Abstract
Background
Air pollution has been shown to promote cardiovascular disease in adults. Possible mechanisms include air pollution induced changes in arterial wall function and structure. Atherosclerotic vascular disease is a lifelong process and childhood exposure may play a critical role. We investigated whether air pollution is related to arterial wall changes in 5-year old children. To this aim, we developed an air pollution exposure methodology including time-weighted activity patterns improving upon epidemiological studies which assess exposure only at residential addresses.
Methods
The study is part of an existing cohort study in which measurements of carotid artery intima-media thickness, carotid artery distensibility, elastic modulus, diastolic and systolic blood pressure have been obtained. Air pollution assessments were based on annual average concentration maps of Particulate Matter and Nitrogen Oxides at 5 m resolution derived from the European Study of Cohorts for Air Pollution Effects. We defined children’s likely primary activities and for each activity we calculated the mean air pollution exposure within the assumed area visited by the child. The exposure was then weighted by the time spent performing each activity to retrieve personal air pollution exposure for each child. Time spent in these activities was based upon a Dutch mobility survey. To assess the relation between the vascular status and air pollution exposure we applied linear regressions in order to adjust for potential confounders.
Results
Carotid artery distensibility was consistently associated with the exposures among the 733 5-years olds. Regression analysis showed that for air pollution exposures carotid artery distensibility decreased per standard deviation. Specifically, for NO2, carotid artery distensibility decreased by − 1.53 mPa− 1 (95% CI: -2.84, − 0.21), for NOx by − 1.35 mPa− 1 (95% CI: -2.67, − 0.04), for PM2.5 by − 1.38 mPa− 1 (95% CI: -2.73, − 0.02), for PM10 by − 1.56 mPa− 1 (95% CI: -2.73, − 0.39), and for PM2.5absorbance by − 1.63 (95% CI: -2.30, − 0.18). No associations were observed for the rest outcomes.
Conclusions
The results of this study support the view that air pollution exposure may reduce arterial distensibility starting in young children. If the reduced distensibility persists, this may have clinical relevance later in life. The results of this study further stress the importance of reducing environmental pollutant exposures.
Similar content being viewed by others
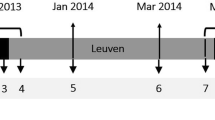
Background
Air pollution may have been related to as many as 4.2 million premature deaths in 2016 globally, 44% of which are due to cardiovascular disease [1]. Oxidative stress and inflammation are suggested to be an important link between air pollution and cardiovascular risk due to atherosclerosis [2,3,4]. Compared to adults, children are more sensitive to air pollution because they breathe in more air per unit body-weight and consequently more air pollution [5]. The impact of air pollution is even more severe for children as their bodies are developing [6]. Additionally, children are exposed to higher concentrations of air pollution because their shorter stature results in them inhaling air from lower heights where some pollutants are in higher concentrations [6]. Thus, air pollution may impact cardiovascular health already early in life [7,8,9,10]. An emerging number of epidemiological studies have observed air pollution related changes in the carotid artery in adults [11,12,13,14,15] and young adults [16]. However, only one study has been reported in young children [17]. Carotid measurements suggest an end organ, non-invasive vascular detection of early signs of atherosclerosis in children. Moreover, there is evidence that there is a relation between tobacco smoke and carotid changes in the young [18]. As the mechanism which is hypothesized to lead to carotid changes from tobacco smoke is the same as with air pollution; we set out to determine the putative association between air pollutants and vascular characteristics in young children using a novel methodology that takes movements across various levels of exposure into account.
So far the methodology applied in large epidemiological studies, to estimate air pollution exposures, is mainly based on air pollution (point) estimates which either derived from individuals’ residential addresses [16, 19,20,21,22], or from schools’ addresses [23], or from the combination of the afore mentioned [8]. However, this might not be representative of the true exposure because humans are not static and do not spend their entire day at a fixed location [24]. Thus, person’s displacements are important to consider since air pollution differs considerably between areas where the activities take place [25,26,27]. Personal exposure can be described as the time-weighted average air pollution concentration of all activities of a person [28]. For this reason, there is a need to apply improved exposure assessment methods in epidemiological studies [29, 30] when sensor data are not available [31].
Our first aim was to model air pollution exposure based on a time-weighted activity pattern and detailed spatial maps of air pollution. Next, we assessed the relation between multiple air pollution exposures (PM10, PM2.5, PM2.5absorbance, NOx and NO2) and cardiovascular markers in 5-yearold children.
Subjects and methods
Study population
This study is part of the Wheezing Illnesses Study Leidsche Rijn (WHISTLER), an ongoing population based prospective birth cohort study [32]. Virtually all participants are living in a 25 km2 residential area in the north-west of the Utrecht metropolitan area or nearby areas of Utrecht Municipality (Fig. 1).
The study was initiated in 2001 with a focus on lung disease and expanded in 2007 to include a range of measurements on cardiovascular development [18]. The current analyses used data obtained when the children had reached 5-years of age, which included measurements of carotid artery wall structure, and function (carotid Intima Media Thickness, carotid distensibility and Elastic Modulus). The carotid artery variables were measured by ultrasonography using high-resolution echo-tracking technology (Art.laboratory, Esaote, Italy) as described previously [18].
