Abstract
Due to limitations imposed by cored wells, lithological data are often incomplete, and correct identification of lithofacies is problematic. Identification is actually an issue of pattern recognition, and based on newly proved findings, LightGBM (light gradient boosting machine) is considered to be an excellent pattern recognizer and, therefore, well suited for recognizing lithofacies. To remove remaining disadvantageous features and to further enhance the prediction performance of LightGBM, CRBM (continuous restricted Boltzmann machine) and AFSA (artificial fish swarm algorithm) are adopted as assistants to provide, respectively, high-quality learning data and to create optimal hyper-parameter settings during data processing. Subsequently, a predictor characterized by new ensemble learning is proposed, named CRBM-AFSA-LightGBM. To establish comprehensive verification, several validations are designed based on logging data derived from pre-salt carbonate reservoirs of the Santos Basin. Validations demonstrate the effectiveness and significance of integrating CRBM and AFSA; a further two validations are aimed at revealing whether a change in the learning data has an impact on prediction. To highlight the validation effect, PNN (probabilistic neural network) and SVM (support vector machine) are introduced as contrasting predictors. The test results demonstrate three important points: (1) CRBM and AFSA are preferred to assist in the capability of LightGBM; (2) the LightGBM-cored predictor performs better when compared with PNN-cored and SVM-cored predictors, especially when dealing with larger-scale learning data; (3) better robustness of the new predictor because reliable identification still can be achieved even when learning samples are sparse. Because all the validation evidences are optimistic, CRBM-AFSA-LightGBM is verified as a highly efficient and robust prediction tool for lithofacies identification in carbonate reservoirs.














Similar content being viewed by others
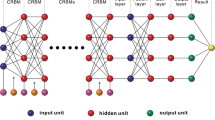
Data Availability Statements
The data that support the findings of this study are available from Sinopec Group but restrictions apply to the availability of these data, which were used under license for the current study, and so are not publicly available. Data are however available from the authors upon reasonable request and with permission of Sinopec Group.
References
Ahlbrandt TS (2002) Future petroleum energy resources of the world. Int Geol 44:1092–1104
Ahmadi MA, Zendehboudi S, Lohi A, Elkamel A, Chatzis I (2013) Reservoir permeability prediction by neural networks combined with hybrid genetic algorithm and particle swarm optimization. Geophys Prospect 61(3):582–598
Al-Anazi A, Gates ID (2010a) A support vector machine algorithm to classify lithofacies and model permeability in heterogeneous reservoirs. Eng Geol 114(3–4):267–277
Al-Anazi A, Gates ID (2010b) On the capability of support vector machines to classify lithology from well logs. Nat Resour Res 19(2):125–139
Alves TM, Fetter M, Cláudio L, Cartwright JA, Cosgrove J, Adriana G (2017) An incomplete correlation between pre-salt topography, top reservoir erosion, and salt deformation in deep-water Santos Basin (SE Brazil). Mar Petrol Geol 79:300–320
Ao Y, Li H, Zhu L, Ali S, Yang Z (2019) Logging lithology discrimination in the prototype similarity space with random forest. IEEE Geosci Remote Sens Lett 16(5):687–691
Burke JA, Schmidt AW, Campbell J, Raymond L (1969) The litho-porosity cross plot: a method of determining rock characteristics for computation of log data. Log Analyst 10:25–43
Carminatti M, Dias JL, Wolff B (2009) From turbidites to carbonates: breaking paradigms in deep waters. Offshore Technology Conference, 4–7 May, Houston, Texas, USA
Castro JC, Azambuja Filho NC, Xavier AAPG (1981) Fácies e analise estratigráfica da Formação Lagoa Feia, Cretáceo Inferior da Bacia da Campos. VIII Congreso Geológico Argentino, 20–26 September. San Luis 2:567–576
Chen T, Guestrin C (2016) XGboost: A scalable tree boosting system. ACM SIGKDD International Conference on Konwledge Discovery and Data Mining, 785–794
