Abstract
The blood-based Ag and TiO2 Hybrid nanofluids (HNFs) flow between the two tubes are used for drug delivery applications. Ag and TiO2 hybrid nanofluids have immense potential as drug delivery agents due to their unique properties, controlled release capabilities, targeting abilities, and synergistic effects. Extensive research is being conducted to optimize their design and maximize their effectiveness in various therapeutic applications using experimental approaches. The recent work has been focused on theoretical analysis using the existing experimental data. These HNFs are functionalized with ligands or antibodies to specifically target and deliver drugs to diseased tissues or cells. This targeted approach enhances drug accumulation at the desired site, minimizing systemic toxicity and improving treatment outcomes. An external magnetic field is applied to control the release of drugs from the nanofluids. Magnetic nanoparticles such as iron oxide nanoparticles are incorporated into the nanofluids, which respond to the magnetic field and release the drug at a specific location and time. This offers a controlled and targeted drug delivery system. The graphical and numerical outcomes of the dimensionless momentum and thermal boundary layers are investigated and discussed. It is observed that hybrid nanofluids (HNFs) often exhibit superior heat transfer (HT) properties, primarily due to the high thermal conductivity of nanoparticles. Improving heat transfer helps reduce skin friction by maintaining a more uniform temperature distribution near the surface. Also, this acts in the optimization of the blood flow analysis. In terms of drug delivery applications, hybrid nanofluids are more prominent in refining applications through optimized heat transfer, as shown by the comparison.
Article Highlights
-
The blood-based Ag and TiO2 hybrid nanofluids flow between the two concentric cylinders is used for the applications of drug delivery and is a novel attempt for such kind of model.
-
The inclusion of the magnetic field enhances the ability of the Ag and TiO2 nanomaterials to work a the drug carrier. Thermal radiation also works to improve the thermal performance of the temperature and enhance the heat transfer rate.
-
The CVFEM, RK-4, and ANN are the three different techniques that are used in combination to handle the proposed model. Also, the comparison with the existing literature validates the obtained results
Similar content being viewed by others
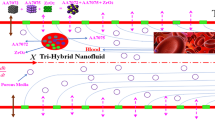
1 Introduction
The fluid flow between two concentric cylinders plays a crucial role in drug delivery systems and other engineering, biomedical, and industrial applications. In the case of biomedical engineering, the fluid flow between the two cylinders regulates the drug release. By adjusting the flow rate, the drug is released evenly or in a controlled manner over a specific period. This is particularly useful for sustained or controlled drug delivery systems, where the drug needs to be released at a specific rate to achieve the desired therapeutic effect [1, 2]. The fluid flow between the two cylinders promotes mixing and homogeneity of the drug solution. This is important to ensure uniform drug concentration throughout the delivery system. By achieving homogeneity, the drug can be administered consistently and accurately, avoiding potential variation in dosage. The fluid flow between the cylinders increases the rate of mass transfer, allowing for more efficient drug diffusion or dissolution [3,4,5]. This is particularly beneficial when dealing with drugs that have low solubility or limited permeation across biological barriers. The enhanced mass transfer helps to increase drug absorption and bioavailability. The fluid flow between the cylinders is used to guide and direct the drug to specific target sites [6]. By manipulating the fluid flow, the drug is delivered to a specific region or tissue, ensuring precise drug targeting and reducing potential side effects on other healthy tissues. The fluid flow between the cylinders replicates physiological conditions, such as blood flow or fluid movement in organs. This is important to mimic in vivo conditions for drug development and testing. By simulating the fluid flow, drug delivery systems can be optimized and validated under realistic conditions, enhancing their effectiveness and safety. Hybrid nanofluids, which consist of nanoparticles suspended in a base fluid, play a crucial role in drug delivery using flow between two tubes. The nanoparticles in hybrid nanofluids enhance the solubility of hydrophobic drugs by providing a large surface area for drug loading. This improved solubility allows for a higher drug concentration in the fluid, leading to better drug delivery efficiency. Hybrid nanofluids are engineered to have specific properties to enable controlled drug release. Hybrid nanofluids are utilized as carriers for controlled and sustained drug release. The nanoparticles are functionalized with drugs and encapsulated within polymeric matrices, allowing for controlled release over a prolonged period. This enables targeted drug delivery and reduces the frequency of drug administration. The small size and large surface area-to-volume ratio of hybrid nanofluids enhance drug bioavailability. The nanoparticles efficiently penetrate physiological barriers, such as cell membranes or blood–brain barrier, and deliver the drug to the desired site of action. This leads to improved therapeutic efficacy and reduced side effects [7].