Outcome and potential confounders
In this analysis the outcomes studied are the vascular conditions of the right common carotid artery which were measured ultrasonographically as described elsewhere [18, 32]. Carotid Intima Media Thickness (cIMT), carotid Distensibility (cD) and Elastic Modulus (EM) per individual were used to assess the elastic properties of the carotid artery, including the blood pressure measurements (systolic and diastolic).
As potential confounders we included maternal smoking in pregnancy [18, 33] and exposure to tobacco smoke [34, 35] which are suggested to affect vascular determinants in childhood and can be also associated with the exposure [36]. Socio-economic status (SES) was considered possible confounder because of its putative relation with determinant and outcome [37]. Although it is reported that normal carotid arterial wall is unaffected by age and sex until a certain age (10-years), it is uncertain if the uniform results are due to the low sensitivity of the imaging methods [38] and as such differences were observed in adults we included age and sex as potential confounders [39]. The general confounders (age, sex) and cigarette smoke exposure were extracted from the questionnaire filled during the 5-years old visit. The maternal smoking in pregnancy and SES questions were gathered by a questionnaire filled in by the mother during baseline examination [32].
Modelling the individual air pollution exposures
To assess air pollution levels at the home addresses we used land use regression (LUR) models. The models were originally developed in the European Study of Cohorts for Air Pollution Effects (ESCAPE) project and described elsewhere [40, 41]. The models provide the annual average concentrations of several air pollutants at any location in the study area in the year 2010, including NO2, NOx, PM10, PM2.5 and PM2.5absorbance [42].
To extract information about the time spent at each activity we used the Onderzoek Verplaatsingen in Nederland 2010 (OViN: Study on mobility in the Netherlands) dataset [43]. This dataset includes information about the mobility of 1847 children aged 4–6 years living in the Netherlands. The parents who participated created a log over one week containing information about the place of origin and destination, the time when transport takes place, the used means of transport and the travel motives for each movement of their child [43].
To define where the children’s activities took place we used land use data: TOP10NL and Basisregistratie Adressen en Gebouwen (BAG), which is the Dutch cadastral information. Both datasets were available at the Dutch National Spatial Data Infrastructure (SDI): the Publieke Dienstverlening Op de Kaart (PDOK) [44]. Finally, school locations in the study area were recorded by University of Groningen Open Data [45].
The WHISTLER dataset included the residential address for each child defined by street name and house number. To link the address information to air pollution we transformed the addresses to coordinates by geocoding using Nominatim -a search engine for OpenStreetMap (OSM) [46]. The OSM point data were retrieved in WGS84 datum (EPSG 4326). We have re-projected the OSM point data to the local coordinate system: Amersfoort RD New datum (EPSG 28992) to correspond with the rest of the datasets.
To calculate each child’s individual exposures we defined their primary activities (being at home, playing in the neighbourhood, travelling to/from school or other destinations, and being at school) and then we calculated individualized exposures using the following formula:
In Eq. 1, E is the personal exposure (μg/m3), Ch the air pollution concentration (μg/m3) representative for the being at home activity, and Th the time (minutes) spent at an activity place, Cp the air pollution concentration for playing in the neighbourhood, Tp the time spent playing in the neighbourhood, Cs the air pollution concentration at school, Ts the time spent at school or at other educational activities, Ct the air pollution concentration at the road network, Tt the time spent travelling, i is the child id from 1 up to 733 and j the air pollutant 1 up to 6. All time units were measured in minutes;the denominator represents the total number of minutes in a day.
To compute the average air pollution concentration for each activity (Ch, Cp, Cs, Ct) we followed three primary steps. First, for each activity we defined the area where that activity can take place based on distance from home -by estimating the maximum distance away from home that a child would go during that particular activity. To define the buffer-sizes we made an educated guess based on the spatial scale of Leidsche Rijn. To represent the activity ‘being at home’ (Ch) we used a 20 m buffer (Fig. 2a). To represent the activity ‘playing in the neighbourhood’ (Cp), which includes activities such as playing at a nearby green area or visiting a neighbour, we used a 500 m buffer (Fig. 2b). A buffer of 2000 m was applied to represent activities which include travelling in the broader area, such as ‘travelling to/from school’ (Ct) or following their parents to the super market (Fig. 2c). Finally, to represent the activity ‘being at school’ (Cs) or to other educational activities, we applied 20 m buffers around all primary schools -by using the 20 m buffer we made sure that we included the complete educational building including their facilities-. Then, to identify the possible schools visited by each child we used a 2000 m buffer around each child’s house. For all schools within a 2000 m buffer we averaged their 20 m buffer air pollution concentrations (Fig. 2d).