Chen Y, Lu L, Li X (2014) Application of continuous restricted Boltzmann machine to identify multivariate geochemical anomaly. J Geochem Explor 140(4):56–63
Chen H, Murray AF (2003) Continuous restricted Boltzmann machine with an implementable training algorithm. IEE Proc vis Image Signal Process 150(3):153–158
Cobbold PR, Szatmari P, Demercian LS, Coelho D, Rossello EA (1995) Seismic experimental evidence for thin-skinned horizontal shortening by convergent radial gliding on evaporites, deep-water Santos Basin. In: Jackson MPA, Roberts RG, Snelson S (eds) Salt Tectonics: a Global Perspective, vol 65. AAPG. Tulsa, Memoir, pp 305–321
Cobbold PR, Meisling KE, Mount VS (2001) Reactivation of an obliquely rifted margin, Campos and Santos basins, southeastern Brazil. AAPG Bull 11:1925–1944
Davison I (2007) Geology and tectonics of the south Atlantic Brazilian salt basins. In: Reis AC, Butler RWH, Graham RH (Eds.) Deformation of the Continental Crust: the Legacy of Mike Coward. Geological Society, London, pp. 345–359. Special Publications, 272
Demercian LS, Szatmari P, Cobbold PR (1993) Style and pattern of salt diapirs due to thin-skinned gravitational gliding, Campos and Santos basins, offshore Brazil. Tectonophysics 228:393–433
Dev VA, Eden MR (2019) Formation lithology classification using scalable gradient boosted decision trees. Comput Chem Eng 128:392–404
Doventon JH (1994) Geologic Log Interpretation: SEPM Short Course #29
Eberhart RC, Kennedy J (1995) A new optimizer using particle swarm theory. Proceedings of the 6th Symposium on Micro Machine and Human Science, 39–43
Fawcett T (2005) An introduction to ROC analysis. Pattern Recogn Lett 27(8):861–874
Feng R, Dario G, Niels B (2021) Uncertainty quantification in fault detection using convolutional neural networks. Geophysics 86(3):M41–M48
Feng R (2021) Improving uncertainty analysis in well log classification by machine learning with a scaling algorithm. J Petrol Sci Eng 196:107995
Gao H, Ye Z, Dong J, Gao H, Ouyang D (2020) Predicting drug/phospholipid complexation by the LightGBM method. Chem Phys Lett 747:137354
Gibbs PB, Brush ER, Fiduk JC (20030 The evolution of the syn-rift and transition phases of the central/southern Brazilian and west African conjugate margins: the implications for source rock distribution in time and space, and their recognition on seismic data. VIII International Congress of the Brazilian Geophysical Society, 14 September, Rio de Janeiro, Brazil
Goldberg DE (1989) Genetic algorithm in search optimization and machine learning. Addison Wesley, xiii(7): 2104–2116
Goovaerts P (1997) Geostatistics for Natural Resources Evaluation. Oxford University Press, New York
Gu YF, Bao ZD, Rui ZH (2018) Complex lithofacies identification using improved probabilistic neural networks. Petrophysics 59(2):245–267
Gu YF, Bao ZD, Song XM, Patil S, Ling KG (2019) Complex lithology prediction using probabilistic neural network improved by continuous restricted Boltzmann machine and particle swarm optimization. J Petrol Sci Eng 179:966–978
Gu YF, Zhang ZM, Zhang DM, Zhu YX, Bao ZD, Zhang DY (2020) Complex lithology prediction using mean impact value, particle swarm optimization, and probabilistic neural network techniques. Acta Geophys 68(6):1727–1752
Gu YF, Bao ZD, Zhang DY (2021) A smart predictor used for lithologies of tight sandstone reservoirs: a case study of member of Chang 4+5, Jiyuan Oilfield, Ordos Basin. Petrol Sci Technol 39(7–8):175–195
Guan X, Yin YX (2012) An improved artificial fish swarm algorithm and its application. Adv Mater Res 433–440:4434–4438
Guardado LR, Gamboa LAP, Lucchesi CF (1989) Petroleum geology of the Campos Basin, Brazil, a model for a producing Atlantic-type basin. In: Edwards JD, Santogrossi PA (Eds.), Divergent/Passive Margin Basins: AAPG Memoir 48. AAPG, Tulsa, Oklahoma, USA, pp. 3–79
Guardado LR, Spadini AR, Brandão JSL (2000) Petroleum system of the campos Basin, Brazil. In: Mello MR, Katz BJ (Eds.), Petroleum systems of South Atlantic Margins: AAPG Memoir 73. AAPG, Tulsa, Oklahoma, USA, pp. 317–324
Hanley JA, Mcneil BJ (1982) The meaning and use of the area under a receiver operating characteristic (ROC) curve. Radiology 143(1):29–36