By modifying the nanoparticles’ surface properties or incorporating stimuli-responsive materials, the release of the drug is triggered in a controlled manner, such as in response to changes in temperature, pH, or light exposure. The flow between two tubes is designed to mimic blood vessels or specific organs. By incorporating targeting ligands on the surface of the nanoparticles in the nanofluid, they can selectively bind to receptors or proteins present in the target cells, allowing for site-specific drug delivery. This targeting strategy improves drug efficacy while minimizing potential side effects on healthy cells. The use of hybrid nanofluids for a variety of applications in the field of engineering can be seen in [8, 9].
The role of heat transfer (HT) in the shape of nanofluids for blood flow is primarily to enhance the thermal properties of the fluid and improve the HT efficiency. In the context of blood flow, nanofluids are used in medical applications, such as thermal management during surgeries, hyperthermia treatment, and drug delivery. The improved heat transfer properties of nanofluids make them effective for dissipating heat generated during surgical procedures or targeted heat delivery for hyperthermia treatment. This helps regulate and maintain the desired body temperature during surgeries or deliver controlled localized heating for therapeutic purposes [10,11,12,13,14].
The heat transfer optimization and biological applications consisting of titanium dioxide and other materials can be seen in [15]. Where the blood-based hybrid nanofluids using mathematical modeling can be seen in [16, 17]. In the field of biology, TiO2–Ag/Blood hybrid nanofluids that flow in arteries have great potential for drug delivery applications.
The unique properties and mechanisms of action of titanium oxide and silver nanoparticles make them promising candidates for cancer therapy. TiO2 NPs are suitable candidates for cancer treatment due to their biocompatibility, high photoactivity, and low toxicity. These nanoparticles can produce reactive oxygen species (ROS) by absorbing and utilizing light energy through photocatalysis. The therapeutic efficacy and side effects can be enhanced by improving the ability of TiO2 NPs to target cancer cells by modifying their surface [18, 19]. The attractiveness of silver nanoparticles (AgNPs) in cancer therapy is due to their unique properties. The high surface-to-volume ratio of AgNPs makes it possible to efficiently deliver therapeutic agents to cancer cells. Ag NPs’ cytotoxic effects can be boosted by TiO2 NPs’ ROS production, which can result in additional cancer cell death [20, 21]. By activating Ag NPs through the photocatalytic properties of TiO2 NPs, the therapeutic potential can be enhanced. These nanoparticles are highly promising due to their potential to develop novel and effective cancer therapies. The flow between concentric tubes for a variety of applications can be seen in [22,23,24,25].
1.1 The newness of the proposed model is demonstrated as
The inner cylinder is commonly used to represent the drug carrier during drug delivery, which can be either a catheter or a needle. In this study, the inner cylinder is used as a drug carrier. Where the blood base liquid dispersed with titanium dioxide (TiO2) and silver (Ag) nanoparticles is used for the application of drug delivery. The literature survey mentioned above did not find any articles that discussed this model and the results of conducting blood in the presence of Ag and TiO2 nanoparticles. In addition, magnetic field, thermal radiation, and heat convection involve other physical parameters as well. Furthermore, the obtained mathematical model is numerically solved via the CVFEM [26,27,28] technique and RK-4 scheme [29, 30]. A neural networking strategy is also used to validate the obtained results.
2 Materials and methods
The principles of fluid dynamics are used to describe the flow of blood-based hybrid nanofluids (HNFs) between concentric cylinder gaps for drug delivery, known as annular flow. When delivering drugs, the inner cylinder is usually what represents the drug carrier, such as a catheter or needle having radius \(r0 = a > 0\). The inner cylinder is responsible for delivering the drug to the blood vessel or tissue. The cylinders’ gap enables the movement of fluid, in particular blood, and the delivery of the drug. The outer cylinder radius is taken \(b > a > 0\). Blood is saturated with \({\text{TiO}}_{{2}} \& {\text{ Ag}}\) nanocomposites. The geometry includes an inertial frame that involves a cylindrical coordinates system \(\left( {r,\theta ,x} \right)\). x-axis is considered along axial reference while the radial line is denoted by the r-axis. The velocity compnents are considered \(vr\) constant and \(v\theta = v\theta (r)\). The temperature of the inner cylinder is \(Ta\) and outer cylinder is \(Tb\) such that \(Ta > Tb\). The perpendicular magnetic field \(B0\), is applied to the flow field.
The governing equations are demonstrated in the context of [4, 5].
