Abstract
The study explored the role of circadian rhythm genes (CRGs) in lower grade glioma (LGG) development and found that certain genes, such as CRY1, NPAS2, and RORB, were associated with increased or decreased risk of LGG. The study also investigated the correlation between CRGs and immune cell infiltration, revealing a negative association with macrophage infiltration and a positive correlation with B cell and CD8 + T cell infiltration. Additionally, the study identified major mutated CRGs, including PER2, BMAL1, CLOCK, and BMAL2, and their potential interaction with other CNS-associated genes. The study suggests that CRGs play a crucial role in immune response and tumorigenesis in LGG patients and warrants further investigation.
Similar content being viewed by others
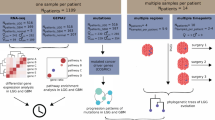
Introduction
One kind of cancer that starts in the brain's glial cells is called glioma. Lower grade gliomas (LGG), which include grades II and III, were investigated by TCGA. They can be due to some genetic mutations or environmental factors that are responsible for the growth of these tumors. There are no known causes of gliomas, and the risk factors favoring the development are poorly understood1.
The circadian rhythm genes (CRGs) regulate physiological processes such as sleep, metabolism, immune function, and cell cycle in the human body2. Brain tumor is a genetic-related disease, and a recent research has found that CRGs may play a crucial role in the occurrence and progression of brain tumors3. Patients with brain tumors may experience disruptions in their circadian rhythm, manifested as sleep disorders, abnormal mental states, and disturbances in activity patterns. The investigation of the correlation between brain tumors and CRGs aids in comprehending how disturbances in the circadian rhythm impact the disease progression and quality of life of individuals with brain tumors.
The CRGs play a crucial role in drug metabolism and drug responsiveness4. By studying the variations in these genes among brain tumor patients, treatment strategies can be optimized through personalized administration timing and dosage, ultimately leading to improved therapeutic outcomes. CRGs may have potential value in the prognosis evaluation and prediction of brain tumor patients5. By analyzing the association between CRGs and patient survival rates, treatment response, and risk of recurrence, more accurate prognostic indicators and personalized treatment recommendations can be provided to clinicians.
Materials and methods
Study design
This study aimed to explore the relationship between core CRGs and various aspects of brain tumor biology. Multiple datasets, including gene expression, methylation, mutation, and clinical data from brain tumor patients, were utilized to conduct comprehensive analyses.
The study began by examining the expression levels of CRGs in brain tumors. Gene expression data from tumor samples was analyzed and correlated with patient clinical data. Survival analyses were performed to assess the association between gene expression levels and patient survival. Next, the study investigated the correlation between CRGs and immune cell infiltration in brain tumors. RNA sequencing data was utilized for this analysis. Through deconvolution analysis, we estimated the presence and abundance of immune cell populations within the tumor microenvironment. Additionally, the study explored the relationship between CRGs methylation levels and transcriptional regulation. By analyzing DNA methylation and gene expression data from brain tumor samples, we identified potential associations and correlations. To further investigate causal relationships, Mendelian randomization was employed. This allowed us to explore the potential causal effects of multiple genes on brain tumor development and immune cell infiltration.
Overall, our study utilized a multidimensional approach, integrating various datasets and conducting extensive statistical and bioinformatics analyses. By investigating the expression, methylation, and causal effects of CRGs, as well as their correlations with immune cell infiltration, we aimed to provide insights into the complex biology of brain tumors.
Data source
The expression data of CRGs in LGG patients and control group with was downloaded in Gene Expression Profiling Interactive Analysis (GEPIA) (http://gepia.cancer-pku.cn/) and cBioPortal for Cancer Genomics (https://www.cbioportal.org). Immunity infiltration data was downloaded in TIMER2.0 database (http://timer.cistrome.org/). Circadian gene information was collected from Circadian Gene DataBase (CGBD) (http://cgdb.biocuckoo.org/).
