Abstract
Variation in freshwater flow into estuaries can profoundly alter abundance of estuarine organisms through a variety of mechanisms. In the San Francisco Estuary, California, an annual abundance index of juvenile longfin smelt Spirinchus thaleichthys has varied by ~ 100-fold over the range of flow, and over the last five decades the index has declined by over 100-fold. The unknown mechanisms for variation with flow may include removal of larvae by freshwater diversions during low-flow periods. Using data from larval trawl surveys during January–March 2009–2020, we estimated larval population size, its response to freshwater flow, and losses of larvae to freshwater diversions. Population size was estimated by a Bayesian hierarchical model linking a process model, with salinity and water clarity as covariates, to an observation model representing catch by a negative binomial distribution. Population size averaged across surveys within years—an index of the number of larvae produced—decreased over the study period from ~ 109 to 108 larvae. Population size was unrelated to freshwater flow in the year of hatching but positively related to the subsequent juvenile abundance index. Thus, the mechanisms underlying the strong variability in the annual abundance index of longfin smelt with freshwater flow are constrained to occur after March. Estimated proportional losses to water diversions accumulated over the period of vulnerability averaged 1.5% of the population, too low to measurably influence population dynamics.
Similar content being viewed by others
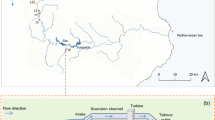
Introduction
The effects of human activities on populations of pelagic organisms in estuaries can be difficult to assess. First, these populations are subject to myriad influences, not all under human control. Second, pelagic organisms are largely unseen and their distribution and abundance can be inferred only through sampling, which involves a known but unresolved set of difficulties including uncertain capture efficiency and its size dependence, incomplete coverage of the species’ range, overdispersion, and small sample sizes. Third, the influences on populations may occur at time scales shorter than sampling intervals or at unobserved locations or life stages. And fourth, the dynamics of fish or macroinvertebrate populations are often assessed through indices assumed to be correlated with population size, whereas the actual number of organisms in the population can be more useful for understanding the environmental cost of human activities and the risk of extirpation.
Controversies arise when human activities induce damage which must be balanced against the value of these activities, or mitigated at a cost that may exceed the value of those activities. In estuaries, numerous such controversies revolve around eutrophication, contamination, over-fishing, protection of at-risk species, and uses of fresh water (Nichols et al. 1986; Montagna et al. 2002; Paerl et al. 2006; Breitburg et al. 2018). Resolution can be clouded by the uncertainties in the magnitude of the harm to the species of concern, which is most clearly defined relative to population size (Rothschild et al. 1994).
In the San Francisco Estuary (SFE), the most intense controversies surround the use of freshwater in the watershed. California’s climate is Mediterranean, with most of the precipitation occurring in winter-spring, high interannual variability, and a pronounced latitudinal gradient with greater precipitation in the northern part of the state. About 29 million people and a US $50 billion agriculture industry rely on the watershed for all or part of their water supply. At the same time, the highly urbanized and modified estuary (Nichols et al. 1986; Whipple et al. 2012) is home to numerous species in decline, resulting in government actions for protection that have come into conflict with the needs of water users (Williams 1989; Hanak et al. 2008; Lund et al. 2008).
The temporal and spatial patterns of precipitation in this watershed would constrain its intensive use for agriculture, industry, and a large urban population were it not for the transfer of water from times and places of abundance to those of shortage. Extensive water infrastructure, built throughout the watershed over the last seven decades, achieves this transfer. The centerpiece of this system is a set of immense pumps that divert up to ~ 36 million m3 day−1 of freshwater from the tidal freshwater reach of the California Delta formed by the confluence of the Sacramento and San Joaquin Rivers (Fig. 1). During the wet season (roughly November–May), these pumps are operated to capture water to be used or stored south of the Delta, while during the dry season (roughly June–October) water stored in reservoirs to the north of the Delta is released into the rivers and some of it is pumped out in the Delta for use to the south. These diversions remove 29% (median; range 5–54% from 1980 to 2020) of the annual river flow into the Delta (CNRA 2021).
Map of the upper San Francisco Estuary showing all sampling stations from the Smelt Larva Survey that were used in the analysis. All circles are stations used to estimate the response of abundance to covariates. Filled circles are stations used also to estimate population size, each of which represents an area enclosed in polygons; lines in black enclose the four stations used to characterize population density in the south Delta and thereby loss rates to diversions. Diamond shapes indicate intake sites for water-diversion facilities in the south Delta. Numbers indicate approximate locations of the salinity 2 isohaline for X2 at 55, 75, and 95 km
River flow into the Delta is termed “inflow,” while “outflow” equals inflow less diversion (or export) flow and net consumption within the Delta. These and other net flows are reported as daily estimates by the Dayflow accounting program (CNRA 2021).
The diversion of young fish from the estuary has a long history of contention. The water-diversion facilities are equipped with louvers that divert fish out of the flow in order to return them to the estuary, but the louvers are ineffective for fish smaller than ~ 20 mm, whose losses to diversions are unobserved (Brown et al. 1996). Early concerns over the role of diversion losses in a decline in abundance of striped bass Morone saxatilis (Stevens et al. 1985) were not supported by subsequent analyses showing density dependence of juveniles and increasing mortality of adults (Kimmerer et al. 2000, 2001). More recently the focus of concerns over diversion effects has been on the endangered delta smelt Hypomesus transpacificus. As an endemic species in brackish to fresh regions of the estuary with a 1-year life cycle and a declining population, this fish appears uniquely vulnerable to losses to diversions during both adult and larval life stages (Moyle et al. 1992; Kimmerer 2008; Korman et al. 2021), and as a result efforts to protect delta smelt, including limitations on water diversions, have engendered intense controversy (Moyle et al. 2018).
The longfin smelt Spirinchus thaleichthys is another small pelagic fish in the estuary that may be vulnerable to losses in water diversions. The annual abundance index of juvenile longfin smelt has declined by ~ 100-fold over the last five decades (Nobriga and Rosenfield 2016, and see below), and the population is listed as threatened by the State of California and as eligible for listing under US endangered species regulations (Federal Register 77 FR 19755, 85 FR 73164). However, its distribution may make it less vulnerable than delta smelt to diversions. Much of the life cycle occurs in brackish to saline waters far removed from the diversion points (Rosenfield and Baxter 2007), and a substantial spawning population has been found in South San Francisco Bay (Hobbs et al. 2010) over 200 km by water from the diversion point. Although the species can spawn in freshwater (Chigbu 2000; Moyle 2002), in the SFE longfin smelt hatch mostly in brackish water and the larvae move seaward as they develop (Lewis et al. 2020, Gross et al. in review).
Superimposed on the ~ 100-fold decline in the annual abundance index is a strong interannual covariation with winter–spring freshwater flow into the estuary, also of ~ 100-fold magnitude (Stevens and Miller 1983). Several candidate mechanisms for this variation have been suggested (Jassby et al. 1995; Kimmerer 2002), one of which is high proportional losses to diversions during years of low freshwater flow. These losses have been somewhat mitigated following the 2009 Incidental Take Permit (CDFW 2009) which set limits on the southward flows of water in the southern Delta during the January–March hatching period of longfin smelt; these limits were met by reducing diversion flow (Fig. S1).
Using data on distributions of catches from six surveys of fish abundance, we determined which life stages were most vulnerable to diversion losses based on their occurrence near the diversion points and in low-salinity water. We then estimated the population size of longfin smelt larvae during winter 2009–2020 in the upper San Francisco Estuary using data from a larval survey aimed at this species. This study attempted to answer two questions: (1) Does the population size of larval longfin smelt vary with freshwater flow into the estuary? and (2) What is the likely contribution of diversion losses of larvae to the long-term decline in the fall abundance index and to its relationship to freshwater flow? Using the same data set for 2013 and 2017, a related study applied particle-tracking methods with a Bayesian analysis to back-calculate hatching locations from observed locations of larvae (Gross et al. in review). That analysis also estimated cumulative losses of larvae to diversions for those years.
Methods
Study Site and Species
The San Francisco Estuary (SFE) links the rivers of California’s Central Valley to the Pacific Ocean via the California Delta and a series of shallow bays with deeper channels: Suisun Bay, San Pablo Bay, and San Francisco Bay (Fig. 1). The Delta is largely fresh except for intrusion of salt from the west during dry periods. The salinity gradient shifts by ~ 50 km in response to seasonal and interannual variation in freshwater flows (Jassby et al. 1995; Monismith et al. 2002). During January–March of the study years, freshwater outflow (from the Delta into Suisun Bay) had a median of 534 × 106 m3 day−1 and a range of (12–658) × 106 m3 day−1, while diversion flows had a median of 13 × 106 m3 day−1 and a range of (4–29) × 106 m3 day−1 (CNRA 2021). The highest diversion flow rates of this period occurred in 2017 to capture some of the high winter flows in that very wet year.
Longfin smelt is native to lakes and estuaries from the SFE to Alaska (Moyle 2002). In the SFE, longfin smelt spawn mostly at age 2 years, laying adhesive eggs that hatch in Suisun Bay, San Pablo Bay, the western Delta, and south San Francisco Bay during January–March (Moyle 2002; Hobbs et al. 2010; Gross et al. in review). Longfin smelt larvae were abundant in Suisun Marsh during high-flow periods in February and March (Meng and Matern 2001). Although spawning habitat has been described as freshwater, otolith microchemistry of adults and distributions of larvae suggested that they hatch at an average salinity of 2 (Hobbs et al. 2010; Lewis et al. 2020).