Physical conditions shall be adapted as follows:
In the above equations the subscripts \(hnf\) and \(f\) are used to represent the hybrid nanofluids (HNFs) and base fluid blood. Where, \(v_{\theta }\) is the velocity component, \(\beta ,\mu ,k\) are the thermal expansion, absolute viscosity, and thermal conductivity. The idea of Rossland’s approximation is used to define the radiative flux \(qr\) as.
where σ∗ represents the Stefan–Boltzman constant and κ∗ symbolizes the mean absorption coefficient. The thermophysical properties of prime interest are mentioned in Table 1, while their relations for mono and bi-hybrid nanofluid are given below [21].
3 Transformations
The similarity variable is proposed as [4, 5].
Equation (1) is satisfied that fulfilled the basic requirement of the flow field while the rest of the Eqs. (2)−(4) are altered as
The physical parmeters are displayed as
Relations for skin friction and Nusselt number are as follows [4].
With the help of Eqs. (13) and (14) revises so:
4 Solution methodologies
The RK-4 method, also known as the fourth-order Runge–Kutta method, is a numerical technique used for solving ordinary differential equations. It is commonly utilized for solving fluid flow models, which describe the behavior of fluids in various systems [29, 30]. The RK-4 method involves breaking down the fluid flow model into a series of discrete time steps. At each time step, the values of the dependent variables (such as velocity and pressure) are updated based on the current state and the rates of change determined by the differential equations. The steps involved in the RK-4 method are as follows:
-
i.
Start with an initial condition for the dependent variables at the initial time.
-
ii.
Calculate the rates of change at the current time using the partial differential equations.
-
iii.
Use these rates of change to estimate the values of the dependent variables at the midpoint of the time step.
-
iv.
Recalculate the rates of change at the midpoint using the estimated values.
-
v.
Use these new rates of change to estimate the values of the dependent variables at the end of the time step.
-
vi.
Calculate the average of the rates of change at the start, midpoint, and end of the time step.
-
vii.
Multiply this average by the time step and add it to the initial values to obtain the updated values for the next time step.
-
viii.
Repeat steps 2–7 for all subsequent time steps until the desired time is reached.
4.1 Control volume finite element method (CVFEM)
The finite element method (FEM) is a numerical technique used to solve partial differential equations (PDEs), including fluid flow problems. When applying FEM [26,27,28] to the control volume method, the following steps are used:
-
i.
The control volume domain is divided into smaller subdomains or elements, forming a mesh. The size and shape of these elements can vary, but they are usually simple geometrical shapes such as triangles or quadrilaterals in 2D, or tetrahedra or hexahedra in 3D.
-
ii.
The PDEs governing fluid flow, such as the Navier–Stokes equations, are transformed into variational or weak form. This is done by multiplying the equations by test functions and integrating them over each element.
-
iii.
The displacement field, pressure field, or any other unknown variables are approximated using interpolation functions called basis functions. These functions are defined over each element and are usually chosen to be polynomials.
-
iv.
Substituting the basis functions into the weak form equations, and integrating over each element, results in a set of element equations. These equations are typically expressed as systems of algebraic equations in terms of the unknown nodal values.
-
v.
Applying the appropriate boundary conditions is necessary to solve the fluid flow problem. This involves specifying the values or behavior of the unknown variables at certain points or boundaries of the control volume domain.
4.2 An artificial neural network (ANN)
An artificial neural network (ANN) [31,32,33,34,35,36,37,38,39] is used to model and predict the behavior of hybrid nanofluid flow in concentric cylinders.
To use an ANN for modeling the hybrid nanofluid flow, the following steps.
-
i.
The data should include input variables and corresponding output variables in the form of parameters.
-
ii.
Design the architecture of the ANN, which includes the number of input and output nodes, as well as the number and type of hidden layers.
-
iii.
Use the collected data to train the ANN. The training process involves adjusting the weights and biases of the network to minimize the difference between the predicted output and the actual output. Various training algorithms such as backpropagation are used.
-
iv.
Validating the trained ANN using a separate set of data that is not used during the training phase. This ensures that the ANN’s performance is not biased towards the training data.
-
v.
Evaluate the performance of the trained ANN by using it to predict the behavior of hybrid nanofluid flow for unseen data.
