Abstract
In cities, traffic is one of the major determinants for air quality. Studies have shown that proximity to roadways with heavy traffic has been linked with increased incidence and prevalence of a range of health effects such as adverse birth outcomes, dementia, asthma, and increased risk of bronchitis, wheezing, deficits in lung function growth and airway inflammation, especially among children. In Mexico, 103 urban areas have air quality monitoring, whilst more than 2,000 cities lack any information and only 29 have monitoring systems that are robust enough to estimate exposure. This poses serious limitations for environmental and health authorities when aiming at assessing current exposure levels to Traffic-Related Air Pollution (TRAP) with the purpose of designing and implementing policies to reduce the impacts of poor air quality in the population. This study proposes an approach to estimate the population potentially exposed to TRAP using Space Syntax’s accessibility index as the urban form variable. We selected the ten most accessible roads of the five Mexican cities to estimate the proportion of vulnerable population (residents, employees, schoolchildren, hospital patients) with the highest potential exposure to TRAP at a distance of 500 m. Our findings show a similar proportion of the population continually exposed to TRAP due to the proximity to roads with heavy traffic than studies using more complex models. Finally, this study presents alternatives to reduce current population exposure to traffic emissions in Mexican cities.
Similar content being viewed by others
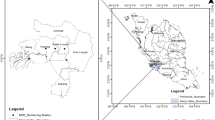
Avoid common mistakes on your manuscript.
Introduction
Air pollution is considered an important environmental risk to health. According to the World Health Organization (World Health Organization, 2018) in 2016, 91% of the world population was living in places where air pollution levels were harmful. Due to ambient air pollution, premature deaths worldwide per year were estimated at 4.2 million, mostly associated with exposure to particulate matter (PM) and ozone (O3). Exposure to these pollutants has been linked to increased risk of mortality due to diseases that affect different organs, for example, lung cancer, chronic obstructive pulmonary disease (COPD), acute respiratory disease, ischemic heart disease, stroke, dementia, type 2 diabetes and low-weight birth in pregnant women (Health Effects Institute, 2019).
Studies have shown that proximity to roadways with heavy traffic is positively associated with increased incidence and prevalence of asthma, and increased risk of bronchitis, wheezing, deficits in lung function growth and airway inflammation, especially among children. These effects have been observed independent of regional air pollution levels and are also observable on sensitive adults and low-income and racial/ethnic minority groups (Chen et al., 2015; Huynh et al., 2010; Khreis et al., 2017; Meng et al., 2006).
In Mexico, the most recent report on urban air quality (Instituto Nacional de Ecología y Cambio Climático, 2019) shows that even though 83 urban areas have robust air quality monitoring, only 29 are robust and consistent enough to provide reliable data on air quality. In most of these cities air quality standards are frequently exceeded. According to that same report, the Institute for Health Metrics and Evaluation estimated that 48,072 people died prematurely in Mexico due to poor air quality in 2017, whilst the System of National Accounts estimated the costs of environmental degradation attributable to air pollution for that same year as equivalent to 2.8% of GDP (IHME, 2019 and INEGI, 2019 in Instituto Nacional de Ecología y Cambio Climático, 2019).
Emissions inventories show that vehicles are primarily responsible for the declining air quality in growing Mexican urban areas. However, only a handful of cities besides Mexico City have implemented primary or secondary strategies (as defined by Künzli, et al (2003)) to reduce population exposure to Traffic-Related Air Pollution (TRAP). TRAP is a mixture of pollutants present in exhaust emissions, non-combustion emissions (e.g. road dust, tire wear) and secondary compounds formed in the atmosphere. This mixture typically includes contaminants like particulate matter that have been linked to increased risk of premature mortality due to respiratory and cardiovascular disease, and precursors of ozone formation, such as hydrocarbons (HC) and nitrogen oxides (NOX) among other pollutants like carbon dioxide (CO2), carbon monoxide (CO), mobile source air toxics (MSATs) that also pose significant health risks to the population.
