Abstract
Background
Several unhealthy lifestyle behaviors in adolescence are often linked to overweight/obesity. Some of them may be present simultaneously, leading to combined effects on health. Therefore, the clustering of several unhealthy behaviors in adolescents might be associated with adiposity excess.
Purpose
To identify lifestyle patterns and analyze their association with adiposity in early adolescents.
Methods
A cross-sectional cluster analysis was performed in 1183 adolescents (50.5% girls) with a mean age of 12.5 (0.4) years included in the SI! Program for Secondary Schools in Spain to identify lifestyle patterns based on healthy diet, step counts, sleep time, and leisure screen time. Generalized mixed models were applied to estimate the association between lifestyle patterns and adiposity indices.
Results
Four lifestyle patterns were derived: Cluster 1-higher screen time and poorer diet (n = 213), Cluster 2-lower activity and longer sleepers (n = 388), Cluster 3-active and shorter sleepers (n = 280), and Cluster 4-healthiest (n = 302). Except for the number of steps (12,008 (2357) day), the lifestyle behaviors in our sample presented levels far below the recommendations, especially for sleep duration. Cluster 4 included the largest proportion of adolescents from high socioeconomic status families (47.7%) and the lowest prevalence of overweight/obesity (23.1%). Compared to Cluster 4-healthiest, adolescents in the remaining clusters presented a higher prevalence of overweight/obesity and central obesity, showing Cluster 3 the highest prevalences (PR:1.31 [95%CI: 1.31, 1.31] and PR:1.40 [95%CI: 1.33, 1.47]).
Conclusions
Clustering of lifestyle patterns in early adolescence allows the identification of individuals with excess adiposity, in whom health promotion strategies should be stressed, especially in socioeconomically disadvantaged groups.
Trial registration
Clinical Trial Registry, NCT03504059. Registered 20/04/2018—Retrospectively registered, https://clinicaltrials.gov/ct2/show/NCT03504059.
Similar content being viewed by others
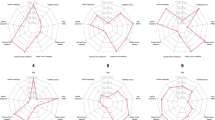
Introduction
According to the World Health Organization, the prevalence of overweight and obesity among children and adolescents has risen dramatically from just 4% to just over 18% [1]. Strong evidence suggests that childhood obesity is a risk to develop chronic diseases during adulthood like type 2 diabetes, cardiovascular (CV) diseases, cancer, and mental health disorders [2, 3]. Regarding specific lifestyle behaviors, there is established evidence in the literature showing that a sedentary lifestyle and high screen time are associated with unhealthier anthropometric indicators [4, 5], with an increased risk of overweight/obesity and their continuation into adulthood [6,7,8]. Sleep time and sleep quality also play an important role in obesity development [9,10,11,12]. Lifestyle habits are interrelated and linked to adiposity levels; it is well known that improving one component positively influences the rest [7, 10, 13]. Assessing the clustering of several behaviors considers the existence of multiple and interactive influences on lifestyles. This approach can facilitate understanding which behaviors are often combined and linked to obesity, and in which subgroups of the population prevention initiatives are most needed. The aim of this study was to identify and analyze the relationship between lifestyle patterns and adiposity in early adolescents included in the SI! Program for Secondary Schools (Salud Integral – Comprehensive Health) trial.
Materials and methods
Study design and sample
The SI! Program for Secondary Schools is a cluster-randomized controlled intervention trial (NCT03504059. Registered 20/04/2018—Retrospectively registered, https://clinicaltrials.gov/ct2/show/NCT03504059) involving 1326 adolescents attending public secondary schools in Barcelona and Madrid, Spain. The study was approved by the corresponding ethics committees, and all participants gave written informed consent. All details on recruitment and trial design have been published previously [14].
The present study included 1183 participants aged 11–14 years with complete baseline information (obtained in 2017) for the variables included in the lifestyle patterns analysis: diet, number of steps, sleep time, and leisure screen time.