Statistical analysis
Most correlation analysis, differential analysis, immune cell infiltration and survival analysis are based on online database GEPIA2.06 (http://gepia2.cancer-pku.cn/#index), TIMER2.07,8,9 and cBioPortal10,11,12. Mendelian randomization (MR) study is based on the online platform MR-base (http://app.mrbase.org/)13,14. When we did MR studies, we set p-value threshold for exposure selection as 5e-08. Linkage Disequilibrium R-square (LD Rsq) setting was 0.001 to exclude LD. Clumping distance was 10,000 kb for clumping analysis.
We used online platform Evenn (http://www.ehbio.com/test/venn/#/) to make venn graphs15. Other statistical analyses are based on R (Version 4.3.1).
Results
CRGs expression level in LGG
15 core CRGs were identified, with 7 showing high expression (CRY1, CRY2, NR1D2, PER1, PER2, PER3, RORA) and 5 showing low expression (BMAL1/ARNTL, BMAL2/ARNTL2, NPAS2, NR1D1, RORB). CRY1 was significantly upregulated, while NPAS2 and RORB were significantly downregulated (Fig. 1). CLOCK, NR1D2, PER1, PER2, PER3, and RORA showed relatively high expression, while ARNTL, ARNTL2, and NR1D1 showed relatively low expression (Figure S1).
Survival analysis based on gene expression levels of CRGs in LGG
Genes including CRY2, NR1D2, and PER3, were found to have a positive correlation with high overall survival (OS) and disease-free survival (DFS) in brain tumor patients. They were proved to be the hinder to LGG development (Hazard Ratio (HR) < 1, P < 0.05) (Fig. 2A, B). Specifically, among low and high NR1D2 group, the survival difference is significant (p value = 0.012; HR = 0.6312). Conversely, genes including ARNTL, ARNTL2, CRY1, NPAS2, PER1, and RORB, were demonstrated to promote the LGG (HR > 1, P < 0.05) (Fig. 2A) and some of them (ARNTL, CRY1, NPAS2) showed a negative correlation with OS and DFS (Fig. 2B). Specifically, low expression of ARNTL, CRY1, and NPAS2 was associated with high OS and DFS, while high expression of CRY2, NR1D2, and PER3 was associated with high OS and DFS (Figure S2). Notably, ARNTL1 shows significant survival difference (p value < 0.0001; HR = 2.6535). Additionally, in the survival curves, there is an intersection observed of two Kaplan–Meier (KM) curves for ARNTL2, NR1D1, RORA, and RORC (Figure S2).
Immune cell infiltration levels
It has been discussed that EPIC and QUANTISEQ results should be recommended as a routine computational method used to assess immune cell infiltration in tumors16. We selected EPIC results about B cell, CD4 + T cell, CD8 + T cell and Macrophage, QUANTISEQ result from Myeloid dendritic cell and CIBERSORT result from Neutrophil. As shown in Table 1, the expression of most CRGs showed a negative correlation with macrophage infiltration. PER1 showed an opposite trend compared to other CRGs. The expression of most CRGs had a strong positive correlation with B cell and CD8 + T cell infiltration, followed by CD4 + T cells and myeloid dendritic cells. The correlation between the expression of most CRGs and neutrophil infiltration was not significant, except for CLOCK, CRY2, and NR1D1.