Previous studies have found that a significant proportion (30–45%) of urban population in large cities in North America live within a 300 to 500 m distance from a highway or major roadway, the area most highly affected by traffic emissions, which suggests that exposures to traffic pollutants are a public health concern in those urban areas (HEI Panel on the Health Effects of Traffic-Related Air Pollution, 2010). Our study represents a first approach to find out the proportion of Mexican residential population potentially exposed to TRAP in five medium-sized cities in Central Mexico where population and vehicle ownershipFootnote 1 have increased significantly in the last decades (Islas Rivera et al., 2011; SEDESOL, 2011): Aguascalientes, Irapuato, Morelia, Querétaro and San Luis Potosí (Table 1). We also estimate the number of employees, school attendants and hospital beds that could potentially be regularly exposed to near-roadway vehicle emissions.
Our aim is, therefore, to add salience to air pollution from traffic and its potential effects on nearby population and provide policymakers with accessible data and tools to work towards reducing exposure of sensitive populations to poor air quality in Mexican cities in the near future.
Urban form Analysis Using Space Syntax
We first analyze the urban configuration using Space Syntax, a topological network methodology for urban analysis that has proven to be a robust urban-movement predictor (Hillier, 1996). Integration (or spatial accessibility), as calculated with the Space Syntax methodology, represents the shortest routes in a network resulting from the underlying planar graph of the physical connections (Batty, 2009, p. 192). The Integration Index measures the relative nearness of each component of the street network and can be considered as a variation of network analysis for Spatial Accessibility (Handy & Niemeier, 1997) because it measures the to-movement, i.e. the highest the Integration Index, “the easier it is to get to than other streets” (Hillier & Vaughan, 2007, p. 6). This feature is closely related to the definition of accessibility: “the ease with which a certain site (destination) can be reached, from other points in the territory (origins), synthesizing the opportunities for contact and interaction between certain origins and destinations” (Goodall in Garrocho & Campos Alanís, 2006, p. 353).
Previous studies have shown that the Integration value can be taken as a potential indicator of movement (pedestrian or vehicular) for each line, with urban implications such as the movement around the city, land-use location decisions, land value, safety and security (Chiaradia et al., 2005; Figueiredo & Amorim, 2007; Hillier & Vaughan, 2007; Hillier et al., 1993; Paul, 2009; Penn et al., 1998). We therefore use this index as a proxy of relative traffic volume to select the roads to include in this study.
For the network analysis, we use publicly available information of local and federal road networks from the National Institute of Statistics and Geography (INEGI, 2016). The road center line network of each city is complemented with the federal highways database, where applicable, to ensure that the whole urban area is completely connected. A process of revision and cleaning using ArcGIS (ESRI, 2018) is done to ensure that there are no duplicate connections and all real roadway intersections are indeed recognized on the file, using GoogleEarth to verify inconsistencies and doubtful road classifications. Using the criteria proposed by Figuereido and Amorim (2004), we then proceed to build an axial map of continuous lines with Mindwalk 1.0 (Figueiredo, 2005), indicating continuity of vehicle flow, based on the intersection angle of two roadways. Afterwards, using the same software, we estimate the global integration index for each line in the network.Footnote 2
Figure 1 shows the Global Integration Index by street segment for the city of Querétaro. Although the centre of the urban fabric is the most integrated, indicated by red lines and higher values, long lines come out of this core and connect the whole network. The global integration values decrease gradually, until they reach the most segregated areas (lines in blue).
Method and Databases
Roadway selection
Su and others (2015), analyzed how differences in urban form affect exposure to TRAP in near-roadway environments in seven global cities, including Mexico City, using as a proxy the population that resides within an exposure zone of 500 m (maximum) along all “highways and major roadways”. As noted by the authors, one of the limitations presented for doing this study was the variability in road classification criteria between cities, e.g. Mexican data “did not include specific categories for highways or major roadways” (p. 83). Hence, they resorted to constructing their own classification using Google Earth and expert judgment.
To overcome this limitation, our approach was to use Space Syntax’s Integration Index as the main criterion to select which roads to include in our analysis due to the strong correlation between the index and potential movement in the street network. We used as a basis a previous analysis for Mexico City with Space Syntax (Garnica Monroy, 2012) which showed that 9% of the highest integrated lines covered 27% of the whole urban area and 21% of the street networkFootnote 3 and crossed the central area of the city in two directions: north-to-south and east-to-west. The study then observed only the 40 most accessible lines, with two remarkable findings for the present study. The first one is that these lines were not concentrated in just one small area or cluster, but they connect the center with areas considered as peripherals. Considering that our study wants to look at streets distributed all over the studied cities, we found this fact as pertinent. The second finding was, that when the most accessible lines were compared with “main roads” (per INEGI’s classification), there was a big coincidence in most of them. Considering that Space Syntax analyzes the street network without taking into account any type of urban classification, width, or any other data relative to the street use, we confirmed our decision to use it as the “selector” tool.
