Abstract
The shortage of water resources in Yemen has an implication on the availability and supply of safe water in the country. This study assessed the water quality in Wadi Al-Dabab, Taiz, Yemen. Water samples were collected from the springs and different types of wells (tube, manual) with depths ranging from 9 to 500 m. Multivariate statistical analysis was performed on 15 water quality parameters (WQP) from 15 locations to investigate the significant WQP and the possibility of data reduction. The water quality index (WQI) approach was used to assess its suitability for drinking purposes. Four principal components were identified to be significant, which explained 86.2% of the overall variance while four varifactors (VF) explained 80% of the data variance. The findings showed the possibility of data reduction by 20%, which could assist in water quality monitoring at a reduced cost. The WQI map shows that the water quality is good in a limited area and poor to very poor in most of the study area. The findings are likely to assist in identifying the important WQP for the protection of the drinking water sources while the less important WQP can be excluded, which might reduce the cost of water quality monitoring. The proposed approach is likely to be a cost-effective approach for the economically weak and water-stressed countries, which can contribute positively for the sustainable water resource management in Yemen and other water-stressed regions.
Similar content being viewed by others
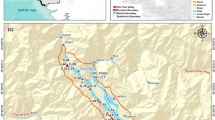
Avoid common mistakes on your manuscript.
Introduction
Over the past few decades, Yemen has experienced an increase in freshwater demand due to rapid population growth, leading to a widened disparity between the available water resources and demands (Hellegers et al. 2008). The groundwater resources in Yemen, particularly in the Sana’a basin, have shown declining trends in water levels and increase in salinity due to the reductions in water reserves caused by urbanization, industrialization, and irrigation activities (Taher 2016). Protecting and monitoring these resources are crucial to ensure safe water supplies (Hellegers et al. 2008). The anthropogenic activities in the arid countries including the industrial and municipal wastewater discharges, and surface runoff can cause constant or seasonal contamination of water sources, leading to a significant problem in many countries (Qadir., et al. 2008,; Singh et al. 2004; Alberto et al. 2001). Protecting water quality is essential to minimize water treatment costs and to keep water sources usable (WHO 2017; Canada 2017; EPA, 2018). However, the complex nature and uncertainty of water quality parameters (WQP) can pose significant challenges in controlling water quality (Elhatip et al. 2008). Regular monitoring and assessment of WQP are necessary to address these limitations (Prathumratana et al. 2008).
Multivariate statistical analysis is commonly used in water quality interpretation because of its capability to explain and interpret complex water quality data matrices, and identify possible factors that impact water quality, offering reasonable solutions to pollution issues and water resource management (Reghunath et al. 2002; Lee et al., 2001). In addition, multivariate statistical approaches have successfully assessed the spatial variation of water quality (Chowdhury and Husain 2020; Phung et al., 2015; Marinović and Ruždjak, 2015; Muangthong and Shrestha 2015; Olsen et al. 2012; Zhang et al. 2009a, b; Zeilhoferet al. 2006; Simeonov et al. 2003) and can be applied to characterize, evaluate, and validate tempo-spatial variability of freshwater quality (Chowdhury & Al-Zahrani 2014; Singh et al. 2005). The hydrochemical and statistical analysis have been used in various studies to evaluate groundwater resources and their hydrogeochemical characteristics in the Arabian Peninsula including Saudi Arabia (Nazzal et al. 2015; Al-Omran et al. 2018), United Arab Emirates (Mohamed and Hassane 2016) and Yemen (Nasher and El-Sagheer, 2012; Nasher et al. 2013; Saleh et al. 2018).
The Geographical Information System (GIS) plays an essential role in environmental data management, enabling the presentation of multiple scenarios to managers and scientists to forecast spatial distribution and data trends, thereby avoiding impending environmental crises (Igboekwe and Akankpo 2011; Zeilhofer et al. 2007). In addition, GIS has essential applications in water resource management and pollution control, as demonstrated in various studies, including those conducted in Al-Howban Basin, Taiz-Yemen (Aqeel et al. 2017), El Khairat deep aquifer, Tunisia (Gebrehiwot et al. 2011), and Al-Ula Area, Saudi Arabia (Toumi et al. 2015).
Limited studies have been conducted in Taiz, Yemen where the current study area is located. Metwali (2003) evaluated Taiz city's physicochemical and bacteriological drinking water quality where the author reported that most of the water samples, especially from private wells, contained a high concentration of total coliforms. Al-Amry (2009) reported that groundwater in the Hidehran and Alburayhi basin, northwest of Taiz city, has excessive fluoride ion concentrations, which poses a high risk to humans due to its ingestion, and the majority of groundwater samples are oversaturated with respect to calcite and undersaturated with respect to fluoride. Ahmed Al-Shargabi (2015) concluded that the majority of Taiz city's groundwater is unsuited for drinking due to the high concentrations of various pollutants. Naser et al. (2020) assessed the intensity and spatial extent of fluoride in groundwater in the southern part of upper Wadi Rasyan, Taiz. The results showed that 71% samples exceeded the WHO guideline of 1.5 mg/l, with wide variation in fluoride content within the same aquifer and water type. Alansi et al. (2021) reported heavy metal contamination in groundwater around Al-Buraihi sewage station in Taiz, due to treated sewage, which is unsuitable for irrigation and can have adverse effects on plants, animals, and human life.
