Abstract
Background
This study aims to deeply explore the relationship between m6A methylation modification and peripheral immune cells in patients with advanced sepsis and mine potential epigenetic therapeutic targets by analyzing the differential expression patterns of m6A-related genes in healthy subjects and advanced sepsis patients.
Methods
A single cell expression dataset of peripheral immune cells containing blood samples from 4 patients with advanced sepsis and 5 healthy subjects was obtained from the gene expression comprehensive database (GSE175453). Differential expression analysis and cluster analysis were performed on 21 m6A-related genes. The characteristic gene was identified based on random forest algorithm, and the correlation between the characteristic gene METTL16 and 23 immune cells in patients with advanced sepsis was evaluated using single-sample gene set enrichment analysis.
Results
IGFBP1, IGFBP2, IGF2BP1, and WTAP were highly expressed in patients with advanced sepsis and m6A cluster B. IGFBP1, IGFBP2, and IGF2BP1 were positively correlated with Th17 helper T cells. The characteristic gene METTL16 exhibited a significant positive correlation with the proportion of various immune cells.
Conclusion
IGFBP1, IGFBP2, IGF2BP1, WTAP, and METTL16 may accelerate the development of advanced sepsis by regulating m6A methylation modification and promoting immune cell infiltration. The discovery of these characteristic genes related to advanced sepsis provides potential therapeutic targets for the diagnosis and treatment of sepsis.
Similar content being viewed by others
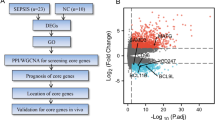
Background
Sepsis is a life-threatening syndrome caused by a deregulated host response to bacterial, fungal, or viral infection, involving physiological, pathological, and biochemical abnormalities [1, 2]. It is generally believed that the symptoms of early sepsis are mild, manifested as non-specific symptoms such as fever, headache, and fatigue. However, physical examination may reveal inflammatory reactions and symptoms of redness and swelling at the site of local infection. Over time, the condition may further worsen, with severe symptoms such as high fever, chills, shortness of breath, and increased heart rate. Advanced sepsis typically manifests as multiple organ dysfunction syndrome (MODS), which involves multiple organ dysfunction and severe symptoms such as renal insufficiency, pneumonia, myocardial injury, and bleeding. At this time, the condition is already very critical and requires urgent treatment [3]. In advanced sepsis, the number and function of various peripheral immune cells, including CD4 + T cells, CD8 + T cells, B cells, and natural killer cell, may be affected, resulting in the inability of the immune system to effectively eliminate pathogens [4]. Therefore, analyzing the expression of peripheral immune cells in patients with advanced sepsis can provide detailed information on the immune response to sepsis and help to gain a deeper understanding of the specific molecular mechanisms underlying the high mortality rate caused by this disease.
N6 methyladenosine (m6A) is the most prevalent post-transcriptional chemical modification on RNA molecules, which participates in the regulation of various biological processes including immunity, metabolism, proliferation, and apoptosis, accounting for more than 60% of all RNA epigenetics [5]. The biological function of m6A modification is dynamically and reversibly mediated by methyltransferases (writers), demethylases (erasers), and m6A recognition proteins (readers), which is involved in the occurrence and development of various diseases and is also related to the high heterogeneity in advanced sepsis [6,7,8]. We speculate that m6A modification plays an important role in immune regulation in advanced sepsis. In the present study, bioinformatics methods were used to analyze the differential expression patterns of m6A methylation regulators and explore their relationship with immune cell infiltration, aiming to provide a theoretical basis for the individualized risk determination and treatment target selection of advanced sepsis.
Methods
Source of data
The dataset GSE175453 containing the transcriptome data of peripheral blood mononuclear cells (PBMCs) in the blood samples from 4 patients with advanced sepsis (14–21 days after sepsis) and 5 healthy subjects was obtained from the Gene Expression Omnibus (GEO) [9].