Dietary intake
Adolescents answered a validated 43-item food frequency questionnaire (Children’s Eating Habits Questionnaire) [15, 16] related to their food consumption during the preceding 4 weeks. The questionnaire was guided by trained nutritionists and completed by the adolescents via an online application. Response options were ‘Never/less than once/week’, ‘1–3 times/week’, ‘4–6 times/week’, ‘1 time/day’, ‘2 times/day’, ‘3 times/day’, ‘ ≥ 4 times/day’, and ‘I have no idea’ [16]. Participants with more than 50% of answers missing or who answered ‘I have no idea’ to all questions were excluded from the analysis. For the analysis, a conversion factor ranging from 0 (‘I have no idea’ or ‘missing data’) to 30 (≥ 4 times/day’) was used to transform answers into weekly consumption frequencies [17]. For missing data in water intake, the data was imputed according to mean intake by age [17].
Based on this questionnaire, three dietary patterns (Processed, Traditional and Healthy) were derived by principal component analysis using a protocol previously described [18]. The Healthy diet pattern was selected as an overall indicator for healthy diet in the lifestyle clusters. It was characterized by high loadings on vegetables, fruits, unsweetened cereals and dairy, non-fried meat and fish, dried fruits and whole bread, and low loadings on sauces, white bread, chocolate, sweets, snacks, and sweetened drinks.
Step counts
Adolescents wore an accelerometer (Actigraph wGT3X-BT) on their non-dominant wrist for 7 consecutive days except when bathing or swimming. Step counts were estimated by the pedometers included in the devices. Records were considered valid if they provided data from a minimum of 4 days with at least 600 min per day of wear time [19].
Sleep time
Sleep time was assessed by accelerometry (Actigraph wGT3X-BT) according to the cutoff points proposed by Cole-Kripke et al. [20,21,22]. Sleep records were considered valid if they provided data from a minimum of 4 nights of wear time with a maximum of 960 min of sleep per night.
Screen time
Passive and active leisure screen time was assessed through two questions adapted from the Spanish National Health Survey [23]: 1) ‘How much leisure time per day do you spend watching TV/series/videos?’ for passive screen time, and 2) ‘How much leisure time per day do you spend playing with a computer/tablet/videogame…?’ for active screen time. Response options were ‘maximum 1 h/day’, ‘maximum 2 h/day’, ‘maximum 3 h/day’, or ‘ ≥ 3 h/day’, separately for weekdays and weekends. Daily screen hours were calculated as follows: total screen time = ((5 × passive screen time on weekdays + 2 × passive screen time on weekends)/7) + ((5 × active screen time on weekday + 2 × active screen time on weekends)/7).
Anthropometric measurements
All participants were instructed to fast overnight before measurements. Trained nutritionists measured participants’ weight and percentage of body fat through bioelectrical impedance analysis (OMRON BF511 body composition scale), height (Seca 213 stadiometer), and waist circumference (WC) (Holtain tape) [24]. Body mass index (BMI) was calculated as body weight divided by height squared (kg/m2). Fat mass was calculated by multiplying the percentage of body fat by weight (kg) and dividing by 100. Fat mass index (FMI) was calculated by dividing body fat mass by height squared (kg/m2). Waist-to-height ratio (WHtR) was calculated by dividing WC (cm) by height (cm). Age- and sex-adjusted z-scores were calculated according to the Center for Disease Control (CDC) standards for BMI [25]; and the Third National Health and Nutrition Examination Survey (NHANES III) cutoff points for WC and WHtR [26]. Specific age and sex FMI z-scores were calculated based on our sample. The participant age range was 11–14. Participants aged 13 and 14 (n = 88 and n = 12, respectively) were combined into a single age group (13 years) to calculate the age- and sex-specific z-scores of FMI. Maturation stage (from I to V) according to Tanner [27] was self-reported by participants with the support of pictograms. Missing values in anthropometric variables were not imputed.
Socioeconomic status
Socioeconomic information was reported through the family questionnaire. Household income was categorized into three levels based on the average annual household income in Spain in 2016 (26,730 €), the most recent data available at the time of recruitment [28] (‘below average’, ‘average’, and ‘above average’). Parental education level was categorized into three levels according to the International Standard Classification of Education (ISCED) [29]: low (secondary studies or below, ISCED levels 0 to 3), medium (postsecondary non-tertiary education or short-cycle tertiary education, ISCED levels 4 to 5), and high (university studies, ISCED levels 6 to 8) taking as a reference the highest educational level reported in the household. Migrant background was assumed when at least one of the parents was born outside Spain. Socioeconomic status was classified as unknown if no information was available.