CRGs in LGG with gene mutation and correlation
LGG mainly mutated CRGs are PER2, BMAL1, CLOCK, and BMAL2 (Fig. 3). According to the recent study, the significantly mutated genes in LGG are ARIDIA, CIC, EGFR, IDH1, KAT6B, PIK3R1, TP53 and FLG17. In differential analysis of CRGs expression between mutated and non-mutated groups of the mutated genes above in LGG (Figure S3), we found that NPAS2 showed significant differential expression between the ARID1A mutated group and the non-mutated group. CRY2, PER2, and PER3 displayed significant differential expression between the CIC mutated group and the non-mutated group. ARNTL, ARNTL2, CRY2, NPAS2, NR1D2, PER3, RORB, and RORC exhibited significant differential expression between the EGFR mutated group and the non-mutated group. CRY2 demonstrated significant differential expression between the FLG mutated group and the non-mutated group (Wilcoxon, p = 0.017). ARNTL, ARNTL2, CLOCK, CRY2, NPAS2, RORB, and RORC showed significant differential expression between the IDH1 mutated group and the non-mutated group. PER3 displayed significant differential expression between the KAT6B mutated group and the non-mutated group (Wilcoxon, p = 0.012). ARNTL, ARNTL2, NPAS2, NR1D1, PER2, PER3, RORB, and RORC exhibited significant differential expression between the TP53 mutated group and the non-mutated group. Also, we discovered EGFR, IDH1 and TP53 mutated groups have more altered CRGs via Venn network analysis (Fig. 4A, B).
Then we did the differential gene analysis in immune related genes among CRGs mutation groups. Differential gene expression analysis revealed significant differences between mutated and non-mutated groups for several immune-related genes (Figure S4). Specifically, AADAT showed significant differential expression in ARNTL2 mutated groups (Wilcoxon, p = 0.026), while AAAS showed significant differential expression in the same group (Wilcoxon, p = 0.044). In CREBBP mutated groups, differential expression was found for APITD1, APOBEC3B, LAMP3, CXCL9, TIGIT, TNFRSF9, GPNMB, MMP9, PHEX, and TAP1. Furthermore, the PER3 mutated group exhibited significant differential expression of NR1D1 (Wilcoxon, p = 0.046), while CREBBP mutated groups showed significant differential expression of ARNTL2 and CRY1.
In the correlation analysis of CRGs, significant associations were identified between different genes (Fig. 5A, B). Particularly, a strong positive correlation was observed between NPAS2 and ARNTL2. Additionally, a strong positive correlation was also found between CRY2 and NR1D2. On the other hand, a strong negative correlation was observed between NPAS2 and PER3. Similarly, a strong negative correlation was identified between CRY2 and ARNTL2, further highlighting a potential regulatory interaction between these CRGs.
In the correlation analysis of CRGs and immune-related genes, distinct associations were observed among various gene pairs (Figure S5). Notably, ARNTL1 exhibited a correlation with AAAS (rho = − 0.128, p = 3.52 × 10–3). Furthermore, strong correlations were found between ARNTL2 and A2BP1, AAAS, CXCL9, GPNMB, MMP9, PHEX, TAP1, TIGIT, and TNFRSF9. Similarly, CLOCK displayed positive correlations with A2BP1, CXCL9, PHEX, TIGIT, and TNFRSF9. Moreover, CREBBP showed significant correlations with APITD1, APOBEC3B, GPNMB, and TNFRSF9. Additionally, NPAS2, NR1D1, PER3, RORB, and RORC were positively correlated with AAAS.
CRGs methylation
We have done correlation analysis between CRGs methlation and their mRNA expression. Methylation of various CRGs showed a significant negative correlation with their mRNA expression levels (Fig. 6). Particularly, NPAS2 methylation showed a strong negative correlation with its transcription level (Spearman’s rho: − 0.60, p = 1.21 × 10−53; Pearson’s r: − 0.60, p = 1.75 × 10−53).
Mendelian randomization (MR) studies
In two-sample MR study (Table 2), CREB-binding protein (CREBBP) has a positive causality with benign CNS tumors (Inverse variance weighted beta = 0.2329, p = 0.01911). Besides, TNF receptor superfamily member 11b (TNFRSF11B) shows weak positive causality with benign CNS tumors as well (Weighted median beta = 0.1243, p = 0.03215). Apolipoprotein B has a negative causality with neutrophil cell count and sleeping disorders including trouble falling or staying asleep, or sleeping too much. GPNMB shows positive causality with CD8 + T cell Absolute Count (Wald ratio beta = 0.2068, p = 0.004226). CXCL9 has weak negative causality with daytime nap meanwhile its reduction can be explained by brain tumor. All single SNP analysis, method comparison plots, leave-one-out analysis and funnel plots are shown in Figure S6.