Screening of m6A methylation regulators
A total of 21 m6A-related genes were obtained from the literature to identify their different m6A modification modes: RNA binding motif protein 15B (RBM15B), insulin-like growth factor binding protein 3 (IGFBP3), IGFBP1, IGFBP2, insulin-like growth factor 2 mRNA binding protein 1 (IGF2BP1), zinc finger CCCH domain-containing protein 13 (ZC3H13), Casitas B-lineage lymphoma-transforming sequence-like protein 1 (CBLL1), YTH N6-methyladenosine RNA binding protein 1 (YTHDF1), YTHDF2, heterogeneous nuclear ribonucleoprotein C (HNRNPC), embryonic lethal-abnormal vision like protein 1 (ELAVL1), methyltransferase-like 3 (METTL3), RNA-binding motif protein X-linked (RBMX), leucine-rich pentatricopeptide repeat (PPR)-motif-containing protein (LRPPRC), METTL16, fat mass and obesity-associated gene (FTO), Wilms tumor 1-associated protein (WTAP), YTH domain containing 1 (YTHDC1), fragile X mental retardation type 1 (FMR1), RBM15, and Heterogeneous nuclear ribonucleoprotein A2B1 (HNRNPA2B1).
Analysis of sepsis characteristic genes based on random forest (RF) algorithm
The m6A methylation regulatory factors differentially expressed in peripheral blood immune cells between healthy subjects and advanced sepsis patients were analyzed using the “limma” R package, with the screening criteria of P < 0.05 [10]. Pearson correlation coefficient was used to analyze the correlation of the differentially expressed m6A methylation regulators (r > 0.3, P < 0.05). The upport vector machine (SVM) algorithm and RF algorithm were performed using the “randomForest” R package to construct a model for predicting the occurrence of advanced sepsis [11, 12]. The prediction accuracy of the model was evaluated using the residual boxplot, the residual reverse cumulative distribution, and the receiver operating characteristic (ROC) curve.
m6A clustering and immunocyte infiltration analysis
Using the “ConsensusClusterPlus” R package, the m6A clusters were identified by consensus clustering based on the m6A methylation regulatory factors [13]. Then, the abundance of 23 immune cells in advanced sepsis was evaluated using single-sample gene set enrichment analysis (ssGSEA) to further investigate the correlation [14,15,16,17].
Statistical analysis
Statistical analysis was performed using R (version 4.1.0). Linear regression analysis and Pearson correlation coefficient (r) were used to determine the correlation between gene expression patterns. Nonparametric one-way analysis of variance (ANOVA) was used to compare the variables between different groups. The comparison between two groups was performed using the t-test. A value of P < 0.05 was indicative of statistical significance.
Results
Differentially expressed m6A methylation regulators in peripheral immune cells in advanced sepsis
There were 21 differentially expressed m6A methylation regulators in peripheral immune cells between healthy subjects and advanced sepsis patients in the GSE175453 dataset. As shown in Fig. 1A, IGFBP1, IGFBP2, IGF2BP1, and WTAP were highly expressed in advanced sepsis patients, and the remaining 17 regulators were poorly expressed. Further analysis showed that there was a positive correlation between the expression of METTL3 (Fig. 1B), METTL16 (Fig. 1C), and FTO. These results suggested that m6A methylation might play an important role in sepsis.
Differential expression of m6A methylation regulatory factors in sepsis. (A) Heatmap analysis of differentially expressed m6A methylation regulators; (B) Correlation analysis of gene expression between METTL3 and FTO; (C) Correlation analysis of gene expression between METTL16 and FTO. Con: control patients; treat: sepsis patients
Identification of two m6A clusters
Consensus cluster analysis was performed using the “ConsensusClusterPlus” R package based on differential m6A methylation regulators, and two m6A clusters (m6A cluster A and m6A cluster B) were identified (Fig. 2A). The results of principal component analysis (PCA) showed that m6A RNA methylation regulators could be classified into two m6A clusters (Fig. 2B). Figure 2 C shows the heat map of the differentially expressed genes in two m6A clusters (Additional file 1: Table S1). IGFBP1, IGFBP2, and IGF2BP1 were highly expressed in cluster B (Additional file 2: Table S2).
