Abstract
Gastric cancer (GC) is one of the most common and deadliest cancers worldwide. Lipid homeostasis is essential for tumour development because lipid metabolism is one of the most important metabolic reprogramming pathways within tumours. Elucidating the mechanism of lipid homeostasis in GC might significantly improve treatment strategies and patient prognosis. GSE62254 was applied to construct a lipid homeostasis-related gene signature score (HGSscore) by multiple bioinformatic algorithms including weighted gene coexpression network analysis (WGCNA) and LASSO-Cox regression. A nomogram based on HGSscore and relevant clinical characteristics was constructed to predict the survival of patients with GC. TIMER and xCell were used to evaluate immune and stromal cell infiltration in the tumour microenvironment. Correlations between lipid homeostasis-related genes and chemotherapeutic efficacy were analysed in GSCAlite. RT‒qPCR and cell viability assays were applied to verify the findings in this study. HGSscore was constructed based on eighteen lipid homeostasis-related genes that were selected by WGCNA and LASSO-Cox regression. HGSscore was strongly associated with advanced TNM stage and showed satisfactory value in predicting GC prognosis in three independent cohorts. Furthermore, we found that HGSscore was associated with the tumour mutation burden (TMB) and immune/stromal cell infiltration, which are related to GC prognosis, indicating that lipid homeostasis impacts the formation of the tumour microenvironment (TME). With respect to the GSCAlite platform, PLOD2 and TGFB2 were shown to be positively related to chemotherapeutic resistance, while SLC10A7 was a favourable factor for chemotherapy efficacy. Cell viability assays showed that disrupted lipid homeostasis could attenuate GC cell viability. Moreover, RT‒qPCR revealed that lipid homeostasis could influence expression of specific genes. We identified a lipid homeostasis-related gene signature that correlated with survival, clinical characteristics, the TME, and chemotherapeutic efficacy in GC patients. This research provides a new perspective for improving prognosis and guiding individualized chemotherapy for patients with GC.
Similar content being viewed by others
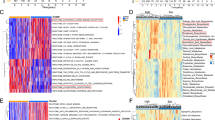
Introduction
Gastric cancer (GC) is a significant health concern in eastern Asia, and GC generally has poor prognosis because it is often diagnosed at an advanced stage1. The TNM stage is still by far the most commonly used prognostic indicator. Nevertheless, several studies have demonstrated that the TNM staging system is insufficient for recent clinical treatment of GC patients2,3. Therefore, exploring a new prognostic tool for gastric cancer is essential.
Lipids are a complex superfamily that includes phospholipids, fatty acids, and cholesterol. These biomolecules not only constitute the basis of biological membranes but also participate in the energy supply process and intracellular signal transduction. In our previous study, we found that fatty acids derived from autophagy were needed for cancer cell survival4. Moreover, we revealed that the lipid metabolism patterns of GC tissues differ from those of normal stomach tissues, which is a risk factor for patient survival5. In addition, it has been reported that altered lipid metabolism is among the most prominent metabolic alterations in cancer6, and lipid homeostasis is crucial for maintaining cancer cell malignancy7. We are interested in determining whether lipid homeostasis can predict the prognosis of GC patients.
In this study, we demonstrated the importance of lipid homeostasis in GC and further explored a series of genes associated with the expression pattern of lipid homeostasis. Additionally, we defined this gene set as the lipid homeostasis-related gene signature score (HGSscore) and evaluated its prognostic value in three independent GC cohorts. Finally, we analysed correlations between HGSscore and immune/stromal cell infiltration and between HGSscore and chemotherapeutic efficacy.
Materials and methods
Cohorts and data processing
For confidence in this study, three independent cohorts involving sufficient cases were analysed in this research, namely, GSE622548 and GSE844379 from the Gene Expression Omnibus (GEO) database and TCGA-STAD from The Cancer Genome Atlas (TCGA). The GSE62254 dataset was used as the training cohort to construct the prognostic prediction model, and external validation was performed in the other two cohorts. All the data were analysed with R software.
Single sample gene set enrichment analysis
The package “GSVA” in R software was used to perform single-sample gene set enrichment analysis (ssGSEA). ssGSEA was used to explore the expression patterns of genes related to lipid homeostasis and other tumour-related pathways (Supplementary Table S1) for analysis. Tumour-related pathway information was obtained from MsigDB.
