Abstract
Background
Perturbation-based balance training on a treadmill is an emerging method of gait stability training with a characteristic task nature that has had positive and sustained effects on balance recovery strategies and fall reduction. Little is known about the effects produced by shod and barefoot walking. We aimed to investigate which is more appropriate, shod or barefoot walking, for perturbation-based balance training in older adults.
Methods
Fourteen healthy older adults (age: 68.29 ± 3.41 years; body height: 1.76 ± 0.10 m; body mass: 81.14 ± 14.52 kg) performed normal and trip-like perturbed walking trials, shod and barefoot, on a treadmill of the Gait Real-time Analysis Interactive Lab. The marker trajectories data were processed by Human Body Model software embedded in the Gait Offline Analysis Tool. The outcomes of stride length variability, stride time variability, step width variability, and swing time variability were computed and statistically analyzed by a two-way repeated-measures analysis of variance (ANOVA) based on gait pattern (normal gait versus perturbed recovery gait) and footwear condition (shod versus barefoot).
Results
Footwear condition effect (p = 0.0310) and gait pattern by footwear condition interaction effect (p = 0.0055) were only observed in swing time variability. Gait pattern effects were detected in all four outcomes of gait variability.
Conclusions
Swing time variability, independent of gait speed, could be a valid indicator to differentiate between footwear conditions. The lower swing time variability in perturbed recovery gait suggests that barefoot walking may be superior to shod walking for perturbation-based balance training in older adults.
Similar content being viewed by others
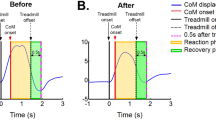
Background
Falls during walking put older adults at risk of serious injuries, e.g., fractures, brain injuries, or even death [1,2,3]. Trips are the most prevalent known cause of outdoor falls [4] and are considered to be the most challenging of falls [5, 6].
Due to the task-specific nature of improving gait stability in older adults at risk of falls in daily life [3, 7], perturbation-based balance training has been applied to simulate the occurrence of falls in a real environment [8, 9]. This form of training has produced positive and sustained effects on balance recovery strategies and on reducing falls in older adults and those with gait disorders [8, 10]. However, to our knowledge, researchers have not yet fully explored footwear condition (shod versus barefoot) as an independent variable that may affect the training effect. To date, clear evidence on whether shod or barefoot walking is preferable in perturbation-based balance training is still pending.
Footwear has a direct effect on gait performance [11,12,13], which has led to the popularity of barefoot locomotion (walking and running) in recent years and has evoked an increasing scientific interest in its benefits and limitations [14]. Typically, a well-fitting standard shoe with laces, a low and wide heel, a firm heel collar, and a grooved, moderately hard sole is recommended for older adults in rehabilitation and daily use [11]. However, footwear interferes with balance and, as a result, the risk of slipping, tripping, and falls by varying somatosensory feedback to the foot and ankle and altering frictional conditions at the shoe/floor interface [15], which has been demonstrated to hinder kinesthesia [16]. Due to the structural limitations of the shoe, forefoot spreading under load can be reduced [13]. As the sole thickness increases, neutral running footwear can significantly increase the activation of the peroneus longus muscle and interfere more with ankle stability [17]. An elevated heel of only 4.5 cm height significantly impairs balance in older adults whereas a high heel collar and a hard sole showed trends towards being beneficial [18]. Minimalist shoes improve dynamic stability in older adults better than barefoot [19], while it remains to be investigated whether it is superior to conventional footwear [20]. A recent study highlights barefoot walking has clinical potential based on gait stability and variability outcomes in both young and older adults [12]. Compared to normal gait, perturbated gait is more challenging and the essence lies in stability control. It has been suggested that gait variability, i.e., the coefficient of variation or standard deviation of spatiotemporal parameters, may better reflect subtle changes in natural gait fluctuations [21, 22], as it is a unique indicator of walking control [23] and maybe a direct predictor of falls in older adults [24,25,26,27]. The smaller the gait variability, the higher the gait stability [24, 27]. An increase in gait variability is also a clinically relevant biomarker for fall risk diagnosis [28, 29].
Therefore, we aimed to investigate whether gait variability parameters associated with falls remain consistent in healthy older adults during perturbation-based balance training while shod and barefoot walking to determine which footwear condition is more appropriate. We proposed the following hypothesis that barefoot walking could be more stable in both normal and perturbed recovery gait.
Methods
Participants
A total of 14 community-dwelling healthy older adults (age: 68.29 ± 3.41 years; body height: 1.76 ± 0.10 m; body mass: 81.14 ± 14.52 kg; Body Mass Index, BMI: 26.18 ± 5.00 kg/m2; Timed Up and Go test, TUG: 10.21 ± 1.21 s; 4 females) were recruited to participate in this study. The 14 sample sizes were selected based on a priori statistical power analysis obtained with G*Power v3.1.9.7, using a two-factor repeated measures analysis of variance (ANOVA) within interaction (α = 0.05, 1-β = 0.80, effect size f = 0.50, correlation among repeated measures = 0.50). The inclusion criteria for participants were no history of lower extremity disorders or injuries as well as no foot problems and gait abnormalities like spastic, scissors, steppage, waddling, and propulsive gait. The dominant leg was right. Participants were asked to wear tight-fitting athletic shorts and T-shirts provided by the investigation, as well as their own appropriate neutral running shoes. Written consent was obtained after comprehensive information before the test was conducted. Ethical approval was granted by the committee of the Rostock University Medical Center, Germany (A2019-0231). The Declaration of Helsinki was followed in all measurements.