Representation of the buffers used, shown for a single (arbitrarily chosen) residential address (red) within the area of interest. Blue indicates the land use related to each activity (dark blue: intersecting polygons (within buffer), light blue: outside the buffer. a 20 m buffer around house to represent staying at home activity (note that the buffer is calculated around the location of the front door of the house, located at the upper-side in the figure), b 500 m buffer around house including open public and private space to represent the activity playing in the neighbourhood, c 2000 m buffer around the house including the road network to represent activities which include travelling, d 20 m buffer around schools which were included in the 2000 m buffer around the house to represent being at school activity
Second, to estimate where a certain activity takes place, the land-uses were important to consider because there were certain land-use types that were not accessible (e.g. railways and industrial areas) or were not related to the activity we wanted to represent (e.g. cemeteries). Thus, we created maps of the area that could be visited during a particular activity as a function of land-use by producing maps that indicate the areas that can be reached as a function of distance from home location (step 1). For the activity staying at home (Ch) we did not remove any land-uses (Fig. 2a). For the activity playing in the neighbourhood (Cp) we computed the union of all possible land-uses from TOP10NL where a child can play (mainly open public and private spaces) (Fig. 2b). For the travelling activity (Ct), the road network layer was used (Fig. 2c). For the activity being at school (Cs) we used only the school areas (Fig. 2d).
Third, for each child and for each activity we created the intersection between the area that can be reached based on distance (step 1) and the area that will be visited during a particular activity (step 2). The intersection resulted in a map with the area visited during a particular activity for each child. This was done by first rasterizing (5 m cell size) the vector information generated in step 1 and 2 and executing their intersection.
Then, for each activity place and for each child, we calculated the average air pollution (respectively Ch, Cp, Cs, Ct) by averaging the air pollution concentration within the area that was visited during the activity (calculated in step 3), assuming that the presence of the child was uniformly distributed over the area representing an activity. This was done by first calculating air pollution concentration levels over the entire study area -by applying the LUR models, derived from the ESCAPE models- and then averaging the values over the area which represented the activity for each child.
Finally, we used the OViN dataset to calculate the predicted average time children spent performing each of the primary activities (Eq. 1). To be able to deduct to the children participated in WHISTLER we selected all children between 4 and 6 year olds from the OViN dataset.. One of the assumptions we used in the model was the duration children spent at each activity place because this information was not available in the Whistler cohort. Therefore, we obtained this information from 4 to 6 years old children in the OViN study which is a random sample from the Dutch population and assumed that the children in the Whistler cohort would spend the same time at each activity place.
We found that children spent on average spent 964 min at home (Th) per day, in addition to this 28 min were spent playing in the neighbourhood (Tp), 49 min travelling to school or travelling to other everyday activity (Tt) and 399 min being at school and at other educational activities (Ts).
All spatial computations based on vector files were performed using ArcGIS 10.4.1. and the raster calculations were performed in the PCRaster environment [47].
Data analyses
We fitted models for all dependent variables (carotid Intima Media Thickness: cIMT, carotid distensibility: cD, Elastic Modulus: EM, Diastolic Blood Pressure: DBP and Systolic Blood Pressure: SBP) and for all personal exposures (NO2, NOx, PM10, PM2.5 and PM2.5absorbance) calculated using Eq. 1. The exposures were entered as continuous variables. We tested the regression assumptions including linearity and found no deviation from linearity. We therefore used linear regression to obtain the association and the 95% confidence interval (CI) between the air pollutants and health variables.
We first fitted unadjusted models (Model 0) and then we adjusted for possible confounders by specifying four models with increasing levels of adjustment. In the first adjusted model (Model 1) we included sex and age. In the second model (Model 2a) we additionally adjusted for individual socio-economic status (SES) of parents (parental SES definition; 0: none of the parents was highly educated, 1: one of the parents was highly educated (university degree), 2: both of parents were highly educated). Finally, in Model 2b we added smoking of mother during pregnancy: Did you smoke during the pregnancy? (yes/no) and exposure to smoke in later life: Is your child exposed to smoke? (‘yes’, ‘no or not anymore’) in Model 2a. Finally, we performed subgroup analysis to the fullest model based on sex.
The confounding variables: sex, smoking of mother during pregnancy, smoking next to the child and individual parental SES were entered as categorical variables while age was entered as a continuous variable (Table 1). Observations with missing values for a variable were dropped from models including that variable. Specifically, we started with all the observations in Model 0 and Model 1 but approximately 16% of observations were lost in the most extensive confounder model (Model 2b) because values were missing for one or more of the confounders. The statistical analysis was performed using R version 3.5.0.
Results
Data on 733 healthy young children (mean age, 5.42 years) were used in the analyses. 51% of the participants were girls. 7.0% of children were exposed to cigarette smoke during pregnancy and 6.1% later in life (Table 1).
Air pollution concentrations of the five air pollutants for each activity are presented in Table 3. Contrasts in exposures were moderate for NO2 and NOx while for PM2.5 and PM10 contrasts were rather limited (Table 3). Concentration levels differ considerably between the activities for all air pollutants (Fig. 3). For all air pollutants the 2000 m buffer, which includes the road network to represent the activity ‘travelling to every-day activities’, had the highest concentration (Table 3); the distributions of the exposures are presented in Fig. 4. Correlations between the time-weighted activity pattern exposures (E) and front door exposure (Cd) were above 0.84 with substantial scatter for NO2 (Additional file 1: Figure A1) as well as the correlations of the five air pollutants (Tables 2 and 3).