Early larvae in the SFE are surface-oriented and most abundant around a salinity of 2, moving seaward with the net flow, but as they reach approximately 10–12 mm they spread throughout the water column, and begin to migrate vertically on a diel pattern and possibly also a tidal pattern (Bennett et al. 2002; Dege and Brown 2004). Larval longfin smelt that hatch in the northern estuary disperse at least as far as San Pablo Bay (Grimaldo et al. 2021). Both tidal vertical migration and distribution into the lower part of the water column may help to retain the larvae and possibly move them landward to the low-salinity zone (salinity 0.5–5), as suggested by results of particle-tracking modeling (Kimmerer et al. 2014).
Setting the Context
The background for this study is the long-term declines in a key abundance index of longfin smelt and in its short-term interannual variation with freshwater flow (Jassby et al. 1995; Kimmerer et al. 2009; Nobriga and Rosenfield 2016). The index is derived from catches in the midwater trawl survey conducted annually from September to December in 1967 through 2020 except 1974 and 1979 (Moyle et al. 1992; https://www.dfg.ca.gov/delta/data/fmwt/indices.asp, accessed 29 June 2021; Table S1). We updated the trend analysis using “X2,” the estimated distance up the estuary to where daily-averaged near-bottom salinity is 2 (Jassby et al. 1995). X2 is a measure of the physical response of the estuary to freshwater flow and the extent of the estuarine salinity field (Monismith et al. 2002). We analyzed the abundance index using a generalized linear model (function glm, R Core Team 2020) with a log link function and variance proportional to the mean squared, with mean X2 from January to June as a linear predictor and a second-order polynomial in year (function poly in R) to allow for deviation of the year trend from linear. X2 for the hatching period (January–March) was also used to analyze the immediate effect of the position of the salinity field on the distribution of the larvae (below).
Additional background was provided by comparing the distributions of longfin smelt in salinity space and in proximity to the diversion intakes using data collected by all six fish-monitoring programs that cover a large part of the upper estuary (Table S1; Stompe et al. 2020; Tempel et al. 2021; Bashevkin et al. 2022). The intent of this comparison was to determine which of the monitoring programs showed the greatest proportion of fish in fresh water and near the diversion intakes. The diversion operators must meet salinity standards for ecosystem protection and to prevent pumping saline water. Therefore, the proportion of total catch in each program that is taken in fresh water and, in particular, near the diversion intakes should indicate which program shows the highest potential for proportional losses of fish to diversions. To assess this, we determined the catch of longfin smelt per trawl using all data from each monitoring program near the intakes and in three salinity bins, < 0.5 (essentially fresh), 0.5–5, and > 5.
Overview of Abundance Modeling
The population size of larvae was estimated from catch data collected by the Smelt Larva Survey (SLS) designed to collect larval longfin smelt (Mitchell et al. 2019; Tempel et al. 2021). “Population size” here means the estimated total number of larvae in the region sampled by the SLS during a given survey. “Population density” refers to the estimated number of larvae m−3 at each sampling station during each survey. “Adjusted population size” is population size adjusted to account for larvae outside of the spatial extent of the survey, as explained below. An annual mean population size index and proportional diversion losses were calculated as arithmetic means from the surveys in each year; some conceptual difficulties with this practice are discussed below.
The SLS began collecting data on abundance of larval fishes in 2009. Five or six surveys (i.e., single sampling events) were taken at 2-week intervals during January–March. Each survey was taken over 4 days at nominally 32 stations. At each station, a single 10-min oblique tow was taken with a 500-µm or 505-µm mesh net with a mouth area of 0.37 m2, attached to a frame equipped with skis to limit damage if the net hit bottom. The net was deployed while underway and lowered to a target depth by adjusting the amount of towing cable let out to attain an angle of the wire to the vertical of 71°; the target depth compared favorably with measurements using depth sensors (T. Tempel, California Department of Water Resources, pers. comm. 30 August 2021). The estimated sampling depth averaged ~ 2 m greater than the water depth measured by a depth sounder on the vessel, and was greater in 95% of the tows, indicating that the net was usually reaching the bottom and sampling the full water column.
Each sample was preserved in 4% formaldehyde. A flowmeter was used to estimate volume sampled. Data on salinity, temperature, and Secchi depth were also collected at each station, and turbidity was measured beginning in 2010. Larval fish were subsequently identified to species and either all (2009 and 2010) or up to 50 (2011–2020) longfin smelt larvae were measured to the nearest millimeter notochord length.
Population size estimates for each survey were made with a Bayesian hierarchical model (Gelman et al. 2004) in which a process model related the unobserved true population density to salinity and water clarity, and an observational model linked the population density estimated by the process model to the field observations. Then, the proportional losses of longfin smelt larvae to diversions in the Delta were estimated with a method conceptually similar to that used in a study of delta smelt (Kimmerer 2008), in which the flux of larvae to the diversion facilities was estimated as the product of estimated local population density and diversion flow rate.
The year 2017 had the second-highest flow among water years (October 2016–September 2017), and the highest mean flow in January–March, for any year since 1955. Because the estuarine salinity field moves in response to variation in freshwater flow (Jassby et al. 1995; MacWilliams et al. 2015) and larval longfin smelt are generally found at salinity ~ 2 (Dege and Brown 2004; Kimmerer et al. 2013), most of the larvae in 2017 were seaward of the region sampled by the SLS (Lewis et al. 2020; Grimaldo et al. 2021). Although we included these data in most analyses, the results for 2017 were so anomalous that they were excluded from our analyses of interannual trends and the consequences of losses to diversions.
Key assumptions in this analysis were as follows: (1) Sampling locations were representative of the distribution of the population; (2) larvae (mostly 5–10 mm length) are unable to avoid the sampling gear, or any avoidance was similar in the southern Delta to that in the broader region; (3) the distribution of larvae is unimodal in salinity space, with some effect of water clarity; and (4) a negative binomial error distribution is suitable for these data. The degree to which sampling was representative cannot be determined independently. The weakly swimming larvae (Bennett et al. 2002) were almost certainly collected quantitatively, since the net mesh was selected to capture larvae at all sizes, and the 5–10-mm larvae are unlikely to avoid the net. The distributions of most estuarine plankton and fish are unimodal in salinity space (Kimmerer et al. 2013). The negative binomial distribution is commonly used to represent the statistical distribution of planktonic organisms (Taft 1960); it is identical to a Poisson distribution in which the parameter λ increases with the predicted mean.
Data Preparation
The distribution of larvae was analyzed using salinity and Secchi depth as covariates. Like most estuarine organisms, larval longfin smelt are most abundant over a range of salinity and less abundant at higher or lower salinities. Since salinity due to ocean salts decreases with distance up the main channel of the estuary, salinity can be used as a measure of distance along the channel in the reference frame of the fish. Also, like many fishes in the estuary (Latour 2016), larval longfin smelt are more abundant in turbid water than clear. Secchi depth measurements have been taken in every year of the SLS with only one missing value. Turbidity measurements began in 2010 (though with 22 missing values since 2010). Because Secchi depth is more closely aligned than turbidity with the sight distance of a visually oriented organism, and the data were more complete, we used Secchi depth (cm) as a covariate representing water clarity.
Data were available for 2009–2020. Out of 70 surveys conducted during that time, one had samples from only half of the stations and one was missing data from three of the four stations in the southern Delta. Stations in the lower Napa River (Fig. 1) were excluded because they were sampled during only half of the years. Three stations in the San Joaquin River (stations 906, 910, and 912) were excluded because salinity is often elevated by agricultural return flow, i.e., not due to ocean salts, and including these stations distorting the relationships of catch to salinity.
This left a total of 68 surveys (5 each in 4 years, 6 in the remaining years) comprising 2165 samples from 32 stations. Eleven samples were missing, with two stations unsampled twice and seven stations unsampled once, while all other stations were sampled during every survey. Catches ranged from 0 to 1678 fish, with a median of 3 and a mean of 37. Salinity data were missing for three samples and Secchi depth for one sample, and these values were filled in from nearby stations by linear interpolation. All 32 stations were used in the Bayesian analysis to determine the relationships between environment and catch per trawl, but missing catch data were excluded from this analysis.
A subset of 28 stations was selected as representative for estimating population size from catch per trawl predicted by the Bayesian analysis. Stations excluded from this part of the analysis (Fig. 1) were three in Suisun Marsh, whose habitat volume is negligible, and one in a small slough in the eastern Delta far removed from the larger channels, which therefore did not seem representative. This resulted in a complete set of 1904 samples in the 68 surveys of 28 stations.
Volume sampled from the flowmeter measurements had a median and mean of 187 m3 and a range of 6–345 m3, with 10th and 90th percentiles of 153 and 220 m3 respectively. Eighteen samples had volume estimates < 100 m3 and these values were unrelated to tow duration or catch and therefore appear spuriously low. Since most of the volume estimates were within a narrow range, whereas the catch data were wildly variable and highly skewed, we used the median volume sampled of 187 m3 to convert catch to catch per unit volume.