Measurement protocol
The Gait Real-time Analysis Interactive Lab (GRAIL, Motek Medical B.V., Houten, the Netherlands), with an integrated 10-camera motion capture system (Vicon Metrics Ltd., Oxford, United Kingdom), was utilized to capture the three-dimensional marker trajectories in a virtual reality environment at a sampling frequency of 100 Hz (Fig. 1A). The D-Flow software v3.34 (Motek Medical B.V., Houten, the Netherlands) was used for signal triggering and data acquisition. According to the Human Body Model 2 (HBM2, Motek Medical B.V., Houten, the Netherlands), 26 reflective markers were attached to the anatomical bone landmarks. A total of four trials were performed for each participant, including normal and trip-induced walking with shod and barefoot condition, respectively (Fig. 1B). Thus, gait patterns included both normal and perturbed gait as well as footwear conditions including shod and barefoot walking in this study. An adequate break (approximately 5 min) was given between each trial until the participant felt ready to continue with the next test [19]. A safety rope system was used throughout the test to prevent potential falls of participants.
Investigation scenario for this study using the Gait Real-time Analysis Interactive Lab (A); Experimental protocol of the trip-like perturbation training: the protocol consisted of two sessions of shod and barefoot walking, for a total of four trials. Each session started with a 6-min familiarization during which the participant’s self-selected speed was recorded. The first trial was performed by walking shod at a self-selected speed for 3 min, and the second trial was performed with 6 blocks of trip perturbation with an interval of 15–20 s between each. The third and fourth trials of barefoot walking were performed with the exact process as shod walking (B); Schematic diagram of spatiotemporal parameters of the gait cycle, trip perturbation occurrence step, and the first recovery step (C); Trip perturbation is where the belt decelerates at 3 m/s.2 at normal gait speed until the speed is reduced by almost 1.2 m/s and held for 300 ms, and then accelerates to normal gait speed. Schematic diagrams are the curves generated for participant with an average gait speed of 1.35 m/s (shod, black dashed line) and 1.32 m/s (barefoot, gray solid line) with the x and y axes representing the time and speed offset, respectively (D)
A familiarization period of 6 min was maintained before the formal measurements in shod and barefoot, respectively [30,31,32]. During this period, the average of the participant's two self-reported comfortable speeds was used as normal speed [6]. After the self-selected speed was determined, normal walking was performed for three minutes each and trip-induced perturbed walking was performed six times each in the subsequent four trials. The timing of the occurrence of the perturbations was pseudo-random, with intervals of 15–20 s. The novel trip-like perturbation induced by treadmill belt deceleration was automatically delivered by a custom-made program written in the Lua language based on D-Flow software with an intensity of 3 m/s2. The perturbation occurred at the right heel strike (Fig. 1C) and lasted for 300 ms, then returned to normal speed (Fig. 1D), as described in our latest study [33].
Data processing
The data involved were processed using the HBM software embedded in the Gait Offline Analysis Tool (GOAT v4.1, Motek Medical, B.V., the Netherlands). The three-dimensional coordinates of the markers were filtered with a second-order low pass Butterworth (zero-phase) at a 6 Hz cutoff frequency [34, 35]. The local maxima in the anterior–posterior position of the heel marker relative to the pelvis was used to identify the heel-strike and toe-off events [36]. The gait cycle is defined as shown in Fig. 1. The following outcome gait parameters were identified via the data extraction process: stride length, stride time, step width, and swing time. Gait variability was defined as the coefficient of variation (CoV) calculated as the standard deviation (SD) divided by the mean value [25, 37].
The first recovery step (Rec1) following perturbation is the most important protective strategy [38, 39], where humans can quickly regain stability and maintain balance from the same type of perturbation [40]. To eliminate the unknown anticipation of the body proprioceptive response to the first perturbation, the Rec1 of the 2nd-6th perturbation was processed and averaged. For normal walking, 25 consecutive strides were averaged for each participant [28, 41].
Statistical analysis
GraphPad Prism v9.3.1 (GraphPad Software Inc., San Diego, CA, USA) was utilized for all statistical analysis. Normality was checked for each variable using the D’Agostino-Pearson test. The Wilcoxon signed rank test and paired t-test were performed to analyze the statistical differences in normal gait speed and perturbed recovery gait speed in shod and barefoot walking, respectively. A two-way repeated-measures ANOVA with gait pattern and footwear condition as factors, based on a General Linear Model, was applied to identify differences in gait variability [19]. When a significant main effect or factor interaction effect was found, a Tukey’s post hoc analysis with individual variances was performed to correct for multiple comparison tests. Each p value was adjusted to account for any pairwise comparisons. The significance level was set at p < 0.05.
Results
Gait speeds for normal gait and the first recovery step following trip perturbation when walking shod and barefoot are presented in Table 1. There was a significant decrease in barefoot walking speed compared to shod walking in both normal (p = 0.0103) and trip-Rec1 gaits (p < 0.0001).
