Abstract
Time series prediction of wind speed has been widely used in wind power generation. The volatility and instability of wind speed have a large negative impact on wind turbines and power systems, which can lead to grid collapse in severe cases. Therefore, accurate wind speed prediction is crucial for wind power generation. In this paper, considering the influence of different parameters on algorithm training and prediction, an improved moth flame optimization algorithm is constructed to optimize the LSTM wind energy prediction system to obtain better performance. The system consists of three modules: data preprocessing, optimization, and prediction. The data preprocessing module uses fuzzy information granulation to blur the input data. On this basis, the combination of swarm intelligent optimization algorithm and prediction model can effectively predict wind speed time series. Taking the California wind farm as an example, the MAPE of the experiment in the short-term forecast is 3.15%, the MAPE of the medium-term forecast is 4.38%, and the MAPE of the long-term forecast is 18.28%. The experimental results show that the proposed model has obvious advantages over the previous model.
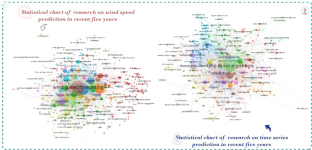











Similar content being viewed by others
Data availability
The data sets generated and analyzed during the current study are available from the corresponding author upon reasonable request.
References
Abou Houran M, Bukhari SMS, Zafar MH, Mansoor M, Chen W (2023) COA-CNN-LSTM: cCoati optimization algorithm-based hybrid deep learning model for PV/wind power forecasting in smart grid applications, Applied Energy, Volume 349. ISSN 121638:0306–2619. https://doi.org/10.1016/j.apenergy.2023.121638
Abualigah L, Hanandeh ES, Zitar RA, Thanh CL, Khatir S, Gandomi AH (2023) Revolutionizing sustainable supply chain management: aA review of metaheuristics, Engineering Applications of Artificial Intelligence, Volume 126, Part A. ISSN 106839:0952–1976. https://doi.org/10.1016/j.engappai.2023.106839
Al-qaness MA, Ewees AA, Fan H, Abualigah L, Abd Elaziz M (2022) Boosted ANFIS model using augmented marine predator algorithm with mutation operators for wind power forecasting. Appl Energy 314:118851
Cai Z, Dai S, Ding Q, Zhang J, Xu D, Li Y (2023) Gray wolf optimization-based wind power load mid-long term forecasting algorithm. Comput Electr Eng 109:108769
Dai Z, Zhang Z, Chen M (2023) The home health care location-routing problem with a mixed fleet and battery swapping stations using a competitive simulated annealing algorithm, Expert Systems with Applications, Volume 228. ISSN 120374:0957–4174. https://doi.org/10.1016/j.eswa.2023.120374
Ehteram M, Ghanbari-Adivi E (2023) Self-attention (SA) temporal convolutional network (SATCN)-long short-term memory neural network (SATCN-LSTM): an advanced python code for predicting groundwater level. Environ Sci Pollut Res 30:92903–92921. https://doi.org/10.1007/s11356-023-28771-8
Gao P, Zhou L, Zhao X, Shao B (2023) Research on ship collision avoidance path planning based on modified potential field ant colony algorithm, Ocean & Coastal Management, Volume 235. ISSN 106482:0964–5691. https://doi.org/10.1016/j.ocecoaman.2023.106482
Gers FA, Schmidhuber J, Cummins F (2000) Learning to forget: continual prediction with LSTM. Neural Computation 12(10):2451–2471. https://doi.org/10.1162/089976600300015015
Graves A, Fernández S, Schmidhuber J (2005) Bidirectional LSTM networks for improved phoneme classification and recognition. In International conference on artificial neural networks. Springer, Berlin Heidelberg, Berlin, Heidelberg
Han B, Li B, Qin C (2023) A novel hybrid particle swarm optimization with marine predators, Swarm and Evolutionary Computation. ISSN 101375:2210–6502. https://doi.org/10.1016/j.swevo.2023.101375
He P, Fang Q, Jin H, Ji Y, Gong Z, Dong J (2022) Coordinated design of PSS and STATCOM-POD based on the GA-PSO algorithm to improve the stability of wind-PV-thermal-bundled power system, International Journal of Electrical Power & Energy Systems, Volume 141. ISSN 108208:0142–0615. https://doi.org/10.1016/j.ijepes.2022.108208
Hochreiter S, Schmidhuber J (1997) Long short-term memory. Neural Computation 9(8):1735–1780. https://doi.org/10.1162/neco.1997.9.8.1735