Length data were used to develop length-frequency plots and to estimate the age ranges of larvae vulnerable to the net. Longfin smelt hatch at 5.3– 6.8 mm length (Wang 2007), with a mean based on field sampling of 6.2 mm (Gross et al. in review). We calculated the frequency by length from 5 to 30 mm at 1-mm length intervals. This was converted to age using a growth rate of ~ 0.19 mm day−1, determined on cultured larvae of known age and on wild-caught fish using age determined from otoliths (Gross et al. in review). All larvae with interpolated sizes of 6.2 mm or smaller were assigned age 0; then, 7 mm fish were assigned age 4.2 d, 8 mm fish 9.5 d, and so on.
Process Model
We conducted a preliminary analysis of the SLS data set to determine a reasonable representation of covariates. Since both the salinity distribution and abundance varied among years and among surveys within years, we used survey number (1 to 68) as a blocking variable. Other covariates included Secchi depth (linear) and salinity (unimodal), both from measurements taken with each fish sample. Salinity was log-transformed to spread out the scale where longfin larvae are most abundant and to make the scale closer to linear in geographic distance. The response variable for this preliminary analysis only was the log of (catch per trawl + 1).
We explored various methods for representing a unimodal distribution in salinity space, including generalized additive models (gam in R, R Core Team 2020), locally weighted smoothing (loess in R), and polynomials of order 2 to 5. Although criteria for model selection such as AIC (Akaike 1974) are helpful generally, we had two specific criteria related to our purpose. The first was that the fit should not underestimate abundance at the freshwater end of the larval distribution, and the second was that it should not overestimate abundance near the peak. Most of the above models failed one or both criteria, particularly the first. We selected a quadratic fit as the most parsimonious representation of the abundance pattern, although it did not fit as well overall as gam in this exploratory analysis. A symmetrical quadratic fit to a log-transformed response variable is a Gaussian curve in raw data units.
The final process model selected was
where i is the survey, k is the station, µi,k is the estimated mean catch per trawl at station k for survey i, a through d are the parameters to be estimated for each survey, S is the log of salinity (Practical Salinity Scale), and D is the Secchi depth (cm).
Observation Model
The distribution of catch per trawl about the mean catch per trawl predicted by Eq. 1 was modeled with a negative binomial distribution
where pi,k is the probability parameter for the negative binomial function dnegbin, α is the overdispersion parameter which was the same for all samples and surveys, and Ci,k is the observed catch of longfin smelt for survey i at station k. Overdispersion increases with α > 0, while with α ≈ 0 the negative binomial distribution becomes a Poisson distribution (alternative formulations of the negative binomial use λ or θ = 1/α to represent overdispersion). Preliminary analyses with values of α that varied by survey gave similar means of population size index to those using a single value of α, but with greater uncertainty.
A similar result was obtained using a 4th-order process model and an observation model using a Poisson distribution with parameter λ that was lognormally distributed to allow for overdispersion (Royle and Dorazio 2008). Predictions of the annual estimates of losses to diversions were similar between the two models in most years (Fig. S2), but diagnostic statistics for this model showed evidence of instability and this model is not discussed further.
The observation model might have been improved by using zero inflation to account for an excess of zeros in the catch data (shown below) compared to the model predictions (Wenger and Freeman 2008). However, zero-inflated models are suitable only when the zero inflation arises through a different process from the one that generates the negative binomial component of the model (Royle and Dorazio 2008), which is not the case for these data.
Post hoc Calculations
Station locations were mapped using ggmap. Each station was assigned a region whose volume was used to extrapolate estimates of local density to population size. Polygons around each station were calculated using a tessellation function (deldir in R) that assigns every geographic point to the nearest station. Some of the polygons are unbounded, so they were constrained using tile.list with points defining lines bordering the estuary that were selected on a Google Earth map of the sampling domain (Fig. 1). This resulted in some anomalies where boundaries crossed land, connecting water bodies that would logically be in different polygons (Fig. 1), but the volumes so assigned were negligible and this was ignored for simplicity. The volume of water in each polygon was calculated from a spatial grid used in a recent version of the UnTRIM hydrodynamic model (Gross et al. 2019).
Post hoc calculations of population size and daily diversion loss rate, both by survey and by year, were taken directly from the iterations of the Bayesian model. Population size (number of fish) from each survey was calculated as
where Ai is the population size for survey i, Vk is the volume of water in the polygon around station k, and v is the median (also mean) volume sampled of 187 m3 used for all samples. Then, the mean daily proportional loss of larvae to diversions was calculated under Assumption 1, i.e., that the density of larvae in the diverted water was the mean of that in the four stations near the diversion facilities,
where Li is the daily proportional loss during survey i, Qi is the diversion (export) flow rate on that day (m3 day−1), and n is the number of stations (4) and j the index for each station in the south Delta (Fig. 1). The annual population size index and the mean of the daily proportional loss rates were calculated as the means of the respective values from the surveys in each year. To assess the cumulative effect of these losses on the larval population, we accumulated losses over the mean and 90th percentile of age of the population calculated from length distributions (see the “Discussion” section).
The sampling program did not cover the full salinity range of the larvae, and in many surveys no samples were taken at salinity above ~ 10. In surveys that covered salinities up to 15, the decline in abundance at high salinity became obvious (examples below). Therefore, in those surveys lacking data at high salinity, the population size (Ai in Eq. 4) was underestimated and the impact of diversion was overestimated. To provide a rough estimate of these biases, we estimated the proportion of the population missed by the sampling program. The key assumption was that the distribution of habitat volume by salinity range does not vary much with X2 values above ~ 55 km (Figs. 3 and 5 in Kimmerer et al. 2013). We used the parameters in Eq. 1 calculated for each of 14 surveys with maximum salinity > 15 (which occurred in 7 years spanning 2009–2020). Predicted catch was calculated for these surveys in 21 bins of salinity (0 to 1, 1 to 2, etc.), with Secchi depth set to its median of 51 cm. From these, we calculated the cumulative proportion of the catch in each salinity bin and averaged those proportions over the 14 surveys. The maximum observed salinity in each of the 68 surveys was then used with the cumulative mean proportions by salinity to estimate the proportion of the population that was missed in the survey. This proportion was then used to estimate the fraction by which the estimated population size should be increased and the fractional loss to diversions decreased. Because these calculations were crude and post hoc, we first present results below focusing on the observed data and then discuss the proportional losses both as calculated and after this adjustment.
Model Fitting
The model was run in JAGS v. 4.3.0 (Plummer 2017) from R (R Core Team 2020) using the function jags in package jagsUI. Prior distributions (priors) for a, b, c, and d (Eq. 1) were normal with means of 0 and standard deviations of 10, and therefore uninformative except that b was constrained to be positive and c and d were constrained to be negative. This reflects our intent that the salinity functions should be concave downward since the larvae are most abundant at intermediate salinity and uncommon in fresh or highly saline water, and that larvae should be rarer in clear water than turbid. The single value of α was given a uniform prior U (0.01, 10). Examination of extreme values of the output showed that effects of these priors on the posterior distributions of the parameters were negligible.
Run parameters included three Markov chains with a burn-in of 1000 iterations to minimize the effect of (randomly selected) initial values, tenfold thinning, and 5000 iterations (samples from the posterior distribution) after thinning. The algorithm was verified by running it with simulated data and comparing the computed mean and distribution of the simulated data with values from the Bayesian model.
Standard post hoc diagnostic tests were conducted. The Gelman-Rubin statistic rhat (Gelman et al. 2004) for annual population size index had a maximum value of 1.015 and that for annual proportional loss to diversion had a maximum value of 1.001, both indicating convergence. Autocorrelation plots (not shown) indicated that the number of iterations was sufficient. An additional check ensured that parameter estimates were similar between the first and last 10% of the series of iterations after burn-in. Finally, we repeated the run with the standard deviations of the priors for parameters in Eq. 1 set to 30 instead of 10, and increased run time to a burn-in of 2000 iterations and 10,000 iterations retained after thinning. This run produced similar values to the original run, and values for the annual mean population size index and mean annual proportional loss were within 3% of those in the original run, but diagnostic statistics indicated poorer convergence for some of the surveys.
A comparison of predicted and observed catch per trawl was made graphically and by summary statistics (see the “Results” section). To provide an order-of-magnitude check of the calculations through the entire analysis, the mean catch per trawl from all surveys was used with the total habitat volume to calculate an expected mean population size across all surveys, which was 68% of the overall mean from the Bayesian analysis.
Results
The autumn abundance index for juvenile longfin smelt continued downward with shorter-term variation largely related to spring X2 (Fig. 2A). The model explained 81% of the deviance in the index, and partial residuals show that the two components had approximately the same influence on the index (Fig. 2B, C). Partial residuals for both year and X2 had ranges near log(100), meaning that the index varied by 100-fold over its 54-year span and over the 43-km range of X2. The slight downward curvature in the partial residual for year suggests an accelerating decline.