Weighted gene coexpression network analysis
Weighted gene coexpression network analysis (WGCNA) was performed based on the whole-transcriptome profile of GSE62254. After eliminating the genes above the cut-off height, we selected 3 as the best soft threshold, and the minimum number of genes within a module was 60. The module that was significantly associated with lipid homeostasis was retained for further study.
Establishment and validation of HGSscore based on the LASSO-Cox algorithm
Using univariate Cox regression, overall survival (OS)-related genes were identified from the selected module. Subsequently, LASSO-Cox regression was conducted to determine genes highly correlating with patient prognosis and their correlation coefficients. The above parameters were used to calculate the lipid homeostasis-related gene signature score (HGSscore), which was calculated as Σ(coefficient * gene expression). Next, the HGSscore was validated for various clinical characteristics, such as TNM stage, OS, and disease-free survival (DFS), in all patients. The R packages “survival” and “rms” were applied to construct a nomogram based on HGSscore and other clinical characteristics. Finally, 5-, 6-, and 7-year survival probabilities were estimated by calibration curves.
External validation of HGSscore
The Xtile program was used to distinguish HGSscore-high and -low groups in the validation cohort. K‒M OS curves were generated to determine the prognostic differences between HGSscore-high and -low groups in the GSE84437 and TCGA-STAD cohorts. In addition, we conducted multivariate Cox regression to validate the efficiency of the model.
Infiltration of immune and stromal cells
We used the TIMER online server to estimate correlations between tumour-infiltrating immune cells and the highest 2 risk and protective genes, TGFB2, UBE2D1, NAB2, and ASPRV1. The xCell10 algorithm was subsequently used to evaluate infiltration of various stromal and immune cells in the TME. The ESTIMATE algorithm was applied to assess the tumour purity of the GC tissue.
Prediction of chemotherapeutic effect
The GSCAlite11 platform was used to predict chemotherapeutic efficacy. The relationship between HGSscore and chemotherapeutic efficacy was evaluated by Spearman correlation analysis.
Cell viability and RT‒qPCR
SGC7901 cells were incubated in DMEM containing 10% FBS (Promega). For the cell viability assay, 10,000 cells were seeded in 96-well plates overnight. Then, the cells were exposed to various lipids (Aladdin), including unsaturated fatty acids such as arachidonic acid (AA), α-linolenic acid (ALA), docosahexaenoic acid (DHA), eicosapentaenoic acid (EPA), linolenic acid (LA), and γ-linolenic acid (γLA), and saturated fatty acids such as C15, 17, and C18 at 20 µM for 24 h. Cell viability was evaluated using a CCK8 assay (Biosharp). For the RT‒qPCR assay, 600,000 cells were seeded in 6-well plates. The next day, the cells were treated with ethanol and 20 µM γLA for 24 h. Total mRNA was extracted with RNAiso Plus (Takara) and reverse transcribed to cDNA. For each sample, a total volume of 20 µl was used, containing 4 µl of mRNA, 6 µl of Master Mix (Takara) and 10 µl of RNase-free H2O, and the mixture was diluted to 160 µl after the reaction. Finally, we performed RT‒qPCR using TB Green (Takara) and a StepOne Real-Time PCR System in a reaction including 4 µl of cDNA, 5 µl of TB Green II, 0.8 µl of reverse and forward primers and 0.2 µl of Rox in each well. The primers used are listed in Supplementary Table S2.
Statistical analysis
Data were analysed with R software (version 4.2.3). mRNA expression levels of the genes and LASSO-Cox coefficients were used to construct the HGSscore. The hazard ratio (HR) in the forest plot was determined via univariate Cox and multivariate Cox regression analyses. K‒M curves and log-rank tests were used for survival analysis. Differences between groups were assessed using the Wilcoxon test. The level of statistical significance was 0.05.
Results
Prognostic value of lipid homeostasis and identification of HGSscore
The expression pattern of genes related to lipid homeostasis was assessed based on the transcriptome profile from GSE62254 using ssGSEA. The association between lipid homeostasis and 5-year OS in patients with GC was evaluated by using Kaplan‒Meier curves. We found that lipid homeostasis was significantly associated with 5-year OS (Fig. 1a). WGCNA was applied to construct a gene coexpression network to select the lipid homeostasis-related module from the whole transcriptome profile (Fig. 1b). The optimal soft threshold was set to three to achieve a scale-free network (Fig. 1c). Seventeen modules were identified. The yellow module correlated with lipid homeostasis the most (Fig. 1d–f). The genes within the yellow module were enriched in oxidoreductase activity, hexosyltransferase activity, and UDP-glycosyltransferase activity (Fig. 2a). Univariate Cox (Supplementary Table S3) and LASSO-Cox (Fig. 2b,c) analyses were used to identify highly prognosis-related genes. Finally, 18 genes and their coefficients were extracted for further study. We found that TGFB2, NAB2, PJA1, ZNF382, GPX3, and PLOD2 were risk factors for GC; the other factors were protective factors for GC (Fig. 2d).