The effects of gait pattern and footwear condition on stride length variability, stride time variability, step width variability, and swing time variability in older adults are demonstrated in Fig. 2.
Effects of gait pattern (normal versus trip-Rec1) and footwear condition (shod versus barefoot walking) on stride length variability (A), stride time variability (B), step width variability (C), and swing time variability (D). The interleaved scatter with bars and error bars indicates mean values and standard deviation, showing with the individual values. Variability was defined as the coefficient of variation (CoV, %) calculated as the standard deviation divided by the mean value. Gait pattern effect, footwear condition effect and the interaction effect between the two are shown at the top of each plot. Asterisks represent p-value classification for Tukey’s post hoc multiple comparisons. *: p < 0.05, **: p < 0.01; ****: p < 0.0001
The results of two-way repeated measures ANOVA revealed significant gait pattern effects in terms of the outcomes of stride length variability (F(1,13) = 51.24, p < 0.0001, η2p = 0.4253, Fig. 2a), stride time variability (F(1,13) = 32.47, p < 0.0001, η2p = 0.3752, Fig. 2B), step width variability (F(1,13) = 5.180, p = 0.0404, η2p = 0.0223, Fig. 2C), and swing time variability (F(1,13) = 14.94, p = 0.0019, η2p = 0.1456, Fig. 2D). Tukey’s post hoc tests for multiple comparisons revealed that increased stride length and stride time variability both in shod and barefoot walking, and increased swing time variability in shod walking were observed in trip_Rec1 relative to normal gait (Fig. 2A–D). This indicates that older adults have an increased likelihood of falling during trip_Rec1 compared to normal gait. However, no significant differences for step width were found in Tukey’s post hoc multiple comparisons (Fig. 2C).
No significant footwear condition effects and gait pattern by footwear condition interaction effects were found for stride length variability (F(1,13) = 3.978, p = 0.0675, η2p = 0.0324; F(1,13) = 4.143, p = 0.0627, η2p = 0.0306, Fig. 2A), stride time variability (F(1,13) = 0.1250, p = 0.7293, η2p = 0.1790; F(1,13) = 0.0033, p = 0.9551, η2p = 0.00004, Fig. 2B), or step width variability (F(1,13) = 0.2.431, p = 0.1430, η2p = 0.0411; F(1,13) = 0.9052, p = 0.3587, η2p = 0.0156, Fig. 2C).
Significant footwear condition effect as well as gait pattern by footwear condition interaction effect were only found in swing time variability (F(1,13) = 5.852, p = 0.031, η2p = 0.0632 and F(1,13) = 11.06, p = 0.006, η2p = 0.0829, Fig. 2D). Tukey’s post hoc analysis revealed significant differences in swing time variability between shod walking and barefoot walking in trip_Rec1 gait (Fig. 2D). Specifically, lower swing time variability (better) in barefoot walking in trip_Rec1 gait was observed.
Discussion
We examined stride length variability, stride time variability, step width variability, and swing time variability, between shod and barefoot walking in normal and trip-Rec1 gait among older adults during perturbation-based balance training. The main findings of the present study were, that stride length, stride time, and step width variability all had gait pattern main effects. Swing time variability had both gait pattern, footwear condition main effects and gait pattern by footwear condition interaction effects.
Significant differences in stride length, stride time, step width and swing time variability were observed across normal gait versus trip_Rec1 gait. Variability of spatiotemporal parameters provides a feasible approach for a quantitative assessment of gait stability [42]. A larger relative level of variability was indicated by a higher CoV [25, 43]. Significantly increased gait variability, as a response to perturbations, might be associated with poorer stability of the recovery step following a perturbation [25, 44]. To some extent, it indicated that the trip perturbation setup in this study was sufficient to cause an unstable recovery gait and even induce the risk of falls. Although no real cases of falls occurred in any of the participants during the investigation, these results seem to demonstrate that even minor external perturbation magnitudes can elicit stepping reactions in older adults and these responses are highly comparable to responses elicited by larger perturbation magnitudes [45], leading to a significant increase in gait variability of spatiotemporal parameters. These results provided a prerequisite for demonstrating the proposed hypothesis, i.e., whether the footwear condition of shod and barefoot walking induced gait variability in the same gait patterns of normal or trip-Rec1 gait.
In normal gait, barefoot walking could reduce the risk of falling [12] according to gait variability measurements [46]. However, no differences were observed in stride length variability, stride time variability, and step width variability between shod and barefoot walking either in normal or trip_Rec1 gaits in the present study. Footwear condition differed significantly only in swing time variability of trip_Rec1 gait as well as footwear condition by gait pattern, showing a smaller CoV in the case of barefoot walking.