5 Results and discussion
The flow field in the form of geometry is presented in Fig. 1. The geometry of the problem is displayed in Fig. 1a, while the grids of the proposed model are displayed in Fig. 1b using the CVFEM technique. The current study examines how different nanofluids, mono (Ag + Blood) and bi-hybrid (Ag + TiO2 + Blood), are compared. The flow chart is displayed in Fig. 2. The solution to the problem was found by utilizing the methods of Range Kutta 4 (RK-4), and Control Volume Finite Element (CVFEM). The obtained solution is verified through error analysis by using the artificial neural network (ANN) strategy.
5.1 Analyzing velocity and temperature profiles
In this section the obtained resuts are discussed in the form of Figures and Tables. These out puts consist of the physical parameters versus the HNF motion and thermal profiles. Figure 3a–f illustrate how the parameters Gr, M and ϕ = ϕ1 + ϕ2 influence f(η) field TiO2 + Blood and Ag + TiO2 + blood. The Grashof number is a dimensionless number that characterizes the importance of buoyancy forces in a fluid flow. An increasing Grashof number indicates increasing buoyancy forces.
In the case of Ag + TiO2 hybrid nanofluids flow for drug delivery, an increasing Grashof number impact is shown in Fig. 3a. With increasing buoyancy forces, the convective heat transfer in the nanofluid flow can be significantly enhanced. This can result in more efficient heating or cooling of the drug in the fluid, which is beneficial for drug delivery applications that require specific temperature conditions.
Figure 3b stands for the AE representation in the case of the Gr choosing Ag + TiO2 + Blood.
The magnetic factor M has paramount significance upon the motion of both TiO2 + Blood and Ag + TiO2 + Blood that is been successfully plotted in (3c). Velocity \(f(\eta )\) declines as M values rise. The increasing magnetic parameter has a significant impact on the motion of both TiO2 + Blood and Ag + TiO2 + Blood for drug delivery applications.
In drug delivery systems, the use of magnetic nanoparticles has gained great attention due to their unique properties, such as biocompatibility, easy surface modification, and controllable movement under magnetic fields. The addition of magnetic nanoparticles to drug carriers, such as TiO2 nanoparticles and Ag nanoparticles, enhances their targeting efficiency and improves drug release control. When exposed to an external magnetic field, magnetic nanoparticles can be guided and manipulated to specific target sites, allowing for targeted drug delivery. The increased magnetic parameter significance results in a stronger magnetic response of the nanoparticles, enabling better control over their movement and localization within the body.
Figure 3d stands for the AE representation in case of the (M) choosing Ag + TiO2 + Blood.
The significance of \(\phi\) (volume fraction) to \(f(\eta )\) for the TiO2 + Blood and Ag + TiO2 + Blood nanofluids is shown in Fig. 3e. When the volume fraction \(\phi\) of TiO2, and Ag + TiO2 nanoparticles in blood-based nanofluids increases, it generally leads to a decrease in the velocity of the nanofluids. This is because the nanoparticles introduce additional viscosity and resistance to the flow of the fluid. As the volume fraction of nanoparticles increases, the fluid becomes more concentrated with nanoparticles, resulting in a higher viscosity. This increased viscosity hinders the flow of the fluid, causing a decrease in velocity. Figure 3f stands for the AE representation in case of the \(\phi\) choosing Ag + TiO2 + Blood.
Figure 4a–f frame the sundry outcomes in dimensional temperature opposite to varying values of model parameters like Rd, Ec, and ϕ, sequentially, with both TiO2 + Blood and Ag + TiO2 + Blood nanofluids. Figure 4a shows that increasing the radiation parameter improves the thermal profile and this impact is more effective for Ag + TiO2 + Blood nanofluid.
The radiation parameter determines the rate at which heat is transferred between the nanofluid and its surroundings. Increasing this parameter enhances the efficiency of heat generation, leading to faster and more effective drug delivery. This is particularly important for localized hyperthermia treatment, where controlled heating is used to release drugs from nanocarriers.
Figure 4b stands for the AE representation in the case of the (Rd) choosing Ag + TiO2 + Blood.
The Eckert number is a dimensionless quantity that relates the rate of kinetic energy transfer to the rate of heat transfer in a fluid flow. As the Eckert number increases, it signifies a greater dominance of kinetic energy transfer compared to heat transfer. In the context of TiO2 + Blood and Ag + TiO2 + Blood hybrid nanofluids, an increasing Eckert number implies an increased level of kinetic energy transfer, which has effects on the temperature profile \(\theta (\eta )\) as shown in Fig. 4c. The enhanced flow dynamics are associated with higher Eckert numbers that promote convective heat transfer. Figure 4d stands for the AE representation in the case of the (Ec) choosing Ag + TiO2 + Blood. The volume fraction \(\phi\) of nanoparticles in a nanofluid has a significant influence on the temperature field as estimated in Fig. 4e. In the case of Ag + TiO2 blood hybrid nanofluids, an increasing \(\phi\) of nanoparticles, such as silver (Ag) and titanium dioxide (TiO2), affects the thermal behavior of the nanofluid. When the volume fraction of nanoparticles increases, the thermal conductivity of the nanofluid also increases.