Harrington B, P.D., (2018) Feature expansion by a continuous restricted Boltzmann machine for near-infrared spectrometric calibration. Anal Chim Acta 1010:20–28
Hsieh BZ, Lewis C, Lin ZS (2005) Lithology identification of aquifers from geophysical well logs and fuzzy logic analysis: Shui-Lin area, Taiwan. Computers Geosci 31(3):263–275
Huc AY (2004) Petroleum in the south Atlantic. Oil Gas Sci Technol 59(3):243–253
Jain S, Kumar A, Bajaj V (2016) Technique for QRS complex detection using particle swarm optimisation. IET Sci Meas Technol 10(6):626–636
Jiang X, Osl M, Kim J, Ohno-Machado L (2012) Calibrating predictive model estimates to support personalized medicine. J Am Med Inform Assoc 19(2):263–274
Ke G, Meng Q, Finley T, Wang T, Chen W (2017) LightGBM: A highly efficient gradient boosting decision tree. Adv Neural Inform Process Syst 30:3146–3154
Larsen AL, Ulvmoen M, Omre H, Buland A (2006) Bayesian lithology/fluid prediction and simulation on the basis of a Markov-chain prior model. Geophysics 71(5):R69–R78
Li LX, Shao ZJ, Qian JX (2002) An optimizing method based on autonomous animals: fish-swarm algorithm. Syst Eng Theory Pract 22(11):32–38
Lilliefors HW (1967) On the kolmogorov-smirnov test for normality with mean and variance unknown. Publ Am Stat Assoc 62(318):399–402
Liu YJ, Huang CY, Zhou YQ, Lu YC, Ma Q (2020) The controlling factors of lacustrine shale lithofacies in the upper Yangtze platform (south china) using artificial neural networks. Marine Petroleum Geol 118:104350
Lucia FJ (2007) Carbonate Reservoir Characterization. Springer-Verlag, Berlin Heidelberg
Ma C, He R (2019) Green wave traffic control system optimization based on adaptive genetic-artificial fish swarm algorithm. Neural Comput Appl 31(7):2073–2083
Meisling KE, Cobbold PR, Mount VS (2001) Segmentation of an obliquely rifted margin, Campos and Santos basins, southeastern Brazil. AAPG Bull 11:1903–1924
Minh CC, Saldungaray P, Belowi AR, Ong LW, Al-Mudhhi MA (2011) Combining resistivity and capture sigma logs for formation evaluation in unknown water salinity–a case study in a mature carbonate field. SPE Reservoir Eval Eng 14(4):205–229
Modica CJ, Brush ER (2004) Post-rift sequence stratigraphy, paleogeography, and fill history of the deepwater Santos Basin, offshore southeast Brazil. AAPG Bull 88:923–946
Mohriak WU, Nemcok M, Enciso G (2008) South Atlantic divergent margin evolution: rift-border uplift and salt tectonics in the basins of SE Brazil. In: Pankhurst RJ, Trouw RAJ, Brito Neves BB, de Wit MJ (Eds.), West Gondwana Pre-cenozoic Correlations across the South Atlantic Region. Geological Society, London, pp. 365–398. Special Publications, 294
Neshat M, Sepidnam G, Sargolzaei M, Toosi AN (2014) Artificial fish swarm algorithm: a survey of the state-of-the-art, hybridization, combinatorial and indicative applications. Artif Intell Rev 42(4):965–997
Niculescu-Mizil A, Caruana R (2005) Predicting good probabilities with supervised learning. In: Proceedings of the 22nd International Conference on Machine Learning
Ouadfeul SA, Aliouane L (2013) Lithofacies prediction from well log data using a multilayer perceptron (MLP) and Kohonen’s self-organizing map (SOM)-a case study from the Algerian Sahara. Pattern Recognit Phys 1(1):59–62
Paass G, Birlinghoven S, Augustin DS (2010) Probabilistic reasoning and probabilistic neural networks. Int J Intell Syst 7(1):47–59
Parker JR (2000) Rank and response combination from confusion matrix data. Information Fusion 2(2):113–120
Patra PK, Nayak M, Nayak SK, Gobbak NK (2002) Probabilistic neural network for pattern classification. IEEE 2:1200–1205
Porter CR, Pickett GR, Whitman WW (1969) A method of determining rock characteristics for computation of log data: the litho-porosity cross plot. Log Analyst 6:1–19
Quirk DG, Hirsch KK, Hsu D, Von Nicolai C, Ings SJ, Lassen B, Schoedt NH (2012) Salt tectonics on passive margins: examples from Santos, Campos and Kwanza basins. In: Alsop GI, Archer SG, Hartley AJ, Grant NT, Hodgkinson R (Eds.) Salt Tectonics, Sediments and Prospectivity. Geological Society, London, pp. 207–244. Special Publications, 363