Huang Y, Li Y, Zhang Z, Sun Q (2023) A novel path planning approach for AUV based on improved whale optimization algorithm using segment learning and adaptive operator selection, Ocean Engineering, Volume 280. ISSN 114591:0029–8018. https://doi.org/10.1016/j.oceaneng.2023.114591
Khamees AK, Abdelaziz AY, Ali ZM, Alharthi MM, Ghoneim SS, Eskaros MR, Attia MA (2022) Mixture probability distribution functions using novel metaheuristic method in wind speed modeling, Ain Shams Engineering Journal, 13(3). ISSN 101613:2090–4479. https://doi.org/10.1016/j.asej.2021.10.008
Li J, Song Z, Wang X, Wang Y, Jia Y (2022) A novel offshore wind farm typhoon wind speed prediction model based on PSO–Bi-LSTM improved by VMD, Energy, Volume 251. ISSN 123848:0360–5442. https://doi.org/10.1016/j.energy.2022.123848
Li H, You X, Liu S (2023) Multi-ant colony optimization algorithm based on finite history archiving and boxed pigs game, Applied Soft Computing, Volume 138. ISSN 110193:1568–4946. https://doi.org/10.1016/j.asoc.2023.110193
Liu J, Han C (2023) Design and optimization of heat extraction section in energy tunnel using simulated annealing algorithm, Renewable Energy, Volume 213. ISSN 218–232:0960–1481. https://doi.org/10.1016/j.renene.2023.05.135
Liu L, Wang Q, Wang J, Liu M (2014) A rolling grey model optimized by particle swarm optimization in economic prediction. Comput Intell 32(3):391–419. https://doi.org/10.1111/coin.12059
Liu X, Lin Z, Feng Z (2021) Short-term offshore wind speed forecast by seasonal ARIMA - aA comparison against GRU and LSTM, Energy, Volume 227. ISSN 120492:0360–5442. https://doi.org/10.1016/j.energy.2021.120492
Luo J, Gong Y (2023) Air pollutant prediction based on ARIMA-WOA-LSTM model, Atmospheric Pollution Research, 14(6). ISSN 101761:1309–1042. https://doi.org/10.1016/j.apr.2023.101761
May R (1976) Simple mathematical models with very complicated dynamics. Nature 261:459–467. https://doi.org/10.1038/261459a0
Mirjalili S (2015) Moth-flame optimization algorithm: A a novel nature-inspired heuristic paradigm, Knowledge-Based Systems, Volume 89. ISSN 228–249:0950–7051. https://doi.org/10.1016/j.knosys.2015.07.006
Nguyen THT, Phan QB (2022) Hourly day ahead wind speed forecasting based on a hybrid model of EEMD, CNN-Bi-LSTM embedded with GA optimization, Energy Reports, Volume 8, Supplement 10. ISSN 53–60:2352–4847. https://doi.org/10.1016/j.egyr.2022.05.110
Pan S, Yang B, Wang S, Guo Z, Wang L, Liu J, Wu S (2021) Oil well production prediction based on CNN-LSTM model with self-attention mechanism, Energy, Volume 284. ISSN 128701:0360–5442. https://doi.org/10.1016/j.energy.2023.128701
Patidar H, Shende V, Baredar P et al (2023) Comparative evaluation of optimal Weibull parameters for wind power predictions using numerical and metaheuristic optimization methods for different Indian terrains. Environ Sci Pollut Res 30:30874–30891. https://doi.org/10.1007/s11356-022-24395-6
Pedrycz W (1991) Neurocomputations in relational systems. IEEE Trans Pattern Anal Mach Intell 13(3):289–297. https://doi.org/10.1109/34.75517
Qian Y, Wang J, Zhang H et al (2023) Research of a combination system based on fuzzy sets and multi-objective marine predator algorithm for point and interval prediction of wind speed. Environ Sci Pollut Res 30:35781–35807. https://doi.org/10.1007/s11356-022-23773-4
Rahmatian M, Nazarian A, Moeini S (2023) Single-objective optimization design of convergent-divergent ducts of ducted wind turbine using RSM and GA, to increase power coefficient of a small-scale horizontal axis wind turbine, Energy, Volume 269. ISSN 126822:0360–5442. https://doi.org/10.1016/j.energy.2023.126822
Santos VO, Rocha PAC, Scott J, Thé JVG, Gharabaghi B (2023) Spatiotemporal analysis of bidimensional wind speed forecasting: dDevelopment and thorough assessment of LSTM and ensemble graph neural networks on the Dutch database, Energy, Volume 278, Part A. ISSN 127852:0360–5442. https://doi.org/10.1016/j.energy.2023.127852
Singh M, Singh U (2020) An optimized moth-flame optimization algorithm for solving mechanical engineering problems. J Comput Design Eng 7(2):169–175. https://doi.org/10.1016/j.jcde.2019.09.005
Su J, Fu Y, Gao K, Dong H, Mou J (2023) Integrated scheduling problems of open shop and vehicle routing using an ensemble of group teaching optimization and simulated annealing, Swarm and Evolutionary Computation, Volume 83. ISSN 101373:2210–6502. https://doi.org/10.1016/j.swevo.2023.101373