Relationship of annual fall abundance index of juvenile longfin smelt to year and X2 averaged over the preceding January–June. A Abundance index (points) with line fitted to the index with a generalized linear model in X2 and a quadratic function for year; the shaded region shows 95% the confidence interval. B and C Partial residuals (natural-log scale) from the fit in A for year and X2 respectively. The model (function glm in R) was glm (index ~ X2 + poly(year, 2), family = quasi (link = log, variance = mean2)). The fit was index = 15.4 − (0.13 ± 0.02)X2 − (11.7 ± 1.7)P1 − (2.5 ± 1.7)P2, parameters with 95% confidence intervals, 48 degrees of freedom, where P1 and P2 are the terms of an orthogonal transformation for a quadratic function of year (function poly). This model explained 81% of the deviance in the abundance index
Boxplots of catches from six monitoring programs compare the likely vulnerability of early larvae to diversions with that of other life stages (Fig. 3, Table S1). Mean catch per trawl was highest in the 0.5–5 salinity range for all sampling programs. Among sampling programs, mean catch near the diversion points was highest for early larvae (Fig. 3A), near zero for late larvae (Fig. 3B), and zero for juveniles and adults (Fig. 3C-E). Mean and median catches at salinity < 0.5, excluding stations near the diversion points, were higher for early larvae than for any other life stage, and only early larvae had a median and an upper quartile > 0 in this salinity range.
Boxplots showing catch per trawl of longfin smelt for each of six sampling programs in the upper estuary (panels A–F; see Table S1). The four boxes in each panel show differences among four regions: the south Delta near the diversion intakes (“SDel”), and three regions defined by salinity ranges but excluding the south Delta. Boxes show quartiles, whiskers extend to the furthest point within 1.5 times the interquartile range from the boxes, and points are outliers. Circles give means, and numbers at the top of each panel give the percent of each mean to the highest mean in the panel, rounded to one decimal place if < 0.5. The south Delta was not sampled by the San Francisco Bay Study (F). Data are from all years when the program operated; confining the data to the years when the Smelt Larva Survey was operating, 2009–2020, gave essentially the same result
Environmental conditions during each study year show substantial variation in Delta outflow and corresponding shifts in X2 during the larval period (Table 1). Salinity ranges covered by the SLS always included fresh water but had maxima that varied with X2. Results of the Bayesian analysis (also summarized in Table 1) are discussed below.
The larvae collected by the Smelt Larva Survey were small, with a mode at 7 mm and medians of 7–8 mm. The < 0.5 salinity range had a greater proportion of 6 mm fish than the other two salinity ranges (Fig. 4). About 99% of all fish measured were between 5 and 13 mm and 95% were between 5 and 10 mm. Using the assumed growth rate of 0.19 mm day−1, about 26% of the fish were age 0 days, the mean age was 6.8 days, and the 50th, 75th, and 90th percentiles of age were 3, 7, and 13 days respectively.
The variance:mean ratio of a Poisson distribution is 1, and overdispersion causes that ratio to increase above 1 in a negative binomial distribution at a rate that itself increases with the mean and also with the α parameter. The Bayesian analysis gave a mean for the single value of α of 1.43 (95% credible interval ± 0.11, median 1.43). At the median predicted catch per trawl of 8 fish, the median variance:mean ratio was 7, while at the mean predicted catch per trawl of 50 fish the median variance:mean ratio was 24.
Predicted and observed catch per trawl for all data had a correlation coefficient of 0.6, but scatter was wide and related to predicted mean, as expected for an overdispersed distribution (Fig. S3A, B). About 32% of the observed catches were zero, while 16% of the predicted catches were 0 when rounded to the nearest whole number, and 81% of the samples were on the principal diagonal of the presence/absence matrix (Table S2). Residuals from the analysis, determined separately for three salinity ranges, had interquartile ranges that included zero and a wide scatter of outliers, as expected from the overdispersion of the catches (Fig. S3C).
Example plots of observed and predicted catch per trawl show how patterns varied depending on the range of salinity covered by the surveys (Fig. S4). The underlying response to salinity was quadratic and therefore smooth, so the jagged appearance of the lines is due to variation in Secchi depth. The model predictions agree broadly with the observed catches, with the highest values generally occurring at salinity between 0.5 and 5 (Fig. S4B, C, E, F). However, many surveys did not cover the high-salinity end of the range of larval longfin smelt (e.g., Fig. S4A, D). Below we discuss consequences of the resulting underestimate of population size, particularly for samples taken during high-flow periods such as in 2017.
As a check on whether the modeled population estimate was reasonable, we calculated the population size for each survey by simply multiplying the observed catch m−3 by the volume assigned to each station and summing the result across stations. The mean of the annual means calculated from data from surveys without missing data (60 out of 68 surveys) was 0.41 × 109 compared with 0.49 × 109 from the model, and all of the individual annual means so calculated were within the 95% credible intervals of the model-generated results.
The annual population size index of larvae showed a large decline from 2013 to 2015 with an intermediate value in 2014 and an anomalously low value in 2017 (Fig. 5). The population size index in the earlier period was ~ 109 fish, while that in the latter period excluding 2017 was ~ 108 fish. Variability was high among surveys within years, as the first and last surveys caught fewer fish than the other surveys (in a few cases, the sixth survey was dropped when the fifth produced few longfin smelt).
Population size estimates by survey and indices by year. Symbols give population size estimates by survey with 95% credible intervals. Boxes give medians (cross-bar) and quartiles (edges of boxes) of annual population size indices by year. Colors and shapes distinguish adjacent years but have no other meaning. Asterisks indicate that results for 2017 were unreliable because the surveys covered so little of the habitat of larval longfin smelt
Adjusting the population size estimates for each survey for the incomplete coverage of the salinity range gave increases that scaled with the position of the salinity field and therefore the maximum salinity during each survey, and had variable effects on the annual population size index (Table 1). As expected, these adjustments were most extreme in wet years such as 2017 and 2019.
The annual population size index of larvae, whether adjusted as above or not, was positively related to the subsequent fall index of juvenile abundance (Fig. 6, 2017 not included). The adjusted indices gave a somewhat better fit than the raw indices (AIC of 30 and 34 respectively). The larval population size index was unrelated to flow conditions as indexed by the mean X2 value for January to March of each year (Fig. 7). The slope of the population size index vs. X2 was within 1 standard error of 0 in linear models with and without adjustment for incomplete coverage of the salinity range and with and without the outlier year 2017. Adding a linear effect for year, or a step function for year occurring after 2013 (Fig. 5), did not improve the fit.
Population size indices of larval longfin smelt vs. subsequent value of the Fall Midwater Trawl Index by year with 2017 excluded. A Raw population size index; y = − 0.66 + 0.80 ± 0.50x (95% CI), R2 = 0.56 for log–log regression. B Population size index adjusted for incomplete sampling; y = − 1.42 + 0.86 ± 0.40x (95% CI), R2 = 0.69 for log–log regression. Numbers indicate years. Data for 2017 were as follows: abundance index 141, larval population in A, 2 × 106; B, 26 × 10.6
Population size index of larval longfin smelt vs. mean X2 for January–March with 2017 omitted; y = 9 − 0.04 ± 0.16 x (95% CI), R2 = 0.03 for log-linear regression. Numbers indicate years as in Fig. 6. The data point for 2017 is at X2 = 48.5 km, population = 25 × 10.6
Losses of larval longfin smelt to diversions were highly variable with large error bars around some of the survey-specific values (Fig. 8), especially for 2017. Annual mean values of the daily losses had an overall mean of 0.19% day−1 and a range of 0.05–0.23% (Table 1). However, adjusting values for the proportion of the habitat not sampled reduced some of the values, so that the adjusted mean was 0.12% day−1. After this adjustment, the percent daily loss by survey was above 0.2% day−1 only when X2 > ~ 70 km, when the maximum salinity values on each survey were often > 15 resulting in maximum precision in the population size estimate (Table 1, Fig. 9).
As in Fig. 5 for daily loss of fish to diversions as a percent of the estimated population size, not adjusted for incomplete sampling. Asterisks indicate that results for 2017 were unreliable because the surveys covered so little of the habitat of larval longfin smelt
A Adjusted percent daily loss by survey of the larval longfin smelt population to diversions, and B maximum salinity by survey, as a function of X2. Percent daily loss in A has been adjusted to account for incomplete sampling as indicated by the maximum salinity in B. Symbol shapes and colors as in Fig. 8
Discussion
This paper contributes to the growing body of literature on the effects of freshwater flow and flow diversions on populations of estuarine organisms (Livingston et al. 1997; Montagna et al. 2002; Kimmel and Roman 2004). These effects are of scientific interest for understanding the factors driving estuarine populations, and of management interest for developing ways to minimize harmful human impacts. Our results show that the strong relationship of the fall index of abundance to flow (as X2) continues to hold, although the temporal decline includes a worrisome acceleration (Fig. 2). This decline in the fall index is mirrored in the abundance of longfin smelt during the early larval stage (January–March) which declined over the duration of this study, between 2009 and 2020. However, larval abundance is unrelated to freshwater outflow during January–March, and losses of larvae to diversions appear far too low to contribute measurably to the population response to flow, as discussed below.
Evaluation
The selection of a negative binomial observation model was somewhat arbitrary, although this model has a long history of use in analyzing distributions of organisms (Taft 1960; Jahn and Smith 1987; Drexler and Ainsworth 2013). This use is consistent with the schooling behavior of many estuarine fish populations even as larvae, which causes catches to be overdispersed. For example, the catches in the Smelt Larva Survey had a mean of 37 fish and a maximum of 1678 with 34% of the values being zero; a Poisson distribution with the same mean would have 1st and 99th percentiles of 24 and 52, respectively. Several alternatives to the negative binomial were rejected as either inappropriate for overdispersed data (Poisson) or difficult to fit (zero-inflated models as discussed above). An alternative model using a Poisson model with a lognormally distributed prior for the single parameter λ and a process model that was fourth-order polynomial in salinity gave results that were similar to those of the model described in Eqs. 1–3 (Fig. S2), but convergence was poor in some cases.