WGCNA algorithm for the GSE62254 cohort. (a) Kaplan–Meier OS curve showing that lipid homeostasis was significantly associated with worse GC prognosis. (b) Clustering dendrogram of samples from WGCNA. (c) The optimal soft threshold for the GSE62254 cohort. (d) Clustering dendrogram of all genes. (e) Heatmap of the correlations between the modules and lipid homeostasis. (f) Correlations between genes within the yellow module and lipid homeostasis.
Establishment of HGSscore in the GSE62254 cohort. (a) Gene Ontology (GO) enrichment for genes in the yellow module. (b) LASSO coefficient profiles of lipid homeostasis-related prognostic genes. (c) Tenfold cross-validation for penalty parameter λ selection in the LASSO model. (d) LASSO coefficients of 18 selected genes. (e) K‒M OS curve for patients in the HGSscore-high and -low groups. (f) Kaplan–Meier DFS curve for patients in the HGSscore-high and -low groups.
HGSscore for each patient was calculated using the mRNA expression level of the genes and their LASSO coefficients. Then, the GSE62254 dataset was divided into HGSscore-high and -low groups using the median HGSscore as the cut-off. K‒M OS and DFS curves demonstrated that the two groups had significantly different prognoses (Fig. 2e,f).
HGSscore and clinical characteristics in the training cohort
A nomogram was developed based on clinical characteristics and HGSscore to predict the 5-, 6-, and 7-year OS of patients with GC (Fig. 3a). The areas under the ROC curves were 0.8305, 0.8296, and 0.7902, respectively (Fig. 3b). Calibration curves revealed great consistency between the nomogram-predicted OS and actual OS (Fig. 3c, Supplementary Fig. S1). Cox multivariate regression demonstrated that HGSscore was an independent risk factor for GC prognosis (Fig. 3d). Subsequently, correlations between HGSscore and pathological characteristics revealed that HGSscore was significantly associated with advanced TNM and clinical stage (Fig. 3e). GSEA of the HGSscore-high cohort revealed that the DEGs are related to cancer hallmark pathways, including angiogenesis, EMT, FA (fatty acid) metabolism, HYPOXIA, and OXPHOS, as well as the lipid homeostasis pathway (Fig. 3f,g). Finally, we found that patients with higher HGSscore often had worse prognoses (Fig. 3h).
Validation of HGSscore in the GSE62254 cohort. (a) Nomogram based on HGSscore and clinical characteristics. (b) ROC curves at 5, 6, and 7 years. (c) Calibration curve for five-year OS. (d) Forest plot for multiple Cox regression coefficients, including HGSscore and clinical characteristics. (e) Correlations between HGSscore and clinical characteristics. (f,g) GSEA of several cancer hallmarks and lipid homeostasis pathways based on HGSscore-DEGs. (h) HGSscore of surviving and deceased groups.
Validation of HGSscore in the TCGA and GEO cohorts
To validate the prognostic value of HGSscore, we evaluated two independent cohorts (TCGA-STAD and GSE84437). In the TCGA-STAD cohort, lipid homeostasis was the riskiest pathway (HR = 2.41, p = 0.0051) among the 12 lipid-related pathways (Fig. 4a). K‒M curves demonstrated a significant difference in OS between patients in the HGSscore-high and -low groups (Fig. 4b). Cox multivariate regression also revealed that HGSscore was an independent risk factor for patient survival in patients with GC (Fig. 4c). Notably, we found that patients with higher HGSscore had lower TMBs (Fig. 4d), which indicated worse ICI response in this population. To extend the relationship between HGSscore and TMB, a comprehensive landscape of TMB in TCGA-STAD was constructed. We found that missense mutations dominated the variant subtypes in GC and that C>T single nucleotide polymorphisms accounted for more than 50% of the variant subtypes (Fig. 4e). Among the top 20 mutated genes, TTN, TP53, MUC16, ARID1A, and LRP1B were the most frequently mutated (Fig. 4e). We further analysed differences in TMB between the HGSscore-high and HGSscore-low groups and found that TP53 was frequently mutated in the HGSscore-high subgroup (Fig. 4f) and that the TMB profile in the HGSscore-low subgroup was similar to that in the general population (Fig. 4g).