Despite the effectiveness of multivariate statistical analysis in interpreting the water quality data, no previous study applied this technique for water quality management in the cities of Yemen. To determine the loadings on the major principal components (PCs) and varifactors (VFs), and the pollution sources, principal component analysis (PCA) and factor analysis (FA) were performed to analyze 15 WQP (Potassium (K), alkalinity (T.ALK), sulfate (SO4), calcium (Ca), magnesium (Mg), hardness (T.H), total dissolved solids (TDS), sodium (Na), iron (Fe), and pH). The GIS was used to generate a WQI map for Wadi Al-Dabab in Taiz, Yemen. Finally, the possibility of reducing the dimension of WQP was discussed.
Methods
Study area
The Wadi Al-Dabab study area is about 9 km southwest of Taiz city. Taiz city is about 100 km to the east of the Red Sea and 253 km to the south of Sana city, the largest capital city of Yemen (Fig. 1). Wadi Al-Dabab lies between north latitudes (1,502,000–1444000) and east longitudes (389,000–384000) and covers 117 square kilometers. The springwater, tube wells, and manual wells are the major water sources for the populations living in the Wadi Al-Dabab region. The aquifer of Wadi Al-Dabab consists of two porous layers resulting in the increased probability of water contamination by natural/anthropogenic activities. There are non-point pollutant sources that randomly disposed pollutants in the study area. Wastewater, industrial waste, municipal solid waste, oils, and structural waste are the primary sources of non-point pollutants. These sources affect the water quality in the basin, resulting in the elevated risks to approximately 24,140 people as they frequently use these sources.
The rocks in the study area are characterized as the rock units formed in the Cenozoic Volcanic Group. These were formed by volcanic activities that affected Yemen clouds during the Tertiary age as a result of the tectonic movements that formed the Red Sea and the Gulf of Aden. The rock units can be divided into three groups:
-
The basal volcanic rocks appear in the form of hills and plateaus on both sides, and in the middle of the fog valley, which are hard or fracture volcanic rocks made of basalt, scoria, rhyolite, andisite, and diabase
-
The granitic tectonic intrusions appear in the eastern part of the study area
-
3–The alluvial sediments of the Quaternary age, consisting of boulders, gravel, sand, silt and clay, which were formed in the fan environments covering most of the streams of the river in the main valley and mountain heights.
As for the geological structures, the most important rifts appear through a major rift extending from east to west and a group of local rifts extending northwest-southeast perpendicular to the main rift (Fig. 2).
Sampling and sample preparation
The National Water Resource Authority (NWRA) in Sana, Yemen, reviewed previous technical studies on water quality for Wadi Al-Dhabab and Taiz city. These studies were conducted as the parts of the periodic monitoring program for water quality in the Taiz governorate. Based on these studies, 15 sampling points were identified in Wadi Al-Dabab for water quality sampling (Fig. 3). The NWRA adopted criteria for selecting water facilities to perform sampling based on hydrogeological and socio-economic variables including comprehensive representation of water layers and reservoirs, different water sources and their qualitative behavior, sampling from polluted and residential areas, and consideration of different applications of water sources.
The NWRA took several measures to ensure the accuracy and quality of the field samples including visiting the sites, assigning two teams to take five samples per day over three days from the water fields, adhering to technical conditions and standards when taking field samples, and properly sterilizing and preserving the samples for laboratory analysis. Field electrical conductivity, pH, temperature, and turbidity measurements were also taken. The team followed standards for water sampling, including selecting standard plastic bottles, sterilizing tools and containers, and taking samples with experienced laboratory specialists. All laboratory instruments and parameters used in water quality analysis followed the recommended standards. The equipment used include Thermometer ( Bench top), Conduct meter ( Bench top), EC x o.65, pH meter (Bench top), EDTA Titrimetric method, Flame photometry, DR2800, and Titration with acid.
Sample analysis
The WQP were analyzed in the laboratory of Taiz's General Authority for Water Resources. The laboratory specialists and field sample collectors collected five samples daily and conducted laboratory analysis immediately after collection. Both chemical and biological tests were performed on the samples, and field measurements were taken for each sample (electrical conductivity, pH, and temperature). Chemical elements, such as calcium, sodium, potassium, chloride, sulfate, nitrate, iron, and fluoride, were analyzed in the laboratory. In contrast, other elements were calculated, including total dissolved salts, total hardness, total alkalinity, magnesium, and bicarbonates.
