Abstract
The presented research was performed in order to model the fire risk in a part of Hyrcanian forests of Iran. The fuzzy sets integrated with analytic hierarchy process (AHP) in a decision-making algorithm using geographic information system (GIS) was used to model the fire risk in the study area. The used factors included four major criteria (topographic, biologic, climatic, and human factors) and their 17 sub-criteria. Fuzzy AHP method was used for estimating the importance (weight) of the effective factors in forest fire. Based on this modeling method, the expert ideas were used to express the relative importance and priority of the major criteria and sub-criteria in forest fire risk in the study area. The expert ideas mean was analyzed based on fuzzy extent analysis. Then, the fuzzy weights of criteria and sub-criteria were obtained. The major criteria models and fire risk model were presented based on these fuzzy weights. On the other hand, the spatial data of 17 sub-criteria were provided and organized in GIS to obtain the sub-criteria maps. Each sub-criterion map was converted to raster format and it was reclassified based on risk of its classes to fire occurrence. Then, all sub-criteria maps were converted to fuzzy format using fuzzy membership function in GIS. The fuzzy map of each major criterion (topographic, biologic, climatic, and human criteria) was obtained by weighted overlay of its sub-criteria fuzzy maps considering to major criterion model in GIS. Finally, the fuzzy map of fire risk was obtained by weighted overlay of major criteria fuzzy maps considering to fire risk model in GIS. The actual fire map was used for validation of fire risk model and map. The results showed that the fuzzy estimated weights of human, biologic, climatic, and topographic criteria in fire risk were 0.301, 0.2595, 0.2315, and 0.208, respectively. The results obtained from the fire risk map showed that 38.74% of the study area has very high and high risk for fire occurrence. Results of validation of the fire risk map showed that 80% of the actual fires were located in the very high and high risk areas in fire risk map. It can show the acceptable accuracy of the fire risk model and map obtained from fuzzy AHP in this study. The obtained fire risk map can be used as a decision support system for predicting of the future fires in the study area.






Similar content being viewed by others
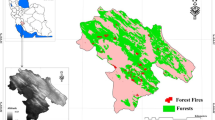
References
Allgower B, Carlson JD, Wagtendonk JWV (2003) Introduction to fire danger rating and remote sensing-will remote sensing enhance wild land fire danger rating? In: Chuvieco E (ed) Wild land fire danger estimation and mapping, the role of remote sensing data. World Scientific, Singapore
Alonso-Betanzos A, Fontenla-Romero O, Guijarro-Berdiñas B, Hernández-Pereira E, Canda E, Jimenez J, Luis Legido J, Muñiz S, Paz-Andrade C, Paz-Andrade MI (2002) A neural network approach for forest fire risk estimation. In: van Harmelen F (ed) Proceedings of the 15th European Conference on Artificial Intelligence. Lyon, France, 643–647.
Atesoglu A (2014) Forest hazard identifying, mapping using satellite imagery-geographic information system and analytic hierarchy process: Bartin-Turkey. J Environ Prot Ecol 15(2):715–725
Bonazountas M, Kallidromitou D, Kassomenos PA, Passas N (2005) Fire risk analysis. Hum Ecol Risk Assess 11:617–626
Chang DY (1996) Applications of the extent analysis method on fuzzy AHP. Eur J Oper Res 95:649–655
Chen SJ, Hwang CL (1992) Fuzzy multiple attribute decision-making. Springer, Berlin
Chuvieco E, Agaudo I, Cocero D, Riano D (2003b) Design of an empirical index to estimate fuel moisture content from NOAA-AVHRR analysis in forest fire danger studies. Int J Remote Sens 24:1621–1637
Chuvieco E, Aguado I, Jurdao S, Pettinari ML, Yebra M, Salas J, Hantson S, de la Riva J, Ibarra P, Rodrigues M, Echeverria M, Azqueta D, Roman MV, Bastarrika A, Martinez S, Recondo C, Zapico E, Martinez-Vega FJ (2014) Integrating geospatial information into fire risk assessment. Int J Wildland Fire 23:606–619
Chuvieco E, Allgower B, Salas J (2003a) Integration of physical and human factors in fire danger assessment. In: Chuvieco E (ed) Wild land fire danger estimation and mapping, the role of remote sensing data. World Scientific, Singapore, pp 197–218
Chuvieco E, Congalton RG (1989) Application of remote sensing and geographic information systems to forest fire hazard mapping. Remote Sens Environ 29:147–159
Cortez PG, Morais A (2007) A data mining approach to predict forest fires using meteorological data. Proceedings of the 13th Portuguese Conference on Artificial Intelligence, Portugal, 512–523.