Validation of lipid homeostasis and HGSscore in the TCGA-STAD cohort. (a) Forest plot for univariate Cox regression of 12 lipid metabolism pathways. (b) K‒M OS curve for patients in the HGSscore-high and -low groups. (c) Forest plot for multivariate Cox regression, including HGSscore and clinical characteristics. (d) Comparison of total TMB in the HGSscore-high and -low groups. (e) Global landscapes of somatic mutations in TCGA-STAD cohort. (f,g) Top 20 somatic mutations in the HGSscore-high and -low groups.
HGSscore also showed great prognostic value in the GSE84437 cohort (Supplementary Fig. S2). K‒M curves revealed that patients in different groups had completely different prognoses (p < 0.001).
Mechanism of HGSscore in the tumour microenvironment
Three immune/stromal cell infiltration predictive tools were used to reveal the potential mechanism of HGSscore within the TME. Specifically, TIMER was used to reveal the correlation between a single gene in HGSscore and immune cell infiltration. The highest risk and protective genes (TGFB2, NAB2, UBE2D1, and ASPRV1) in HGSscore were also analysed. TGFB2 is related to macrophage infiltration and that NAB2 is associated with CD4+ T-cell infiltration. UBE2D1 correlates significantly with DC infiltration, and ASPRV1 is related to CD4+ T-cell infiltration (Fig. 5a).
Correlations between HGSscore and tumour microenvironment components. (a) Immune cell infiltration predicted by TIMER. (b) Heatmaps displaying the correlation between HGSscore and various immune or stromal cells according to the xCell dataset. (c) Hazard ratio of 12 selected immune and stromal cells. (d) The correlation between the stromal score (xCell) and HGSscore. (e) Comparison of PDL1 expression in the HGSscore-high and -low groups. (f) Association between HGSscore and tumour purity according to the ESTIMATE database. (g) The correlation between the stromal score (ESTIMATE) and HGSscore.
Additionally, the xCell algorithm was applied to outline relationships between various immune/stromal cells and HGSscore (Fig. 5b). For immune cell infiltration, HGSscore was found to be negatively associated with NKT, Th1 cells, Tgd cells, basophils, pro-B cells, CD4+ memory T cells, and pDCs and positively related to CD8+ naive T cells in the TME. The correlations between HGSscore and stromal cells were much more complex than those between HGSscore and stromal cells, and HGSscore correlated with many stromal cells, such as epithelial cells and fibroblasts. Moreover, univariate Cox regression was used to evaluate the prognostic value of twelve immune/stromal cells (Fig. 5c). Among these selected cells, ly endothelial cells (lymphatic endothelial cells) were the highest risk factor (HR = 5.02) for GC, whereas pro-B cells were the most protective factor (HR = − 11.99). Spearman correlation analysis revealed that HGSscore correlated positively with the stromal score according to the xCell dataset (Fig. 5d). By comparing PDL1 mRNA levels, we found that PDL1 was lower in the HGSscore-high group (Fig. 5e).
Finally, ESTIMATE was used to assess tumour purity in the TME. The Wilcoxon test showed that tumour purity was slightly greater in the HGSscore-low subgroup than in the HGSscore-high subgroup (Fig. 5f). Interestingly, correlation analysis indicated that HGSscore was positively related to the stromal score determined via the ESTIMATE tool (Fig. 5g).
Relationship between HGSscore and chemotherapeutic efficacy
The Cancer Therapeutics Response Portal (CTRP) and Genomics of Drug Sensitivity in Cancer (GDSC) databases were used to demonstrate the correlation between HGSscore and chemotherapeutic efficacy. We found that eight genes, SLC10A7, ASPRV1, ZNF382, CDC42EP5, GPX3, STYK1, TGFB2, and PLOD2, were significantly associated with the chemotherapeutic effect of GC on CTRP. Among these genes, SLC10A7, ASPRV1, and ZNF382 are related to chemotherapy response; CDC42EP5, GPX3, STYK1, TGFB2, and PLOD2 are related to chemotherapy resistance (Fig. 6a). In GDSCs, PLOD2 is considered to be linked to drug resistance, and SLC10A7 and ASPRV1 are linked to drug response (Supplementary Fig. S3). GSEA of gene sets related to doxorubicin, cisplatin, and fluorouracil resistance revealed that patients in the HGSscore-high cohort had a satisfactory response to doxorubicin, though they were resistant to cisplatin and fluorouracil therapy (Fig. 6b).