NO2 distributions during children’s primary activities. a, NO2 at home – 20 m buffer around home location (Ch); b, NO2 playing in the neighbourhood – 500 m around home location (Cp); c, NO2 going at school or following parents – 2000 m around home location (Ct); d, NO2 being at school – 20 m around all schools within 2000 m around home (Cs)
Distributions of air pollution exposures (Ej), calculated from Eq. 1. a NO2 distribution; b.NOx distribution; c PM2.5 distribution; d PM10 distribution; e PM2.5absorbance distribution
We observed statistically significant associations between air pollutants and carotid artery distensibility (Table 4). In model 0 (unadjusted model) and model 1 (adjusted for age and sex) only PM10 and PM2.5absorbance were associated with carotid artery distensibility (Table 4), whereas we observed an association for all air pollutants in model 2a and 2b. The regression slope of the full model (2b) was − 1.53 mPa-1 per SD NO2 increase (95% CI: -2.84, − 0.21), − 1.35 mPa-1 per SD NOx increase (95% CI: -2.67, − 0.04), − 1.38 mPa-1 per SD PM2.5 increase (95% CI: -2.73, − 0.02), − 1.56 mPa-1 per SD PM10 increase (95% CI: -2.73,-0.39), and - 1.63 mPa-1 per SD PM2.5absorbance increase (95% CI -2.30,-0.18). No statistically significant associations between the air pollutants and carotid artery intima-media thickness, elastic modulus and diastolic and systolic blood pressure were observed. Subgroup analysis for the fullest model stratified by sex showed stronger association for cD for boys but the difference in effect estimates were not statistically significant (Additional file 2: Table S1).
Discussion
In the present study -using detailed vascular measurements and improved air pollution exposures in a large group of young children- all pollutants showed adverse relationships with carotid arterial distensibility independent from confounding variables. We did not observe any association between air pollution exposures and carotid artery intima-media thickness, elastic modulus or diastolic and systolic blood pressure at this young age.
To appreciate these findings, we have to address some aspects of the present study. First, a primary strength of this study is the use of a sophisticated exposure assessment based on validated geo-data to derive information for the possible activity pattern of children. Second, we enriched air pollution exposure data with time in order to derive a time-weighted activity pattern with the air pollution maps at a fine spatial scale. Third, we were able to assess several air pollutants including particulate matter (PM10, PM2.5, PM2.5absorbance) and nitrogen oxides (NOx, and NO2). Finally, this study is the first to evaluate the associations between air pollution and early atherosclerotic markers in the age of 5-years old children.
Some limitations should be addressed. A common challenge in similar studies is the individual exposure assessment, particularly because of the high spatio-temporal variation in air pollution. In this study, we present a method that takes into account the air pollution spatial variation by integrating air pollution over multiple activity zones corresponding to particular daily activities of children. In principle, this should lead to more realistic air pollution exposure values as the values represent the areas visited for a certain time. This is not the case when front-door pollution values are used to represent individual exposures. However, our method is prone to uncertainties in the estimation of the activity zones as well as the time spend on each activity. Specifically, we had no information on child-specific individual location of and time spent in activities, but we used the mobility survey to derive likely patterns. Given that few epidemiological studies have actual data on time activity, our approach represents a realistic method to incorporate time activity data. This approach can be further improved by incorporating temporal and seasonal variation in air pollution as well as indoor air pollution estimates if the interest lies in other sources of pollution. Moreover, the exposure assessment can be improved if more data related to the exact spatio-temporal locations visited by the children are available [48]. This would lead to an approach that relies on fewer assumptions. Furthermore, the air pollution exposures were calculated by using ESCAPE models for the year 2010 while the health measurements were collected between 2007 and 2013. Thus, the air pollution datasets cover partially the health measurement timeframe. However, as supported by previous research, an annual average of a single year is representative for a larger time-frame because the distribution of air pollution is stable for up to 8 years [49, 50], thus the models can be considered valid for the complete timeframe (2007–2013). Additionally, we did not access the particle composition which would be informative in terms of specific toxicants. We were unable to correct for possible confounders related to maternal cardio-metabolic conditions and nutritional status due to data unavailability. Although there were missing values within the dataset, the small differences in effect estimates between the different models argue against important selection bias derived from missing data. This study showed that increased air pollution is adversely related to carotid artery distensibility. Arterial distensibility is a measure of the arterial ability to expand and contract with cardiac pulsation and relaxation [51]. A decrease in arterial distensibility (increased artery wall stiffness) is generally observed with ageing, is accelerated by a number of cardiovascular risk factors such as smoking and blood pressure elevation and promotes the occurrence of symptomatic cardiovascular disease [52, 53]. Impairment of arterial wall function typically occurs in an early stage of the atherosclerotic process before structural wall changes (cIMT) become detectable [16, 54].
A previous study from Iannuzzi et al. (2010), which included 52 children aged 6 to 14 years, similarly reported a relation between air pollution exposure and carotid intima stiffness –in contrast no relation was found for arterial thickness. In addition, they observed no association between air pollution exposure and diastolic blood pressure. These results are all in line with the findings of this current study. In contrast, associations between air pollution exposure and higher blood pressure have been observed in a number of studies with children between 8 and 12 years [8, 23, 55]. From the stratified analysis based on sex analysis we did not observe differences between sexes.