This is because nanoparticles have a higher thermal conductivity compared to the base fluid, in this case, blood. As a result, the heat transfer capability of the nanofluid is enhanced, leading to better heat dissipation and lower temperatures. Furthermore, an increase in the volume fraction of nanoparticles also leads to an increase in the heat capacity of the nanofluid. Figure 4f stands for the AE representation in the case of the \(\phi\) choosing Ag + TiO2 + Blood. The inspirations about the velocity of HNFs under the variation of Gr are outlined in Fig. 5a. The position in a fluid domain determines how a physical property changes, as depicted by contour plots. These properties make it possible to visualize and quantify the spatial distribution of these properties. Analyzing the distribution of nanoparticle concentration, temperature gradients, pressure variations, or any other relevant parameter can be done by using contours in the flow of hybrid nanofluids. Likewise, it is clear from Fig. 5b that the flux response is more important in the positive region for the rising values of Gr. On the other hand, the velocity etiquette responses to the upper and lower cylinder surfaces are successfully illustrated in Fig. 5c, d, accordingly concerning different views.
5.2 Analyzing Cf and Nux
The flow properties of a fluid can be altered and skin friction can be decreased by adding nanoparticles, such as silver and titanium oxide. The skin-friction coefficient Cf is schemed versus the increasing values of M and Gr in the Figure of 6. The parameters M = 0,1,2,3 and Gr = 1,2,3,4 are varied versus the skin friction. In this figure the results of the Mano and hybrid nanofluids are compared. The innermost surface wall’s flow rates are shown in Fig. 6a, b in a constructive manner with increasing Gr and decreasing M. One can see the increasing behavior of the skin friction using HNFs. The thermophysical properties efficiency in percentage wise displayed in Fig. 7a–c. Table 1 demonstrate the thermophysical properties of the nanoparticles and base fluid. Table 2 summarizes the significant efforts of non-dimensional Nusselt number Nu vs variations in vigorous related parameters. Blood flow for drug delivery applications is significantly impacted by the presence of HNF due to the heat transfer rate (HT). These nanoparticles improve heat transfer rates by increasing the thermal conductivity of the fluid. HT rate of the blood flow is affected by viscous dissipation (Ec), which is energy that is dissipated in a fluid by internal friction. Using HNF (Ag + TiO2) increases the precision of the Eckert number enlargement’s impact on the HT rate. Heat transfer and blood flow are affected by thermal radiation in drug delivery applications. The temperature distribution and blood flow dynamics is modified by the presence of (\(Rd\)), which may impact the drug delivery process. The heat transfer rate is improved when the radiation parameter (\(Rd\)) is increased. The force exerted on the fluid particles by the magnetic field affects the movement and flow behavior of blood flow. The drug is carried by blood that changes its HT rate, velocity, and flow patterns due to the magnetic field (\(M\)). The variation in nanoparticle volume fraction \(\phi\) has an impact on the HT rate and blood flow. Changes in \(\phi\) have an impact on the fluid’s thermal conductivity and heat transfer efficiency. After incorporating \(\phi\) of both mono/hybrid nanoparticles and installing M and Rd, Blood exhibits significant improvements in HT rates.
Hybrid nanofluids (HNFs) often exhibit superior heat transfer (HT) properties, primarily due to the high thermal conductivity of nanoparticles. Improving heat transfer helps reduce skin friction by maintaining a more uniform temperature distribution near the surface. Also, this acts in the optimization of the blood flow analysis. The comparison of the present work and existing literature is shown in Table 3. The closed agreement has been observed which shows the authentication of the present work. Furthermore, interesting results obtained from this table that when reducing the gap between the two cylinders the heat transfer rate increases.