Calculation and statistics
The study aimed to evaluate the water quality of a specific area using multivariate statistics and water quality index (WQI). The laboratory analysis involved both physical and chemical tests such as electrical conductivity, hydrogen ion concentration, temperature, calcium, sodium, potassium, chloride, sulfate, nitrate, iron, fluoride, total dissolved solids, total hardness, total alkalinity, and bicarbonate. PCA and FA were employed to identify the main factors influencing water quality using the chemical parameters obtained from the laboratory analysis. The WQI was calculated based on the physical and chemical parameters to provide a single value representing the overall quality of water. In summary, the study used a combination of sample collection, laboratory analysis, multivariate statistics, and the WQI to assess the water quality of the study area.
Scatterplots and pairwise correlations
The Scatterplots and pairwise correlations for the WQP in the Wadi Al-Dabab were investigated using the JMP and the MINITAB™ statistical packages. Scatterplots and pairwise correlations indicate potential correlations as well as nonlinear and linear relationships among the WQP.
Principle component analysis (PCA)
Using PCA, the original correlated variables are transformed into new uncorrelated variables known as PCs. The most important parameters identified by PCA can be used to describe the majority of the data set (Helena et al. 2000). PCA can also reduce data dimensionality, resulting in fewer transformed variables that reflect the majority of data variance. (Jackson et al., 1991; Helena et al. 2000). The PC can be represented by the equation below:
where Z = a component score, i = component number, a = component loading, x = a measured value of a variable, j = sample number, and m = number of variables.
The factor analysis (FA), which reduces the contribution of less important variables to create a new set of variables called varifactors (VF), will further simplify the transformed variables obtained from PCA. The VF includes hypothetical, unobservable, and latent water quality variables (Vega et al. 1998; Helena et al. 2000). The significant PCs are extracted using the normalized variables in PCA analysis to exclude the less significant variables’ contribution (Bu et al. 2010; Zhang et al. 2009a, b). As a result, information derived from a considerably larger collection of original correlated variables can now be extracted from a smaller number of uncorrelated variables. The factor analysis (FA) can be represented by the equation below:
where Z = measured variable, f = factor score, a = factor loading, e = residuals, m = the number of factors, and i = sample number. The PCs are considered significant if eigenvalues ≥ 1.0 (Chowdhury and Al-Zahrani 2014; Shrestha and Kazama 2007; Varol et al. 2012). This study subjected fifteen WQP from fifteen sampling sites for Wadi Al-Dabab to FA/ PCA.
Water quality index (WQI)
The water quality index is a score that reflects the composite impact of various WQP on the overall water quality (Sahu and Sikdar 2008). The WQI is used to extract easily usable and understandable statistics from complicated water quality data. The selected WQP (e.g., pH, TH, TDS, HCO3, SO4, Cl, Fe, NO3, etc.) were assigned different weights (wi) based on their relative importance in the drinking purposes and according to their impacts on health to calculate the WQI (Sahu and Sikdar 2008). Equation (3) is used to calculate the relative weight (Wi) for each parameter:
where wi = weight of each parameter, and 'n' = number of parameters. Then Eq. (4) is used to assign a quality rating scale (qi) for each parameter.
where Ci = concentration of each chemical parameter (mg/l), and Si = value/desirable limit (mg/l) according to Yemen’s guidelines. To calculate WQI, Eq. (5) is used to determine the sub-index (SI) for each parameter:
where qi = rating (Ramakrishnaiah et al. 2009). Sahu and Sikdar (2008) classified the WQI into five categories as Excellent water (less than 50); Good water (50–100); Poor water (100–200); Very poor water (200–300); Water is considered unfit for human consumption at the WQI > 300.
Results and discussions
Descriptive statistics
Table 1 shows the summation of the anions and cations for checking the errors or discrepancies in the sample analysis, water sampling, or the data entry that have occurred (Rice et al., 2017). Since all potable waters are electrically neutral, the sum of the cations and anions must balance when expressed as milliequivalents per liter, which can be achieved if the difference is within 1.5–5% (Rice et al. 2017). In this study, the overall difference was calculated to be 3.2% (Table 2). The percentage difference was calculated using the following equation:
The statistical summary of 15 WQP is shown in Table 2. The maximum likelihood methodology was used to estimate the statistical distributions, and the Chi-square and Kolmogorov–Smirnov methods were used to test it. The log-normal distributions were found to be the best fits for the WQP.