Considering the size of the chosen cities for the present study (and due to future work for a larger study),Footnote 4 we decided to select only the ten roadways with the highest Integration Index (called corridors from this point forward) to estimate the fraction of the population potentially exposed to TRAP.
Figure 2 shows the ten most integrated streets in each city. In general, they provide a good coverage of most of the urban footprint (in grey). Comparison with Google Earth maps and informal interviews with government representatives in each city provided confirmation that the selected lines represent major roadways where all kinds of vehicles (heavy-duty, buses and all types of cars) move through, proving useful to our larger study objectives.
Population
Su and others (2015) used the data available at an aggregated level (similar to a census tract) called the Basic Geostatistical Area (AGEB) for 2005. Due to the disaggregated data available for the first time in the National Census of Population and Housing (INEGI, 2016), our analysis uses population databases for 2010 at a block level to estimate, in a more accurate way, the number of residents living within 500 m of the center road line of each of the ten most integrated roadways (Fig. 3). Our decision of considering the size of this buffer is that it represents a five-minutes’ walk, which is a common parameter used by urban designers to measure access to basic urban services (including transit).
An important consideration we took into account was the perimeter of the buffer, when it did not cover a whole block. To overcome this, we used GIS to calculate the proportion of the block covered by the buffer. Then, using that ratio, we computed the proportion of population that lives in the block.
In addition to the estimates by Su and others (2015) we also calculate the number of employees, schoolchildren and hospital beds located in these buffers to underscore potential areas of high chronic exposure. Information on the number of employees for each economic unit is available from the National Institute of Geography and Statistics but is reported in ranges (e.g., 0–5, 6–10, 11–30, 31–50, 51–100, 101–250, and over 250) in the National Economic Census (INEGI, 2015). Hence, we decided to use the mean of these ranges for our estimates. We used publicly available records from the Public Education Secretariat (Secretaría de Educación Pública) (SEP & INEGI, 2013) of students and staff -administrative and teachers- officially registered in public or private schools (from K1 to high school). We could only account for staff and number of beds in public hospitals, available in the Public Health system databases from the Health Secretariat (Secretaría de Salud) (Secretaria de Salud, 2012); unfortunately, there is no information available regarding private hospitals or the number of outpatients.
Population’s Exposure to TRAP
In this section, we analyze the results regarding the proportion and characteristics of residents, employees, and schools and hospitals exposed to traffic-related air pollution along the selected corridors within a 500 m buffer per city. To determine the size for these buffers, we used the criteria of a range of “up to 300 to 500 m from a highway or a major road as the area most highly affected by traffic emissions” proposed by the Health Effects Institute study (HEI Panel on the Health Effects of Traffic-Related Air Pollution, 2010, p. ix), and also used by Su and others (2015). Then, we compare them with the Health Effects Institute study (HEI Panel on the Health Effects of Traffic-Related Air Pollution, 2010), which is also used as a reference by Su and others. To avoid double-counting and in the absence of data on the residence of hospital and school staff, employees, schoolchildren and patients, we present the results separately for each type of population.
Residents
In our analysis, we find that the proportion of total residents living within the 500 m buffer is similar but slightly larger than the results presented for North America by the HEI (2010) representing 48% of the total population of the five cities (Table 2). However, individually, the selected roadways in Irapuato and Aguascalientes include more than 60% of the population, whilst those in Morelia and San Luis Potosi include almost half of the population. In contrast, less than one-third of the population lives along Querétaro’s 10 most integrated roadways.
Given the increase in urban expansion rate of these five cities in the last 30 years (Table 1), we also analyze residential density using three different criteria: the overall mean residential density, the mean density at block level inside the buffer and the mean density at block level outside the buffer (Table 3). Figure 4 shows density estimates for Irapuato.
We find that for all cities the density inside the buffer is always higher than the mean density for the whole city. For example, the mean residential densities inside the buffer in Aguascalientes and San Luis Potosí are at least 50% larger than the mean residential density of the city, whilst in Irapuato and Morelia the inside-buffer residential density is 30% larger than the city’s mean residential density. Querétaro shows the lowest density (but nonetheless higher than the mean), which might be because of the great expansion of the city compared with its population growth.