6 Conclusions
In the current study the focus has been given to study the Ag and TiO2 hybrid nanifluids for the applications of drug delvery using blood as a base solvent. The flow field is the gap of two tubes. Heat transfer in terms of drug delivery is achieved by the hybrid nanofluids flowing between the concentric cylinders.CVFEM techniques are employed to solve the PDEs that govern the equations. The RK-4 technique is employed to solve the transform equations. The ANN solution is obtained through the use of this solution scheme. The outcomes of the physical parameters are displayed here. The stable dispersion of the Ag + TiO2 nanopartciles in the blood shows improment in the convective motion caused by hybrid nanofluids. The used of the magnetic field (\(M\)) improving the targeting efficiency of nanofluids by controlling the fluid motion and improving the thermal profile. The increasing Eckert number \(Ec\) enhancing the thermal profile and consequently improving the drug delivery. The neural networking strategy is used to validate the obtained results through error analysis by adopting the square residual error. With increasing \(\phi\), the skin friction at the boundaries increases, though the pattern reverses for escalating Gr. It is noteworthy that the hybrid nanofluid exhibits useful and greater increases concerning the heat transfer phenomena when a comparison is made with mono nanofluid.
As a future work, the recent analysis is extendable using the porous medium for the fluid flow, using other nanoparticles, other non-Newtonian fluids, and other solution techniques.
Availability of data and materials
Data sets generated during the current study are available from the corresponding author upon reasonable request.
Abbreviations
- \(\left( {v_{r} \& v_{\theta } } \right)\) :
-
Velocity components (ms−1)
- \(Nu -\) :
-
Nusselt number
- \(g\) :
-
Acceleration (ms−2)
- \(qr\) :
-
Radiative flux
- \(a\) :
-
Inner cylinder radius (m)
- \(Cp\) :
-
Heat capacitance (J/kg K)
- \(\Pr\) :
-
Prandtl number
- \(f\) :
-
Dimensionless velocity component
- \(b\) :
-
Outer cylinder radius (m)
- \(T_{w} ,T_{\infty }\) :
-
Components of Temperature (K)
- \(M\) :
-
Magnetic field parameter
- \(Rd\) :
-
Radiation parameter
- \(Gr\) :
-
Grashof number
- \({\text{Re}}\) :
-
Reynolds number
- \(C_{f}\) :
-
Skin friction coefficient
- \(\theta ,\phi\) :
-
Dimensionless temperature and concentration
- \(\rho_{f}\) :
-
Fluid density (kg m−3)
- \(k\) :
-
Thermal conductivity (W/mK)
- \(\mu\) :
-
Viscosity of fluid (mPa)
- \(\eta\) :
-
Dimensionless transform variable
- \(\sigma\) :
-
Electrical conductivity
- \(B_{0}\) :
-
Magnetic field
References
Xu J, Zhang S, Machado A, Lecommandoux S, Sandre O, Gu F, Colin A. Controllable microfluidic production of drug-loaded PLGA nanoparticles using partially water-miscible mixed solvent microdroplets as a precursor. Sci Rep. 2017;7(1):4794. https://doi.org/10.1038/s41598-017-05184-5.
Laidoudi H, Helmaoui M, Bouzit M, Ghenaim A. Natural-convection of Newtonian fluids between two concentric cylinders of a special cross-sectional form. Therm Sci. 2021;25(5):3701–14.
Al-Kouz W, Alshare A, Alkhalidi A, Kiwan S. Two dimensional analysis of low pressure flows in the annulus region between two concentric cylinders. Springerplus. 2016;5:1–22.
Gouran S, Mohsenian S, Ghasemi SE. Theoretical analysis on MHD nanofluid flow between two concentric cylinders using efficient computational techniques. Alex Eng J. 2022;61(4):3237–48.
Mohsenian S, Gouran S, Ghasemi SE. Evaluation of weighted residual methods for thermal radiation on nanofluid flow between two tubes in presence of magnetic field. Case Stud Therm Eng. 2022;32:101867.
Etminan A, Dahaghin A, Emadiyanrazavi S, Salimibani M, Eivazzadeh-Keihan R, Haghpanahi M, Maleki A. Simulation of heat transfer, mass transfer and tissue damage in magnetic nanoparticle hyperthermia with blood vessels. J Therm Biol. 2022;110:103371.
Barnoon P, Bakhshandehfard F. Thermal management in a biological tissue in order to destroy tissue under local heating process. Case Stud Therm Eng. 2021;26:101105.
Shahsavar A, Noori S, Toghraie D, Barnoon P. Free convection of non-Newtonian nanofluid flow inside an eccentric annulus from the point of view of first-law and second-law of thermodynamics. ZAMM-J Appl Math Mech/Z Angew Math Mech. 2021;101(5):e202000266.
Geridonmez BP, Oztop HF. MHD natural convection in a cavity in the presence of cross partial magnetic fields and Al2O3-water nanofluid. Comput Math Appl. 2020;80(12):2796–810.