What’s more, we have discovered daytime nap reasons apolipoprotein B and apolipoprotein causes the brain tumor (Table 2). However, there is no causality between daytime nap and brain tumor. Thus, this is the complete mediation case.
In multivariate MR study (Table 3), we tried to discover caualities between multiple kinds of instruments affected by circadian rhythm and CREBBP. These instruments include: variation in diet, leisure/social activities (Sports club or gym), daytime dozing / sleeping (narcolepsy) and mixture of day and night shifts. No significant results were gained (significance level: 0.05).
Discussion
We mainly explored core CRGs in mRNA expression level, survival analysis, and immune cell infiltration levels. We found out CRY1 is significantly associated with increased risk of LGG. Survival analysis also showed CRY1 caused lower OS and DFS. CRY1 has been found to be pro-tumorigenic by promoting DNA repair and cell survival through temporal transcriptional regulation18. CRY1 is hormone-induced in tumors, indicating that circadian hormone might be able to affect LGG prognosis. Also, NPAS2 and RORB were both found to decrease LGG risk. It is suspected that NPAS2 modulates tumorigenesis through the following two aspects: directly carcinogenic mechanism and interaction with other clock proteins19,20. Both NPAS2 and RORB also showed lower OS and DFS in survival analysis. However, NPAS2 might have bidirectional effects in different tumors and deserve further studies. Likewise, although NR1D2 and PER3 do not show significant differece between tumor and normal tissues, they do show significant correlation with survival conditions. It indicates that the mechanism behind how genes influence tumors is complicated and equally-expressed genes deserve more attention in the future studies.
In survival analysis, in ARNTL2 group two KM curves got intersected. From 0 to 8 years, the survival rate was higher when the ARNTL2 expression is higher. It demonstrated that ARNTL2 is anti-tumor during this time. However, after 9 years, it showed the opposite outcome which has been proved to be a potential oncogene, contributing to immunosuppressive tumor microenvironment21. Thus, contradictory results shows the value of continuing to dig up how ARNTL2 influences LGG.
In immune cell infiltration analysis, we detected the negative association between macrophage infiltration and most CRGs. As we have known, tumor-associated macrophages facilitate tumor proliferation, survival and migration22. However, macrophages in tumor micro-environment are categorized into pro-tumorigenic and anti-tumorigenic23, thus making the mechanism of different CRGs on LGG through macrophage infiltration more complicated. Particularly, the correlation between the PER1 gene and immune cell infiltration often exhibits an opposite direction compared to other genes. The downregulation of PER1 can lead to decreased sensitivity of U343 glioma cells to X-ray irradiation by altering the expression of genes associated with cell cycle arrest and apoptosis, such as c-myc, P53, p21, cdc2 and cyclineB1. This is achieved through the activation of the CHK2-P53 pathway, ultimately resulting in reduced apoptosis24. Further studies are needed to figure out how PER1 inhibits tumor growth via affecting different immune cell infiltration.
Most CRGs correlated strongly with B cell and CD8 + T cell infiltration in LGG. This indicates that most CRGs promote or suppress glioma mediated by both immune cells. No significant correlation with neutrophil infiltration except CRY2 and NR1D1 whose high expression has been estimated to high OS and DFS. Altered expression of CRGs including CRY2, PER2 and BMAL1 has been explored to be explained by reduced neutrophil infiltration in liver25. We still has no clue how these genes get modulated by neutrophil infiltration in brain which might uncover the specific function of CRY2 and NR1D1 on glioma development.