Higher stride length variability is an important indicator of poor balance mechanisms, indicating the body’s inability to improve or recover from future fall-related events [47]. Irrespective of single-task, motor dual-task, or cognitive dual-task, barefoot walking results in a significantly slower gait pattern accompanied by increased stride length variability compared to standard shod walking [11]. These researchers suggested that barefoot walking is not recommended for older women since it could have a detrimental effect on gait patterns [11]. Separately, a recent study on barefoot and minimalist shoe walking showed a significant effect of stride length variability in both young and older adults with footwear condition. Stride length variability was significantly lower in the minimalist shoe condition [19]. The effect of footwear on stride length was not equivalent [48]. Our findings showed no statistical difference in stride length variability between shod and barefoot walking. Both of the above-mentioned studies were conducted overground, while our study was performed on a treadmill. Two recent systematic reviews indicate that saptiotemporal outcomes for treadmill and overground locomotion (walking and running) are essentially equivalent [49, 50], therefore, further studies are still required to confirm the equivalence of the stride length variability when walking on different conditions.
An increase in stride time variability indicates that the gait pattern is less rhythmic [51]. Stride time variability in our study was not consistent with a recent study [12], from which it is known that stride time variability in older adults when overground barefoot walking is lower than in those shod walking. It has been suggested that stride time variability increases with decreasing gait speed [28]. However, some studies concluded that there is no negative correlation between the two [52]. Our data were consistent with this study. Barefoot walking speed decreased significantly compared to shod walking for both normal and recovery gait, but there was no significant difference in stride time variability. Stride time variability may be the result of a multifactorial interaction, such as muscle strength, balance function, and gait speed [25]. Nevertheless, the relationship with footwear conditions has not been determined. From our data, we suspect that gait training on a treadmill with or without shoes has no direct effect on this metric.
Step width variability represents the more sensitive descriptor of locomotion control of older and young adults [53, 54]. As an indicator of the required active control [55], inaccurate control is likely to lead to increased step width variability in older adults [54]. The criteria for the step width variability were classified as: low, < 7–8%; medium, 8–27%; and high, > 27–30% according to Skiadopoulos et al. [54]. Older adults with extreme step width variability have a higher frequency of falls, specifically explained by the fact that individuals with too low step width variability may lack the necessary skills to adjust their step width to maintain balance, while too high step width variability indicates unsteady walking in clinical practice [56]. Our results are in the middle range in both shod and barefoot walking. It has been reported that footwear use is an influential factor in gait variability in healthy older adults, and habitual footwear increases step width variability [57]. Our findings were inconsistent with those described above. No statistical differences were found for step width variability between footwear condition for either normal or trip-Rec1. Although the participants were all similarly aged older adults, gait speed was not consistent. One study reported that step width variability was associated with falls in older adults walking at near-normal speeds, but not in those with gait speeds below 1.0 m/s [56]. Whether the inconsistency in results was related to gait speed requires further investigation.
Swing time variability was the only one of the four variables of gait variability we investigated that had both the main effect of footwear condition and interaction effect between footwear condition by gait pattern. This is not consistent with some of the results of a previous study. Grabiner et al. [58] reported that swing time variability was typically affected by disease and degree of aging, but does not appear to be affected by the subject’s age, gait speed, or the presence of shoes. Another controlled study of Parkinson patients versus a healthy population confirmed the finding that swing time variability was not affected by gait speed [23], which could be a speed-independent predictor of stability and fall risk [23]. Swing time variability may be mainly determined by the balance control mechanism [59]. If so, we could speculate that swing time variability on treadmill gait perturbation measurements may be a useful indicator to explore gait stability control mechanisms under conditions of constant, controlled or variable gait speed, as well as to gain insight into whether it is influenced by other external factors, such as footwear condition, perturbation intensity, etc. Our hypothesis was also partially confirmed, namely, that swing time variability could be an important dependent variable in differentiating footwear condition during perturbation-based balance training on a treadmill.
Our study is not without limitations. Firstly, our findings may only be generalizable to a non-habitually barefoot older population. The different foot-strike patterns of habitual barefoot and non-habitual barefoot may lead to differences in the spatiotemporal parameters of gait [13]. However, this seems to be of only minor importance for the question of whether training is better with shoes or without. In the present experimental design, all participants wore well-fitting neutral running shoes. A monolithic form of footwear was compared to walking barefoot, which may limit the applicability of the findings to diverse footwear [12]. Gait variability was reduced when walking in minimalist shoes compared to walking barefoot, with equivalent age group effects [19]. Moreover, different footwear has differential sole thickness and hardness, which may affect the balance of older adults [60]. Future studies will be necessary to include multifunctional footwear or socks-only versus barefoot conditions depending on the specific study population, such as patients with foot injuries or gait abnormalities, to differentiate the applicability of footwear condition for perturbation-based balance training on a treadmill. Secondly, the number of first recovery steps after perturbation included in the study was relatively small. However, to date, the number of steps/strides required to reach reliability of gait variability is still under exploration [61]. Moreover, the number of steps required varies for different indicators of spatiotemporal parameter variability [62], ranging from less than six to more than several hundred steps [28, 62,63,64]. It has been revealed that a single familiarization trial can significantly improve the reliability of gait variability in older adults [31, 65], which could take as little as 6 min on the treadmill [28, 32]. Last but not least, “our experimental design was to apply perturbation-based balance training to simulate the likelihood of a fall occurring” does not seem to hold, as no falls were observed. Further studies on trip perturbations leading to falls are needed to confirm our findings.