In general, we observed that the proportion of exposed residential population follows roughly the city’s mean population density, i.e. the city with the largest population density (Aguascalientes, 153 inhab/Ha) has the second largest proportion of population exposed to TRAP (62 out of 100 residents live within the 500 m buffer, an estimated 578,714). In contrast, Querétaro has the least population density (120 inhab/Ha) and also the least proportion of resident population exposed to TRAP within the 500 m buffer (30%—326,376 people) (Table 3).
We find larger disparities when comparing the densities inside and outside the buffer areas. On average, the inside-buffer/outside-buffer density ratio is 1.60, being Aguascalientes the city that presents the largest ratio (2.13) and Querétaro the one that presents the smaller ratio (1.04).
Employees and Economic Activities
Table 4 shows the estimated employment density for all five cities. The mean inside-buffer/city employment density ratio for the five cities is 1.60, Morelia being the one that has the largest ratio and San Luis Potosí the lowest ratio (2.18 and 1.28 respectively). The inside/outside ratios of employment density are larger than the ratios observed for population densities (1.96). However, Querétaro (Fig. 5) has the highest employment density ratio (4.22), and San Luis Potosí has lowest (1.16).
It is worth noticing that the mean residential density is six times larger than the mean employment density (Table 5), although this ratio is slightly lower in the case of mean densities inside the buffers.
On average, 68% of the economic units, mostly service industry (e.g., retail and wholesale sales, transportation and distribution, restaurants, clerical services, media, tourism, insurance, banking, etc.), are located inside the 500 m buffers of the five cities (Table 6). Aguascalientes has the highest proportion of economic units inside the buffer, closely followed by Morelia; Querétaro has the lowest number (Fig. 6).
Economic units located inside 500 m buffer: Querétaro. Note: To allow for a clear visualization, only those economic units within the buffer are shown. Classification per number of employees was provided by the Economic Census (INEGI, 2015)
Schools and Hospitals
The proximity of schools and hospitals to important roadways is helpful to underscore the potential chronic exposure of vulnerable populations to traffic-related air pollutants (Green et al., 2004; Wu & Batterman, 2006). Table 7 shows our estimates of schoolchildren, school and hospital staff and hospital beds within the 500 m buffers. On average, more than 200,000 children attend school in each city in the proximity of major roadways. Aguascalientes, the densest city, has the largest number of registered students potentially exposed to traffic-related air pollution, followed by Irapuato, the least populated city in our study.
It is worth noting that, up to 2011, Federal regulations stipulated that public schools and hospitals should be located adjacent to a main road to facilitate access (SEDESOL, 1999). Also, since the 500 m buffers cover most of the central areas and some important roads of the five cities, we expected to find larger public hospitals (e.g., the Regional and General Hospitals) located within the buffers. Querétaro, with the largest population of the five cities, has the largest number of beds inside the buffer and the largest number of potentially exposed hospital and school staff (Fig. 7), followed by Aguascalientes (the densest city), Morelia, San Luis Potosí, and Irapuato.
Strategies and Public Policies for Urban Planning to Improve Public Health
The results from our study are quite relevant from a public health perspective. They show that there is a considerable percentage of residents and schoolchildren that are potentially chronically exposed to TRAP, increasing their risk of suffering detrimental effects on their health. Additionally, we identified exposure “hotspots” in each city with the highest potential concentration of people, i.e. residents, schoolchildren, hospital beds, employees located within the buffers (Fig. 8). We believe these “hotspots” can be very useful to local authorities in identifying specific areas of concern that could be good candidates for establishing additional air quality monitoring and implementing exposure-reducing strategies.