Major mutated CRGs in LGG are PER2, BMAL1, CLOCK and BMAL2, which might indicate these genes are most influencial CRGs to glioma development or metastasis. Besides, significant expression difference of most CRGs existed in TP53, EGFR and IDH1 mutated groups. This might imply that CRGs greatly influence LGG patients under those mutation background. In addition, within CRGs mutated groups, some CNS-associated genes like AADAT and AAAS showed significant differencial results. AADAT has been firstly investigated in immune response in meningitis patients26. AAAS may be involved in normal development of the peripheral and central nervous system27,28,29. Also we have discovered AAAS has a significant correlation with many CRGs. There is no clear evidence that both genes are related to LGG but it might hint the interaction between CRGs and these genes thus modulating CNS system via circadian rhythm.
Besides, in mutation differential analysis we have found out many significant results from CREBBP within extended core clock network genes while core CRGs got nothing. CREBBP is a tumor suppressor, whose decreased expression cooperates with the oncogene MYCN to induce malignant brain tumors in mice30. In our two-sample MR study, CREBBP affected benign brain tumors. We found many immune-related genes (APITD1、APOBEC3B、LAMP3、CXCL9、TIGIT、TNFRSF9、GPNMB、MMP9、PHEX、TAP1) expressed differently between CREBBP-mutated group and non-mutated group. Notably, MMP might be important to cancer cell morphology and angiogenesis with the extracellular matrix31. Some of them are even correlated to each other. In our MR studies, we have found some caualities associated with those immune-related genes. Among them, another TNF receptor superfamily has been proved to cause benign CNS tumors. When TNFRSF is present in a gene collection, it can be a valuable molecular marker, a reliable predictor of survival for GBM patients, and a potential target for cancer immunotherapy32. Studies have also shown that immune system matters a lot to tumor development33,34. And CXCL9 modulating daytime nap also builds the bridge between immune response and circadian rhythm. Apolipoprotein B can control sleeping disorders and also neutrophil cell count. In mediation effect analysis, we proved apolipoprotein B completely mediates between daytime nap and brain tumor. This signals that this molecule might be necessary target between circadian rhythm disruption and brain tumor. Also GPNMB affects CD8 + T cell. In this way, continued research about APOBEC3B and GPNMB in immune cell infiltration and circadian rhythm should be carried out. These genes might become potential immune targets to LGG.
Some CRGs have shown strong positive or negative correlation with other CRGs. Moreover, some CRGs mutations have differential expression of some other CRGs. Especially, the negative correlation between NPAS2 methylation and its transcription levels indicates that the regulation of this gene may be inhibited in LGG. Methylation of NPAS2 may disrupt the normal functioning of circadian rhythm, leading to aberrant timing of physiological processes. It could potentially play a role in the development and progression of LGG. The interaction among different CRGs in LGG is undoubted and awaits more studies.
Our study does have several limitations. One potential limitation is our research lacks in vitro experiments to support the theoretical research points. We look forward to future studies that can complement and refine our findings. Besides there are studies showing that miRNAs associated with cancer immunity can be a useful prognostic marker, so more non-coding RNA researches are required to improve our results35,36.
In summary, CRGs play a crucial role in immune response and tumorigenesis in LGG patients. The bridge between circadian rhythm and glioma is expected to strengthened in the near future.
Data availability
Data from GEPIA, TIMER 2.0, cBioPortal and CGDB is freely available and we have cited necessary papers as requested.
References
Aiman, W., Gasalberti, D. P. & Rayi, A. Low-Grade Gliomas. In StatPearls (eds Aiman, W. et al.) (StatPearls Publishing, 2023).
Bollinger, T. & Schibler, U. Circadian rhythms—From genes to physiology and disease. Swiss Med. Wkly. 144, w13984 (2014).
Petkovic, M., Yalçin, M., Heese, O. & Relógio, A. Differential expression of the circadian clock network correlates with tumour progression in gliomas. BMC Med. Genom. 16, 154 (2023).
Lu, D., Zhao, M., Chen, M. & Wu, B. Circadian clock-controlled drug metabolism: Implications for chronotherapeutics. Drug Metab. Dispos. 48, 395–406 (2020).