Each parameter's minimum and maximum values were calculated. The spatial distributions of fifteen parameters were characterized. The conductivity(EC) values were in the range of 1160–9520 mg/l with higher concentrations in the northern part of the study region (Fig. 4a). Figure 4b shows that alkaline water was in most of the study area with pH in the range of 6.15–8.8, high only in the N1-040 well and low only in the N2-089 well. As seen in Fig. 4c, total hardness (T.H) concentration was high in most of the sampling region and ranged from 250 to 1998 mg/l. The presence of industrial waste caused an increase in total dissolved solids (TDS) concentration, which varied from 754 to 6188 mg/l (Fig. 4d). The Potassium(K) concentration, which ranged from 0.59 to 17.16 mg/l, was low in the whole study area except N1-045, N1-052, and N2-089 wells (Fig. 4e). The sodium(Na) concentration ranged from 39.1 to 1239.7 mg/l, with the highest concentration toward the north (Fig. 4f). The manganese (Mg) concentrations ranged from 24.08 to 348.4 mg/l, with the highest concentration in the northern part (Fig. 4g).
Similarly, the highest concentration of calcium(Ca) in the north part, and its concentration ranged from 60.12 to 405.2 mg/l over the whole study area (Fig. 4h). The bicarbonate concentration (HCO3) was in the range of 57.34–830.82 mg/l and was high over the entire study area except N1-040 and N1-052 wells, which were within the allowable range. In the N2-103 well, HCO3 was under the permissible range (Fig. 4i). The spatial distribution of chloride(Cl) is shown in Fig. 4j, which ranged from 35 to 1617.7 mg/l in the study area, with the concentrations being highest toward the north. Sulfate (SO4) concentrations in the northern section of the research area ranged from 30 to 1710 mg/l over the whole site (Fig. 4k). Figure 2l shows the spatial distribution of nitrate (NO3) concentrations, ranging from 2.39 to 146.19 mg/l, with higher concentrations in the N1-052 and N2-089 wells. The iron (Fe) concentrations varied from 0 to 1.7 mg/l with higher values in the N2-139 well (Fig. 4m). The fluoride (F) concentrations were in the range of 0.1—3.46 mg/l with a low value in N2-103 and high values in the northern part (Fig. 2n). Figure 4o shows the spatial distribution of total alkalinity(T.ALK), which was in the range of 48.7–681 mg/l.
Water quality assessment
The water quality of Wadi Al-Dabab was assessed using the WQI. The relative weight of each WQP was estimated based on its relative importance (Table 3) (Gebrehiwot et al. 2011), and the WQI was computed for each well (Table 4). It should be mentioned that 26.7% of the samples fell into the good water category, 46.7% fell into the poor water category, and 26.7% fell into the water unfit for human consumption category. Figure 5 shows the WQI map of the study area, which was created using GIS. Accordingly, the water quality of Wadi Al-Dabab was classified as “Good” (17.2%), poor (66.7%, very poor (8.3%), and unsuitable (7.9%) drinking water source.
Multivariate statistical analysis
Correlation analysis
Table 5 shows the correlations of the WQP. The results showed that Cl had a strong positive correlation with Mg, T.H, TDS, and EC; HCO3 with T.ALK; Mg with T.H, TDS, and EC; T.H with TDS and EC; TDS with EC and very good positive correlations between SO4 with K, Na, Ca, T.H, TDS, and EC; Cl with Na; K with Na; Na with T.H, TDS, and EC; Ca with T.H, TDS, and EC. While F had a moderate positive correlation with HCO3, Cl, Na, Mg, T.ALK, T.H, TDS, and EC, it had very weak and weak positive correlations with Fe, NO3, SO4, K, Ca. Except for NO3, K, and pH, Fe had a moderate positive correlation with Ca, but it had very weak and weak positive correlations with all other parameters. SO4 showed moderate positive correlations with Cl, Mg; Cl with K and Ca; K with T.H, TDS, and EC; Na with Mg and Ca; Mg with Ca. Weak positive correlations were shown by F with SO4, and K; Fe with SO4, Mg and T.H; NO3 with K; HCO3 with Mg, TDS, and EC; K with Mg and Ca; T.ALK with T.H, Mg, TDS, and EC and weak negative correlations between SO4 with pH; K with pH. Except for SO4, Cl, and Na, NO3 had very weak and weak positive correlations with all other parameters. SO4 showed similar very weak positive correlations with HCO3, and T.ALK; Cl with HCO3, and T.ALK; HCO3 with K, Na, and Ca; T.ALK with K, Na, and Ca and very weak negative correlations between pH with all parameters except for NO3; Fe with K; NO3 with SO4, Cl, and Na.