We did not observe an association between air pollution exposure and structural arterial wall changes as measured by ultra-sonographic measurements of carotid intima media thickness. The most likely explanation is the young age of the participants, as structural abnormalities have not yet developed and therefore cannot be detected. No previous studies have attempted to relate air pollution to carotid artery intima-media thickness at such a young age. In adults though, there is evidence of structural arterial wall changes due to air pollution exposure [11, 56].
Another explanation is that the level of exposure was too low. Exposure levels were moderate compared to other European countries [57] and low in the global context [58]. Systematic inflammation is triggered by the increased levels of particulate matter and nitrogen oxides [3, 16]. Finally, there was low variation of especially PM2.5 and PM10 exposure in the study area. For NO2 and NOx the contrast in exposure was moderate, consistent with previous work documenting that local sources affect NO2 more than PM2.5 [57]. Consistently, the confidence intervals for NO2 indicated that we were able to estimate air pollution effect sizes with good precision. The significant effect estimate for cD for NO2 translates into a 2–3% decrease per 1 SD.
The cross-sectional design does not allow detecting vascular changes through time as individual exposure to air pollution varied in different years. To elucidate more about the nature of the association and to be able to show causality a prospective study design is recommended. It would be of high interest to test the associations for children that have been exposed over a longer period to investigate if the associations persist, for example using the same population when they reach the adolescence stage. Furthermore, our exposure assessment method could be replicated in an area with greater air-pollution variation.
Conclusions
The results of this study suggest that air pollution may contribute to vascular disease starting at a very young age. Therefore, it is likely that that early-life air pollution exposures might be the key to more effective strategies for prevention of cardio-vascular disease. In view of the enormous numbers of children facing lifelong exposure to environmental air pollution and the epidemic of cardiovascular disease, the findings stress the need for reductions in air pollution and reduction of individual exposure.
Abbreviations
- cD:
-
carotid Distensibility
- CI:
-
Confidence Interval
- cIMT:
-
carotid Intima Media Thickness
- DBP:
-
Diastolic Blood Pressure
- EM:
-
Elastic Modulus
- ESCAPE:
-
European Study of Cohorts for Air Pollution Effects
- LUR:
-
Land Use Regression
- NO2 :
-
Nitrogen Dioxide
- NOx :
-
Nitrogen Oxides
- OViN:
-
Onderzoek Verplaatsingen in Nederland (eng: Study on mobility in the Netherlands)
- PM10 :
-
Particulate matters with diameter < 10 μm
- PM2.5 :
-
Particulate matters with diameter < 2.5 μm
- SBP:
-
Systolic Blood Pressure
- SD:
-
Standard Deviation
- SES:
-
Socio-economic status
- WHISTLER:
-
Wheezing Illnesses Study Leidsche Rijn
References
World Health Organization. Burden of disease from ambient air pollution for 2016. Geneva; 2018. https://www.who.int/airpollution/data/AAP_BoD_results_May2018_final.pdf. Accessed 12 Nov 2018
Chin MT. Basic mechanisms for adverse cardiovascular events associated with air pollution. Heart. 2015;101:253–6. https://doi.org/10.1136/heartjnl-2014-306379.
Newby DE, Mannucci PM, Tell GS, Baccarelli AA, Brook RD, Donaldson K, et al. Expert position paper on air pollution and cardiovascular disease. Eur Heart J. 2015;36:83–93.
Brook RD, Rajagopalan S, Pope CA III, Brook JR, Bhatnagar A, Diez-Roux AV, et al. Particulate matter air pollution and cardiovascular disease: an update to the scientific statement from the American Heart Association. Circulation. 2010;121:2331–78.
Kleinman MT. The health effects of air pollution on children. California; 2000. http://www.aqmd.gov/docs/default-source/students/health-effects.pdf. Accessed 12 Nov 2018.
World Health Organization. Air pollution and child health: prescribing clean air. Geneva: World Health Organization; 2018. http://www.who.int/ceh/publications/air-pollution-child-health/en/. Accessed 12 Nov 2018
Bateson TF, Schwartz J. Children’s response to air pollutants. J Toxicol Environ Heal Part A. 2007;71:238–43. https://doi.org/10.1080/15287390701598234.
Bilenko N, van Rossem L, Brunekreef B, Beelen R, Eeftens M, Hoek G, et al. Traffic-related air pollution and noise and children’s blood pressure: results from the PIAMA birth cohort study. Eur J Prev Cardiol. 2013:2047487313505821. https://doi.org/10.1177/2047487313505821.
Landrigan PJ. Environmental hazards for children in USA. Int J Occup Med Environ Health. 1998;11:189–94 http://www.ncbi.nlm.nih.gov/pubmed/9753898. Accessed 26 Oct 2017.
Salvi S. Health effects of ambient air pollution in children. Paediatr Respir Rev. 2007;8:275–80. https://doi.org/10.1016/j.prrv.2007.08.008.