Liu, Y. et al. CRS: A circadian rhythm score model for predicting prognosis and treatment response in cancer patients. J. Transl. Med. 21, 185 (2023).
Tang, Z. et al. GEPIA: A web server for cancer and normal gene expression profiling and interactive analyses. Nucl. Acids Res. 45, W98–W102 (2017).
Li, T. et al. TIMER2.0 for analysis of tumor-infiltrating immune cells. Nucl. Acids Res. 48, W509–W514 (2020).
Li, T. et al. TIMER: A web server for comprehensive analysis of tumor-infiltrating immune cells. Cancer Res. 77, e108–e110 (2017).
Li, B. et al. Comprehensive analyses of tumor immunity: Implications for cancer immunotherapy. Genome Biol. 17, 174 (2016).
Cerami, E. et al. The cBio cancer genomics portal: An open platform for exploring multidimensional cancer genomics data. Cancer Discov. 2, 401–404 (2012).
de Bruijn, I. et al. Analysis and visualization of longitudinal genomic and clinical data from the AACR project GENIE biopharma collaborative in cBioPortal. Cancer Res. https://doi.org/10.1158/0008-5472.CAN-23-0816 (2023).
Gao, J. et al. Integrative analysis of complex cancer genomics and clinical profiles using the cBioPortal. Sci. Signal https://doi.org/10.1126/scisignal.2004088 (2013).
G, H., et al. The MR-Base platform supports systematic causal inference across the human phenome. eLife https://doi.org/10.7554/eLife.34408 (2018).
Elsworth, B. et al. The MRC IEU OpenGWAS data infrastructure. bioRxiv (2020).
Chen, T., Zhang, H., Liu, Y., Liu, Y.-X. & Huang, L. EVenn: Easy to create repeatable and editable Venn diagrams and Venn networks online. J. Genet. Genom. 48, 863–866 (2021).
Sturm, G. et al. Comprehensive evaluation of transcriptome-based cell-type quantification methods for immuno-oncology. Bioinformatics 35, i436–i445 (2019).
Lin, W., Ou, G. & Zhao, W. Mutational profiling of low-grade gliomas identifies prognosis and immunotherapy-related biomarkers and tumour immune microenvironment characteristics. J. Cell. Mol. Med. 25, 10111–10125 (2021).
Shafi, A. A. et al. The circadian cryptochrome, CRY1, is a pro-tumorigenic factor that rhythmically modulates DNA repair. Nat. Commun. 12, 401 (2021).
Peng, L. U., Bai, G. & Pang, Y. Roles of NPAS2 in circadian rhythm and disease. Acta Biochim. Biophys. Sin. 53, 1257–1265 (2021).
Sancar, A. et al. Circadian clock, cancer, and chemotherapy. Biochemistry 54, 110–123 (2015).
Wu, G. et al. The circadian rhythm key gene ARNTL2: A novel prognostic biomarker for immunosuppressive tumor microenvironment identification and immunotherapy outcome prediction in human cancers. Front. Immunol. 14, 1115809 (2023).
Hambardzumyan, D., Gutmann, D. H. & Kettenmann, H. The role of microglia and macrophages in glioma maintenance and progression. Nat. Neurosci. 19, 20–27 (2016).
Abdelfattah, N. et al. Single-cell analysis of human glioma and immune cells identifies S100A4 as an immunotherapy target. Nat. Commun. 13, 767 (2022).
Zhu, L., Wang, Q., Hu, Y. & Wang, F. The circadian gene Per1 plays an important role in radiation-induced apoptosis and DNA damage in Glioma. Asian Pac. J. Cancer Prev. 20, 2195–2201 (2019).
M, C., et al. Neutrophil infiltration regulates clock-gene expression to organize daily hepatic metabolism. eLife https://doi.org/10.7554/eLife.59258 (2020).
de Souza, F. R. S. et al. Association of kynurenine aminotransferase II gene C401T polymorphism with immune response in patients with meningitis. BMC Med. Genet. 12, 51 (2011).