Statistical methods
Cluster analysis was performed in order to construct groups or clusters ensuring that within a group the observations are as similar as possible, while observations belonging to different groups are as different as possible, based on four lifestyle variables: healthy diet, step counts, sleep time, and leisure screen time. Z-scores were calculated to standardize the variables and prevent some from having more weight than others in the final solution [18]. Lifestyle clusters were derived through a combination of hierarchical and non-hierarchical approaches [30, 31]. In brief, first, Ward’s method based on squared Euclidean distances was applied as a hierarchical cluster analysis, and several possible cluster solutions were identified and compared. Second, a non-hierarchical K-means cluster algorithm was applied with a predefined maximum 100 iterations to further refine the preliminary solution by optimizing the classification. Finally, the stability of the cluster solution was examined by randomly splitting the database into halves and repeating the same clustering procedure and comparing the solution stability (the kappa degree of concordance values for the first and second halves were 0.877 and 0.942, respectively). As a result of cluster analysis, a new categorical variable was generated, with as many categories as the number of clusters derived. Since clusters are mutually exclusive and each category corresponds to a cluster, participants are therefore included in a single category.
Statistical differences were identified by chi-square test for categorical variables and by analysis of variance (one-way ANOVA) for continuous variables and Bonferroni correction was used to adjust for multiple pairwise comparisons. Linear mixed-effect models were used to assess the association between lifestyle clustering and anthropometric indicators. Fixed effects were fasting status (yes/no), maturation stage (continuous, scale 1 to 5/unknow), migrant background (yes/no/unknow), and parental educational level (low/average/high/unknow). Region (Madrid or Barcelona) and schools were handled as random effects.
The associations between lifestyle patterns and overweight/obesity (z-BMI percentile ≥ 85th) or central obesity (z-WC percentile ≥ 90th) prevalence were assessed with generalized mixed models using a Poisson distribution with a log link and robust error variance. In these cases, the same fixed effects and random effects described above were applied.
Statistical significance was set at a threshold of p < 0.05. Dietary and lifestyle patterns derived by principal component analysis and cluster analysis, respectively, as well as the descriptive statistics, were carried out with SPSS Statistics version 23.0 (Armonk, NY: IBM Corp). Generalized mixed models were carried out using Stata version 15 (StataCorp, College Station, Texas).
Results
The analysis included a total of 1183 adolescents aged 12.5 (0.4) years (50.5% girls) from schools located in Barcelona (n = 818) and Madrid (n = 365).
Four lifestyle clusters were identified (Fig. 1 and Table 1). Participants in Cluster 1 (C1) (n = 213, 46.9% girls) showed the highest leisure screen time scores and the lowest scores in healthy diet. Participants in Cluster 2 (C2) (n = 388, 60.3% girls) only scored above average in sleep time and presented the lowest step counts. Participants in Cluster 3 (C3) (n = 280, 41.8% girls) presented the lowest sleep time score and the highest step counts score. Finally, Cluster 4 (C4) (n = 302, 48.3% girls) showed the healthiest lifestyle profile with scores above average in healthy diet, step counts and sleep time, and the lowest score in leisure screen time.
The clusters differed in all the evaluated lifestyle variables and in the socioeconomic characteristics, except in the migrant background (Table 1). Participants in C1 included the highest percentage of families with lower education (29.6%) and income (41.8%) levels, while C4 included a higher percentage of families with high socioeconomic status (47.4% of high parental education and 44.7% of high household income levels). Significant differences between clusters in the nutritional status were also observed, showing C3 the highest prevalence of overweight/obesity (31.4%) and C4 the lowest (23.1%).
The associations of lifestyle clusters with anthropometric indicators are shown in Fig. 2. Participants in C3 had the least favorable overall anthropometric profile, although significant differences were only found in z-WHtR against C2.