Künzli N, Jerrett M, Mack WJ, Beckerman B, LaBree L, Gilliland F, et al. Ambient air pollution and atherosclerosis in Los Angeles. Environ Health Perspect. 2005;113:201–6. https://doi.org/10.1289/ehp.7523.
Künzli N, Jerrett M, Garcia-Esteban R, Basagaña X, Beckermann B, Gilliland F, et al. Ambient air pollution and the progression of atherosclerosis in adults. PLoS One. 2010;5. https://doi.org/10.1371/journal.pone.0009096.
Diez Roux AV, Auchincloss AH, Franklin TG, Raghunathan T, Barr RG, Kaufman J, et al. Long-term exposure to ambient particulate matter and prevalence of subclinical atherosclerosis in the multi-ethnic study of atherosclerosis. Am J Epidemiol. 2008;167:667–75. https://doi.org/10.1093/aje/kwm359.
Wilker EH, Mittleman MA, Coull BA, Gryparis A, Bots ML, Schwartz J, et al. Long-term exposure to black carbon and carotid intima-media thickness: the normative aging study. Environ Health Perspect. 2013;121:1061–7. https://doi.org/10.1289/ehp.1104845.
Tonne C, Yanosky JD, Beevers S, Wilkinson P, Kelly FJ. PM mass concentration and PM oxidative potential in relation to carotid intima-media thickness. Epidemiology. 2012;23:486–94. https://doi.org/10.1097/EDE.0b013e31824e613e.
Lenters V, Uiterwaal CS, Beelen R, Bots ML, Fischer P, Brunekreef B, et al. Long-term exposure to air pollution and vascular damage in young adults. Epidemiology. 2010;21:512–20. https://doi.org/10.1097/EDE.0b013e3181dec3a7.
Iannuzzi A, Verga MC, Renis M, Schiavo A, Salvatore V, Santoriello C, et al. Air pollution and carotid arterial stiffness in children. Cardiol Young. 2010;20:186–90. https://doi.org/10.1017/S1047951109992010.
Geerts CC, Bots ML, Van Der ECK, Grobbee DE, Uiterwaal CSPM. Parental smoking and vascular damage in their 5-year-old children. Pediatrics. 2013;129:45–54.
Clark NA, Demers PA, Karr CJ, Koehoorn M, Lencar C, Tamburic L, et al. Effect of early life exposure to air pollution on development of childhood asthma. Environ Health Perspect. 2010;118:284–90.
Gehring U, Wijga AH, Brauer M, Fischer P, De Jongste JC, Kerkhof M, et al. Traffic-related air pollution and the development of asthma and allergies during the first 8 years of life. Am J Respir Crit Care Med. 2010;181:596–603.
Jerrett M, McConnell R, Wolch J, Chang R, Lam C, Dunton G, et al. Traffic-related air pollution and obesity formation in children: a longitudinal, multilevel analysis. Environ Health. 2014;13:49. https://doi.org/10.1186/1476-069X-13-49.
Nordling E, Berglind N, Melén E, Emenius G, Hallberg J, Nyberg F, et al. Traffic-related air pollution and childhood respiratory symptoms, function and allergies. Epidemiology. 2008;19:401–8. https://doi.org/10.1097/EDE.0b013e31816a1ce3.
Sughis M, Nawrot TS, Ihsan-ul-Haque S, Amjad A, Nemery B. Blood pressure and particulate air pollution in schoolchildren of Lahore, Pakistan. BMC Public Health. 2012;12:378. https://doi.org/10.1186/1471-2458-12-378.
Dijst M. Time Geographic Analysis Int Encycl Hum Geogr. 2009:266–78. https://doi.org/10.1016/B978-008044910-4.00548-4.
Park YM, Kwan MP. Individual exposure estimates may be erroneous when spatiotemporal variability of air pollution and human mobility are ignored. Heal Place. 2017;43:85–94. https://doi.org/10.1016/j.healthplace.2016.10.002.
Setton EM, Peter CP, Cloutier-Fisher D, Hystad PW. Spatial variations in estimated chronic exposure to traffic-related air pollution in working populations: a simulation. Int J Health Geogr. 2008;7. https://doi.org/10.1186/1476-072X-7-39.
Dons E, Panis LI, Poppel M Van, Theunis J, Willems H, Torfs R, et al. Impact of timeeactivity patterns on personal exposure to black carbon. 2011. https://doi.org/10.1016/j.atmosenv.2011.03.064.
World Health Organization. Monitoring ambient air quality for health impact assessment. WHO Reg Publ Eur Ser. 1999;85:i–xvii, 1–196. http://www.euro.who.int/__data/assets/pdf_file/0010/119674/E67902.pdf. Accessed 14 Nov 2017.
Bråbäck L, Forsberg B. Does traffic exhaust contribute to the development of asthma and allergic sensitization in children: findings from recent cohort studies. Environ Health. 2009;8:17. https://doi.org/10.1186/1476-069X-8-17.
Dias D, Tchepel O. Spatial and temporal dynamics in air pollution exposure assessment. Int J Environ Res Public Health. 2018;15.
Nieuwenhuijsen MJ, Donaire-Gonzalez D, Foraster M, Martinez D, Cisneros A. Using personal sensors to assess the exposome and acute health effects. Int J Environ Res Public Health. 2014;11:7805–19. https://doi.org/10.3390/ijerph110807805.