Disruption of the lipid homeostasis pathway mediated by exogenous lipids
To determine the effect of lipid homeostasis on GC, we detected relative cell viability and expression of several mRNAs in the SGC7901 cell line treated with fatty acids. According to the results of the cell viability assay, SGC7901 cells exposed to lipids exhibited reduced cell viability compared to those in the ethanol group (Supplementary Fig. S4a). RT‒qPCR revealed that ASPRV1 was upregulated after treatment; the other five genes were downregulated (Supplementary Fig. S4b). Taken together, these findings suggest that lipid homeostasis has an impact on GC cells.
Discussion
In the last decade, treatment of gastric cancer has progressed continuously, but prognostic information has not been updated much. Lipid homeostasis is widely reported to be critical for GC growth12, metastasis13, chemotherapeutic resistance14, and immunity15. However, studies that highlight the correlation between lipid homeostasis and GC prognosis from both clinical and molecular biological perspectives are limited.
In the present study, we found lipid homeostasis to be a risk factor for GC prognosis, and a GC prognostic model was constructed based on eighteen lipid homeostasis-related genes. As expected, this model demonstrated satisfactory value in risk stratification, survival prediction, tumour immunity, and chemotherapeutic response. Recently, several studies have explored the clinical value of altered lipidomic profiles in tumour tissue or serum16,17,18 and revealed that abnormal lipid metabolites may lead to poor clinical outcomes. In colorectal cancer16, the triacylglycerol (TG) profile is significantly associated with disease-free survival and lymphovascular invasion. In addition, lipogenic genes such as fatty acid synthase (FASN) were found to be significantly upregulated in CRC patients and risk factors for patient survival. In summary, lipids not only play a prominent role in clinical progression of cancer but also predict the prognosis of patients precisely.
The TME consists of various cell types embedded in the extracellular matrix, including cancer cells, immune cells, and stromal cells, which contribute to an immunosuppressive environment during the progression of cancer. Recent studies have elucidated the important role of lipids in remodelling the TME. In this study, we found that the HGSscore was negatively associated with infiltration of immune cells, including CD8+ T cells, DCs, NKT cells, and Th1 cells. Accumulated evidence has demonstrated that lipids can promote CD8+ T-cell exhaustion and further undermine CD8+ T-cell function19,20. In 2020, Alison E. Ringel reported that high-fat diet-induced obesity could impair CD8+ T-cell function and promote tumour growth21, which proved that systemic disruption of lipid homeostasis could affect intratumoral immunity. In addition, fatty acids in tumour-derived exosomes induce immune dysfunction in DCs via PPAR-alpha activation and facilitate immune evasion in colon cancer22. Another study reported that tumours impair DC function through induction of the ER stress response and disruption of lipid metabolic homeostasis23. Wenshu Tang revealed that NKT cell function is inhibited by mTORC1/SREBP2-induced cholesterol accumulation and subsequently promotes progression of obesity-related HCC24. In inflammatory disease, investigators have shown that cholesterol homeostasis is essential for Th1 cells to accomplish effector-regulatory switching, and perturbations in cholesterol flux are risk factors for RA25.
Moreover, this study showed that lipid homeostasis is strongly associated with stromal cell infiltration. Fibroblasts in the TME are also known as carcinoma-associated fibroblasts (CAFs) and are critically associated with tumorigenesis and tumour progression by boosting angiogenesis, cancer cell proliferation, and invasion. Gui-Qi Zhu reported that CD36+ CAFs in hepatocellular carcinoma exhibit high lipid metabolism and secrete macrophage migration inhibitory factor via the lipid peroxidation/p38/CEBPs axis, leading to an immunosuppressive TME and tumour stemness26. Additionally, after reprogramming of lipid metabolism, lipid metabolites secreted by CAFs were proven to promote CRC migration in a CD36-dependent manner27. Interestingly, prostaglandin E2 (PGE2), a common lipid inflammatory factor, is considered to be a key molecule in CAFs that regulates breast cancer growth and metastasis28. In our study, lipid homeostasis was shown to facilitate CAF infiltration, which is a risk factor for GC prognosis.