Cox E (1995) Fuzzy logic for business and industry. Charles River Media.
Dong XU, Li-min D, Guo-fan S, Lei T, Hui W (2005) Forest fire risk zone mapping from satellite images and GIS for Baihe Forestry Bureau, Jilin, China. J For Res 16(3):169–174
Erten E, Kurgun V, Musaolu N (2005) Forest fire risk zone mapping from satellite imagery and GIS, a case study. Civil Engineering Faculty, Remote Sensing Division, p 7p
Eskandari S (2015) Investigation on relationship between climate change and fire in the forests of Golestan forests. Iranian Journal of Forest and Range Protection Research 13(1):1–10
Eskandari S, Chuvieco E (2015) Fire danger assessment in Iran based on geospatial information. Int J Appl Earth Obs Geoinf 42:57–64
Eskandari S, Jalilvand H (2012) Climate change and its impact on number and area of fire of forest areas of Eastern Mazandaran. Proceedings of the Third International Conference on Climate Change and Tree Chronology, Sari, Iran, 15 p.
Eskandari S, Oladi J, Jalilvand H, Saradjian MR (2013a) Role of human factors on fire occurrence in District Three of Neka Zalemroud forests- Iran. World Applied Sciences Journal 27(9):1146–1150
Eskandari S, Oladi J, Jalilvand H, Saradjian MR (2013b) Detection of fire high-risk areas in Northern forests of Iran using Dong model. World Applied Sciences Journal 27(6):770–773
Eskandari S, Oladi J, Jalilvand H, Saradjian MR (2015) Prediction of future forest fires using the MCDM method. Pol J Environ Stud 24(5):2309–2314
Food and Agricultural Organization (FAO) (1986) Wild land fire management terminology. FAO Forestry Paper, Rome
Goncalves ZJ, Lourenco L (1990) Meteorological index of forest fire risk in the Portuguese mainland territory. Proceedings of the International Conference on Forest Fire Research, Coimbra, Portugal, 1–14.
Hernandez-Leal PA, Arbelo M, Gonzalez-Calvo A (2006) Fire risk assessment using satellite data. Adv Space Res 37:741–746
Jaiswal RK, Mukherjee S, Raju DK, Saxena R (2002) Forest fire risk zone mapping from satellite imagery and GIS. Int J Appl Earth Obs Geoinf 4:1–10
Jurdao S, Chuvieco E, Arevalillo JM (2012) Modelling fire ignition probability from satellite estimates of live fuel moisture content. Fire Ecology 8(1):77–97
Kahraman C, Ruan D, Dogan I (2003) Fuzzy group decision-making for facility location selection. Inf Sci 157:135–153
Leung LC, Cao D (2001) On the efficacy of modeling multi-attribute decision making problem using AHP and Sinarchy. Eur J Oper Res 132(1):39–49
Lozano FJ, Suárez-Seoane S, Kelly M, Luis E (2008) A multi-scale approach for modeling fire occurrence probability using satellite data and classification trees: a case study in a mountainous Mediterranean region. Remote Sens Environ 112:708–719
Malczewski J (1999) GIS and multi-criteria decision analysis. Wiley, NewYork
Malczewski J (2002) Fuzzy screening for land suitability analysis. Geogr Environ Model 6:27–39
Martinez J, Vega-Garcia C, Chuvieco E (2009) Human-caused wildfire risk rating for prevention planning in Spain. J Environ Manag 90:1241–1252
Maselli F, Romanelli S, Bottai L, Zipoli G (2003) Use of NOAA-AVHRR NDVI images for the estimation of dynamic fire risk in Mediterranean areas. Remote Sens Environ 86:187–197
Mazandaran Natural Resources Administration (MNRA) (2010) Forestry plan of DTNZ forests. Iran’s Forests, Rangeland and Watershed organization, Mazandaran
Mazandaran Natural Resources Administration (MNRA) (2014) Statistics and data of fire in 2010 in Mazandaran, sari. Protection unit of MNRA, Mazandaran
Merrill DF, Alexander ME (1987) Glossary of Forest fire management terms. National Research Council of Canada, Ontario
Perry DA (1998) The scientific basis of forestry. Annu Rev Ecol Syst 29:435–466
Robinson V (2003) A perspective on the fundamentals of fuzzy sets and their use in geographic information systems. Trans GIS 7:3–30
Rollins MG, Keane RE, Parsons RA (2004) Mapping fuels and fire regimes using remote sensing, ecosystem simulation and gradient modeling. Ecol Appl 14(1):75–95
Rothermel RC (1983) How to predict the spread and intensity of forest and range fires. USDA Forest Service.