Handschug, K. et al. Triple A syndrome is caused by mutations in AAAS, a new WD-repeat protein gene. Hum. Mol. Genet. 10, 283–290 (2001).
Tullio-Pelet, A. et al. Mutant WD-repeat protein in triple-A syndrome. Nat. Genet. 26, 332 (2000).
Li, X. et al. Molecular cloning and characterization of AAAS-V2, a novel splice variant of human AAAS. Mol. Biol. Rep. 32, 127 (2005).
Schoof, M. et al. The tumor suppressor CREBBP and the oncogene MYCN cooperate to induce malignant brain tumors in mice. Oncogenesis 12, 36 (2023).
Takashima, Y. et al. Survival prediction based on the gene expression associated with cancer morphology and microenvironment in primary central nervous system lymphoma. PLoS One 16, e0251272 (2021).
Takashima, Y., Kawaguchi, A., Kanayama, T., Hayano, A. & Yamanaka, R. Correlation between lower balance of Th2 helper T-cells and expression of PD-L1/PD-1 axis genes enables prognostic prediction in patients with glioblastoma. Oncotarget 9, 19065–19078 (2018).
Takashima, Y., Kawaguchi, A., Hayano, A. & Yamanaka, R. CD276 and the gene signature composed of GATA3 and LGALS3 enable prognosis prediction of glioblastoma multiforme. PLoS One 14, e0216825 (2019).
Takashima, Y. et al. Differential expression of individual transcript variants of PD-1 and PD-L2 genes on Th-1/Th-2 status is guaranteed for prognosis prediction in PCNSL. Sci. Rep. 9, 10004 (2019).
Takashima, Y. et al. MicroRNA signature constituted of miR-30d, miR-93, and miR-181b is a promising prognostic marker in primary central nervous system lymphoma. PLoS One 14, e0210400 (2019).
Takashima, Y. et al. miR-101, miR-548b, miR-554, and miR-1202 are reliable prognosis predictors of the miRNAs associated with cancer immunity in primary central nervous system lymphoma. PLoS One 15, e0229577 (2020).
Acknowledgements
We appreciate Evenn online platform to provide free service for making venn graphs.
Author information
Authors and Affiliations
Contributions
Weiyu Hou and Xueming Zhao conceived and supervised the study. Weiyu Hou and Weiming Hou contributed to literature searching, study screening, and data collection. Weiyu Hou and Weiming Hou performed data analysis. Weiyu Hou and Weiming Hou wrote and edited the manuscript. All authors approved the final version of the manuscript.
Corresponding author
Ethics declarations
Competing interests
The authors declare no competing interests.
Additional information
Publisher's note
Springer Nature remains neutral with regard to jurisdictional claims in published maps and institutional affiliations.
Supplementary Information
Rights and permissions
Open Access This article is licensed under a Creative Commons Attribution 4.0 International License, which permits use, sharing, adaptation, distribution and reproduction in any medium or format, as long as you give appropriate credit to the original author(s) and the source, provide a link to the Creative Commons licence, and indicate if changes were made. The images or other third party material in this article are included in the article's Creative Commons licence, unless indicated otherwise in a credit line to the material. If material is not included in the article's Creative Commons licence and your intended use is not permitted by statutory regulation or exceeds the permitted use, you will need to obtain permission directly from the copyright holder. To view a copy of this licence, visit http://creativecommons.org/licenses/by/4.0/.
About this article
Cite this article
Hou, W., Hou, W. & Zhao, X. Exploring transcriptomic databases: unraveling circadian gene disruptions in lower grade glioma. Sci Rep 14, 16960 (2024). https://doi.org/10.1038/s41598-024-67559-9
Received:
Accepted:
Published:
DOI: https://doi.org/10.1038/s41598-024-67559-9
- Springer Nature Limited