Barnoon P. Electroosmotic flow and heat transfer of a hybrid nanofluid in a microchannel: a structural optimization. Int J Thermofluids. 2023;20:100499.
Sheremet MA, Oztop HF, Pop I. MHD natural convection in an inclined wavy cavity with corner heater filled with a nanofluid. J Magn Magn Mater. 2016;416:37–47.
Sheremet MA, Oztop HF, Pop I, Al-Salem K. MHD free convection in a wavy open porous tall cavity filled with nanofluids under an effect of corner heater. Int J Heat Mass Transf. 2016;103:955–64.
Alnahdi AS, Gul T. MHD hybrid nanofluid flow over a stretched surface with convective boundary conditions: applications of heat transfer. Mod Phys Lett B. 2024;38(08):2450046.
Zari I, Ali F, Gul T, Madubueze CE, Ali I (2023) Squeezing flow in the existence of carbon nanotubes past a Riga plate. Int J Modern Phys B 2450370.
Hariharan D, Thangamuniyandi P, Christy AJ, Vasantharaja R, Selvakumar P, Sagadevan S, Nehru LC. Enhanced photocatalysis and anticancer activity of green hydrothermal synthesized Ag@ TiO2 nanoparticles. J Photochem Photobiol B. 2022;202:111636.
Zarzzeka C, Goldoni J, Marafon F, Sganzerla WG, Forster-Carneiro T, Bagatini MD, Colpini LMS. Use of titanium dioxide nanoparticles for cancer treatment: a comprehensive review and bibliometric analysis. Biocatal Agric Biotechnol. 2023;50:102710. https://doi.org/10.1016/j.bcab.2023.102710.
Lagopati N, Kotsinas A, Veroutis D, Evangelou K, Papaspyropoulos A, Arfanis M, Gorgoulis VG. Biological effect of silver-modified nanostructured titanium dioxide in cancer. Cancer Genom Proteom. 2021;18:425–39.
Alnahdi, A. S., Khan, A., Gul, T., & Ahmad, H. Stagnation Point Nanofluid Flow in a Variable Darcy Space Subject to Thermal Convection Using Artificial Neural Network Technique. Arabian Journal for Science and Engineering, 2024;1–18.
Ahmed A, Nadeem S. The study of (Cu, TiO2, Al2O3) nanoparticles as antimicrobials of Blood flow through diseased arteries. J Mol Liq. 2016;216:615–23.
Basit MA, Farooq U, Imran M, Fatima N, Alhushaybari A, Noreen S, Akgül A. Comprehensive investigations of (Au–Ag/Blood and Cu–Fe3O4/Blood) hybrid nanofluid over two rotating disks: numerical and computational approach. Alex Eng J. 2023;72:19–36.
Shojaie Chahregh H, Dinarvand S. TiO2-Ag/Blood hybrid nanofluid flow through an artery with applications of drug delivery and Blood circulation in the respiratory system. Int J Numer Meth Heat Fluid Flow. 2020;30(11):4775–96.
Dinarvand S, Berrehal H, Pop I, Chamkha AJ. Blood-based hybrid nanofluid flow through converging/diverging channel with multiple slips effect: a development of Jeffery-Hamel problem. Int J Numer Meth Heat Fluid Flow. 2023;33(3):1144–60.
Gul T, Ali B, Alghamdi W, Nasir S, Saeed A, Kumam P, Jawad M. Mixed convection stagnation point flow of the Blood based hybrid nanofluid around a rotating sphere. Sci Rep. 2021;11:1–15.
Sheikhzadeh GA, Teimouri H, Mahmoodi M. Numerical study of mixed convection of nanofluid in a concentric annulus with rotating inner cylinder. Challeng Nano Micro Scale Sci Technol. 2013;1:26–36.
Shahsavar A, Moradi M, Bahiraei M. Heat transfer and entropy generation optimization for flow of a non-Newtonian hybrid nanofluid containing coated CNT/Fe3O4 nanoparticles in a concentric annulus. J Taiwan Inst Chem Eng. 2018;84:28–40.
Mukhtar S, Gul T. Solar radiation and thermal convection of hybrid nanofluids for the optimization of solar collector. Mathematics. 2023;11(5):1175.
Gul T, Alharbi SO, Khan I, Khan MS, Alzahrani S. Comparative analysis of the flow of the hybrid nanofluid stagnation point on the slippery surface by the CVFEM approach. Alex Eng J. 2023;76:629–39.