Of the 2165 samples in the survey data, 1472 had at least one longfin smelt (Table S2) and 1810 had a predicted catch per trawl of at least 1 after rounding to whole numbers. Graphical and tabular analyses revealed that predictions of positive catch when actual catch was zero were more frequent in later years than in early years. This may be an artifact of fitting a model with a single value of the overdispersion parameter α to data spanning a tenfold decline. Since the other diagnostics of the fit were satisfactory and varying α degraded the fit, we used the results with constant α. There was no evidence that catch per trawl at low salinity was underpredicted on average (Fig. S3C), which would result in an underestimate of the proportion of the population lost to diversions.
Like other pelagic estuarine organisms, longfin smelt larvae are most abundant across a range of salinity, and are not strongly linked to geographic position (Grimaldo et al. 2017, 2021). However, many surveys did not fully cover the salinity range where larvae are likely to occur. The maximum salinity in any one survey ranged from 0.2 to 21, but the seaward limit of the population was reasonably well defined only when the salinity ranged at least up to 15 (e.g., contrast Fig. S4A and D with C and F). The adjustment for incomplete sampling was inversely related to the maximum salinity during each survey, and for some of the surveys in the very wet 2017 the estimates of population size were highly uncertain (Fig. S4D). The basis for the adjustment was that the abundance at salinity higher than sampled could be extrapolated from the fit of the model to the available data (e.g., Fig. S4), assuming that the volume of habitat in each salinity range did not depend strongly on where that salinity range was. This assumption was supported by a finding that the volume of oligohaline habitat did not change much as X2 moved between 90 and 55 km (Figs. 3 and 5 in Kimmerer et al. 2013), which encompassed all of the X2 values during these surveys except on four dates in 2017 and one in 2019.
In some circumstances, different processes may govern presence or abundance of a species and probability of detection (e.g., McGowan et al. 2013). For example, turbidity might affect the probability of observation of a pelagic fish more than it does the underlying distribution of fish. However, it is more likely that turbidity is a fundamental habitat attribute that determines where the fish are (Utne-Palm 2002; DeRobertis et al. 2000 Aksnes et al. 2004), whatever the underlying mechanism. In the SFE, the frequency of occurrence of delta smelt in net samples and in samples taken at the entrance to the diversion facilities were similarly affected by turbidity suggesting that, instead of being harder to catch in clear water than turbid, the fish were simply absent from clear water (Grimaldo et al. 2009). This observation led resource managers to limit diversion rates during times of high turbidity to reduce mortality to this endangered species, which is more vulnerable than longfin smelt to diversion losses because of its distribution in lower-salinity water (Kimmerer 2008, 2011; Kimmerer et al. 2013).
Abundance–Flow Relationships
A variety of mechanisms have been shown or proposed to underlie relationships between freshwater flow and the abundance or distribution of estuarine species (e.g., Drinkwater and Frank 1994). These can generally be divided into mechanisms involving correlations of loading with flow (e.g., nutrients in the “agricultural model,” Nixon et al. 1986; Day et al. 1994; Vörösmarty et al. 2003), and those involving the physical response of estuarine habitats to changes in flow. Physical responses to changing flow may include floodplain inundation (Sommer et al. 2001, 2020), decreased residence time (Livingston et al. 1997), compression of the longitudinal salinity field (Monismith et al. 2002) or its extension into the coastal ocean (Hickey and Banas 2003), and increased stratification with attendant intensification of two-layer net circulation. In some estuaries, entrainment of organisms into large water intakes can be a source of concern over mortality, and this entrainment may be inversely related to ambient freshwater flow. How these play out depends on the dynamic ranges of flow and tides and the details of bathymetry and extent of the estuary (Monismith et al. 2002).
Longfin smelt has the strongest known relationship to freshwater flow of any pelagic fish or invertebrate in the SFE (Jassby et al. 1995; Fig. 2; Kimmerer 2002; Fig. 6). Most of the mechanisms suggested to explain this relationship have emphasized physical dynamics rather than the agricultural model (Kimmerer 2002; Kimmerer et al. 2013). Losses to diversions are likely to be a minor contributor to the flow relationship of longfin smelt, as discussed below.
Pelagic estuarine organisms are generally capable of behaviors that are flexible or adaptable enough to accommodate the effects of tidal fluctuations, changing freshwater flow, and spatial variation in water depth. For example, zooplankton and fish can maintain position in estuaries through a variety of behaviors that respond to the flow field (Greer Walker et al. 1978; Forward et al. 1999; Kimmerer et al. 2014), and young salmon lacking previous experience of tides quickly learn which way is flood and ebb when they enter estuaries (Lacroix and McCurdy 1996). Longfin smelt may undergo tidal migration and maintain a position near the bottom to avoid being swept to sea (Bennett et al. 2002), but the period in the life cycle where this happens is uncertain. This timing may be critical for ensuring a good year class, especially during high-flow years.
The length distributions of larval longfin smelt (Fig. 4) show a sharp decline at larger sizes. This decline could be due to avoidance of the net by larger larvae, mortality, or departure of the larvae from the region sampled by the larval nets. Net avoidance is unlikely for these larvae. Probability of capture for delta smelt larvae collected using the same net was high for larvae < 20 mm, though confidence intervals were large (Mitchell et al. 2019). The SLS net captures numerous Pacific herring larvae with a median length of 11 mm (data not shown). We calculated an apparent mortality rate from the rate of decline in size for each year using growth rate of 0.19 mm day−1 (Gross et al. in review) and found a median of 15% day−1. This seems too high to be the actual mortality rate, as it would result in only 0.04% of larvae reaching 16 mm; a more refined analysis of data from 2013 that combined particle-tracking and Bayesian models calculated a mortality rate of 2.4% day−1 (Gross et al., in review). This contrast implies that the larvae were progressively less available to the SLS sampling gear as they grew.
The larger larvae could have been unavailable to the nets by being out of the sampled area, either in depth, laterally, or along the channels. The available information on sample depth indicates that the entire water column was fished for many if not most samples. Longfin smelt larvae appear to be largely surface-oriented up to about 10–12 mm length (Bennett et al. 2002), so it is unlikely that larger larvae were abundant at the sampling stations but missed. Catch per volume in 2016 and 2017 was similar between shoals and channels, ruling out lateral avoidance of the sampled area (Grimaldo et al. 2017). In a particle-tracking study, most of the passive particles released in inferred hatching regions drifted far seaward of the sampled region in the time it would take for larvae to reach approximately 10–12 mm (Gross et al. in review). Longfin smelt larger than approximately 10–12 mm length begin to disperse vertically and possibly to migrate tidally (Bennett et al. 2002), which can result in retention (Kimmerer et al. 2014). We speculate that the lack of the larger larvae in the SLS samples was a result of seaward drift of early larvae followed by an ontogenetic shift from passive behavior to bottom-oriented or tidally migrating behavior.
Each individual survey is a sample of a limited temporal and size range in a growing population. With a sampling interval of ~ 2 weeks, about 92% of the larvae that were in the population on one sampling day would be gone by the next, through mortality and seaward movement out of the range of the survey. This means that each survey sampled a largely different population of larvae hatched over a different period (Gross et al. in review). This is why we refer to the annual mean as a population size index rather than an estimate. A more informative measure of the annual population may be the number of larvae that passed through the size of vulnerability to the nets during the entire season, which is an estimate of annual production of larvae at that size range. Dividing the mean adjusted population size index by the mean age of the larvae (6.8 days) and multiplying by the duration of the sampling program (median 70 days) give ~ 19 × 109 larvae over the first 5 years of the survey and ~ 1.5 × 109 over the last 7 years. Gross et al. (in review) obtained a population estimate of 12.6 × 109 total fish hatched during 2013. Using the unadjusted value for consistency with Gross et al. (in review), our population index for 2013 only was (18 ± 9) × 109 fish passing through age 6.8 days. After correcting for 6.8 days’ mortality using estimates from Gross et al. (in review), we estimate that (21 ± 11) × 109 fish hatched in 2013, not very divergent from their value of 12.6 × 109 given the difference in approaches (though using the same data).
Stevens et al. (1983) first identified the positive relationship between the fall abundance index of longfin smelt and freshwater flow in the SFE. The authors speculated that this relationship was due to dispersal of larvae by high flows resulting in an expanded habitat and range and therefore reduced density-dependent mortality. Jassby et al. (1995) formalized flow-abundance relationships for several species including longfin smelt, using X2 in spring as an index of freshwater flow. Kimmerer (2002) and Kimmerer et al. (2009) updated these relationships and showed how the abundance index of longfin smelt had declined markedly in relation to the original relationship, though the index was still strongly related to X2. Thomson et al. (2010) developed a statistical model of the long-term pattern of the abundance index; in addition to the strong relationship with X2, two declines were detected that were not explained by flow or other covariates, one around 1989 and the other around 2004. The first decline was likely related to decreased availability of their zooplankton food following the introduction of the “overbite” clam Potamocorbula amurensis (Kimmerer 2002; Feyrer et al. 2003; Mac Nally et al. 2010). The cause of the second decline remains unknown.