Mean z-scores and 95% confidence intervals for anthropometric variables by lifestyle behavior cluster. Estimated marginal mean z-scores (95% confidence intervals) obtained from multilevel linear mixed-effects models. Fixed-effect variables were fasting status, maturation stage, migrant background, and parental education level. Region and school were handled as random effects to account for the clustered study design. Cluster 1-higher screen time and poorer diet; Cluster 2-lower activity and longer sleepers; Cluster 3-active and shorter sleepers; and Cluster 4-healthiest. z-BMI, body mass index; z-WC, waist circumference; z-WHtR, waist-to-height ratio; z-FMI, fat mass index. Significant differences between clusters after Bonferroni correction (p < 0.05): * Cluster 2 vs Cluster 3
Adjusted models show that adolescents in C1, C2, and C3 showed a higher prevalence of overweight/obesity than the cluster with the healthiest lifestyle profile (C4): C1 PR: 1.12 (95% CI: 1.11, 1.13); C2 PR: 1.07 (95% CI: 1.02, 1.12); and C3 PR: 1.31 (95% CI: 1.31,1.31) (Table 2). C3 also showed a higher prevalence of central obesity than C4 (PR: 1.40 [95% CI: 1.33, 1.47]).
Discussion
In a large sample of early adolescents included in the SI! Program for Secondary Schools in Spain, four lifestyle clusters were derived from four key lifestyle behaviors (healthy diet, step counts, sleep time, and leisure screen time). Three of them (C1, C2, and C3) showed mixed behavioral profiles, while the C4 was nearest to a healthy profile. Except for the number of steps, which was above the recommendations, the other lifestyle behaviors described in the clusters presented levels far below recommendations. The clusters showed an unequal distribution of the participants with respect to the socioeconomic characteristics. Adolescents in C3-active and shorter sleepers had the most unfavorable anthropometric profile and the highest prevalence of overweight/obesity or central obesity; in contrast adolescents in C4-healthiest showed the most favorable body composition profiles. These findings are relevant and suggest that adolescents with relatively shorter sleep time were at particular high risk of presenting an unhealthier adiposity profile, even if they presented high activity levels.
Socioeconomic differences during childhood and adolescence are relevant, since their effect in lifestyle accumulates over time, broadening the gap in health inequalities.
Previous studies reported that adolescents with high parental education level are often allocated to healthier clusters (better diet quality, lower sedentary behavior, or higher physical activity levels), while adolescents with low parental education level are frequently allocated to less favorable clusters [7, 32]. Socioeconomic differences were confirmed in our study, with the healthier cluster having the largest proportion of high parental education and high household income levels.
The relationship between childhood and adolescent lifestyle patterns and health indicators has been widely described, although no consistent trends were found [6, 13, 30,31,32,33,34,35,36]. The literature shows that lifestyles play an important role in CV health [6, 13, 33, 35,36,37,38]. Our results showed that participants in C4-healthiest presented the most desirable lifestyle and the most favorable anthropometric profile. In contrast, C3-active and shorter sleepers presented the worst anthropometric profile. Regarding nutritional status, participants in all clusters, and mainly in C3, showed a higher probability of having central obesity and/or overweight/obesity than the participants in the healthiest cluster (C4). One of the possible explanations for the higher prevalence of overweight/obesity in C3 compared to C4, might be sleep time, due to the relatively large differences between these clusters. The association between short sleep duration and adverse CV health has been widely described in the literature [10, 30, 39, 40].
It is important to note that the results of cluster analysis are intrinsically related to the study sample and are limited by its behavioral levels, representing participants with better or worse healthy lifestyle profiles. In this sense, it is noteworthy that our sample presents unhealthy lifestyle habits as a whole, similarly to previous results related to lifestyle recommendations [35]. In our sample, a low percentage of adolescents met the recommendations for sleep (9-11 h/day: 4.8%), screen time (≤ 2 h/day: 6.3%), and fruit and vegetable intake (≥ 5 portions/day: 7.9%) [41,42,43], while physical activity was the only behavior showing predominantly adequate levels of performance with 78.9% of the sample accumulating ≥ 10,000 step counts/day [44]. Therefore, it is important to stress that clusters with the best scores for the selected behaviors do not necessarily reflect that the adolescents included therein met the recommendations (e.g., only 19.5% and 12.9% of participants in C4 met the fruit and vegetable consumption and screen time recommendations, respectively). Yet, the cluster solution was able to detect specific groups of adolescents at particular higher risk of presenting overweight/obesity according to four important lifestyle behaviors.
This study has some limitations. This cross-sectional analysis allows the establishment of associations, but not causation. Participation was voluntary, and we therefore cannot exclude selection bias and adolescents with worse CV health or unhealthier lifestyles might have been less willing to participate. In this analysis, only participants with complete information on the behaviors involved in the derived cluster solution were included, showing the excluded participants a differential sociodemographic distribution and thus resulting in a potential additional bias (Additional file 1). Another limitation is that early adolescents engaged in sports were often asked by coaches to remove their accelerometers during training and competition, resulting in potentially underestimated physical activity and therefore we decided to report step counts as an overall indicator of physical activity. Finally, the use of questionnaires introduces the possibility of social desirability bias and unreliability of estimated food intake frequencies or daily leisure screen time.