Construction of sepsis prediction model based on RF algorithm
The performance of SVM algorithm and RF algorithm was compared using residual boxplots (Fig. 3A), residual reverse cumulative distribution (Fig. 3B), and ROC curve (Fig. 3C). The results showed that there was no significant difference between the two algorithms. Compared with SVM algorithm, RF algorithm had higher accuracy, so the subsequent screening of disease characteristic genes was based on RF algorithm.
Immunocyte infiltration analysis
Due to the fact that a large number of immune cells are involved in sepsis, we further analyzed the difference in immune cell infiltration between the two m6A clusters. The activated CD4+T cells, activated CD8 + T cells, regulatory T cells, Th2 helper T cells, and Th17 helper T cells showed significant differences between the two m6A clusters (Fig. 4A). In addition, ssGSEA was used to determine the correlation between the expression of 21 m6A methylation regulatory factors and the infiltration of immune cells (Fig. 4B). Correlation heat map analysis showed that IGFBP1, IGFBP2, and IGF2BP1 had a significant positive correlation with Th17 helper T cells. Based on the RF model, the genetic importance of 21 m6A methylation regulators was ranked (Fig. 4C). Then we selected METTL16 gene to further observe its correlation with immune cell infiltration, and found that it was significantly positively correlated with activated CD4+T cells, activated CD8 + T cells, natural killer T cells, regulatory T cells, Th1 helper T cells, and Th2 helper T cells (Fig. 4D).
Immune cell infiltration analysis. A Boxplots showing the infiltration of immune cells in two m6A clusters; B Correlation heatmaps of 21 m6A methylation regulatory factors and immune cell infiltration by single sample gene cluster enrichment analysis (ssGSEA); C Importance of 21 m6A regulatory factors based on random forest (RF) model; D Positive correlation between METTL16 and immune cell infiltration
Discussion
Advanced sepsis is a serious life-threatening infectious disease, and its pathological and physiological mechanisms are still not fully understood [18, 19]. m6A regulatory factors are closely related to human diseases, and abnormal m6A methylation modification is believed to mediate various immune responses and the pathogenesis of autoimmune related diseases [20]. According to the analysis of m6A-single nucleotide polymorphism (SNP) and expression quantitative trait locus (eQTL) dataset, m6A is involved in regulating bacterial infection. Sun et al. identified 1321 genes as the sites of m6A cis-eQTL [21]. These genes are highly enriched in the pathways involving platelet degranulation and staphylococcus aureus infection, which are crucial to the pathophysiological process of sepsis. In addition, multiple studies have reported the typing role of m6A regulatory factors in sepsis and their correlation with immune cells [8, 22]. These findings provide convincing evidence for the correlation between m6A modification and sepsis episodes.
In recent years, the development of single-cell RNA seq technology has provided a novel approach for sepsis research [9, 23]. Compared with traditional gene expression analysis methods, single cell RNA seq can analyze the transcriptome of a single cell, find more cell types and subtypes, and analyze heterogeneous cells more accurately. Researchers have found unique transcriptome patterns of multiple circulating immune cell subtypes, including B- and CD4+, CD8+, activated CD4+, and activated CD8+T lymphocytes, as well as NK, NKT, and plasma cell like dendritic cells in patients with advanced sepsis [9]. However, the specific molecular mechanisms leading to these changes remain unclear, and the therapeutic targets have not been determined yet.
This study focused on analyzing the relationship between m6A methylation modification and multiple lymphocytes in patients with advanced sepsis, and explored potential epigenetic therapeutic targets. In this study, the ssGSEA method was used to evaluate the abundance of 23 immune cells in advanced sepsis. The results showed that IGFBP1, IGFBP2, IGF2BP1, and WTAP were highly expressed in patients with advanced sepsis and m6A cluster B; IGFBP1, IGFBP2, and IGF2BP1 showed a significant positive correlation with Th17 helper T cells. These results all suggested that m6A methylation played a crucial role in immune regulation in advanced sepsis.