In general, a significant increase in Cl, Na, and SO4 indicate that dissolved minerals are found in large part of the aquifer. Sodium, conductivity, chloride, and sulfate form strong cluster (Fig. 6), which reflect a possible common source for these ions. The strong correlation between calcium and sulfate and the moderate correlation between magnesium and sulfate indicate that calcareous magnesium materials exist in the study area (Singh et al. 2011). SO4 and K have a strong correlation indicating that their input sources might be similar (Nair et al. 2018). The findings show that calcium and magnesium bicarbonate add alkalinity to water. It was found that WQP had nonlinear patterns such as total alkalinity with conductivity, nitrate, and TDS. As a result, these nonlinear relationships require further investigations to understand better the data correlations and their effects on water quality.
Principal component analysis (PCA)
The number of significant principal components (PCs) was calculated using an eigenvalue ≥ 1.0. (SAS Inc 2018). This study found four significant PCs explained 54.2%, 15%, 10.2%, and 6.9% of the variance. According to the findings, the first four PCs can explain 86.2% t of the total variance in WQP in Wadi Al-Dabab.
To determine the significant WQP, it is necessary to understand how WQP contributes to the major PCs. This study used a threshold value of 0.55 to investigate the significant loadings of WQP on the major PCs. This threshold value suffices to recognize between small and large loadings (Chowdhury and Al-Zahrani 2014; Chowdhury and Husain 2020). Figures 7 and 8 show the loading and scatterplots on the significant PCs for Wadi Al-Dabab. F, SO4, Cl, K, Na, Mg, Ca, TH, TDS, and EC were determined to be higher than the 0.55 threshold on PC1 (Fig. 7), resulting in significant loadings in PC1. HCO3 and T.ALK had significant loadings on PC2. In PC3, Fe was assigned significant loadings. NO3 had significant loadings on PC4.
Figure 8 presents the biplots of the four significant PCs. According to the PC1x PC2 plot, HCO3 and T.ALK were only significantly related with PC2, but F, SO4, Cl, K, Na, Mg, Ca, T.H, TDS, and EC were all substantially associated with PC1. One or two metrics from each cluster may be necessary to represent the water quality. As shown in Fig. 6, a strong cluster with correlation coefficient (r) in the range of 0.67–1 was formed by F, HCO3, and T.ALK. Also, Na, Cl, TDS, EC, Mg, and T.H formed a strong cluster with r in the range of 0.9–1. The four PCs were mainly associated with F, SO4, K, Cl, Ca, Mg, Na, T.H, TDS, EC, HCO3, T.ALK, Fe, and NO3. Excluding pH, fourteen WQP significantly influences the first four PCs explaining 86.2% of the overall variance.
Factor analysis (FA)
FA was applied on four major PCs to obtain VFs (Table 6). According to absolute loading values, the factor loadings were classified into strong (> 0.75), moderate (0.75–0.50), and weak (0.50–0.30) (Lin et al., 2003). SO4, Na, Cl, Mg, Ca, TDS, T.H, and EC had strong positive loadings on VF1, which explained 54.2% of the overall variance, while F and K had a medium loading on VF1. VF2 explained 15% of the overall variance and had strong positive loadings from T.ALK and HCO3, while VF3 explained 10.2% of the overall variance and had strong negative loadings from Fe. VF4 had substantial positive loading from NO3 and explained 6.86 6.86% of the total variance.
To evaluate the possibilities of data reduction, the loadings of the original parameters on the major VFs were assessed. If the original parameter's loading on the major VFs is higher than 0.7 or 0.75, it contributes significantly to temporal variation ( Varol et al. 2012; Liu et al. 2003). The four major VFs explain 86.2% of the variance with strong loadings from SO4, Na, Cl, Mg, Ca, TDS, T.H, HCO3, EC, Fe, T.ALK, and NO3 )Table 4). TDS can be selected as the significant parameter since TDS, EC, Cl, H.T, Mg, and Na are all strongly correlated, as demonstrated in Table 5. As a result, a total of twelve parameters (SO3, Cl, Na, Mg, Ca, T.H, TDS, EC, HCO3, T.ALK, NO3, and Fe) are required to explain 86.24% of the data variance (nearly 80% of the 15 parameters) (Table 4) (Liu et al. 2003). The linear/nonlinear relationships can help to reduce the number of WQP further. Table 7 shows examples of nonlinear relationships for selected parameters, which can be applied for more parameters resulting in a further reduction for WQP.
Conclusions
Based on the assessment of WQP in Wadi Al-Dabab, it is evident that the area is highly susceptible to pollution, both from natural and anthropogenic sources. The two layers of water aquifers are vulnerable to contamination with the shallow free water table being directly affected by pollutants due to its unique properties. On the other hand, the deep confined aquifer composed of fractured rock is dependent on fractured zones for water production and has limited wells, making it less important than the shallow water table. However, both layers are potential targets for pollution, and the area is vulnerable to contamination from various sources including household waste, industrial waste, and construction waste. Sources of pollution in Wadi Al-Dabab include wastewater and industrial waste that reaches the area from Hazran, mixed with sewage water that flows into the area. Used oils, construction waste (e.g., saws), and other waste from car workshops in liquids and solid phases reach the area from Hazran. Household waste that is present on the banks of the Wadi Al-Dhabab and has no general drainage is disposed of by digging wells in the valley.