Strengths of the study include the status of the SI! Program for Secondary Schools as a large, longitudinal multi-center study conducted in early adolescents who were assessed through a solid combination of direct measurements and questionnaires, all carried out by trained nutritionists and nurses. Another strength is the use of wrist-worn accelerometers [21] that provide better sleep estimates for adolescents compared to waist-worn accelerometry data [45].
Conclusions
Family sociodemographic determinants were linked to the distribution of the four lifestyle clusters identified. Participants in the healthiest lifestyle pattern profile showed the lowest prevalence of overweight/obesity, while participants in the cluster with the relatively lowest sleep time showed the highest prevalence of overweight/obesity and central obesity. Nevertheless, except for the number of steps, the lifestyle behaviors described in the four clusters presented levels far from the recommended values; thus, finding differences between clusters that reflect the importance of complying with the overall lifestyle recommendations was not feasible. Follow-up of this study cohort will allow to assess the evolution of behaviors that appear to influence CV health in adolescents and the potential effects of a health promotion program.
Availability of data and materials
Data availability to external researchers is restricted to related project proposals upon request to the corresponding author. Based on these premises, deidentified participant data will be available with publication after approval of the proposal by the Steering committee and a signed data sharing agreement.
Abbreviations
- BMI:
-
Body mass index
- C1:
-
Cluster 1
- C2:
-
Cluster 2
- C3:
-
Cluster 3
- C4:
-
Cluster 4
- CDC:
-
Center for Disease Control
- CV:
-
Cardiovascular
- FMI:
-
Fat mass index
- ISCED:
-
International Standard Classification of Education
- NHANES III:
-
Third National Health and Nutrition Examination Survey
- SI!:
-
Salud Integral
- WC:
-
Waist circumference
- WHtR:
-
Waist-to-height ratio
References
WHO. Obesity and overweight WHO: 17–05–2017; 2020 [Available from: https://www.who.int/news-room/fact-sheets/detail/obesity-and-overweight.
Park MH, Falconer C, Viner RM, Kinra S. The impact of childhood obesity on morbidity and mortality in adulthood: a systematic review. Obes Rev. 2012;13(11):985–1000.
Quek YH, Tam WWS, Zhang MWB, Ho RCM. Exploring the association between childhood and adolescent obesity and depression: a meta-analysis. Obes Rev. 2017;18(7):742–54.
Crichton GE, Alkerwi A. Association of sedentary behavior time with ideal cardiovascular health: the ORISCAV-LUX study. PLoS ONE. 2014;9(6): e99829.
Leiva AM, Martinez MA, Cristi-Montero C, Salas C, Ramirez-Campillo R, Diaz Martinez X, et al. Sedentary lifestyle is associated with metabolic and cardiovascular risk factors independent of physical activity. Rev Med Chil. 2017;145(4):458–67.
Seral-Cortes M, Sabroso-Lasa S, Bailo-Aysa A, Gonzalez-Gross M, Molnar D, Censi L, et al. Mediterranean diet, screen-time-based sedentary behavior and their interaction effect on adiposity in European adolescents: the HELENA study. Nutrients. 2021;13(2):474.
Leech RM, McNaughton SA, Timperio A. The clustering of diet, physical activity and sedentary behavior in children and adolescents: a review. Int J Behav Nutr Phys Act. 2014;11:4.
Tarp J, Brond JC, Andersen LB, Moller NC, Froberg K, Grontved A. Physical activity, sedentary behavior, and long-term cardiovascular risk in young people: A review and discussion of methodology in prospective studies. J Sport Health Sci. 2016;5(2):145–50.
Agostinis-Sobrinho C, Gomez-Martinez S, Nova E, Hernandez A, Labayen I, Kafatos A, et al. Lifestyle patterns and endocrine, metabolic, and immunological biomarkers in European adolescents: The HELENA study. Pediatr Diabetes. 2019;20(1):23–31.
Borel AL. Sleep apnea and sleep habits: relationships with metabolic syndrome. Nutrients. 2019;11(11):2628.