Conclusion
Swing time variability, independent of gait speed, could be a valid indicator to differentiate between footwear condition. The lower swing time variability in perturbed recovery gait suggests that barefoot walking might be superior to shod walking for perturbation-based balance training in older adults. Whether in a clinical setting or in biomechanics, swing time variability based on spatiotemporal parameters is an easy-to-measure, easy-to-assess approach that is likely to have promising applications as an assessment of footwear conditions in various populations at risk of falls to evaluate the effects of perturbation-based balance training.
Availability of data and materials
The datasets generated during the current study are available from the corresponding author upon reasonable request.
Abbreviations
- CoV:
-
Coefficient of variation
- Rec1:
-
The first recovery step
- Trip_Rec1:
-
The first recovery step following trip perturbation
- ANOVA:
-
Analysis of variance
- SD:
-
Standard deviation
- GOAT:
-
Gait Offline Analysis Tool
- HBM:
-
Human Body Model
- GRAIL:
-
Gait Real-time Analysis Interactive Lab
References
Stevens JA, Mahoney JE, Ehrenreich H. Circumstances and outcomes of falls among high risk community-dwelling older adults. Inj Epidemiol. 2014;1:5. https://doi.org/10.1186/2197-1714-1-5.
Tinetti ME, Williams CS. Falls, injuries due to falls, and the risk of admission to a nursing home. N Engl J Med. 1997;337:1279–84. https://doi.org/10.1056/NEJM199710303371806.
McCrum C, Gerards MHGG, Karamanidis K, Zijlstra W, Meijer K. A systematic review of gait perturbation paradigms for improving reactive stepping responses and falls risk among healthy older adults. Eur Rev Aging Phys Act. 2017;14:3. https://doi.org/10.1186/s11556-017-0173-7.
Delfi G, Al Bochi A, Dutta T. A scoping review on minimum foot clearance measurement: sensing modalities. Int J Environ Res Public Health. 2021;18:10848. https://doi.org/10.3390/ijerph182010848.
Timsina LR, Willetts JL, Brennan MJ, Marucci-Wellman H, Lombardi DA, Courtney TK, et al. Circumstances of fall-related injuries by age and gender among community-dwelling adults in the United States. PLoS ONE. 2017;12: e0176561. https://doi.org/10.1371/journal.pone.0176561.
Roeles S, Rowe PJ, Bruijn SM, Childs CR, Tarfali GD, Steenbrink F, et al. Gait stability in response to platform, belt, and sensory perturbations in young and older adults. Med Biol Eng Compu. 2018;56:2325–35. https://doi.org/10.1007/s11517-018-1855-7.
Grabiner MD, Crenshaw JR, Hurt CP, Rosenblatt NJ, Troy KL. Exercise-based fall prevention. Exerc Sport Sci Rev. 2014;42:161–8. https://doi.org/10.1249/JES.0000000000000023.
Rieger MM, Papegaaij S, Steenbrink F, van Dieën JH, Pijnappels M. Perturbation-based gait training to improve daily life gait stability in older adults at risk of falling: protocol for the REACT randomized controlled trial. BMC Geriatr. 2020;20:167. https://doi.org/10.1186/s12877-020-01566-z.
Nørgaard JE, Andersen S, Ryg J, Stevenson AJT, Andreasen J, Danielsen MB, et al. Effects of treadmill slip and trip perturbation-based balance training on falls in community-dwelling older adults (STABILITY): study protocol for a randomised controlled trial. BMJ Open. 2022;12: e052492. https://doi.org/10.1136/bmjopen-2021-052492.
Maki BE, Mcilroy WE, Fernie GR. Change-in-support reactions for balance recovery. IEEE Eng Med Biol Mag. 2003;22:20–6. https://doi.org/10.1109/MEMB.2003.1195691.
Roman de Mettelinge T, Calders P, Danneels E, Geeroms S, Du Four C, Cambier D. Does footwear matter when performing spatiotemporal gait analysis among older women? J Geriatr Phys Ther. 2015;38:155–61. https://doi.org/10.1519/JPT.0000000000000052.
Hollander K, Petersen E, Zech A, Hamacher D. Effects of barefoot vs. shod walking during indoor and outdoor conditions in younger and older adults. Gait Posture. 2022;95:284–91. https://doi.org/10.1016/j.gaitpost.2021.04.024.
Franklin S, Grey MJ, Heneghan N, Bowen L, Li F-X. Barefoot vs common footwear: a systematic review of the kinematic, kinetic and muscle activity differences during walking. Gait Posture. 2015;42:230–9. https://doi.org/10.1016/j.gaitpost.2015.05.019.
Hollander K, van der Zwaard BC, de Villiers JE, Braumann K-M, Venter R, Zech A. The effects of being habitually barefoot on foot mechanics and motor performance in children and adolescents aged 6–18 years: study protocol for a multicenter cross-sectional study (Barefoot LIFE project). J Foot Ankle Res. 2016;9:36. https://doi.org/10.1186/s13047-016-0166-1.
Menant JC, Steele JR, Menz HB, Munro BJ, Lord SR. Optimizing footwear for older people at risk of falls. J Rehab Res Dev. 2008;45:1167. https://doi.org/10.1682/JRRD.2007.10.0168.