The study analyzed the spatial variability of WQP in the area and showed that water quality in Wadi Al-Dabab is deteriorating, with most of the water not suitable for drinking purposes unless treated. The chemical analysis revealed that the parameters such as chloride, nitrate, manganese, iron, hardness, bicarbonate, conductivity, total alkalinity, calcium, sodium, total dissolved solids, and sulfate exceeded the WHO and Y.S limits in at least one well. Moreover, the WQI map indicates that 66.7% of the study area had poor water quality, 8.3% very poor, and 7.9% unsuitable for drinking. In light of these findings, effective source protection measures and necessary monitoring plans should be implemented to prevent further deterioration of water quality. The study emphasizes the importance of regular monitoring of WQP, especially in areas prone to pollution. The results can provide useful information to decision makers and local communities in developing effective management strategies to preserve the water resources in Wadi Al-Dabab.
Data availability
The datasets used and/or analyzed during the current study are available from the corresponding author on reasonable request.
References
Ahmed Al-Shargabi W (2015) Environmental contaminants and their impact on some of ground water quality in Taiz Yemen: study evaluation. Am J BioSci 3(2):34
Al-Amry AS (2009) Hydrogeochemistry and origin of fluoride in groundwater of Hidhran & Alburayhi Basin, northwest Taiz City, yemen. Delta J Sci 33(1):10–20
Alansi RQ, Mohammed AMA, Ali MM, Ghalib WAM, Ponnappa SC (2021) Determination of heavy metals in groundwater around Al-Buraihi Sewage station in Taiz City. Yemen J Health Pollut 11(30):1–12. https://doi.org/10.5696/2156-9614-11.30.210604
Alberto WD, del Pilar DM, Valeria AM, Fabiana PS, Cecilia HA, de Los Ángeles BM (2001) Pattern recognition techniques for the evaluation of spatial and temporal variations in water quality. A case study: Suquı́a river Basin (Córdoba–Argentina). Water Res 35(12):2881–2894. https://doi.org/10.1016/S0043-1354(00)00592-3
Al-Omran AM, Mousa MA, AlHarbi MM, Nadeem MEA (2018) Hydrogeochemical characterization and groundwater quality assessment in Al-Hasa, Saudi Arabia. Arab J Geosci. https://doi.org/10.1007/s12517-018-3420-y
Aqeel A, Al-Amry A, Alharbi O (2017) Assessment and geospatial distribution mapping of fluoride concentrations in the groundwater of Al-Howban Basin Taiz-Yemen. Arab J Geosci. https://doi.org/10.1007/s12517-017-3069-y
Bu H, Tan X, Li S, Safety QZ-EE (2010) Temporal and spatial variations of water quality in the Jinshui River of the South Qinling Mts China. Elsevier, Amsterdam
Canada H (2017) Guidelines for Canadian drinking water quality summary table
Chowdhury S, Al-Zahrani M (2014) Water quality change in dam reservoir and shallow aquifer: analysis on trend, seasonal variability and data reduction. Environ Monit Assess 186(10):6127–6143. https://doi.org/10.1007/s10661-014-3844-0
Chowdhury S, Husain T (2020) Reducing the dimension of water quality parameters in source water: an assessment through multivariate analysis on the data from 441 supply systems. J Environ Manag 274(August):111202. https://doi.org/10.1016/j.jenvman.2020.111202
Elhatip H, Hınıs MA, Gülbahar N (2008) Evaluation of the water quality at Tahtali dam watershed in Izmir-Turkey by means of statistical methodology. Stoch Env Res Risk Assess 22(3):391–400. https://doi.org/10.1007/s00477-007-0127-0
EPA(U.S. Environmental Protection Agency) (2018) 2018 Edition of the drinking water standards and health advisories tables (EPA 822-F-18-001)
Gebrehiwot AB, Tadesse N, Jigar E (2011) Application of water quality index to assess suitablity of groundwater quality for drinking purposes in Hantebet watershed, Tigray, Northern Ethiopia. ISABB J Food Agric Sci 1(1):22–30
Helena B, Pardo R, Vega M, Barrado E, Research JF-W (2000) Temporal evolution of groundwater composition in an alluvial aquifer (Pisuerga River, Spain) by principal component analysis. Elsevier, Amsterdam
Hellegers P, Perry C, Allaulaqi N, Rahman A, Allhebshi AM (2008) Incentives to reduce groundwater extraction in Yemen
Igboekwe MU, Akankpo AO (2011) Application of geographic information system (GIS)in mapping groundwater Quality in Uyo. Nigeria Int J Geosci 02(04):394–397. https://doi.org/10.4236/ijg.2011.24042
Jackson JE (1991) A user’s guide to principal components. Wiley, Hoboken