It is well-known that RF is a widely used machine-learning algorithm in bioinformatics, which has been proven to be effective in identifying relevant features and classifying samples. RF is particularly suitable for analyzing high-dimensional and complex data sets such as gene expression data, because it can handle a large number of variables and avoid overfitting. In addition, RF can provide a ranking of feature importance, which is valuable for identifying the genes most relevant to the results of interest. Therefore, in our study, we chose to use RF for feature selection and identify the most important genes associated with sepsis [24,25,26,27,28]. Although other algorithms such as xgboost, GLM, and NB are also popular in similar studies, we chose RF because of its advantages in processing complex datasets and providing feature importance rankings. Based on RF, this study screened the characteristic gene METTL16 as the most important gene in advanced sepsis. Like METTL3, the METTL16 gene also belongs to a class I methyltransferase that contains Rossmann fold of class I methyltransferases and uses S-adenosylmethionine (SAM) as the methyl donor. Moreover, METTL16 also contains other regions outside its methyltransferase domain, and these regions also interact with RNA substrates and may provide specificity. Intriguingly, METTL16 is commonly captured in the identification of mRNA binding proteins separated with polyadenosylated mRNA and endogenous interaction proteins, while the components of METTL3/14 complex are not captured. Although many METTL16 RNA interactants have been identified, only two of them have been confirmed as methylation substrates [29], which may indicate that METTL16 has other functions besides catalytic activity. Our study found that METTL16 gene was significantly positively correlated with the proportion of multiple immune cells, suggesting that METTL16 may promote the infiltration of immune cells in the occurrence and development of sepsis.
Conclusions
In this study, the m6A-related genes differentially expressed in healthy subjects and advanced sepsis patients were obtained. Based on the RF model and correlation analysis, IGFBP1, IGFBP2, IGF2BP1, WTAP, and METTL16 were screened out to accelerate the occurrence and development of sepsis by regulating m6A methylation and promoting immune cell infiltration. These genes provide potential drug targets for the early detection, diagnosis, and treatment of sepsis.
Availability of data and materials
The datasets generated and/or analysed during the current study are available in the GEO [GSE175453] repository (https://www.ncbi.nlm.nih.gov/geo/query/acc.cgi?acc=GSE175453).
Abbreviations
- GEO:
-
Gene expression comprehensive database
- RF:
-
Random forest
- ssGSEA:
-
Single-sample gene set enrichment analysis
- ICUs:
-
Intensive care units
- m6A:
-
N6 methyladenosine
- PBMCs:
-
Peripheral blood mononuclear cells
- RBM15B:
-
RNA binding motif protein 15B
- IGFBP3:
-
Insulin-like growth factor binding protein 3
- IGF2BP1:
-
Insulin-like growth factor 2 mRNA binding protein 1
- ZC3H13:
-
Zinc finger CCCH domain-containing protein 13
- CBLL1:
-
Casitas B-lineage lymphoma-transforming sequence-like protein 1
- YTHDF1:
-
YTH N6-methyladenosine RNA binding protein 1
- HNRNPC:
-
Heterogeneous nuclear ribonucleoprotein C
- ELAVL1:
-
Embryonic lethal-abnormal vision like protein 1
- METTL3:
-
Methyltransferase-like 3
- RBMX:
-
RNA-binding motif protein X-linked
- PPR:
-
Pentatricopeptide repeat
- FTO:
-
Fat mass and obesity-associated gene
- WTAP:
-
Wilms tumor 1-associated protein
- YTHDC1:
-
YTH domain containing 1
- FMR1:
-
Fragile X mental retardation type 1
- HNRNPA2B1:
-
Heterogeneous nuclear ribonucleoprotein A2B1
- SVM:
-
Support vector machine
- ROC:
-
Receiver operating characteristic
- ANOVA:
-
Analysis of variance
References
Kumar V. Targeting macrophage immunometabolism: Dawn in the darkness of sepsis. Int Immunopharmacol. 2018;58:173–85.