Robbins S, Waked E, McClaran J. Proprioception and stability: foot position awareness as a function of age and footware. Age Ageing. 1995;24:67–72. https://doi.org/10.1093/ageing/24.1.67.
Ramanathan AK, Parish EJ, Arnold GP, Drew TS, Wang W, Abboud RJ. The influence of shoe sole’s varying thickness on lower limb muscle activity. Foot Ankle Surg. 2011;17:218–23. https://doi.org/10.1016/j.fas.2010.07.003.
Menant JC, Steele JR, Menz HB, Munro BJ, Lord SR. Effects of footwear features on balance and stepping in older people. Gerontology. 2008;54:18–23. https://doi.org/10.1159/000115850.
Petersen E, Zech A, Hamacher D. Walking barefoot vs. with minimalist footwear – influence on gait in younger and older adults. BMC Geriatrics. 2020;20:88. https://doi.org/10.1186/s12877-020-1486-3.
Cudejko T, Gardiner J, Akpan A, D’Août K. Minimal shoes improve stability and mobility in persons with a history of falls. Sci Rep. 2020;10:21755. https://doi.org/10.1038/s41598-020-78862-6.
Moon Y, Wajda DA, Motl RW, Sosnoff JJ. Stride-time variability and fall risk in persons with multiple sclerosis. Mult Scler Int. 2015;2015:1–7. https://doi.org/10.1155/2015/964790.
Ma L, Mi T-M, Jia Q, Han C, Chhetri JK, Chan P. Gait variability is sensitive to detect Parkinson’s disease patients at high fall risk. Int J Neurosci. 2022;132:888–93. https://doi.org/10.1080/00207454.2020.1849189.
Frenkel-Toledo S, Giladi N, Peretz C, Herman T, Gruendlinger L, Hausdorff JM. Effect of gait speed on gait rhythmicity in Parkinson’s disease: variability of stride time and swing time respond differently. J Neuroeng Rehabil. 2005;2:23. https://doi.org/10.1186/1743-0003-2-23.
Roos PE, Dingwell JB. Influence of simulated neuromuscular noise on movement variability and fall risk in a 3D dynamic walking model. J Biomech. 2010;43:2929–35. https://doi.org/10.1016/j.jbiomech.2010.07.008.
Hausdorff JM, Rios DA, Edelberg HK. Gait variability and fall risk in community-living older adults: a 1-year prospective study. Arch Phys Med Rehabil. 2001;82:1050–6. https://doi.org/10.1053/apmr.2001.24893.
Pieruccini-Faria F, Montero-Odasso M. Obstacle negotiation, gait variability, and risk of falling: results from the “gait and brain study.” J Gerontol Series A. 2019;74:1422–8. https://doi.org/10.1093/gerona/gly254.
Johansson J, Nordström A, Nordström P. Greater fall risk in elderly women than in men is associated with increased gait variability during multitasking. J Am Med Dir Assoc. 2016;17:535–40. https://doi.org/10.1016/j.jamda.2016.02.009.
Kroneberg D, Elshehabi M, Meyer AC, Otte K, Doss S, Paul F, et al. Less is more – estimation of the number of strides required to assess gait variability in spatially confined settings. Front Aging Neurosci. 2019;10:1–13. https://doi.org/10.3389/fnagi.2018.00435.
Amira AS. Effect of robotic assisted gait training on functional and psychological improvement in patients with incomplete spinal cord injury. J Novel Physiother Phys Rehab. 2017;6:083–6. https://doi.org/10.17352/2455-5487.000053.
Matsas A, Taylor N, McBurney H. Knee joint kinematics from familiarised treadmill walking can be generalised to overground walking in young unimpaired subjects. Gait Posture. 2000;11:46–53. https://doi.org/10.1016/S0966-6362(99)00048-X.
Meyer C, Killeen T, Easthope CS, Curt A, Bolliger M, Linnebank M, et al. Familiarization with treadmill walking: how much is enough? Sci Rep. 2019;9:5232. https://doi.org/10.1038/s41598-019-41721-0.
Arnold BJW, Weeks BK, Horan SA. An examination of treadmill running familiarisation in barefoot and shod conditions in healthy men. J Sports Sci. 2019;37:5–12. https://doi.org/10.1080/02640414.2018.1479533.
Ren X, Lutter C, Kebbach M, Bruhn S, Yang Q, Bader R, et al. Compensatory responses during slip-induced perturbation in patients with knee osteoarthritis compared with healthy older adults: an increased risk of falls? Front Bioeng Biotechnol. 2022;10:1–13. https://doi.org/10.3389/fbioe.2022.893840.
van den Bogert AJ, Geijtenbeek T, Even-Zohar O, Steenbrink F, Hardin EC. A real-time system for biomechanical analysis of human movement and muscle function. Med Biol Eng Compu. 2013;51:1069–77. https://doi.org/10.1007/s11517-013-1076-z.
Winter DA, Sidwall HG, Hobson DA. Measurement and reduction of noise in kinematics of locomotion. J Biomech. 1974;7:157–9. https://doi.org/10.1016/0021-9290(74)90056-6.
Zeni JA, Richards JG, Higginson JS. Two simple methods for determining gait events during treadmill and overground walking using kinematic data. Gait Posture. 2008;27:710–4. https://doi.org/10.1016/j.gaitpost.2007.07.007.