Razali NM, Wah YB (2011) Power comparisons of Shapiro-Wilk, Kolmogorov-Smirnov, Lilliefors and Anderson-Darling tests. J Statist Model Analyt 2(1):21–33
Romiani F, Saberi MH, Riahi MA (2020) Evaluation of reservoir properties and fracture estimation using petrophysical logs in a southwestern Iranian hydrocarbon field. Int J Geomech 4(3):44–56
Sahoo S, Jha MK (2017) Pattern recognition in lithology classification: modeling using neural networks, self-organizing maps and genetic algorithms. Hydrogeol J 25(2):311–330
Sebtosheikh MA, Motafakkerfard R, Riahi MA, Moradi S, Sabety N (2015) Support vector machine method, a new technique for lithology prediction in an Iranian heterogeneous carbonate reservoir using petrophysical well logs. Carbonates Evaporites 30(1):59–68
Shan Y, Zhao R, Xu G, Liebich HM, Zhang Y (2002) Application of probabilistic neural network in the clinical diagnosis of cancers based on clinical chemistry data. Anal Chim Acta 471(1):77–86
Shi Y, Eberhart RC (1988) A modified particle swarm optimizer. Proceedings of IEEE International Conference on Evolutionary Computation, 69–73
Specht DF (1990) Probabilistic neural networks. Neural. Network 3(1):109–118
Sun Z, Jiang B, Li X, Li J, Xiao K (2020) A data-driven approach for lithology identification based on parameter-optimized ensemble learning. Energies 13(15):3903
Sun J, Chen MQ, Li Q, Ren L, Dou MY, Zhang JX (2021) A new method for predicting formation lithology while drilling at horizontal well bit. J Petrol Sci Eng 196:107955
Szatmari P, Mohriak WU (2009) Tectonic control during earth history of World's largest petroleum bearing salt Basins. AAPG Int. Conf. Exhib. Abstracts Volume 650435, 15, November
Tang TB, Murray AF (2006) Adaptive sensor modeling and classification using a continuous restricted Boltzmann machine (CRBM). Neurocomputing 70(7–9):1198–1206
Tewari S, Dwivedi UD (2020) A comparative study of heterogeneous ensemble methods for the identification of geological lithofacies. J Petrol Explor Prod Technol 10:1849–1868
Thompson DL, Stilwell JD, Hall M (2015) Lacustrine carbonate reservoirs from early cretaceous rift lakes of western Gondwana: pre-salt coquinas of Brazil and west Africa. Gondwana Res 28(1):26–51
Tukey JW (1975) Mathematics and the picturing of data. In Proceedings of the International Congress of Mathematicians, 523–531
Wang P, Peng S (2019) On a new method of estimating shear wave velocity from conventional well logs. J Petrol Sci Eng 180:105–123
Xia T, Zhong J, Zhang Y (2018) Non-invasive continuous blood pressure measurement based on mean impact value method, BP neural network, and genetic algorithm. Technol Health Care 26(6):1–15
Yan X, Gu H, Xiao Y, Ren H, Ni J (2019) XGBoost algorithm applied in the interpretation of tight-sand gas reservoir on well logging data. Oil Geophys Prospect 54(2):447–455
Zainal N, Zain AM, Sharif S (2015) Overview of artificial fish swarm algorithm and its applications in industrial problems. Appl Mech Mater 815:253–257
Zhang Y, Salisch HA, Mcpherson JG (1999) Application of neural networks to identify lithofacies from well logs. Explor Geophys 30(2):45–49
Author information
Authors and Affiliations
Contributions
YG: Conceptualization, Methodology, Validation, Writing -Review and Editing. DZ: Writing -Original Draft. ZB: Project Administration.
Corresponding author
Ethics declarations
Competing interest
The authors declare that they have no known competing financial interests or personal relationships that could have appeared to influence the work reported in this paper.
Additional information
Publisher's Note
Springer Nature remains neutral with regard to jurisdictional claims in published maps and institutional affiliations.
Supplementary Information
Below is the link to the electronic supplementary material.
Rights and permissions
About this article
Cite this article
Gu, Y., Zhang, D. & Bao, Z. Carbonate lithofacies identification using an improved light gradient boosting machine and conventional logs: a demonstration using pre-salt lacustrine reservoirs, Santos Basin. Carbonates Evaporites 36, 79 (2021). https://doi.org/10.1007/s13146-021-00746-1
Accepted:
Published:
DOI: https://doi.org/10.1007/s13146-021-00746-1