Lee JY, Cheon JY, Lee KK, Lee SY, Lee MH (2001) Statistical evaluation of geochemical parameter distribution in a ground water system contaminated with petroleum hydrocarbons. J Environ Qual 30(5):1548–1563
Liu CW, Lin KH, Kuo YM (2003) Application of factor analysis in the assessment of groundwater quality in a blackfoot disease area in Taiwan. Sci Total Environ 313:77–89
Metwali RM (2003) Water quality of some wells in Taiz City (Yemen Republic) and its surroundings. Folia Microbiol 48(1):90–94. https://doi.org/10.1007/BF02931282
Mohamed MMA, Hassane AB (2016) Hydrochemistry assessment of groundwater quality in Al-Ain city. UAE Environ Earth Sci 75(4):1–17. https://doi.org/10.1007/s12665-015-4942-1
Muangthong S, Shrestha S (2015) Assessment of surface water quality using multivariate statistical techniques: case study of the Nampong River and Songkhram River. Thailand Environ Monit Assess 187(9):548. https://doi.org/10.1007/s10661-015-4774-1
Nair HC, Padmalal D, Joseph A, Gopinthan VP (2018) Hydrogeochemistry and water quality assessment of shallow aquifers in the western flanks of Southern Western Ghats, SW India
Naser R, Bakkali M, Belghyti D (2020) GIS and statistical evaluation of fluoride content in southern part of upper rasyan aquifer. Taiz, Yemen. https://doi.org/10.5772/intechopen.91329
Nasher G, Al-Sayyaghi A, Al-Matary A (2013) Identification and evaluation of the hydrogeochemical processes of the lower part of Wadi Siham catchment area, Tihama plain. Yemen Arab J Geosci 6(6):2131–2146. https://doi.org/10.1007/s12517-011-0471-8
Nazzal Y, Ahmed I, Al-Arifi NSN, Ghrefat H, Batayneh A, Abuamarah BA, Zaidi FK (2015) A combined hydrochemical-statistical analysis of Saq aquifer, Northwestern part of the Kingdom of Saudi Arabia. Geosci J 19(1):145–155. https://doi.org/10.1007/s12303-014-0016-8
Olsen RL, Chappell RW, Loftis JC (2012) Water quality sample collection, data treatment and results presentation for principal components analysis—literature review and Illinois River watershed case study. Water Res 46(9):3110–3122. https://doi.org/10.1016/j.watres.2012.03.028
Prathumratana L, Sthiannopkao S, Kim KW (2008) The relationship of climatic and hydrological parameters to surface water quality in the lower Mekong River. Environ Int 34(6):860–866
Qadir A, Malik RN, Husain SZ (2008) Spatio-temporal variations in water quality of Nullah Aik-tributary of the river Chenab, Pakistan. Environ Monit Assess 140:43–59
Ramakrishnaiah CR, Sadashivaiah C, Ranganna G (2009) Assessment of water quality index for the groundwater in Tumkur taluk, Karnataka state. India E-J Chem 6(2):523–530. https://doi.org/10.1155/2009/757424
Reghunath R, Murthy TRS, Raghavan BR (2002) The utility of multivariate statistical techniques in hydrogeochemical studies: an example from Karnataka, India. Water Res 36:2437–2442
Rice EW, Baird RB, Eaton AD (2017) Standard methods for the examination of water and wastewater, 23rd Edition. American Water Works Association
Sahu P, Sikdar PK (2008) Hydrochemical framework of the aquifer in and around East Kolkata Wetlands, West Bengal India. Environ Geol 55(4):823–835. https://doi.org/10.1007/s00254-007-1034-x
Saleh SM, Al-Alaiy SG, Abdul-Razzak B (2017) Application of water quality index to assessment of groundwater quality. Univ Aden J Nat Appl Sci 21(1):125–134
SAS Inc (2018) Statistical discovery software. SAS Institute Inc, NC, USA
Sh Nasher GA, A El-Sagheer AA (2012) Water quality evaluation of bura’a natural protected area, Hodeidah, Yemen. In: Academic and Scholarly Research Journal 4(6)
Shrestha S, Kazama F (2007) Assessment of surface water quality using multivariate statistical techniques: a case study of the Fuji river basin. Japan Environ Model Softw 22(4):464–475. https://doi.org/10.1016/j.envsoft.2006.02.001
Simeonov V, Stratis J, Samara C, Research GZ-W (2003), Undefined. (n.d.). Assessment of the surface water quality in Northern Greece. Elsevier
Singh KP, Malik A, Mohan D, Sinha S (2004) Multivariate statistical techniques for the evaluation of spatial and temporal variations in water quality of Gomti River (India)—a case study. Water Res 38(18):3980–3992. https://doi.org/10.1016/j.watres.2004.06.011
Singh KP, Malik A, Sinha S (2005) Water quality assessment and apportionment of pollution sources of Gomti river (India) using multivariate statistical techniques—a case study. Anal Chim Acta 538:355–374. https://doi.org/10.1016/j.aca.2005.02.006