Moon Y, Sung J, An R, Hernandez ME, Sosnoff JJ. Gait variability in people with neurological disorders: a systematic review and meta-analysis. Hum Mov Sci. 2016;47:197–208. https://doi.org/10.1016/j.humov.2016.03.010.
Melzer I, Elbar O, Tsedek I, Oddsson LIE. A water-based training program that include perturbation exercises to improve stepping responses in older adults: study protocol for a randomized controlled cross-over trial. BMC Geriatr. 2008;8:19. https://doi.org/10.1186/1471-2318-8-19.
Maki BE, McIlroy WE. The role of limb movements in maintaining upright stance: the “change-in-support” strategy. Phys Ther. 1997;77:488–507. https://doi.org/10.1093/ptj/77.5.488.
Debelle H, Harkness-Armstrong C, Hadwin K, Maganaris CN, O’Brien TD. Recovery from a forward falling slip: measurement of dynamic stability and strength requirements using a split-belt instrumented treadmill. Front Sports Act Living. 2020;2:82. https://doi.org/10.3389/fspor.2020.00082.
Riazati S, Caplan N, Hayes PR. The number of strides required for treadmill running gait analysis is unaffected by either speed or run duration. J Biomech. 2019;97: 109366. https://doi.org/10.1016/j.jbiomech.2019.109366.
Ciprandi D, Bertozzi F, Zago M, Ferreira CLP, Boari G, Sforza C, et al. Study of the association between gait variability and physical activity. Eur Rev Aging Phys Act. 2017;14:19. https://doi.org/10.1186/s11556-017-0188-0.
Taborri J, Santuz A, Brüll L, Arampatzis A, Rossi S. Measuring kinematic response to perturbed locomotion in young adults. Sensors. 2022;22:672. https://doi.org/10.3390/s22020672.
Madehkhaksar F, Klenk J, Sczuka K, Gordt K, Melzer I, Schwenk M. The effects of unexpected mechanical perturbations during treadmill walking on spatiotemporal gait parameters, and the dynamic stability measures by which to quantify postural response. PLoS ONE. 2018;13: e0195902. https://doi.org/10.1371/journal.pone.0195902.
Wang Y, Wang S, Lee A, Pai Y-C, Bhatt T. Treadmill-gait slip training in community-dwelling older adults: mechanisms of immediate adaptation for a progressive ascending-mixed-intensity protocol. Exp Brain Res. 2019;237:2305–17. https://doi.org/10.1007/s00221-019-05582-3.
Bruijn SM, Meijer OG, Beek PJ, van Dieën JH. Assessing the stability of human locomotion: a review of current measures. J R Soc Interface. 2013;10:20120999. https://doi.org/10.1098/rsif.2012.0999.
Bytyçi I, Henein MY. Stride length predicts adverse clinical events in older adults: a systematic review and meta-analysis. J Clin Med. 2021;10:2670. https://doi.org/10.3390/jcm10122670.
Stoneham R, Barry G, Saxby L, Waters L, Wilkinson M. Differences in stride length and lower limb moments of recreational runners during over-ground running while barefoot, in minimalist and in maximalist running shoes. Footwear Sci. 2021;13:133–41. https://doi.org/10.1080/19424280.2021.1878285.
Van Hooren B, Fuller JT, Buckley JD, Miller JR, Sewell K, Rao G, et al. Is motorized treadmill running biomechanically comparable to overground running? A systematic review and meta-analysis of cross-over studies. Sports Med. 2020;50:785–813. https://doi.org/10.1007/s40279-019-01237-z.
Semaan MB, Wallard L, Ruiz V, Gillet C, Leteneur S, Simoneau-Buessinger E. Is treadmill walking biomechanically comparable to overground walking? A systematic review. Gait Posture. 2022;92:249–57. https://doi.org/10.1016/j.gaitpost.2021.11.009.
König Ignasiak N, Ravi DK, Orter S, Hosseini Nasab SH, Taylor WR, Singh NB. Does variability of footfall kinematics correlate with dynamic stability of the centre of mass during walking? PLoS ONE. 2019;14: e0217460. https://doi.org/10.1371/journal.pone.0217460.
Maki BE. Gait changes in older adults: predictors of falls or indicators of fear? J Am Geriatr Soc. 1997;45:313–20. https://doi.org/10.1111/j.1532-5415.1997.tb00946.x.
Owings TM, Grabiner MD. Step width variability, but not step length variability or step time variability, discriminates gait of healthy young and older adults during treadmill locomotion. J Biomech. 2004;37:935–8. https://doi.org/10.1016/j.jbiomech.2003.11.012.
Skiadopoulos A, Moore EE, Sayles HR, Schmid KK, Stergiou N. Step width variability as a discriminator of age-related gait changes. J Neuroeng Rehabil. 2020;17:41. https://doi.org/10.1186/s12984-020-00671-9.
Bauby CE, Kuo AD. Active control of lateral balance in human walking. J Biomech. 2000;33:1433–40. https://doi.org/10.1016/S0021-9290(00)00101-9.