Katier N, Uiterwaal CSPM, De Jong BM, Kimpen JLL, Verheij TJ, Grobbee DE, et al. The wheezing illnesses study Leidsche Rijn (WHISTLER): rationale and design. Eur J Epidemiol. 2004;19:895–903.
Taal HR, de Jonge LL, van Osch-Gevers L, Steegers EA, Hofman A, Helbing WA, et al. Parental smoking during pregnancy and cardiovascular structures and function in childhood: the generation R study. Int J Epidemiol. 2013;42:1371–80. https://doi.org/10.1093/ije/dyt178.
Seyedzadeh A, Hashemi F, Soleimani A. Relationship between blood pressure and passive smoking in elementary school children. Iran J Pediatr. 2012;22:351–6 http://www.ncbi.nlm.nih.gov/pubmed/23400119. Accessed 12 Mar 2019.
Aycicek A, Erel O, Kocyigit A. Increased oxidative stress in infants exposed to passive smoking. Eur J Pediatr. 2005;164:775–8. https://doi.org/10.1007/s00431-005-1720-1.
Ruprecht AA, De Marco C, Pozzi P, Mazza R, Munarini E, Di Paco A, et al. Outdoor second-hand cigarette smoke significantly affects air quality. Eur Respir J. 2016;48:918–20. https://doi.org/10.1183/13993003.00064-2016.
Fecht D, Fischer P, Fortunato L, Hoek G, de Hoogh K, Marra M, et al. Associations between air pollution and socioeconomic characteristics, ethnicity and age profile of neighbourhoods in England and the Netherlands. Environ Pollut. 2015;198:201–10. https://doi.org/10.1016/J.ENVPOL.2014.12.014.
Baroncini LAV, Sylvestre L de C, Pecoits Filho R. Assessment of intima-media thickness in healthy children aged 1 to 15 years. Arq Bras Cardiol. 2016;106:327–32. https://doi.org/10.5935/abc.20160030.
Raitakari OT, Laitinen T, Hutri-Kähönen N, Lehtimäki T, Jokinen E, Magnussen CG, et al. Effect of age, gender and cardiovascular risk factors on carotid distensibility during 6-year follow-up. The cardiovascular risk in young Finns study. Atherosclerosis. 2012;224:474–9. https://doi.org/10.1016/j.atherosclerosis.2012.04.004.
Beelen R, Hoek G, Vienneau D, Eeftens M, Dimakopoulou K, Pedeli X, et al. Development of NO2 and NOx land use regression models for estimating air pollution exposure in 36 study areas in Europe – the ESCAPE project. Atmos Environ. 2013;72:10–23.
Eeftens M, Beelen R, de Hoogh K, Bellander T, Cesaroni G, Cirach M, et al. Development of land use regression models for PM2.5, PM2.5 absorbance, PM10 and PMcoarse in 20 European study areas; results of the ESCAPE project. Environ Sci Technol. 2012;46:11195–205.
Schmitz O, Beelen R, Strak M, Hoek G, Soenario I, Brunekreef B, et al. High resolution air pollution concentration maps for the Netherlands. Sci Data. 2018:1–17.
CBS. Onderzoek Verplaatsingen in Nederland 2014. 2015; july:39. doi:https://doi.org/10.17026/dans-x95-5p7y.
Publieke Dienstverlening Op de Kaart Loket | Landsdekkende Geo Informatie van de Overheid. https://www.pdok.nl/. Accessed 22 Dec 2017.
Home | University of Groningen Open Data. http://opendata.rug.nl/. Accessed 22 Dec 2017.
OpenStreetMap Nominatim: Search. https://nominatim.openstreetmap.org/. Accessed 1 Jun 2018.
Karssenberg D, Schmitz O, Salamon P, De JK, Bierkens MFP. Environmental Modelling & Software a software framework for construction of process-based stochastic spatio-temporal models and data assimilation. Environ Model Softw. 2010;25:489–502. https://doi.org/10.1016/j.envsoft.2009.10.004.
Dijst M, Worrell E, Böcker L, Brunner P, Davoudi S, Geertman S, et al. Exploring urban metabolism—towards an interdisciplinary perspective. Resour Conserv Recycl. 2018;132:190–203. https://doi.org/10.1016/J.RESCONREC.2017.09.014.
Eeftens M, Beelen R, Fischer P, Brunekreef B, Meliefste K, Hoek G. Stability of measured and modelled spatial contrasts in NO2 over time. Occup Environ Med. 2011;68:765–70.
Gulliver J, Morris C, Lee K, Vienneau D, Briggs D, Hansell A. Land use regression modeling to estimate historic (1962−1991) concentrations of black smoke and sulfur dioxide for Great Britain. Environ Sci Technol. 2011;45:3526–32.
Kawasaki T, Sasayama S, Yagi S-I, Asakawa T, Hiray T. Non-invasive assessment of the age related changes in stiffness of major branches of the human arteries. Cardiovasc Res. 1987;21:678–87. https://doi.org/10.1093/cvr/21.9.678.
