurgence of human genetic diseases associated to single point protein mutations with support vector machines and evolutionary information. Bioinformatics 22(22):2729–2734. https://doi.org/10.1093/bioinformatics/btl423
Calabrese R, Capriotti E, Fariselli P, Martelli PL, Casadio R (2009) Functional annotations improve the predictive score of human disease-related mutations in proteins. Hum Mutat 30(8):1237–1244. https://doi.org/10.1002/humu.21047
López-Ferrando V, Gazzo A, De La Cruz X, Orozco M, Gelpí JL (2017) PMut: a web-based tool for the annotation of pathological variants on proteins, 2017 update. Nucleic Acids Res 45(W1):W222–W228. https://doi.org/10.1093/nar/gkx313
Capriotti E, Fariselli P, Casadio R (2005) I-Mutant2.0: predicting stability changes upon mutation from the protein sequence or structure. Nucleic Acids Res 33(2):W306–W310. https://doi.org/10.1093/nar/gki375
Zhang M, Huang C, Wang Z, Lv H, Li X (2020) In silico analysis of non-synonymous single nucleotide polymorphisms (nsSNPs) in the human GJA3 gene associated with congenital cataract. BMC Mol Cell Biol 21(1):1–3. https://doi.org/10.1186/s12860-020-00252-7
Adiba M, Das T, Paul A, Das A, Chakraborty S, Hosen MI, Nabi AN (2021) In silico characterization of coding and non-coding SNPs of the androgen receptor gene. Inf Med Unlocked 24:100556. https://doi.org/10.1016/j.imu.2021.100556
Pejaver V, Urresti J, Lugo-Martinez J, Pagel KA, Lin GN, Nam HJ, Mort M, Cooper DN, Sebat J, Iakoucheva LM, Mooney SD (2020) Inferring the molecular and phenotypic impact of amino acid variants with MutPred2. Nat Commun 11(1):1–3. https://doi.org/10.1038/s41467-020-19669
Landau M, Mayrose I, Rosenberg Y, Glaser F, Martz E, Pupko T, Ben-Tal N (2005) ConSurf 2005: the projection of evolutionary conservation scores of residues on protein structures. Nucleic Acids Res 33(2):W299-302. https://doi.org/10.1093/nar/gki370
Venselaar H, Te Beek TA, Kuipers RK, Hekkelman ML, Vriend G (2010) Protein structure analysis of mutations causing inheritable diseases. An e-Science approach with life scientist friendly interfaces. BMC Bioinform 11(1):1
Szklarczyk D, Franceschini A, Wyder S, Forslund K, Heller D, Huerta-Cepas J, Simonovic M, Roth A, Santos A, Tsafou KP, Kuhn M (2015) STRING v10: protein–protein interaction networks, integrated over the tree of life. Nucleic Acids Res 43(D1):D447–D452. https://doi.org/10.1093/nar/gku1003
Lacny S, Wilson T, Clement F, Roberts DJ, Faris P, Ghali WA, Marshall DA (2018) Kaplan-Meier survival analysis overestimates cumulative incidence of health-related events in competing risk settings: a meta-analysis. J Clin Epidemiol 93:25–35. https://doi.org/10.1016/j.jclinepi.2017.10.006
Nagy Á, Lánczky A, Menyhárt O, Győrffy B (2018) Validation of miRNA prognostic power in hepatocellular carcinoma using expression data of independent datasets. Sci Rep 8(1):1–9. https://doi.org/10.1038/s41598-018-27521-y
Zdanov A, Schalk-Hihi C, Gustchina A, Tsang M, Weatherbee J, Wlodawer A (1995) Crystal structure of interleukin-10 reveals the functional dimer with an unexpected topological similarity to interferon γ. Structure 3(6):591–601. https://doi.org/10.1016/S0969-2126(01)00193-9
Josephson K, Logsdon NJ, Walter MR (2001) Crystal structure of the IL-10/IL-10R1 complex reveals a shared receptor binding site. Immunity 15(1):35–46. https://doi.org/10.1016/S1074-7613(01)00169-8
Hasnain MJ, Shoaib M, Qadri S, Afzal B, Anwar T, Abbas SH, Sarwar A, Talha Malik HM, Tariq Pervez M (2020) Computational analysis of functional single nucleotide polymorphisms associated with SLC26A4 gene. PLoS ONE 15(1):e0225368. https://doi.org/10.1371/journal.pone.0225368
Rangasamy N, Kumar NS, Santhy KS (2021) Computational analysis of missense variants in MMP2 gene linked with Winchester syndrome and Nodulosis-Arthropathy-Osteolysis reveals structural shift in protein-protein and protein-ligand complexes. Meta Gene. https://doi.org/10.1016/j.mgene.2021.100931
Yadav AK, Singh TR (2021) Novel structural and functional impact of damaging single nucleotide polymorphisms (SNPs) on human SMYD2 protein using computational approaches. Meta Gene 28:100871. https://doi.org/10.1016/j.mgene.2021.100871
Deller MC, Kong L, Rupp B (2016) Protein stability: a crystallographer’s perspective. Acta Crystallogr Sect F Struct Biol Commun 72(2):72–95. https://doi.org/10.1107/S2053230X15024619
Witham S, Takano K, Schwartz C, Alexov E (2011) A missense mutation in CLIC2 associated with intellectual disability is predicted by in silico modeling to affect protein stability and dynamics. Proteins Struct Funct Bioinform 79(8):2444–2454. https://doi.org/10.1002/prot.23065
Kongari N, Cabello-Villegas J, Mallela KM (2010) Missense mutations in dystrophin that trigger muscular dystrophy decrease protein stability and lead to cross-β aggregates. Proc Natl Acad Sci 107(34):15069–15074. https://doi.org/10.1073/pnas.1008818107
Reineke U, Sabat R, Volk HD, Schneider-Mergener J (1998) Mapping of the interleukin-10/interleukin-10 receptor combining site. Protein Sci 7(4):951–960. https://doi.org/10.1002/pro.5560070412