Hence, we looked for applied strategies and public policies that could be applied by governments to reduce population exposure to TRAP, and at the same time influence urban planning through the built environment. We found that there are, roughly, two types of strategies that can be applied: 1) those aimed at reducing traffic emissions, and 2) those aimed at increasing air pollutant dispersion. Mobile source emissions can be reduced by implementing different measures, such as reducing traffic speed (Ahn & Rakha, 2009); optimizing vehicular flow through stop-lights coordination (California Air Resources Board, 2017); restricting the access of certain vehicles to specific areas of the city—and establishing Low Emissions Zones—through congestion charging or specific traffic bans, or both—like in Stockholm, London, Berlin, Rome, or Milan (Lutz, 2015); and improving cycling and pedestrian infrastructure to increase non-motorized mobility (Kendrick et al., 2011). Besides improving vehicle technology and fuel quality through mandatory standards, changes in local road infrastructure and traffic conditions can greatly reduce local emissions, which can be highly beneficial, particularly near educational and health facilities. For example, transforming access streets into pedestrian paths, changing traffic flow direction, restricting passenger pick up or drop off sites, identifying highly polluting vehicles and restricting their circulation, designating specific times for heavy traffic circulation and limiting allowed idling time on specific roads. Research has shown that by implementing these types of measures, air pollution can be reduced in the surrounding areas by as much as 40%, depending on the pollutant being evaluated. Additional benefits related to road safety (World Health Organization, 2008) and active mobility can also be accrued.
Air pollutant dispersion can be increased by improving urban design and changing the built environment. Sample measures in this category include, for example, designing streets with wide sidewalks, bike lanes and public open spaces to increase airflow and help reduce pollutant concentrations (Paulson et al., 2017); nature-based solutions (NBS) such as planting trees and plants on sidewalks (McDonald, et al., 2016); and incorporating physical barriers like sound walls around 500 feet of high-volume traffic streets (Ning et al., 2010). Research findings show that these strategies can reduce certain pollutants like NOx and PM between 10 and 50% respectively while also increasing wellbeing and improving humidity and temperature conditions in the community.
Conclusions
Our findings suggest that a very significant proportion of the population in the selected cities could be continually (chronically) exposed to TRAP due to their proximity to roads with heavy traffic. We find that, in 2010, the population living within 500 m distance of the selected roadways was almost half of the whole population living in the selected cities. These estimates are higher than the 30 to 45% estimated by the HEI (2010) for cities in North America, which could be explained by the increased population density within the 500 m buffers we found in the five selected cities. Our estimates of hospital beds and students within the buffers in 2012 and 2013 underscore the vulnerability of children and sensitive populations potentially exposed to TRAP in these cities, largely originated by planning regulations in force until 2011. Our finding that, in 2015, between 5 and 8 out of every 10 economic units in the five cities are located in the buffers along the most integrated roads confirmed our expectations, given the relationship between the integration index and land use seen in previous studies (Hillier, 1996). This, too, has implications for exposure assessment; even though employment density proved less than population density, there is still a significant proportion of employees in all cities that could be exposed daily to traffic emissions for several hours.
We find that using Space Syntax is a reasonable alternative to the strategy used by Su et al. (2015) to identify highways and major roadways, especially when comparing between cities in the absence of consistent road classification criteria. Based on the premise that “the configuration of the urban layout itself is the main generator of movement patterns” (Oliveira, 2016, p.) we consider that selecting the ten roads with the highest integration index using Space Syntax was a good alternative to road classification, as well as a useful tool in the absence of data on traffic flows.
The 500 m buffer analysis is useful not only for exposure analysis, but it is also one of the most common parameters used by urban designers for the provision of basic services (including transit), because it represents a five-minutes’ walk. Thus, the identified buffers represent urban areas with higher density that should be taken into account when planning the provision of basic services and public transit. This study also shows that the top ten highly integrated streets can be considered by urban planners as feasible transport corridors, with proper urban / street design, i.e. shared streets and mixed land-use to create attractive places for people, but with adequate provisions to reduce population’s exposure to TRAP, such as those mentioned in Sect. 5.
Given the rapid population growth in these five cities, we expect that the actual number of exposed populations has increased significantly since 2010 and that the new National Census of Population and Housing of 2020 (to be published in 2021) will be useful to update these estimates. Future exposure studies could focus on collecting vehicle field data to confirm our assumption that the integration index is a good proxy for traffic flow, performing air quality and exposure measurements in the identified “hotspots”, applying air quality modeling to estimate exposure along the 10 most accessible roadways and analyzing environmental justice issues, i.e. the relationship of air pollution exposure and income. Additional urban analyses could also study the ratio of land use occupancy which would be useful to point if these areas have the recommended balance between residential and commercial land use (Rueda, 2012).