Regulations governing freshwater outflow in the SFE have a long history, but regulations specifically for protecting populations of estuarine fish were first established in 2000. These regulations apply from January through June, based on the relationships of several fish and shrimp species to X2 and their life histories (Jassby et al. 1995). The underlying assumption behind the selection of that time period was to protect longfin smelt during the entire period from hatching to the early juvenile stage. Since X2 is strongly autocorrelated across months, the time period when the relationship of the autumn index to X2 comes into effect cannot be determined through statistical analysis, but must instead be inferred from other surveys and other sources of information. Our results show that larval abundance is unrelated to X2, though it is correlated with the autumn index. Therefore, the mechanism for the strong relationship of the index to X2 must arise after early larval development, i.e., after March, rather than during spawning, hatching, or early larval development and movement.
How much do diversion losses contribute to the flow relationship of longfin smelt abundance index? The values determined above are estimated daily proportional losses to the population collected by the SLS net, but larvae in the Delta may be exposed to risk of loss to diversion over more than a day. Since the denominator of this calculation (Eq. 5) is the population size estimated from the SLS, a suitable time frame for accumulating losses is the duration of vulnerability of the larvae to the nets. We used alternatively the mean (6.8 days) and 90th percentile of age of the larvae (13 days); the latter is conservative in overestimating the time of vulnerability and therefore the annual loss rate. The estimated mean annual loss rate accumulated over 6.8 days was 0.8%, and that at 13 days was 1.5% (Table 1). These values can be compared to the range of interannual variability in the autumn abundance index of ~ 100-fold (Fig. 2B). Clearly in this context, the effect of diversion losses is small, and its contribution to the longfin smelt’s flow-abundance relationship is negligible. As discussed above, proportional losses to diversions are likely lower for other life stages than for early larvae, so losses of these stages to diversions are likely even a smaller contributor to interannual variability or the X2 relationship than that for larvae.
Regulations limiting diversion flows were established in ~ 2009, so the entire period of this study took place under more benign conditions than previously existed. The difference in diversion flows between these periods was greatest when inflow was lowest, and the sign of the difference reversed above inflow of about 2400 m3 s−1 (Fig. S1). When inflow (and therefore outflow) is low, the larvae are further landward and therefore more vulnerable to entrainment in the diverted water; therefore, the measures limiting diversion flows were effective in reducing these losses by about half under worst-case conditions. Regardless of the legal requirements to minimize harm to listed populations of fish, even this higher level of loss would have been insufficient to materially affect the population’s response to flow.
Previous studies have examined consequences of losses of estuarine populations to diversions in the SFE, arriving at contrasting conclusions that depend mainly on the vulnerability of the particular species. Diversion flows remove about 2% day−1 of passively transported plankton from the freshwater reaches of the Delta, which is equivalent to about 18% day−1 of phytoplankton production, but this had no statistically detectable effect on biomass trends (Jassby et al. 2002). Much of the work on fish has focused on salmon, mainly on the vulnerability of Chinook salmon Onchorhynchus tschawytsha to poor habitat and diversion losses during migration and residence of juveniles in tidal freshwaters of the Delta (e.g., Buchanan et al. 2013; Zeug and Cavallo 2013; Perry et al. 2018). However, the actual losses to diversions and their consequences have not been determined with sufficient rigor to be reliable (Jahn and Kier 2020). Abundance of Sacramento splittail Pogonichthys macrolepidotus varies strongly with interannual fluctuations in freshwater flow, but the population is maintained by high production of young during years when floodplains are inundated by uncontrollably high flows, and diversion losses cannot contribute much to this variability (Sommer et al. 1997). High loss rates of the larvae of striped bass (Stevens et al. 1985) were found to be offset by strong density dependence between the larval and juvenile stages (Kimmerer et al. 2000). By contrast, estimated losses of delta smelt during winter–spring were large in some years and likely contributed to their decline in abundance (Kimmerer 2008, 2011; Miller 2011; Korman et al. 2021). Our finding that the proportional losses of longfin smelt are negligible adds to the understanding of this controversial source of mortality, but will probably do little to still the controversy.
Management Implications
Management of the San Francisco Estuary is balkanized between communities that focus on San Francisco Bay (e.g., https://bcdc.ca.gov/) and those that focus on the upper estuary, especially the California Delta (Lacan and Resh 2017). Recent grant solicitations have even spelled out a requirement for focus of research within the bounds of the Delta and its tributaries. This is both a partial cause and a result of the management and political focus on the impacts of diversions from the southern Delta.
Longfin smelt, no respecters of geographic boundaries, show why management focus on the Delta is misguided. The SLS program, though designed to sample for longfin smelt larvae, fails to cover their range of abundance in moderate to high-flow years (Fig. S4, Table 1; Grimaldo et al 2021). Only one of the four programs designed to sample juvenile fish in the estuary covers the entire in-estuary range of the fish, and no program samples them during residence in the coastal ocean. Moreover, little monitoring for longfin smelt occurs in shallow habitats where they can be abundant (Grimaldo et al. 2017, 2021; Lewis et al. 2020). Their zooplankton prey are intensively monitored in the Delta and Suisun Bay (5297 and 2291 samples respectively during 2009–2020), less so in San Pablo Bay (1140 samples) and not at all in Central or South San Francisco Bays. None of these programs samples at night, when vertical distributions of most organisms change. It is difficult to provide actionable advice to managers based on such a distorted sampling regime. This shortfall is finally being acknowledged (Anonymous 2020), but it will take some years before expanded monitoring can begin to fill in the missing pieces.
Finally, both this paper and Gross et al. (in review), which used the same data but very different methods, showed the cumulative proportional losses of longfin smelt to diversions to be small in comparison to the 100-fold dynamic range of the population index. This finding indicates that attempts to reverse the decline of this species through manipulation of diversion flows are unlikely to bear fruit.
References
Akaike, H. 1974. A new look at the statistical model identification. IEEE Transactions on Automatic Control 19: 716–723.
Aksnes, D.L., J. Nejstgaard, E. Saedberg, and T. Sornes. 2004. Optical control of fish and zooplankton populations. Limnology and Oceanography 49: 233–238.
Anonymous. 2020. Longfin smelt science plan. Sacramento, CA.
Bashevkin, S.M., J.W. Gaeta, T.X. Nguyen, L. Mitchell, and S. Khanna. 2022. Fish abundance in the San Francisco Estuary (1959-2021), an integration of 9 monitoring surveys. ver 1. Environmental Data Initiative. https://doi.org/10.6073/pasta/0cdf7e5e954be1798ab9bf4f23816e83. Accessed 5 Apr 2022.
Bennett, W.A., W.J. Kimmerer, and J.R. Burau. 2002. Plasticity in vertical migration by native and exotic estuarine fishes in a dynamic low-salinity zone. Limnology and Oceanography 47: 1496–1507.
Breitburg, D., L.A. Levin, A. Oschlies, M. Gregoire, F.P. Chavez, D.J. Conley, V. Garcon, D. Gilbert, D. Gutierrez, K. Isensee, G.S. Jacinto, K.E. Limburg, I. Montes, S.W.A. Naqvi, G.C. Pitcher, N.N. Rabalais, M.R. Roman, K.A. Rose, B.A. Seibel, M. Telszewski, M. Yasuhara, and J. Zhang. 2018. Declining oxygen in the global ocean and coastal waters. Science 359.
Brown, R., S. Greene, P. Coulston, and S. Barrow. 1996. An evaluation of the effectiveness of fish salvage operations at the intake to the California Aqueduct, 1979–1993. In San Francisco Bay: The ecosystem, ed. J.T. Hollibaugh, 497–518. San Francisco: AAAS.
Buchanan, R.A., J.R. Skalski, P.L. Brandes, and A. Fuller. 2013. Route use and survival of juvenile Chinook salmon through the San Joaquin River Delta. North American Journal of Fisheries Management 33: 216–229.
California Department of Fish and Wildlife (CDFW). 2009. Longfin smelt incidental take permit no. 2081-2009-001-03. https://www.dfg.ca.gov/delta/data/longfinsmelt/documents/ITP-Longfin-1a.pdf. Accessed 6 April 2022.
California Natural Resources Agency (CNRA). 2021. Dayflow. https://data.cnra.ca.gov/dataset/dayflow. Accessed 12 April 2022.
Chigbu, P. 2000. Population biology of longfin smelt and aspects of the ecology of other major planktivorous fishes in Lake Washington. Journal of Freshwater Ecology 15: 543–557.
Day, J.W., Jr., C.J. Madden, R.R. Twilley, R.F. Shaw, B.A. McKee, M.J. Dagg, D.L. Childers, R.C. Raynie, and L.J. Rouse. 1994. The influence of Atchafalaya River discharge on Fourleague Bay, Louisiana (USA). In Changes in fluxes in estuaries: implications from science to management, ed. K.R. Dyer and R.J. Orth, 151–160. Fredensborg, Denmark: Olsen & Olsen.
Dege, M., and L.R. Brown. 2004. Effect of outflow on spring and summertime distribution and abundance of larval and juvenile fishes in the upper San Francisco Estuary. In Early life history of fishes in the San Francisco Estuary and Watershed, ed. F. Feyrer, L.R. Brown, R.L. Brown, and J.J. Orsi, 49–65. Bethesda MD: American Fisheries Society.