Bots ML, Dijk JM, Oren A, Grobbee DE. Carotid intima-media thickness, arterial stiffness and risk of cardiovascular disease: current evidence. https://insights.ovid.com/pubmed?pmid=12473847. Accessed 17 Dec 2018.
Oren A, Vos LE, Uiterwaal CSPM, Grobbee DE, Bots ML. Aortic stiffness and carotid intima-media thickness: two independent markers of subclinical vascular damage in young adults? Eur J Clin Investig. 2003;33:949–54. https://doi.org/10.1046/j.1365-2362.2003.01259.x.
Godia EC, Madhok R, Pittman J, Trocio S, Ramas R, Cabral D, et al. Carotid artery distensibility: a reliability study. J Ultrasound Med. 2007;26:1157–65. https://doi.org/10.7863/jum.2007.26.9.1157.
Calderón-Garcidueñas L, Vincent R, Mora-Tiscareño A, Franco-Lira M, Henríquez-Roldán C, Barragán-Mejía G, et al. Elevated plasma endothelin-1 and pulmonary arterial pressure in children exposed to air pollution. Environ Health Perspect. 2007;115:1248–53. https://doi.org/10.1289/ehp.9641.
Zanoli L, Lentini P, Granata A, Gaudio A, Fatuzzo P, Serafino L, et al. A systematic review of arterial stiffness, wave reflection and air pollution. Mol Med Rep. 2017;15:3425–9.
Eeftens M, Tsai MY, Ampe C, Anwander B, Beelen R, Bellander T, et al. Spatial variation of PM2.5, PM10, PM2.5 absorbance and PMcoarse concentrations between and within 20 European study areas and the relationship with NO2 - results of the ESCAPE project. Atmos Environ. 2012;62:303–17.
Cohen AJ, Brauer M, Burnett R, Anderson HR, Frostad J, Estep K, et al. Estimates and 25-year trends of the global burden of disease attributable to ambient air pollution: an analysis of data from the global burden of diseases study 2015. Lancet (London, England). 2017;389:1907–18. https://doi.org/10.1016/S0140-6736(17)30505-6.
Funding
This study was supported by the Global Geo Health Data Centre from Utrecht University (https://globalgeohealthdatacenter.com/).
Availability of data and materials
The datasets generated and/or analysed during the current study are not publicly available due to the sensitive nature of the raw data but are available from the corresponding author on reasonable request.
Author information
Authors and Affiliations
Contributions
AN, DK, IV, DG, MD conceived the manuscript. AN wrote the initial draft and had the responsibility for submitting for publication. DK, IV, DG, MD, GH, OS, CU performed a critical revision of the manuscript. AN conducted the initial analyses. GH, CU provided important feedback on how the study can be improved. OS contributed to the air pollution concentration assignment. GD, CU, KE contributed with offering the WHISTLER data and providing information related to the data collection and measurements. All authors read and approved the final manuscript.
Corresponding author
Ethics declarations
Ethics approval and consent to participate
Written informed consent is obtained from all parents. The paediatric medical ethics committee of the University Medical Centre Utrecht has approved the protocol.
Consent for publication
Not applicable.
Competing interests
The authors declare that they have no actual or potential competing financial interests.
Publisher’s Note
Springer Nature remains neutral with regard to jurisdictional claims in published maps and institutional affiliations.
Additional files
Additional file 1:
Figure S1. Scatterplots showing the relationships between air pollution at the front door location (Cd) and exposures calculated using the time-weighted activity pattern (Ej), calculated from Eq. 1. a: the relationship between NO2 at front door location (Cd NO2) and NO2 after applying Eq.1 (ENO2); b: the relationship between NOx at front door location (Cd NOx) and NOx after applying Eq.1 (ENOx); c: the relationship between PM2.5 at front door location (Cd PM2.5) and PM2.5 after applying Eq.1 (EPM2.5); d: the relationship between PM10 at front door location (Cd PM10) and PM10 after applying Eq.1. (EPM10); e: the relationship between PM2.5absorbance at front door location (Cd PM2.5absorbance) and PM2.5absorbance after applying Eq.1. (EPM2.5absorbance). (PDF 298 kb)
Additional file 2:
Table S1. Regression slopes (95% CI) between air pollutants and cD for the fullest model (Model2b) stratified by sex. (DOCX 13 kb)
Rights and permissions
Open Access This article is distributed under the terms of the Creative Commons Attribution 4.0 International License (http://creativecommons.org/licenses/by/4.0/), which permits unrestricted use, distribution, and reproduction in any medium, provided you give appropriate credit to the original author(s) and the source, provide a link to the Creative Commons license, and indicate if changes were made. The Creative Commons Public Domain Dedication waiver (http://creativecommons.org/publicdomain/zero/1.0/) applies to the data made available in this article, unless otherwise stated.
About this article
Cite this article
Ntarladima, AM., Vaartjes, I., Grobbee, D.E. et al. Relations between air pollution and vascular development in 5-year old children: a cross-sectional study in the Netherlands. Environ Health 18, 50 (2019). https://doi.org/10.1186/s12940-019-0487-1
Received:
Accepted:
Published:
DOI: https://doi.org/10.1186/s12940-019-0487-1