In the absence of air quality monitoring and modeling, this methodology renders interesting findings that could be helpful for policymakers to advocate for urban policies that could reduce health risks and protect the most vulnerable. We hope that, as a consequence of this analysis (or others regarding exposure to TRAP), local and State authorities consider applying the suggested policies to reduce traffic emissions and to increase the dispersion of air pollutants, according to the very specific conditions of the built environment and population’s needs.
Notes
According to Islas Rivera et. al, the proportion of cars per 1,000 inhabitants grew from 160 to 300 between years 2000 and 2010. Medina Ramírez (2012) estimated that the motorization rate in Mexico grew 6.32% in 2012.
Space Syntax being a topological model, the system can be analysed at different urban scales. Global integration shows the concentration of activities throughout the territory and allows us to understand how individuals move and interact throughout the territory, while local integration presents the concentration of activities in certain areas of the territory, thus allowing us to understand how the individuals move and interact within these in more detail.
The total number of lines of the Space Syntax maps was 91,586.
The results of the present study were areas in each city with the highest potential concentration of observed population (Fig. 8). These hotspots were used to determine ten specific points (per city) where field work was done: 1) counting vehicles at three different periods, during different days of the week, 2) classifying vehicles, and 3) register real traffic speed at the selected points. All this data was then used to do a city-wide estimation of emissions of air-pollutants from mobile sources.
References
Ahn, K., & Rakha, H. (2009). A field evaluation case study of the environmental and energy impacts of traffic calming. Transportation Research Part d: Transport and Environment, 14(6), 411–424. https://doi.org/10.1016/j.trd.2009.01.007
Batty, M. (2009). Accessibility: In search of a unified theory. Environment and Planning b: Planning and Design, 36(2), 191–194.
Garrocho, C., & Campos Alanís, J. (2006). Un indicador de accesibilidad a unidades de servicios clave para ciudades mexicanas: Fundamentos, diseño y aplicación. Economía y Sociedad, V, I(22), 349–397.
Green, R. S., Smorodinsky, S., Kim, J. J., McLaughlin, R., & Ostro, B. (2004). Proximity of California public schools to busy roads. Environmental Health Perspectives, 112(1), 61–66. https://doi.org/10.1289/ehp.6566
Handy, S., & Niemeier, D. A. (1997). Measuring Accessibility: An Exploration Of Issues And Alternatives. Environment and Planning A, 29(7), 1175–1194.
Hillier, B. (1996). Cities as movement economies. Urban Design International, 1(1), 41–60.
Hillier, B., Penn, A., Hanson, J., Grajewski, T., & Xu, J. (1993). Natural movement: Or, configuration and attraction in urban pedestrian movement. Environment and Planning b: Planning and Design, 20, 29–66.
Hillier, B., & Vaughan, L. (2007). The City as One Thing. Progress in Planning, 67(3), 205–230.
Kendrick, C. M., Moore, A., Haire, A., Bigazzi, A., Figliozzi, M., Monsere, C. M., & George, L. (2011). Impact of Bicycle Lane Characteristics on Exposure of Bicyclists to Traffic-Related Particulate Matter. Transportation Research Record: Journal of the Transportation Research Board, 2247(1), 24–32. https://doi.org/10.3141/2247-04
Khreis, H., Kelly, C., Tate, J., Parslow, R., Lucas, K., & Nieuwenhuijsen, M. (2017). Exposure to traffic-related air pollution and risk of development of childhood asthma: A systematic review and meta-analysis. Environment International, 100, 1–31. https://doi.org/10.1016/j.envint.2016.11.012
Künzli, N., McConnell, R., Bates, D., Bastain, T., Hricko, A., Lurmann, F., Avol, E., Gilliland, F., & Peters, J. (2003). Breathless in Los Angeles: The Exhausting Search for Clean Air. American Journal of Public Health, 93(9), 1494–1499. https://doi.org/10.2105/AJPH.93.9.1494
Ning, Z., Hudda, N., Daher, N., Kam, W., Herner, J., Kozawa, K., Mara, S., & Sioutas, C. (2010). Impact of roadside noise barriers on particle size distributions and pollutants concentrations near freeways. Atmospheric Environment, 44(26), 3118–3127. https://doi.org/10.1016/j.atmosenv.2010.05.033
Oliveira, V. (2016). Urban Morphology. Springer International Publishing. https://doi.org/10.1007/978-3-319-32083-0
Penn, A., Hillier, B., Banister, D., & Xu, J. (1998). Configurational modelling of urban movement networks. Environment and Planning b: Planning and Design, 25(1), 59–84.