Hense S, Pohlabeln H, De Henauw S, Eiben G, Molnar D, Moreno LA, et al. Sleep duration and overweight in European children: is the association modified by geographic region? Sleep. 2011;34(7):885–90.
Sparano S, Lauria F, Ahrens W, Fraterman A, Thumann B, Iacoviello L, et al. Sleep duration and blood pressure in children: Analysis of the pan-European IDEFICS cohort. J Clin Hypertens (Greenwich). 2019;21(5):572–8.
Leech RM, McNaughton SA, Timperio A. Clustering of diet, physical activity and sedentary behaviour among Australian children: cross-sectional and longitudinal associations with overweight and obesity. Int J Obes (Lond). 2015;39(7):1079–85.
Fernandez-Jimenez R, Santos-Beneit G, Tresserra-Rimbau A, Bodega P, de Miguel M, de Cos-Gandoy A, et al. Rationale and design of the school-based SI! Program to face obesity and promote health among Spanish adolescents: A cluster-randomized controlled trial. Am Heart J. 2019;215:27–40.
Bel-Serrat S, Mouratidou T, Pala V, Huybrechts I, Börnhorst C, Fernández-Alvira JM, et al. Relative validity of the Children’s Eating Habits Questionnaire-food frequency section among young European children: the IDEFICS Study. Public Health Nutr. 2014;17(2):266–76.
Lanfer A, Hebestreit A, Ahrens W, Krogh V, Sieri S, Lissner L, et al. Reproducibility of food consumption frequencies derived from the Children’s Eating Habits Questionnaire used in the IDEFICS study. Int J Obes (Lond). 2011;35(Suppl 1):S61–8.
Fernández-Alvira JM, Börnhorst C, Bammann K, Gwozdz W, Krogh V, Hebestreit A, et al. Prospective associations between socio-economic status and dietary patterns in European children: the Identification and Prevention of Dietary- and Lifestyle-induced Health Effects in Children and Infants (IDEFICS) Study. Br J Nutr. 2015;113(3):517–25.
Bodega P, Fernández-Alvira JM, Santos-Beneit G, de Cos-Gandoy A, Fernández-Jiménez R, Moreno LA, et al. Dietary patterns and cardiovascular risk factors in Spanish adolescents: a cross-sectional analysis of the SI! Program for health promotion in Secondary Schools. Nutrients. 2019;11(10):2297.
Rich C, Geraci M, Griffiths L, Sera F, Dezateux C, Cortina-Borja M. Quality control methods in accelerometer data processing: defining minimum wear time. PLoS ONE. 2013;8(6): e67206.
Cole RJ, Kripke DF, Gruen W, Mullaney DJ, Gillin JC. Automatic sleep/wake identification from wrist activity. Sleep. 1992;15(5):461–9.
Kripke DF, Hahn EK, Grizas AP, Wadiak KH, Loving RT, Poceta JS, et al. Wrist actigraphic scoring for sleep laboratory patients: algorithm development. J Sleep Res. 2010;19(4):612–9.
Quante M, Kaplan ER, Cailler M, Rueschman M, Wang R, Weng J, et al. Actigraphy-based sleep estimation in adolescents and adults: a comparison with polysomnography using two scoring algorithms. Nat Sci Sleep. 2018;10:13–20.
Encuesta Nacional de Salud de España 2017: Ministerio de Sanidad, Consumo y Bienestar Social; 2018 [Available from: https://www.mscbs.gob.es/estadEstudios/estadisticas/encuestaNacional/encuesta2017.htm.
Santos-Beneit G, Sotos-Prieto M, Pocock S, Redondo J, Fuster V, Penalvo JL. Association between anthropometry and high blood pressure in a representative sample of preschoolers in madrid. Rev Esp Cardiol (Engl Ed). 2015;68(6):477–84.
Fryar CD, Gu Q, Ogden CL. Anthropometric reference data for children and adults: United States, 2007–2010. Vital Health Stat 11. 2012;252:1–48.
Sharma AK, Metzger DL, Daymont C, Hadjiyannakis S, Rodd CJ. LMS tables for waist-circumference and waist-height ratio Z-scores in children aged 5–19 y in NHANES III: association with cardio-metabolic risks. Pediatr Res. 2015;78(6):723–9.