Gul T, Nasir S, Berrouk AS, Raizah Z, Alghamdi W, Ali I, Bariq A. Simulation of the water-based hybrid nanofluids flow through a porous cavity for the applications of the heat transfer. Sci Rep. 2023;13(1):7009.
Bakir Y, Mert O. On solution of ordinary differential equations by using HWCM, ADM and RK4. Int J Modern Phys C 2022; 2250135.
Rasheed HU, Khan W, Khan I, Alshammari N, Hamadneh N. Numerical computation of 3D Brownian motion of thin film nanofluid flow of convective heat transfer over a stretchable rotating surface. Sci Rep. 2022;12:1–14.
Awwad FA, Ismail EA, Gul T. Heat and mass transfer gravity driven fluid flow over a symmetrically-vertical plane through neural networks. Symmetry. 2023;15(6):1288.
Shoaib M, Kainat R, Ijaz KM, Prasanna Kumara BC, Naveen Kumar R., Zahoor Raja MA. Darcy-Forchheimer entropy-based hybrid nanofluid flow over a stretchable surface: intelligent computing approach. Waves in Random and Complex Media. 2022; 1–24.
Raja MAZ, Shoaib M, Tabassum R, Khan NM, Kehili S, Bafakeeh OT. Stochastic numerical computing for entropy optimized of Darcy-Forchheimer nanofluid flow: Levenberg Marquardt Algorithm. Chem Phys Lett. 2022;807:140070.
Shoaib M, Tabassum R, Nisar KS, Raja MAZ, Fatima N, Al-Harbi N, Abdel-Aty AH. A design of neuro-computational approach for double-diffusive natural convection nanofluid flow. Heliyon. 2022;9(3):1–15.
Shoaib M, Zubair G, Nisar KS, Raja MAZ, Khan MI, Gowda RP, Prasannakumara BC. Ohmic heating effects and entropy generation for nanofluidic system of Ree-Eyring fluid: intelligent computing paradigm. Int Commun Heat Mass Transfer. 2021;129:105683.
Srilatha P, Gowda RJP, Madhu J, et al. Designing a solid–fluid interface layer and artificial neural network in a nanofluid flow due to rotating rough and porous disk. J Therm Anal Calorim. 2023. https://doi.org/10.1007/s10973-023-12706-z.
Prakash SB, Chandan K, Karthik K, Devanathan S, Kumar RV, Nagaraja KV, Prasannakumara BC. Investigation of the thermal analysis of a wavy fin with radiation impact: an application of extreme learning machine. Phys Scripta. 2023;99(1):015225.
Kumar C, Nimmy P, Nagaraja KV, Kumar RV, Verma A, Alkarni S, Shah NA. Analysis of heat transfer behavior of porous wavy fin with radiation and convection by using a machine learning technique. Symmetry. 2023;15(8):1601.
Poornima BS, Sarris IE, Chandan K, Nagaraja KV, Kumar RV, Ben AS. Evolutionary computing for the radiative-convective heat transfer of a wetted wavy fin using a genetic algorithm-based neural network. Biomimetics. 2023;8(8):574.
Funding
No external funding was used.
Author information
Authors and Affiliations
Contributions
Project PI = SOA; Methodology = HAEl-WK; TG, Software = TG; RA; AA, Manuscript writing = TG, AB, Validation = SOA;.RA; TG. AB.
Corresponding author
Ethics declarations
Competing interests
The authors have no competing interests to declare that are relevant to the content of this article.
Additional information
Publisher's Note
Springer Nature remains neutral with regard to jurisdictional claims in published maps and institutional affiliations.
Rights and permissions
Open Access This article is licensed under a Creative Commons Attribution 4.0 International License, which permits use, sharing, adaptation, distribution and reproduction in any medium or format, as long as you give appropriate credit to the original author(s) and the source, provide a link to the Creative Commons licence, and indicate if changes were made. The images or other third party material in this article are included in the article's Creative Commons licence, unless indicated otherwise in a credit line to the material. If material is not included in the article's Creative Commons licence and your intended use is not permitted by statutory regulation or exceeds the permitted use, you will need to obtain permission directly from the copyright holder. To view a copy of this licence, visit http://creativecommons.org/licenses/by/4.0/.
About this article
Cite this article
Alharbi, S.O., Khalifa, H.A.EW., Gul, T. et al. The use of neural computational analysis for drug delivery applications results in hybrid nanofluid flow between the uniform gap of two concentric tubes. Discov Appl Sci 6, 200 (2024). https://doi.org/10.1007/s42452-024-05742-3
Received:
Accepted:
Published:
DOI: https://doi.org/10.1007/s42452-024-05742-3