Su, J. G., Apte, J. S., Lipsitt, J., Garcia-Gonzales, D. A., Beckerman, B. S., de Nazelle, A., Texcalac-Sangrador, J. L., & Jerrett, M. (2015). Populations potentially exposed to traffic-related air pollution in seven world cities. Environment International, 78, 82–89.
Wu, Y.-C., & Batterman, S. A. (2006). Proximity of schools in Detroit, Michigan to automobile and truck traffic. Journal of Exposure Science and Environmental Epidemiology, 16(5), 457.
California Air Resources Board. (2017). Strategies to Reduce Air Pollution Exposure Near High-Volume Roadways | California Air Resources Board. Strategies to Reduce Air Pollution Exposure Near High-Volume Roadways. https://ww2.arb.ca.gov/es/resources/fact-sheets/strategies-reduce-air-pollution-exposure-near-high-volume-roadways
Chen, Z., Salam, M. T., Eckel, S. P., Breton, C. V., & Gilliland, F. D. (2015). Chronic effects of air pollution on respiratory health in Southern California children: Findings from the Southern California Children’s Health Study. Journal of Thoracic Disease, 7(1), 46–58. https://doi.org/10.3978/j.issn.2072-1439.2014.12.20
Chiaradia, A., Moreau, E., & Raford, N. (2005). Configurational Exploration Of Public Transport Movement Networks: A Case Study, The London Underground. Proceedings of the 5th Space Syntax Symposium, 541–552.
ESRI. (2018). ArcGIS Desktop (10.6.1) [Computer software]. Environmental Systems Research Institute.
Figueiredo, L., & Amorim, L. (2007). Decoding the urban grid: Or why cities are neither trees nor perfect grids. In A. S. Kubat, O. Ertekin, Y. I. Guney, & E. Eyuboglu (Eds.), Proceedings of the 6th International Space Syntax Symposium (pp. 1–17). ITU Faculty of Architectur.
Figueiredo, L. (2005). Mindwalk 1.0: Vol. 1.0.
Garnica Monroy, R. (2012). La accesibilidad como instrumento analítico para comprender la organización espacial de la Ciudad de México: Un estudio a dos escalas usando Space Syntax [Doctorado en Estudios Urbanos y Ambientales, El Colegio de México]. https://colmex.userservices.exlibrisgroup.com/view/delivery/52COLMEX_INST/1265027050002716
Health Effects Institute. (2019). State of Global Air / 2019 (p. 22). Health Effects Institute. https://www.stateofglobalair.org/sites/default/files/soga_2019_report.pdf
HEI Panel on the Health Effects of Traffic-Related Air Pollution. (2010). Traffic-Related Air Pollution: A Critical Review of the Literature on Emissions, Exposure, and Health Effects (HEI Special Report 17; p. 386). Health Effects Institute. https://www.healtheffects.org/system/files/SR17TrafficReview.pdf
Huynh, P., Salam, M. T., Morphew, T., Kwong, K. Y. C., & Scott, L. (2010). Residential Proximity to Freeways is Associated with Uncontrolled Asthma in Inner-City Hispanic Children and Adolescents. Journal of Allergy, 2010. https://doi.org/10.1155/2010/157249
INEGI. (2015). Directorio Nacional de Unidades Económicas. DENUE [Directorio Estadístico Nacional de Unidades Económicas]. Censos Económicos. https://www.inegi.org.mx/app/mapa/denue/
INEGI. (2016). Censo de Población y Vivienda 2010. INEGI. https://www.inegi.org.mx/programas/ccpv/2010/
Instituto Nacional de Ecología y Cambio Climático. (2019). Informe Nacional de Calidad del Aire 2018, México. Coordinación General de Contaminación y Salud Ambiental, Dirección de Investigación de Calidad del Aire y Contaminantes Climáticos. https://sinaica.inecc.gob.mx/archivo/informes/Informe2018.pdf
Islas Rivera, V., Moctezuma Navarro, E., Hernandez Garcia, S., Lelis Zaragoza, M., & RUVALCABA MARTINEZ, J. (2011). Urbanizacion y motorizacion en México. Publicacion Tecnica, 362.