Rocheteau P, Chatre L, Briand D, Mebarki M, Jouvion G, Bardon J, et al. Sepsis induces long-term metabolic and mitochondrial muscle stem cell dysfunction amenable by mesenchymal stem cell therapy. Nat Commun. 2015;6:10145.
Xie J, Wang H, Kang Y, Zhou L, Liu Z, Qin B, et al. The epidemiology of Sepsis in Chinese ICUs: a National Cross-Sectional Survey. Crit Care Med. 2020;48(3):e209–18.
Quan Y, Zhang X, Ping H. Construction of a risk prediction model using m6A RNA methylation regulators in prostate cancer: comprehensive bioinformatic analysis and histological validation. Cancer Cell Int. 2022;22(1):33.
Chen LYC, Du XJ. Bioinformatics analysis of the effect of m6A methylation regulator on survival and prognosis of oral squamous cell carcinoma. J Huazhong Univ Sci Technol. 2020;49(04):443–9.
Zhang S, Liu F, Wu Z, Xie J, Yang Y, Qiu H. Contribution of m6A subtype classification on heterogeneity of sepsis. Ann Transl Med. 2020;8(6):306.
Faix JD. Biomarkers of sepsis. Crit Rev Clin Lab Sci. 2013;50(1):23–36.
Thompson K, Venkatesh B, Finfer S. Sepsis and septic shock: current approaches to management. Intern Med J. 2019;49(2):160–70.
Sun T, Wu R, Ming L. The role of m6A RNA methylation in cancer. Biomed Pharmacother. 2019;112:108613.
Zhang H, Shi X, Huang T, Zhao X, Chen W, Gu N, et al. Dynamic landscape and evolution of m6A methylation in human. Nucleic Acids Res. 2020;48(11):6251–64.
Sun X, Dai Y, Tan G, Liu Y, Li N. Integration analysis of m(6)A-SNPs and eQTLs associated with Sepsis reveals platelet degranulation and Staphylococcus aureus infection are mediated by m(6)a mRNA methylation. Front Genet. 2020;11:7.
Han X, Guo J, Fan Z. Interactions between m6A modification and miRNAs in malignant tumors. Cell Death Dis. 2021;12(6):598.
Maimaiti A, Turhon M, Cheng X, Su R, Kadeer K, Axier A, et al. m6A regulator-mediated RNA methylation modification patterns and immune microenvironment infiltration characterization in patients with intracranial aneurysms. Front Neurol. 2022;13:889141.
Ranke MB. Insulin-like growth factor binding-protein-3 (IGFBP-3). Best Pract Res Clin Endocrinol Metab. 2015;29(5):701–11.
Poli E, Zin A, Cattelan M, Tombolan L, Zanetti I, Scagnellato A et al. Prognostic value of circulating IGFBP2 and related autoantibodies in children with metastatic rhabdomyosarcomas. Diagnostics. 2020;10(2).
Gao S, Sun Y, Zhang X, Hu L, Liu Y, Chua CY, et al. IGFBP2 activates the NF-kappaB pathway to drive epithelial-mesenchymal transition and invasive character in pancreatic ductal adenocarcinoma. Cancer Res. 2016;76(22):6543–54.
Sun L, Zhang X, Song Q, Liu L, Forbes E, Tian W, et al. IGFBP2 promotes tumor progression by inducing alternative polarization of macrophages in pancreatic ductal adenocarcinoma through the STAT3 pathway. Cancer Lett. 2021;500:132–46.
Wu X, Zheng W, Jin P, Hu J, Zhou Q. Role of IGFBP1 in the senescence of vascular endothelial cells and severity of aging–related coronary atherosclerosis. Int J Mol Med. 2019;44(5):1921–31.
Ping XL, Sun BF, Wang L, Xiao W, Yang X, Wang WJ, et al. Mammalian WTAP is a regulatory subunit of the RNA N6-methyladenosine methyltransferase. Cell Res. 2014;24(2):177–89.
Chen Y, Peng C, Chen J, Chen D, Yang B, He B, et al. WTAP facilitates progression of hepatocellular carcinoma via m6A-HuR-dependent epigenetic silencing of ETS1. Mol Cancer. 2019;18(1):127.