Saaty TL (1980) The analytic hierarchy process: planning, priority setting and resource allocation. McGraw-Hill, New York
Saaty TL (2000) Fundamentals of decision making and priority theory with the analytic hierarchy process. RWS, Pittsburg
Saaty TL, Vargas LG (1993) Experiments on rank preservation and reversal in relative measurement. Math Comput Model 17:13–18
Sakr GE, Elhajj IH (2010) Artificial intelligence for forest fire prediction: a comparative study. Proceedings of the Sixth International Conference on Forest Fire Research, Coimbra, pp 653–661
Satir O, Berberoglu S, Donmez C (2016) Mapping regional forest fire probability using artificial neural network model in a Mediterranean forest ecosystem. Geomatics, Natural Hazards and Risk 7(5):1645–1658
Sitanggang IS, Yaakob R, Mustapha N, Ainuddin AN (2013) Predictive models for hotspots occurrence using decision tree algorithm and logistic regression. J Appl Sci 13(2):252–261
Sowmya SV, Somashekar RK (2010) Application of remote sensing and geographical information system in mapping forest fire risk zone at Bhadra wildlife sanctuary, India. J Environ Biol 31(6):969–974
Stolle F, Chomitz KM, Lambin EF, Tomich TP (2003) Human ecological intervention and the role of forest fires in human ecology. For Ecol Manag 179:277–292
Stolzenburg W (2001) Fire in the rainforest. Nature Conservancy 31:22–27
Taylor SW, Alexander ME (2006) Science, technology and human factors in fire danger rating: the Canadian experience. International Journal of Wild land Fire 15:121–135
Teodoro AC, Duarte L (2013) Forest fire risk maps: a GIS open source application—a case study in Norwest of Portugal. Int J Geogr Inf Sci 27(4):699–720
Vadrevu KP, Eaturu A, Badarinath KVS (2009) Fire risk evaluation using multicriteria analysis, a case study. Environment Monitoring and Assessment 166(1–4):223–239
Vahidnia MH, Alesheikh AA, Alimohammadi A (2009) Hospital site selection using fuzzy AHP and its derivatives. J Environ Manag 90:3048–3056
Vakalis D, Sarimveis H, Kiranoudis CT, Alexandridis A, Bafas GV (2004) A GIS based operational system for wildland fire crisis management, I. Mathematical modelling and simulation. Appl Math Model 28(4):389–410
Van Wagner CE (1993) Prediction of crown fire behavior in two stands of Jack Pine. Can J For Res 18:818–820
Vasconcelo MJ, Silva S, Tome M, Alvim M, Perelra JMC (2001) Spatial prediction of fire ignition probabilities: comparing logistic regression and neural networks. Photogramm Eng Remote Sens 67(1):73–81
Vasilakos C, Kalabokidis K, Hatzopoulos J, Matsinos I (2009) Identifying wildland fire ignition factors through sensitivity analysis of a neural network. Nat Hazards 50(1):125–143
Vazquez A, Moreno JM (2001) Spatial distribution of forest fires in Sierra de Gredos (central Spain). For Ecol Manag 147(1):55–65
Wang YM, Elhag TMS, Hau ZS (2006) A modified fuzzy logarithmic least squares method for fuzzy analytic hierarchy process. Fuzzy Sets Syst 157(23):3055–3071
Wu CR, Lin CT, Chen HC (2007) Optimal selection of location for Taiwanese hospitals to ensure a competitive advantage by using the analytic hierarchy process and sensitivity analysis. Build Environ 42:1431–1444
Yanar TA, Akyurek A (2006) The enhancement of the cell-based GIS analyses with fuzzy processing capabilities. Inf Sci 176:1067–1085
Ying MW, Liu J, Elhag TMS (2008) An integrated AHP-DEA methodology for bridge risk assessment. Comput Ind Eng 54(3):513–525
Zadeh LA (1965) Fuzzy sets. Information and Content 8:338–356
Zumbrunnen T, Pezzattic GB, Menéndezd P, Bugmann H, Bürgia M, Conederac M (2011) Weather and human impacts on forest fires: 100 years of fire history in two climatic regions of Switzerland. For Ecol Manag 261:2188–2199
Author information
Authors and Affiliations
Corresponding author
Rights and permissions
About this article
Cite this article
Eskandari, S. A new approach for forest fire risk modeling using fuzzy AHP and GIS in Hyrcanian forests of Iran. Arab J Geosci 10, 190 (2017). https://doi.org/10.1007/s12517-017-2976-2
Received:
Accepted:
Published:
DOI: https://doi.org/10.1007/s12517-017-2976-2