Endothelial cells are the basis of blood and lymphatic vessels in the TME. However, these vessels also exhibit abnormalities in their structure and function, which results in a hostile tumour microenvironment and tumour metastasis29. Vincent Geldhof identified a distinct endothelial cell type, LIPEC, in breast cancer, which was characterized by an active lipid metabolism pathway30. Patients with more LIPEC may be considered to benefit from metformin treatment. LPA and S1P are two critical lipid mediators for tumour angiogenesis. A recent review concluded that LPA and S1P are crucial for endothelial cell migration and promoting tumour invasion and metastasis via specific GPCR pathways31.
Furthermore, we found that lipid homeostasis correlated with the tumour mutation burden (TMB) and chemotherapeutic efficacy. An analysis involving 206 GC patients and 435 cancer-related genes was conducted to explore the prognostic value of the TMB and mutational signatures in GC32. The data from this research showed that the TMB-high subgroup had a relatively worse prognosis, greater lipid metabolism activity and more frequent metabolic disorders than the TMB-low subgroup, indicating that immunotherapy in combination with metabolic inhibitors may be a novel treatment for GC. Nevertheless, our study revealed that TP53 was the most frequently mutated gene in the TMB-high cohort, which demonstrated a notable potential for GC management.
Accumulating evidence has indicated that lipids participate in drug resistance through multiple mechanisms. Recently, investigators have shown that free fatty acids generated by cancer-associated adipocytes (CAAs) can induce antiangiogenic drug (AAD) resistance33,34, and AADs, such as bevacizumab, are widely used in various cancers. Specifically, hypoxia-related lipid metabolic reprogramming in adipocytes was found to be triggered by hypoxia, which revealed the intensive communication between these two cancer hallmarks. Carnitine palmitoyltransferase (CPT), the key enzyme for fatty acid transportation, not only promotes AAD resistance but also facilitates oxaliplatin resistance; oxaliplatin is a fundamental chemical in most chemotherapy regimens14,33. In addition, ferroptosis is a novel cell death pathway characterized by accumulation of lipid ROS35. Several studies have demonstrated that ferroptosis can regulate acquired chemotherapy resistance in gastrointestinal cancers36,37 through lipid-related enzymes and proteins that play pivotal roles in this process. In this study, we found that lipid homeostasis correlated significantly with multidrug resistance in GC. In particular, PLOD2 is reported to stimulate the cancer stem cell phenotype and cisplatin resistance in cancer38. TGFB2 is a common cytokine involved in intercellular communication and FOLFOX resistance39, and we found that it was strongly associated with chemoresistance.
Conclusion
In this study, we constructed a novel gene signature based on 18 lipid homeostasis-related genes that performed well in predicting the prognosis of GC. Subsequently, the correlation between lipid homeostasis and immune/stromal cell infiltration was illustrated, which might be the potential mechanism through which lipids act in the TME. Finally, we identified several genes of HGSscore that were associated with chemotherapeutic response or resistance. Although further prospective studies in larger cohorts are needed to validate the utility of HGSscore, its ability to predict patient prognosis and guide individualized chemotherapy should not be ignored.
Data availability
All data are publicly available in this study. GSE84437 and GSE62254 can be accessed at https://www.ncbi.nlm.nih.gov/gds. TCGA-STAD can be accessed at https://portal.gdc.cancer.gov/projects/TCGA-STAD. MsigDB can be accessed at https://www.gsea-msigdb.org/gsea/msigdb. And TIMER can be accessed at https://cistrome.shinyapps.io/timer/.
References
Sung, H. et al. Global cancer statistics 2020: GLOBOCAN estimates of incidence and mortality worldwide for 36 cancers in 185 countries. CA Cancer J. Clin. 71(3), 209–249 (2021).
Jiang, Y. et al. ImmunoScore signature: A prognostic and predictive tool in gastric cancer. Ann. Surg. 267(3), 504–513 (2018).
Yamashita, K. et al. Prognostic impacts of the combined positive score and the tumor proportion score for programmed death ligand-1 expression by double immunohistochemical staining in patients with advanced gastric cancer. Gastric Cancer 23(1), 95–104 (2020).