DeRobertis, A., J.S. Jaffe, and M.D. Ohman. 2000. Size-dependent visual predation risk and the timing of vertical migration in zooplankton. Limnology and Oceanography 45: 1838–1844.
Drexler, M., and C.H. Ainsworth. 2013. Generalized additive models used to predict species abundance in the Gulf of Mexico: An ecosystem modeling tool. PLoS ONE 8: 1–7.
Drinkwater, K.F., and K.T. Frank. 1994. Effects of river regulation and diversion on marine fish and invertebrates. Aquatic Conservation: Marine and Freshwater Ecosystems 4: 135–151.
Feyrer, F., B. Herbold, S.A. Matern, and P.B. Moyle. 2003. Dietary shifts in a stressed fish assemblage: Consequences of a bivalve invasion in the San Francisco Estuary. Environmental Biology of Fishes 67: 277–288.
Forward, R.B., Jr., K.A. Reinsel, D.S. Peters, R.A. Tankersley, J.H. Churchill, L.B. Crowder, W.F. Hettler, S.M. Warlen, and M.D. Green. 1999. Transport of fish larvae through a tidal inlet. Fisheries Oceanography 8: 153–172.
Gelman, A., J.B. Carlin, H.S. Stern, and D.B. Rubin. 2004. Bayesian data analysis. Boca Raton, FL: CRC Press.
Greer Walker, M., F.R. Harden Jones, and G.P. Arnold. 1978. The movements of plaice Pleuronectes platessa L. tracked in the open sea. Journal Du Conseil International Pour L’exploration De La Mer 38: 58–86.
Grimaldo, L., J. Burns, R.E. Miller, A. Kalmbach, A. Smith, J. Hassrick, and C. Brennan. 2021. Forage fish larvae distribution and habitat use during contrasting years of low and high freshwater flow in the San Francisco Estuary. San Francisco Estuary and Watershed Science 18. https://doi.org/10.15447/sfews.2020v18iss3art5.
Grimaldo, L., F. Feyrer, J. Burns, and D. Maniscalco. 2017. Sampling uncharted waters: Examining rearing habitat of larval longfin smelt (Spirinchus thaleichthys) in the upper San Francisco Estuary. Estuaries and Coasts 40: 1771–1784.
Grimaldo, L.F., T. Sommer, N.V. Ark, G. Jones, E. Holland, P.B. Moyle, B. Herbold, and P. Smith. 2009. Factors affecting fish entrainment into massive water diversions in a tidal freshwater estuary: Can fish losses be managed? North American Journal of Fisheries Management 29: 1253–1270.
Gross, E., S. Andrews, B. Bergamaschi, B. Downing, R. Holleman, S. Burdick, and J. Durand. 2019. The use of stable isotope-based water age to evaluate a hydrodynamic model. Water 11: 2207.
Gross, E., W. Kimmerer, J. Korman, L. Lewis, S. Burdick, L. Grimaldo. In review. Hatching distribution, abundance, and losses to diversions of longfin smelt inferred using hydrodynamic and particle-tracking models. Marine Ecology Progress Series.
Hanak, E., J. Lund, A. Dinar, B. Gray, R. Howitt, J. Mount, P. Moyle, and B. Thompson. 2008. Managing California’s water: From conflict to reconciliation. San Francisco: Public Policy Institute of California.
Hickey, B.M., and N.S. Banas. 2003. Oceanography of the US Pacific Northwest Coastal Ocean and estuaries with application to coastal ecology. Estuaries 26: 1010–1031.
Hobbs, J.A., L.S. Lewis, N. Ikemiyagi, T. Sommer, and R.D. Baxter. 2010. The use of otolith strontium isotopes (Sr-87/Sr-86) to identify nursery habitat for a threatened estuarine fish. Environmental Biology of Fishes 89: 557–569.
Jahn, A., and W. Kier. 2020. Reconsidering the estimation of salmon mortality caused by the State and Federal water export facilities in the Sacramento-San Joaquin Delta, San Francisco Estuary. San Francisco Estuary and Watershed Science 18. https://doi.org/10.15447/sfews.2020v18iss3art3.
Jahn, A.E., and P.E. Smith. 1987. Effects of sample size and contagion on estimating fish egg abundance. California Cooperative Oceanic Fisheries Investigations Reports 28: 171–177.
Jassby, A.D., J.E. Cloern, and B.E. Cole. 2002. Annual primary production: Patterns and mechanisms of change in a nutrient-rich tidal estuary. Limnology and Oceanography 47: 698–712.
Jassby, A.D., W.J. Kimmerer, S.G. Monismith, C. Armor, J.E. Cloern, T.M. Powell, J.R. Schubel, and T.J. Vendlinski. 1995. Isohaline position as a habitat indicator for estuarine populations. Ecological Applications 5: 272–289.
Kimmel, D.G., and M.R. Roman. 2004. Long-term trends in mesozooplankton abundance in Chesapeake Bay, USA: Influence of freshwater input. Marine Ecology Progress Series 267: 71–83.
Kimmerer, W.J. 2002. Effects of freshwater flow on abundance of estuarine organisms: Physical effects or trophic linkages? Marine Ecology Progress Series 243: 39–55.
Kimmerer, W.J. 2008. Losses of Sacramento River Chinook salmon and delta smelt to entrainment in water diversions in the Sacramento-San Joaquin Delta. San Francisco Estuary and Watershed Science 6. https://doi.org/10.15447/sfews.2008v6iss2art2.
Kimmerer, W.J. 2011. Modeling delta smelt losses at the south Delta export facilities. San Francisco Estuary and Watershed Science 9. https://doi.org/10.15447/sfews.2011v9iss1art3.
Kimmerer, W.J., J.H. Cowan Jr., L.W. Miller, and K.A. Rose. 2000. Analysis of an estuarine striped bass population: Influence of density-dependent mortality between metamorphosis and recruitment. Canadian Journal of Fisheries and Aquatic Sciences 57: 478–486.
Kimmerer, W.J., J.H. Cowan, L.W. Miller, and K.A. Rose. 2001. Analysis of an estuarine striped bass population: Effects of environmental conditions during early life. Estuaries 24: 556–574.
Kimmerer, W.J., E.S. Gross, and M. MacWilliams. 2009. Is the response of estuarine nekton to freshwater flow in the San Francisco Estuary explained by variation in habitat volume? Estuaries and Coasts 32: 375–389.
Kimmerer, W.J., E.S. Gross, and M.L. MacWilliams. 2014. Tidal migration and retention of estuarine zooplankton investigated using a particle-tracking model. Limnology and Oceanography 59: 901–906.
Kimmerer, W.J., M.L. MacWilliams, and E.S. Gross. 2013. Variation of fish habitat and extent of the low-salinity zone with freshwater flow in the San Francisco Estuary. San Francisco Estuary and Watershed Science 11. https://doi.org/10.15447/sfews.2013v11iss4art1.
Korman, J., E.S. Gross, and L.F. Grimaldo. 2021. Statistical evaluation of behavior and population dynamics models predicting movement and proportional entrainment loss of adult delta smelt in the Sacramento-San Joaquin River Delta. San Francisco Estuary and Watershed Science 19. https://doi.org/10.15447/sfews.2021v19iss1art1.
Lacan, I., and V.H. Resh. 2017. A case study in integrated management: Sacramento - San Joaquin Rivers and Delta of California, USA. Ecohydrology & Hydrobiology 16: 215–228.
Lacroix, G.L., and P. McCurdy. 1996. Migratory behaviour of post-smolt Atlantic salmon during initial stages of seaward migration. Journal of Fish Biology 49: 1086–1101.
Latour, R.J. 2016. Explaining patterns of pelagic fish abundance in the Sacramento-San Joaquin Delta. Estuaries and Coasts 39: 233–247.
Lewis, L.S., M. Willmes, A. Barros, P.K. Crain, and J.A. Hobbs. 2020. Newly discovered spawning and recruitment of threatened longfin smelt in restored and under-explored tidal wetlands. Ecology 101: ecy.2868 https://esajournals.onlinelibrary.wiley.com/doi/full/10.1002/ecy.2868.
Livingston, R.J., X.F. Niu, F.G. Lewis, and G.C. Woodsum. 1997. Freshwater input to a gulf estuary: Long-term control of trophic organization. Ecological Applications 7: 277–299.
Lund, J., E. Hanak, W. Fleenor, W. Bennett, R. Howitt, J. Mount, and P. Moyle. 2008. Comparing futures for the Sacramento-San Joaquin Delta. San Francisco: Public Policy Institute of California.
Mac Nally, R., J. Thomson, W. Kimmerer, F. Feyrer, K. Newman, A. Sih, W. Bennett, L. Brown, E. Fleishman, S. Culberson, and G. Castillo. 2010. An analysis of pelagic species decline in the upper San Francisco Estuary using multivariate autoregressive modelling (MAR). Ecological Applications 20: 1417–1430.
MacWilliams, M.L., A.J. Bever, E.S. Gross, G.S. Ketefian, and W.J. Kimmerer. 2015. Three-dimensional modeling of hydrodynamics and salinity in the San Francisco Estuary: an evaluation of model accuracy, X2, and the low-salinity zone. San Francisco Estuary and Watershed Science 13. https://doi.org/10.15447/sfews.2015v13iss1art2.