Lutz, M. (2015). Low Emission Zones in Europe: Access restriction criteria, vehicle identification essentials for implementation. http://iki-alliance.mx/download/LEZ-Martin-Lutz.pdf
McDonald, R., Kroeger, T., Boucher, T., Longzhu, W., & Salem, R. (2016). Planting Healthy Air. The Nature Conservancy. https://www.nature.org/content/dam/tnc/nature/en/documents/20160825_PHA_Report_Final.pdf
Medina Ramírez, S. (2012). La importancia de reducción del uso del automóvil en México. ITDP Mexico.
Meng, Y.-Y., Rull, R. P., Wilhelm, M., Ritz, B., & English, P. (2006). Living near heavy traffic increases asthma severity.
Paul, A. (2009). An integrated approach to modeling vehicular movement networks: Trip assignment and space syntax. Texas Tech University.
Paulson, S. E., DeShazo, J., Winer, A., Venkatram, A., Choi, W., Ranasinghe, D., Schulte, N., Wu, L., Bunavage, K., & Seguel, R. (2017). Identifying urban designs and traffic management strategies for southern California that reduce air pollution exposure. https://ww2.arb.ca.gov/sites/default/files/classic//research/apr/past/12-308.pdf
Rueda, S. (2012). El urbanismo ecológico: Su aplicación en el diseño de un ecobarrio en Figueres. Agencia de Ecología Urbana de Barcelona.
Secretaria de Salud. (2012). Catálogos CLUES. Catálogo CLUES. http://www.dgis.salud.gob.mx/contenidos/intercambio/clues_gobmx.html
SEDESOL. (1999). Sistema Normativo De Equipamiento. http://www.inapam.gob.mx/es/SEDESOL/Documentos
SEDESOL. (2011). La expansión de las ciudades 1980–2010. SEDESOL.
SEP, & INEGI. (2013). Atlas Educativo. Censo de Escuelas, Maestros y Alumnos de Educación Básica y Especial 2013. https://www.inegi.org.mx/sistemas/mapa/atlas/
World Health Organization. (2008). Speed management: A road safety manual for decision-makers and practitioners. Global Road Safety Partnership. https://apps.who.int/iris/bitstream/handle/10665/43915/9782940395040_eng.pdf?sequence=1
World Health Organization. (2018). WHO | Promoting health: Guide to national implementation of the Shanghai Declaration. WHO; World Health Organization. http://www.who.int/healthpromotion/publications/guide-national-implementation-shanghai-declaration/en/
Acknowledgements
The team also included: María Tania López-Villegas -Consultant-, Wendy Erika Martínez-Reséndiz and Susana Elisa Medina-Lezama from CONCYTEQ—Consejo de Ciencia y Tecnología del Estado de Querétaro-.
Funding
The present study is part of the project “Development of an urban-environmental methodology to estimate the impacts on public health of vehicle emissions in medium-sized cities with accelerated vehicle and population growth” funded by CONACYT (2015–01-1129 of the Proyectos de Desarrollo Científico para Atender Problemas. Nacionales).
Author information
Authors and Affiliations
Corresponding author
Ethics declarations
Conflict of Interest
The authors declare that they have no conflict of interest.
Additional information
Publisher's Note
Springer Nature remains neutral with regard to jurisdictional claims in published maps and institutional affiliations.
Rights and permissions
Open Access This article is licensed under a Creative Commons Attribution 4.0 International License, which permits use, sharing, adaptation, distribution and reproduction in any medium or format, as long as you give appropriate credit to the original author(s) and the source, provide a link to the Creative Commons licence, and indicate if changes were made. The images or other third party material in this article are included in the article's Creative Commons licence, unless indicated otherwise in a credit line to the material. If material is not included in the article's Creative Commons licence and your intended use is not permitted by statutory regulation or exceeds the permitted use, you will need to obtain permission directly from the copyright holder. To view a copy of this licence, visit http://creativecommons.org/licenses/by/4.0/.
About this article
Cite this article
Garnica-Monroy, R., Garibay-Bravo, V., Gonzalez-Gonzalez, A. et al. Spatial Analysis of Exposure to Traffic-Related Air Pollution in Mexico: Implications for Urban Planning to Improve Public Health. Appl. Spatial Analysis 15, 647–665 (2022). https://doi.org/10.1007/s12061-021-09415-1
Received:
Accepted:
Published:
Issue Date:
DOI: https://doi.org/10.1007/s12061-021-09415-1