Cai, M. et al. Phospholipase D1-regulated autophagy supplies free fatty acids to counter nutrient stress in cancer cells. Cell Death Dis. 7(11), e2448 (2016).
Xiong, Z. et al. Exploration of lipid metabolism in gastric cancer: A novel prognostic genes expression profile. Front. Oncol. 11, 712746 (2021).
Snaebjornsson, M. T., Janaki-Raman, S. & Schulze, A. Greasing the wheels of the cancer machine: The role of lipid metabolism in cancer. Cell Metab. 31(1), 62–76 (2020).
Ackerman, D. et al. Triglycerides promote lipid homeostasis during hypoxic stress by balancing fatty acid saturation. Cell Rep. 24(10), 2596–2605.e5 (2018).
Cristescu, R. et al. Molecular analysis of gastric cancer identifies subtypes associated with distinct clinical outcomes. Nat. Med. 21(5), 449–456 (2015).
Cheong, J. H. et al. Predictive test for chemotherapy response in resectable gastric cancer: A multi-cohort, retrospective analysis. Lancet Oncol. 19(5), 629–638 (2018).
Aran, D., Hu, Z. & Butte, A. J. xCell: Digitally portraying the tissue cellular heterogeneity landscape. Genome Biol. 18(1), 220 (2017).
Liu, C. J. et al. GSCALite: A web server for gene set cancer analysis. Bioinformatics 34(21), 3771–3772 (2018).
Yang, Q. et al. MicroRNA miR-1275 coordinately regulates AEA/LPA signals via targeting FAAH in lipid metabolism reprogramming of gastric cancer. Cell Death Dis. 14(1), 62 (2023).
Zhou, X. et al. Dysregulated ceramides metabolism by fatty acid 2-hydroxylase exposes a metabolic vulnerability to target cancer metastasis. Signal Transduct. Target Ther. 7(1), 370 (2022).
Wang, Y. et al. Inhibition of fatty acid catabolism augments the efficacy of oxaliplatin-based chemotherapy in gastrointestinal cancers. Cancer Lett. 473, 74–89 (2020).
Liao, P. et al. CD8(+) T cells and fatty acids orchestrate tumor ferroptosis and immunity via ACSL4. Cancer Cell 40(4), 365–378.e6 (2022).
Ecker, J. et al. The colorectal cancer lipidome: Identification of a robust tumor-specific lipid species signature. Gastroenterology 161(3), 910–923.e19 (2021).
Wolrab, D. et al. Lipidomic profiling of human serum enables detection of pancreatic cancer. Nat. Commun. 13(1), 124 (2022).
Butler, L. M. et al. Lipidomic profiling of clinical prostate cancer reveals targetable alterations in membrane lipid composition. Cancer Res. 81(19), 4981–4993 (2021).
Ma, X. et al. Cholesterol induces CD8(+) T cell exhaustion in the tumor microenvironment. Cell Metab. 30(1), 143–156.e5 (2019).
Xu, S. et al. Uptake of oxidized lipids by the scavenger receptor CD36 promotes lipid peroxidation and dysfunction in CD8(+) T cells in tumors. Immunity 54(7), 1561–1577.e7 (2021).
Ringel, A. E. et al. Obesity shapes metabolism in the tumor microenvironment to suppress anti-tumor immunity. Cell 183(7), 1848–1866.e26 (2020).
Yin, X. et al. PPARalpha inhibition overcomes tumor-derived exosomal lipid-induced dendritic cell dysfunction. Cell Rep. 33(3), 108278 (2020).
Cubillos-Ruiz, J. R. et al. ER stress sensor XBP1 controls anti-tumor immunity by disrupting dendritic cell homeostasis. Cell 161(7), 1527–1538 (2015).
Tang, W. et al. Aberrant cholesterol metabolic signaling impairs antitumor immunosurveillance through natural killer T cell dysfunction in obese liver. Cell Mol. Immunol. 19(7), 834–847 (2022).
Perucha, E. et al. The cholesterol biosynthesis pathway regulates IL-10 expression in human Th1 cells. Nat. Commun. 10(1), 498 (2019).
Zhu, G. Q. et al. CD36(+) cancer-associated fibroblasts provide immunosuppressive microenvironment for hepatocellular carcinoma via secretion of macrophage migration inhibitory factor. Cell Discov. 9(1), 25 (2023).