Tanner JM, Whitehouse RH. Clinical longitudinal standards for height, weight, height velocity, weight velocity, and stages of puberty. Arch Dis Child. 1976;51(3):170–9.
I.N.E: Instituto Nacional de Estadística. Encuesta anual de estructura salarial [Available from: https://www.ine.es/jaxiT3/Datos.htm?t=9948.
UNESCO. International Standard Classification of Education, ISCED 2011. Montreal: UNESCO Institute for Statistics; 2012.
Perez-Rodrigo C, Gil A, Gonzalez-Gross M, Ortega RM, Serra-Majem L, Varela-Moreiras G, et al. Clustering of dietary patterns, lifestyles, and overweight among Spanish children and adolescents in the ANIBES Study. Nutrients. 2015;8(1):11.
Bel-Serrat S, Ojeda-Rodriguez A, Heinen MM, Buoncristiano M, Abdrakhmanova S, Duleva V, et al. Clustering of multiple energy balance-related behaviors in school children and its association with overweight and obesity-WHO European Childhood Obesity Surveillance Initiative (COSI 2015–2017). Nutrients. 2019;11(3):511.
Ottevaere C, Huybrechts I, Benser J, De Bourdeaudhuij I, Cuenca-Garcia M, Dallongeville J, et al. Clustering patterns of physical activity, sedentary and dietary behavior among European adolescents: The HELENA study. BMC Public Health. 2011;11:328.
Dumuid D, Olds T, Lewis LK, Martin-Fernandez JA, Barreira T, Broyles S, et al. The adiposity of children is associated with their lifestyle behaviours: a cluster analysis of school-aged children from 12 nations. Pediatr Obes. 2018;13(2):111–9.
Sevil-Serrano J, Aibar-Solana A, Abos A, Julian JA, Garcia-Gonzalez L. Healthy or unhealthy? The cocktail of health-related behavior profiles in Spanish adolescents. Int J Environ Res Public Health. 2019;16(17):3151.
Santaliestra-Pasías AM, Mouratidou T, Reisch L, Pigeot I, Ahrens W, Mårild S, et al. Clustering of lifestyle behaviours and relation to body composition in European children. The IDEFICS study. Eur J Clin Nutr. 2015;69(7):811–6.
Bel-Serrat S, Mouratidou T, Santaliestra-Pasias AM, Iacoviello L, Kourides YA, Marild S, et al. Clustering of multiple lifestyle behaviours and its association to cardiovascular risk factors in children: the IDEFICS study. Eur J Clin Nutr. 2013;67(8):848–54.
Veloso SM, Matos MG, Carvalho M, Diniz JA. Psychosocial factors of different health behaviour patterns in adolescents: association with overweight and weight control behaviours. J Obes. 2012;2012: 852672.
van der Sluis ME, Lien N, Twisk JW, Steenhuis IH, Bere E, Klepp KI, et al. Longitudinal associations of energy balance-related behaviours and cross-sectional associations of clusters and body mass index in Norwegian adolescents. Public Health Nutr. 2010;13(10A):1716–21.
Garaulet M, Ortega FB, Ruiz JR, Rey-López JP, Béghin L, Manios Y, et al. Short sleep duration is associated with increased obesity markers in European adolescents: effect of physical activity and dietary habits. The HELENA study Int J Obes (Lond). 2011;35(10):1308–17.
de Lima TR, Silva DAS. Association of sleep quality with sociodemographic factors and lifestyle in adolescents from southern Brazil. World J Pediatr. 2018;14(4):383–91.
Hirshkowitz M, Whiton K, Albert SM, Alessi C, Bruni O, DonCarlos L, et al. National Sleep Foundation’s sleep time duration recommendations: methodology and results summary. Sleep Health. 2015;1(1):40–3.
CoP E. Children, Adolescents, and Television. Pediatrics. 2001;107(2):423–6.
World Health Organization. Office of World Health R. The World health report. reducing risks, promoting healthy life : overview. Geneva: World Health Organization; 2002. p. 2002.
Tudor-Locke C, Craig CL, Beets MW, Belton S, Cardon GM, Duncan S, et al. How many steps/day are enough? for children and adolescents. Int J Behav Nutr Phys Act. 2011;8:78.