McGowan, J., E. Hines, M. Elliott, J. Howar, A. Dransfield, N. Nur, and J. Jahncke. 2013. Using seabird habitat modeling to inform marine spatial planning in central California’s National Marine Sanctuaries. PLoS ONE 8: 1–15.
Meng, L., and S.A. Matern. 2001. Native and introduced larval fishes of Suisun Marsh, California: The effects of freshwater flow. Transactions of the American Fisheries Society 130: 750–765.
Miller, W.J. 2011. Revisiting assumptions that underlie estimates of proportional entrainment of delta smelt by state and federal water diversions from the Sacramento-San Joaquin Delta. San Francisco Estuary and Watershed Science 9. https://doi.org/10.15447/sfews.2011v9iss1art2.
Mitchell, L., K. Newman, and R. Baxter. 2019. Estimating the size selectivity of fishing trawls for a short-lived fish species. San Francisco Estuary and Watershed Science 17. https://doi.org/10.15447/sfews.2019v17iss1art5.
Monismith, S.G., W.J. Kimmerer, J.R. Burau, and M.T. Stacey. 2002. Structure and flow-induced variability of the subtidal salinity field in northern San Francisco Bay. Journal of Physical Oceanography 32: 3003–3019.
Montagna, P.A., M. Alber, P.H. Doering, and M.S. Connor. 2002. Freshwater inflow: Science, policy, management. Estuaries 25: 1243–1245.
Moyle, P.B. 2002. Inland fishes of California. Berkeley: University of California Press.
Moyle, P.B., B. Herbold, D.E. Stevens, and L.W. Miller. 1992. Life history and status of the delta smelt in the Sacramento-San Joaquin Estuary, California. Transactions of the American Fisheries Society 121: 67–77.
Moyle, P.B., J.A. Hobbs, and J.R. Durand. 2018. Delta smelt and water politics in California. Fisheries 43: 42–50.
Nichols, F., J. Cloern, S. Luoma, and D. Peterson. 1986. The modification of an estuary. Science 231: 567–573.
Nixon, S.W., C.A. Oviatt, J. Frithsen, and B. Sullivan. 1986. Nutrients and the productivity of estuarine and coastal marine systems. Journal of the Limnological Society of South Africa 12: 43–71.
Nobriga, M.L., and J.A. Rosenfield. 2016. Population dynamics of an estuarine forage fish: Disaggregating forces driving long-term decline of longfin smelt in California’s San Francisco Estuary. Transactions of the American Fisheries Society 145: 44–58.
Paerl, H.W., L.M. Valdes, B.L. Peierls, J.E. Adolf, and L.W. Harding. 2006. Anthropogenic and climatic influences on the eutrophication of large estuarine ecosystems. Limnology and Oceanography 51: 448–462.
Perry, R.W., A.C. Pope, J.G. Romine, P.L. Brandes, J.R. Burau, A.R. Blake, A.J. Ammann, and C.J. Michel. 2018. Flow-mediated effects on travel time, routing, and survival of juvenile Chinook salmon in a spatially complex, tidally forced river delta. Canadian Journal of Fisheries and Aquatic Sciences: 75: 1886–1901.
Plummer, M. 2017. JAGS Version 4.3.0 user manual.
R Core Team. 2020. R: a language and environment for statistical computing. Vienna, Austria: R Foundation for Statistical Computing.
Rosenfield, J.A., and R.D. Baxter. 2007. Population dynamics and distribution patterns of longfin smelt in the San Francisco Estuary. Transactions of the American Fisheries Society 136: 1577–1592.
Rothschild, B.J., J.S. Ault, P. Goulletquer, and M. Héral. 1994. Decline of the Chesapeake Bay oyster population: A century of habitat destruction and overfishing. Marine Ecology Progress Series 11: 29–39.
Royle, J.A., and R.M. Dorazio. 2008. Hierarchical modeling and inference in ecology: The analysis of data from populations, metapopulations and communities. London: Elsevier.
Sommer, T., R. Baxter, and B. Herbold. 1997. Resilience of splittail in the Sacramento-San Joaquin Estuary. Transactions of the American Fisheries Society 126: 961–976.
Sommer, T., B. Harrell, M. Nobriga, R. Brown, P. Moyle, W. Kimmerer, and L. Schemel. 2001. California’s Yolo Bypass: Evidence that flood control can be compatible with fisheries, wetlands, wildlife, and agriculture. Fisheries 26: 6–16.
Sommer, T., B. Schreier, J.L. Conrad, L. Takata, B. Serup, R. Titus, C. Jeffres, E. Holmes, and J. Katz. 2020. Farm to fish: lessons from a multi-year study on agricultural floodplain habitat. San Francisco Estuary and Watershed Science 18. https://doi.org/10.15447/sfews.2020v18iss3art4.
Stevens, D.E., D.W. Kohlhorst, L.W. Miller, and D.W. Kelley. 1985. The decline of striped bass in the Sacramento-San Joaquin Estuary, California. Transactions of the American Fisheries Society 114: 12–30.
Stevens, D.E., and L.W. Miller. 1983. Effects of river flow on abundance of young Chinook salmon, American shad, longfin smelt, and delta smelt in the Sacramento-San Joaquin River system. North American Journal of Fisheries Management 3: 425–437.
Stompe, D.K., P.B. Moyle, A. Kruger, and J.R. Durand. 2020. Comparing and integrating fish surveys in the San Francisco Estuary: why diverse long-term monitoring programs are important. San Francisco Estuary and Watershed Science 18. https://doi.org/10.15447/sfews.2020v18iss2art4.
Taft, B.A. 1960. A statistical study of the estimation of abundance of sardine (Sardinops caerulea) eggs. Limnol and Oceanogr 5: 245–264.
Tempel, T.L., T.D. Malinich, J. Burns, A. Barros, C.E. Burdi, and J.A. Hobbs. 2021. The value of long-term monitoring of the San Francisco Estuary for Delta Smelt and Longfin Smelt. California Fish and Game 107: 148–171.
Thomson, J., W. Kimmerer, L. Brown, K. Newman, R. Mac Nally, W. Bennett, F. Feyrer, and E. Fleishman. 2010. Bayesian change-point analysis of abundance trends for pelagic fishes in the upper San Francisco Estuary. Ecological Applications 20: 1431–1448.
Utne-Palm, A.C. 2002. Visual feeding of fish in a turbid environment: Physical and behavioural aspects. Marine and Freshwater Behaviour and Physiology 35: 111–128.
Vörösmarty, C.J., M. Meybeck, B. Fekete, K. Sharma, P. Green, and J.P.M. Syvitski. 2003. Anthropogenic sediment retention: Major global impact from registered river impoundments. Global and Planetary Change 39: 169–190.
Wang, J.C.S. 2007. Spawning, early life stages, and early life histories of the Osmerids found in the Sacramento-San Joaquin Delta of California. In Tracy fish facility studies. Denver: U.S. Department of the Interior Bureau of Reclamation, Mid-Pacific Region
Wenger, S.J., and M.C. Freeman. 2008. Estimating species occurrence, abundance, and detection probability using zero-inflated distributions. Ecology 89: 2953–2959.
Whipple, A.A., R.M. Grossinger, D. Rankin, B. Stanford, and R.A. Askevold. 2012. Sacramento-San Joaquin Delta historical ecology investigation: exploring pattern and process. In Publication #672. Richmond, CA: San Francisco Estuary Institute.
Williams, P.B. 1989. Managing freshwater inflow to the San Francisco Bay Estuary. Regulated Rivers - Research and Management 4: 285–298.
Zeug, S.C., and B.J. Cavallo. 2013. Influence of estuary conditions on the recovery rate of coded-wire-tagged Chinook salmon (Oncorhynchus tshawytscha) in an ocean fishery. Ecology of Freshwater Fish 22: 157–168.
Acknowledgements
Longfin smelt monitoring data were provided by California Department of Fish and Wildlife. We thank T. Tempel for help with the monitoring data and L. Lewis, L. Grimaldo, the other members of our extended longfin smelt research team for discussions and insights. We thank M. Weaver and two anonymous reviewers for their thoughtful comments.
Funding
Funding was provided by California Department of Fish and Wildlife under agreement #P1696013 to San Francisco State University.
Author information
Authors and Affiliations
Corresponding author
Additional information
Communicated by Steven Litvin
Supplementary Information
Below is the link to the electronic supplementary material.
Rights and permissions
Open Access This article is licensed under a Creative Commons Attribution 4.0 International License, which permits use, sharing, adaptation, distribution and reproduction in any medium or format, as long as you give appropriate credit to the original author(s) and the source, provide a link to the Creative Commons licence, and indicate if changes were made. The images or other third party material in this article are included in the article's Creative Commons licence, unless indicated otherwise in a credit line to the material. If material is not included in the article's Creative Commons licence and your intended use is not permitted by statutory regulation or exceeds the permitted use, you will need to obtain permission directly from the copyright holder. To view a copy of this licence, visit http://creativecommons.org/licenses/by/4.0/.
About this article
Cite this article
Kimmerer, W., Gross, E. Population Abundance and Diversion Losses in a Threatened Estuarine Pelagic Fish. Estuaries and Coasts 45, 2728–2745 (2022). https://doi.org/10.1007/s12237-022-01101-w
Received:
Revised:
Accepted:
Published:
Issue Date:
DOI: https://doi.org/10.1007/s12237-022-01101-w