Pendleton KE, Chen B, Liu K, Hunter OV, Xie Y, Tu BP, et al. The U6 snRNA m(6)a methyltransferase METTL16 regulates SAM synthetase intron retention. Cell. 2017;169(5):824–35 e14.
Satterwhite ER, Mansfield KD. RNA methyltransferase METTL16: targets and function. Wiley Interdiscip Rev RNA. 2022;13(2):e1681.
Wang T, Zhang X, Liu Z, Yao T, Zheng D, Gan J, Yu S, Li L, Chen P, Sun J. Single-cell RNA sequencing reveals the sustained immune cell dysfunction in the pathogenesis of sepsis secondary to bacterial pneumonia. Genomics. 2021;113:1219–33.
Rigatti SJ. Random Forest J Insur Med. 2017;47:31–9.
Barradas-Bautista D, Cao Z, Vangone A, Oliva R, Cavallo L. A random forest classifier for protein-protein docking models. Bioinform Adv. 2022;2:vbab042.
Ganaie MA, Tanveer M, Suganthan PN, Snasel V. Oblique and rotation double random forest. Neural Netw. 2022;153:496–517.
Ghosh D, Cabrera J. Enriched Random Forest for High Dimensional Genomic Data. IEEE/ACM Trans Comput Biol Bioinform. 2022;19:2817–28.
Utkin LV, Konstantinov AV. Attention-based random forest and contamination model. Neural Netw. 2022;154:346–59.
Satterwhite ER, Mansfield KD. RNA methyltransferase METTL16: Targets and function. Wiley Interdiscip Rev RNA 2022;13:e1681.
Acknowledgements
We would like to acknowledge the reviewers for their helpful comments on this paper.
Funding
This study was supported by the GuangDong Basic and Applied Basic Research Foundation (2022A1515111162).
Author information
Authors and Affiliations
Contributions
SS equally contributed to the conception and design of the research; WQ and JZ contributed to the design of the research; WQ, JZ, and SScontributed to the acquisition and analysis of the data; WQ, JZ, and SS contributed to the interpretation of the data; and WQ, JZ, and SSdrafted the manuscript. All authors critically revised the manuscript, agree to be fully accountable for ensuring the integrity and accuracy of the work, and read and approved the final manuscript.
Corresponding author
Ethics declarations
Ethics approval and consent to participate
Not applicable. All methods were performed in accordance with the relevant guidelines and regulations.
Consent to publication
Not applicable.
Competing interests
The authors declare no competing interests.
Additional information
Publisher’s Note
Springer Nature remains neutral with regard to jurisdictional claims in published maps and institutional affiliations.
Supplementary Information
Additional file 1: Table S1.
Identification of m6A clusters
Additional file 2: Table S2.
m6A differential gene expression
Rights and permissions
Open Access This article is licensed under a Creative Commons Attribution 4.0 International License, which permits use, sharing, adaptation, distribution and reproduction in any medium or format, as long as you give appropriate credit to the original author(s) and the source, provide a link to the Creative Commons licence, and indicate if changes were made. The images or other third party material in this article are included in the article's Creative Commons licence, unless indicated otherwise in a credit line to the material. If material is not included in the article's Creative Commons licence and your intended use is not permitted by statutory regulation or exceeds the permitted use, you will need to obtain permission directly from the copyright holder. To view a copy of this licence, visit http://creativecommons.org/licenses/by/4.0/. The Creative Commons Public Domain Dedication waiver (http://creativecommons.org/publicdomain/zero/1.0/) applies to the data made available in this article, unless otherwise stated in a credit line to the data.
About this article
Cite this article
Qian, W., Zhou, J. & Shou, S. Exploration of m6A methylation regulators as epigenetic targets for immunotherapy in advanced sepsis. BMC Bioinformatics 24, 257 (2023). https://doi.org/10.1186/s12859-023-05379-w
Received:
Accepted:
Published:
DOI: https://doi.org/10.1186/s12859-023-05379-w