Chandler JL, Brazendale K, Beets MW, Mealing BA. Classification of physical activity intensities using a wrist-worn accelerometer in 8–12-year-old children. Pediatr Obes. 2016;11(2):120–7.
Acknowledgements
The authors thank the SHE Foundation (intellectual owner of the SI! Program), especially Isabel Carvajal, Domènec Haro, Vanesa Carral, and Carla Rodríguez, and all the staff who helped with data collection. We also especially thank all participating adolescents and their families, teachers, and schools. Simon Bartlett (CNIC) provided English editing.
Funding
The SI! Program for Secondary Schools trial was supported by the SHE Foundation- “la Caixa” Foundation under the agreement LCF/PR/CE16/10700001 and the Fundació la Marató de TV3 (grant 369/C/2016). GSB is recipient of grant LCF/PR/MS19/12220001 funded by “la Caixa” Foundation (ID 100010434). RFJ is recipient of grants PI19/01704 and PI22/01560 funded by the Instituto de Salud Carlos III (ISCIII) and co-funded by the European Union. ATR is a Serra Húnter Fellow. JMG was a postgraduate fellow of the Ministerio de Ciencia e Innovación at the Residencia de Estudiantes (2020–2022) and is a recipient of grant FPU21/04891 (Ayudas para la formación de profesorado universitario, FPU-2021) from the Ministerio de Educación, Cultura y Deporte. EPLS is a FI-SDUR (EMC/3345/2020) fellowship from the Generalitat de Catalunya. Support was also provided by the Ministerio de Ciencia e Innovación (MCIN) (grant PID2020-114022RB-I00), and Generalitat de Catalunya. The CNIC is supported by the ISCIII, the MCIN, and the Pro CNIC Foundation, and is a Severo Ochoa Center of Excellence grant CEX2020-001041-S funded by MICIN/AEI/10.13039/501100011033.
Author information
Authors and Affiliations
Contributions
JMFA, RFJ, MdM, ATR, SLRG, EPLS, CAR, RE, RMLR, and GSB coordinated data collection and measurements. MdM and XO coordinated the implementation of the intervention. PB, JMFA, JMG, and AdCG conducted statistical analyses. RFJ and LAM provided scientific support over the course of this work. PB drafted the first version of the manuscript along with JMFA and GSB. All authors revised the manuscript critically for intellectual content and approved the published version.
Corresponding authors
Ethics declarations
Ethics approval and consent to participate
The SI! Program trial was conducted in accordance to the Declaration of Helsinki. The study was approved by the Committee for Ethical Research (CEI) of the Instituto de Salud Carlos III in Madrid (CEI PI 35_2016) and by the CEI of the Fundació Unió Catalana d'Hospitals in Barcelona (CEI 16/41) and the University of Barcelona Bioethics Committee (IRB00003099). Informed consent was obtained from the legally acceptable representatives (LAR)/parents of all participants in the study.
Consent for publication
Not applicable.
Competing interests
The authors declare that they have no conflict of interest.
Additional information
Publisher’s Note
Springer Nature remains neutral with regard to jurisdictional claims in published maps and institutional affiliations.
Supplementary Information
Rights and permissions
Open Access This article is licensed under a Creative Commons Attribution 4.0 International License, which permits use, sharing, adaptation, distribution and reproduction in any medium or format, as long as you give appropriate credit to the original author(s) and the source, provide a link to the Creative Commons licence, and indicate if changes were made. The images or other third party material in this article are included in the article's Creative Commons licence, unless indicated otherwise in a credit line to the material. If material is not included in the article's Creative Commons licence and your intended use is not permitted by statutory regulation or exceeds the permitted use, you will need to obtain permission directly from the copyright holder. To view a copy of this licence, visit http://creativecommons.org/licenses/by/4.0/. The Creative Commons Public Domain Dedication waiver (http://creativecommons.org/publicdomain/zero/1.0/) applies to the data made available in this article, unless otherwise stated in a credit line to the data.
About this article
Cite this article
Bodega, P., Santos-Beneit, G., de Cos-Gandoy, A. et al. Clustering of lifestyle behaviors and adiposity in early adolescents in Spain: findings from the SI! Program for Secondary Schools. BMC Public Health 23, 1535 (2023). https://doi.org/10.1186/s12889-023-16461-6
Received:
Accepted:
Published:
DOI: https://doi.org/10.1186/s12889-023-16461-6