Yoon SI, Logsdon NJ, Sheikh F, Donnelly RP, Walter MR (2006) Conformational changes mediate interleukin-10 receptor 2 (IL-10R2) binding to IL-10 and assembly of the signaling complex. J Biol Chem 281(46):35088–35096. https://doi.org/10.1074/jbc.M606791200
Van Boxel-Dezaire AH, Hoff SC, Van Oosten BW, Verweij CL, Dräger AM, Ader HJ, Van Houwelingen JC, Barkhof F, Polman CH, Nagelkerken L (1999) Decreased interleukin-10 and increased interleukin-12p40 mRNA are associated with disease activity and characterize different disease stages in multiple sclerosis. Ann Neurol 45(6):695–703. https://doi.org/10.1002/1531-8249(199906)45:6%3C695::AID-ANA3%3E3.0.CO;2-R
Crawley E, Kay R, Sillibourne J, Patel P, Hutchinson I, Woo P (1999) Polymorphic haplotypes of the interleukin-10 5′ flanking region determine variable interleukin-10 transcription and are associated with particular phenotypes of juvenile rheumatoid arthritis. Arthr Rheum 42(6):1101–1108. https://doi.org/10.1002/1529-0131(199906)42:6%3C1101::AID-ANR6%3E3.0.CO;2-Y
Hajeer A, Lazarus M, Turner D, Mageed R, Vencovsky J, Sinnott P, Hutchinson I, Ollier W (1998) IL-10 gene promoter polymorphisms in rheumatoid arthritis. Scand J Rheumatol 27(2):142–145. https://doi.org/10.1080/030097498441029
Lim S, Crawley E, Woo P, Barnes PJ (1998) Haplotype associated with low interleukin-10 production in patients with severe asthma. The Lancet 352(9122):113. https://doi.org/10.1016/S0140-6736(98)85018-6
Gibson AW, Edberg JC, Wu J, Westendorp RG, Huizinga TW, Kimberly RP (2001) Novel single nucleotide polymorphisms in the distal IL-10 promoter affect IL-10 production and enhance the risk of systemic lupus erythematosus. J Immunol 166(6):3915–3922. https://doi.org/10.4049/jimmunol.166.6.3915
Chen L, Shi Y, Zhu X, Guo W, Zhang M, Che Y, Tang L, Yang X, You Q, Liu Z (2019) IL-10 secreted by cancer-associated macrophages regulates proliferation and invasion in gastric cancer cells via c-Met/STAT3 signaling. Oncol Rep 42(2):595–604. https://doi.org/10.3892/or.2019.7206
Zhang L, Liu W, Wang X, Wang X, Sun H (2019) Prognostic value of serum IL-8 and IL-10 in patients with ovarian cancer undergoing chemotherapy. Oncol Lett 17(2):2365–2369. https://doi.org/10.3892/ol.2018.9842
Wang Y, Liu XH, Li YH, Li O (2013) The paradox of IL-10-mediated modulation in cervical cancer. Biomed Rep 1(3):347–351. https://doi.org/10.3892/br.2013.69
Čačev T, Radošević S, Križanac Š, Kapitanović S (2008) Influence of interleukin-8 and interleukin-10 on sporadic colon cancer development and progression. Carcinogenesis 29(8):1572–1580. https://doi.org/10.1093/carcin/bgn164
Faupel-Badger JM, Albanes D, Virtamo J, Woodson K, Tangrea JA (2008) Association of IL-10 polymorphisms with prostate cancer risk and grade of disease. Cancer Causes Control 19(2):119–124
Acknowledgements
Not applicable.
Funding
This research did not receive any specific grant from funding agencies in the public or commercial sectors.
Author information
Authors and Affiliations
Contributions
Conceptualization contributed SCD, MAR, and SDG. Data curation contributed SCD. Formal analysis contributed SCD. Writing original draft contributed SCD. Writing review and editing contributed MAR and SDG. All authors read and approved the final manuscript.
Corresponding author
Ethics declarations
Ethics approval and consent to participate
Not applicable.
Consent for publication
Not applicable.
Competing interests
No potential conflict of interest relevant to this article was reported.
Additional information
Publisher's Note
Springer Nature remains neutral with regard to jurisdictional claims in published maps and institutional affiliations.
Supplementary Information
Additional file 1
. List of deleterious nsSNPs predicted by different tools.
Rights and permissions
Open Access This article is licensed under a Creative Commons Attribution 4.0 International License, which permits use, sharing, adaptation, distribution and reproduction in any medium or format, as long as you give appropriate credit to the original author(s) and the source, provide a link to the Creative Commons licence, and indicate if changes were made. The images or other third party material in this article are included in the article's Creative Commons licence, unless indicated otherwise in a credit line to the material. If material is not included in the article's Creative Commons licence and your intended use is not permitted by statutory regulation or exceeds the permitted use, you will need to obtain permission directly from the copyright holder. To view a copy of this licence, visit http://creativecommons.org/licenses/by/4.0/.
About this article
Cite this article
Das, S.C., Rahman, M.A. & Das Gupta, S. In-silico analysis unravels the structural and functional consequences of non-synonymous SNPs in the human IL-10 gene. Egypt J Med Hum Genet 23, 10 (2022). https://doi.org/10.1186/s43042-022-00223-x
Received:
Accepted:
Published:
DOI: https://doi.org/10.1186/s43042-022-00223-x