Brach JS, Berlin JE, VanSwearingen JM, Newman AB, Studenski SA. Too much or too little step width variability is associated with a fall history in older persons who walk at or near normal gait speed. J Neuroeng Rehabil. 2005;2:21. https://doi.org/10.1186/1743-0003-2-21.
Pereira MP, Orcioli-Silva D, de Sousa PN, Beretta VS, Gobbi LTB. The effects of habitual footwear in gait outcomes in people with Parkinson’s disease. Gait Posture. 2019;68:111–4. https://doi.org/10.1016/j.gaitpost.2018.11.013.
Grabiner PC, Biswas ST, Grabiner MD. Age-related changes in spatial and temporal gait variables. Arch Phys Med Rehabil. 2001;82:31–5. https://doi.org/10.1053/apmr.2001.18219.
Gabell A, Nayak USL. The effect of age on variability in gait. J Gerontol. 1984;39:662–6. https://doi.org/10.1093/geronj/39.6.662.
Robbins S, Gouw GJ, McClaran J. Shoe sole thickness and hardness influence balance in older men. J Am Geriatr Soc. 1992;40:1089–94. https://doi.org/10.1111/j.1532-5415.1992.tb01795.x.
Nestico J, Novak A, Perry SD, Mansfield A. Does increased gait variability improve stability when faced with an expected balance perturbation during treadmill walking? Gait Posture. 2021;86:94–100. https://doi.org/10.1016/j.gaitpost.2021.03.014.
Perera S, Smith C, Coffman L, Brach J. Number of steps needed for reliable gait variability measurement. Gerontologist. 2016;56(3):335–6. https://doi.org/10.1093/geront/gnw162.1366.
König N, Singh NB, von Beckerath J, Janke L, Taylor WR. Is gait variability reliable? An assessment of spatio-temporal parameters of gait variability during continuous overground walking. Gait Posture. 2014;39:615–7. https://doi.org/10.1016/j.gaitpost.2013.06.014.
Hollman JH, Childs KB, McNeil ML, Mueller AC, Quilter CM, Youdas JW. Number of strides required for reliable measurements of pace, rhythm and variability parameters of gait during normal and dual task walking in older individuals. Gait Posture. 2010;32:23–8. https://doi.org/10.1016/j.gaitpost.2010.02.017.
Hamacher D, Hamacher D, Krowicki M, Schega L. Between-day test–retest reliability of gait variability in older individuals improves with a familiarization trial. Aging Clin Exp Res. 2017;29:327–9. https://doi.org/10.1007/s40520-016-0536-3.
Acknowledgements
We are particularly grateful to all participants for their involvement in this study, as well as the technical support for system applications from Frank Feldhege, Martin Gube, and Yukun Su.
Experimental methods statement
All methods in this study were carried out in accordance with Declaration of Helsinki.
Funding
Open Access funding enabled and organized by Projekt DEAL. This work was co-funded by Zhejiang Normal University (Jinhua, China) and Biomechanics and Implant Technology Research Laboratory (Rostock, Germany). The GRAIL system was supported by the German Research Foundation DFG (INST 264/137 − 1). The funding sources have no role in the research design, data collection, analysis, interpretation, manuscript authoring, and manuscript submission decisions.
Author information
Authors and Affiliations
Contributions
XR, MK, SB, RB, TT, and CL conceived and designed this study. SB and RB provided the lab and equipment for the entire measurement. XR and CL conducted data collecting. XR and HL performed data processing, and statistical analysis. XR, QY, HL, TT, and CL prepared the manuscript. XR, MK, QY, HL, SB, RB, TT, and CL commented, revised the manuscript, and all approved for submission.
Corresponding author
Ethics declarations
Ethics approval and consent to participate
All experimental protocols were approved by the Ethics Committee of the Rostock University Medical Center, Germany (No. A2019-0231).
Before the investigation, all individuals provided written informed consent.
Consent for publication
Not applicable.
Competing interests
The authors declare that they have no competing interests.
Additional information
Publisher’s Note
Springer Nature remains neutral with regard to jurisdictional claims in published maps and institutional affiliations.
Rights and permissions
Open Access This article is licensed under a Creative Commons Attribution 4.0 International License, which permits use, sharing, adaptation, distribution and reproduction in any medium or format, as long as you give appropriate credit to the original author(s) and the source, provide a link to the Creative Commons licence, and indicate if changes were made. The images or other third party material in this article are included in the article's Creative Commons licence, unless indicated otherwise in a credit line to the material. If material is not included in the article's Creative Commons licence and your intended use is not permitted by statutory regulation or exceeds the permitted use, you will need to obtain permission directly from the copyright holder. To view a copy of this licence, visit http://creativecommons.org/licenses/by/4.0/. The Creative Commons Public Domain Dedication waiver (http://creativecommons.org/publicdomain/zero/1.0/) applies to the data made available in this article, unless otherwise stated in a credit line to the data.
About this article
Cite this article
Ren, X., Kebbach, M., Bruhn, S. et al. Barefoot walking is more stable in the gait of balance recovery in older adults. BMC Geriatr 22, 904 (2022). https://doi.org/10.1186/s12877-022-03628-w
Received:
Accepted:
Published:
DOI: https://doi.org/10.1186/s12877-022-03628-w