Gong, J. et al. Reprogramming of lipid metabolism in cancer-associated fibroblasts potentiates migration of colorectal cancer cells. Cell Death Dis. 11(4), 267 (2020).
Elwakeel, E. et al. Disruption of prostaglandin E2 signaling in cancer-associated fibroblasts limits mammary carcinoma growth but promotes metastasis. Cancer Res. 82(7), 1380–1395 (2022).
Carmeliet, P. & Jain, R. K. Principles and mechanisms of vessel normalization for cancer and other angiogenic diseases. Nat. Rev. Drug Discov. 10(6), 417–427 (2011).
Geldhof, V. et al. Single cell atlas identifies lipid-processing and immunomodulatory endothelial cells in healthy and malignant breast. Nat. Commun. 13(1), 5511 (2022).
Hisano, Y. & Hla, T. Bioactive lysolipids in cancer and angiogenesis. Pharmacol. Ther. 193, 91–98 (2019).
Cheng, Y. et al. Genomic and transcriptomic profiling indicates the prognosis significance of mutational signature for TMB-high subtype in Chinese patients with gastric cancer. J. Adv. Res. 51, 121–134 (2022).
Iwamoto, H. et al. Cancer lipid metabolism confers antiangiogenic drug resistance. Cell Metab. 28(1), 104–117.e5 (2018).
Cao, Y. Adipocyte and lipid metabolism in cancer drug resistance. J. Clin. Investig. 129(8), 3006–3017 (2019).
Stockwell, B. R. et al. Ferroptosis: A regulated cell death nexus linking metabolism, redox biology, and disease. Cell 171(2), 273–285 (2017).
Zhang, H. et al. CAF secreted miR-522 suppresses ferroptosis and promotes acquired chemo-resistance in gastric cancer. Mol. Cancer 19(1), 43 (2020).
Zhang, Q. et al. Adipocyte-derived exosomal MTTP suppresses ferroptosis and promotes chemoresistance in colorectal cancer. Adv. Sci. 9(28), e2203357 (2022).
Song, M. et al. Silencing PLOD2 attenuates cancer stem cell-like characteristics and cisplatin-resistant through Integrin beta1 in laryngeal cancer. Transl. Oncol. 22, 101460 (2022).
Tang, Y.-A. et al. Hypoxic tumor microenvironment activates GLI2 via HIF-1α and TGF-β2 to promote chemoresistance in colorectal cancer. Proc. Natl. Acad. Sci. https://doi.org/10.1073/pnas.1801348115 (2018).
Funding
This study was supported by grants from the National Natural Science Foundation of China (Grant numbers: 81972881), and Natural Science Foundation of Hubei Province of China (2019CFB514).
Author information
Authors and Affiliations
Contributions
Conceptualization, M.C. and C.L.; Software, C.L. and Z.X.; Validation, Z.X., and J.H.; Formal analysis, C.L. and J.H.; Data curation, C.L.; Original draft preparation, Z.X. and J.H.; Review and editing, W.N., J.G., Z.W. and K.C.; Project administration, G.W., K.T. and M.C.; Funding acquisition, M.C.
Corresponding author
Ethics declarations
Competing interests
The authors declare no competing interests.
Additional information
Publisher's note
Springer Nature remains neutral with regard to jurisdictional claims in published maps and institutional affiliations.
Rights and permissions
Open Access This article is licensed under a Creative Commons Attribution 4.0 International License, which permits use, sharing, adaptation, distribution and reproduction in any medium or format, as long as you give appropriate credit to the original author(s) and the source, provide a link to the Creative Commons licence, and indicate if changes were made. The images or other third party material in this article are included in the article's Creative Commons licence, unless indicated otherwise in a credit line to the material. If material is not included in the article's Creative Commons licence and your intended use is not permitted by statutory regulation or exceeds the permitted use, you will need to obtain permission directly from the copyright holder. To view a copy of this licence, visit http://creativecommons.org/licenses/by/4.0/.
About this article
Cite this article
Li, C., Xiong, Z., Han, J. et al. Identification of a lipid homeostasis-related gene signature for predicting prognosis, immunity, and chemotherapeutic effect in patients with gastric cancer. Sci Rep 14, 2895 (2024). https://doi.org/10.1038/s41598-024-52647-7
Received:
Accepted:
Published:
DOI: https://doi.org/10.1038/s41598-024-52647-7
- Springer Nature Limited