Singh AK, Tewary BK, Sinha A (2011) Hydrochemistry and quality assessment of groundwater in part of NOIDA metropolitan city, Uttar Pradesh. J Geol Soc India 78(6):523–540. https://doi.org/10.1007/s12594-011-0124-2
Taher TM (2016) Gestion de l’exploitation des eaux souterraines dans le Bassin de Sana’a, Yemen: approche d’une collectivité locale. Hydrogeol J 24(6):1593–1605. https://doi.org/10.1007/s10040-016-1435-6
Toumi N, Hussein BHM, Rafrafi S, El kassas N (2015) Groundwater quality and hydrochemical properties of Al-Ula Region. Saudi Arabia Environ Monit Assess 187(3):1–16. https://doi.org/10.1007/s10661-014-4241-4
Varol M, Gökot B, Bekleyen A, Şen B (2012) Spatial and temporal variations in surface water quality of the dam reservoirs in the Tigris River basin, Turkey. CATENA 92:11–21. https://doi.org/10.1016/j.catena.2011.11.013
Vega M, Pardo R, Barrado E, Debán L (1998) Assessment of seasonal and polluting effects on the quality of river water by exploratory data analysis. Water Res 32(12):3581–3592
WHO (2017) Guidelines for drinking-water quality: Fourth Edition incorporating the first addendum. World Health Organization, Geneva, Switzerland. License: CC BY-NC-SA 3.0 IGO
Zeilhofer P, Lima EBNR, Lima GAR (2006) Spatial patterns of water quality in the Cuiabá River Basin. Central Brazil Environ Monit Assess 123(1–3):41–62. https://doi.org/10.1007/s10661-005-9114-4
Zeilhofer P, Zeilhofer LVAC, Hardoim EL, de Lima ZM, Oliveira CS (2007) GIS applications for mapping and spatial modeling of urban-use water quality: a case study in District of Cuiabá, Mato Grosso. Brazil Cadernos De Saúde Pública 23(4):875–884. https://doi.org/10.1590/S0102-311X2007000400015
Zhang Q, Li Z, Zeng G, Li J, Fang Y, Yuan Q, Wang Y, Ye F (2009a) Assessment of surface water quality using multivariate statistical techniques in red soil hilly region: a case study of Xiangjiang watershed. China Environ Monit Assess 152(1–4):123–131. https://doi.org/10.1007/s10661-008-0301-y
Zhang Y, Guo F, Meng W, Wang X-Q (2009b) Water quality assessment and source identification of Daliao river basin using multivariate statistical methods. Environ Monit Assess 152(1–4):105–121. https://doi.org/10.1007/s10661-008-0300-z
Acknowledgements
The authors would like to acknowledge the support provided by the Interdisciplinary Research Center for Membranes and Water Security at KFUPM.
Funding
The authors declare that this work has been done in the absence of any external source of funding.
Author information
Authors and Affiliations
Corresponding author
Ethics declarations
Conflict of interest
The authors declare that they have no known competing financial interests or personal relationships that could have appeared to influence the work reported in this paper.
Ethics approval
This article does not contain any studies with human participants or animals performed by any of the authors.
Consent to participate
Not applicable.
Consent for publication
Not applicable.
Additional information
Publisher's Note
Springer Nature remains neutral with regard to jurisdictional claims in published maps and institutional affiliations.
Rights and permissions
Open Access This article is licensed under a Creative Commons Attribution 4.0 International License, which permits use, sharing, adaptation, distribution and reproduction in any medium or format, as long as you give appropriate credit to the original author(s) and the source, provide a link to the Creative Commons licence, and indicate if changes were made. The images or other third party material in this article are included in the article's Creative Commons licence, unless indicated otherwise in a credit line to the material. If material is not included in the article's Creative Commons licence and your intended use is not permitted by statutory regulation or exceeds the permitted use, you will need to obtain permission directly from the copyright holder. To view a copy of this licence, visit http://creativecommons.org/licenses/by/4.0/.
About this article
Cite this article
AL-Areeq, A.M., Chowdhury, S., Rahman, M.T. et al. Assessment of water quality using multivariate statistics and geographical information systems (GIS) of Wadi Aldabab, Taiz, Yemen. Appl Water Sci 13, 169 (2023). https://doi.org/10.1007/s13201-023-01973-8
Received:
Accepted:
Published:
DOI: https://doi.org/10.1007/s13201-023-01973-8