Tang G, Chiclana F, Liu P (2020) A decision-theoretic rough set model with q-rung orthopair fuzzy information and its application in stock investment evaluation, Applied Soft Computing, Volume 91. ISSN 106212:1568–4946. https://doi.org/10.1016/j.asoc.2020.106212
Tang Y, Yu F, Pedrycz W, Yang X, Wang J, Liu S (2022) building trend fuzzy granulation-based LSTM recurrent neural network for long-term time-series forecasting. IEEE Trans Fuzzy Syst 30(6):1599–1613. https://doi.org/10.1109/TFUZZ.2021.3062723
Wang H, Wu M (2018) Global shape optimization of free-form cable-stiffened latticed shell based on local optimal solutions, Engineering Structures, Volume 168. ISSN 576–588:0141–0296. https://doi.org/10.1016/j.engstruct.2018.05.008
Wang JZ, Li YJ, Sun YQ, Li HM, Zhang WG (2019) Multiscale decomposition and fuzzy information granulation LSTM model for wind speed forecasting. Appl Soft Comput 84:105653. https://doi.org/10.1016/j.asoc.2019.105653
Wang J, Li H, Wang Y, Lu H (2021) A hesitant fuzzy wind speed forecasting system with novel defuzzification method and multi-objective optimization algorithm, Expert Systems with Applications, Volume 168. ISSN 114364:0957–4174. https://doi.org/10.1016/j.eswa.2020.114364
Wang J, Wang S, Zeng B, Lu H (2022) A novel ensemble probabilistic forecasting system for uncertainty in wind speed, Applied Energy, Volume 313. ISSN 118796:0306–2619. https://doi.org/10.1016/j.apenergy.2022.118796
Wang J, Zhou Y, Jiang H (2023b) A novel interval system based on multi-objective optimization and hybrid data reconstruct strategy, Expert Systems with Applications, Volume 217. ISSN 119539:0957–4174. https://doi.org/10.1016/j.eswa.2023.119539
Wang K, Hua Y, Huang L, Guo X, Liu X, Ma Z, Ma R, Jiang X (2023c) A novel GA-LSTM-based prediction method of ship energy usage based on the characteristics analysis of operational data, Energy. ISSN 128910:0360–5442. https://doi.org/10.1016/j.energy.2023.128910
Wang W, Wang Q, Zhong R, Chen L, Shi X (2023d) Stacking sequence optimization of arbitrary quadrilateral laminated plates for maximum fundamental frequency by hybrid whale optimization algorithm, Composite Structures, Volume 310. ISSN 116764:0263–8223. https://doi.org/10.1016/j.compstruct.2023.116764
Wang J, Bei J, Song H, Zhang H, Zhang P (2023e) A whale optimization algorithm with combined mutation and removing similarity for global optimization and multilevel thresholding image segmentation. Appl Soft Computing 137:110130. https://doi.org/10.1016/j.asoc.2023.110130
Wang, J, Wang, K, Li, Z, Lu, H, Jiang, H, Xing, Q (2023a) A multitask integrated deep-learning probabilistic prediction for load forecasting, in IEEE Trans Power Syst, https://doi.org/10.1109/TPWRS.2023.3257353
Wei J, Wu X, Yang T, Jiao R (2023) Ultra-short-term forecasting of wind power based on multi-task learning and LSTM, International Journal of Electrical Power & Energy Systems, Volume 149. ISSN 109073:0142–0615. https://doi.org/10.1016/j.ijepes.2023.109073
Wen S, Wang H, Qian J, Men X (2023) A novel combined model based on echo state network optimized by whale optimization algorithm for blast furnace gas prediction. Energy 279:128048. https://doi.org/10.1016/j.energy.2023.128048
Yang B, Wu L, Xiong J, Zhang Y, Chen L (2023) Location and path planning for urban emergency rescue by a hybrid clustering and ant colony algorithm approach, Applied Soft Computing. ISSN 110783:1568–4946. https://doi.org/10.1016/j.asoc.2023.110783
Yuan E, Yang G (2023) SA–EMD–LSTM: a novel hybrid method for long-term prediction of classroom PM25 concentration. Exp Syst Appl 230:120670. https://doi.org/10.1016/j.eswa.2023.120670
Zadeh LA (1965) Fuzzy Sets. Inf Control 8(3):338–353
Zhang Y, Pan G, Chen B, Han J, Zhao Y, Zhang C (2020) Short-term wind speed prediction model based on GA-ANN improved by VMD, Renewable Energy, Volume 156. ISSN 1373–1388:0960–1481. https://doi.org/10.1016/j.renene.2019.12.047
Zhang L, Wang J, Li Z, Zeng B, Huang X (2022) Uncertainty quantification of PM2.5 concentrations using a hybrid model based oncharacteristic decomposition and fuzzy granulation. J Environ Manag 324:116282. https://doi.org/10.1016/j.jenvman.2022.116282
Zhao X, Fang Y, Ma S, Liu Z (2022) Multi-swarm improved moth–flame optimization algorithm with chaotic grouping and Gaussian mutation for solving engineering optimization problems, Expert Systems with Applications, Volume 204. ISSN 117562:0957–4174. https://doi.org/10.1016/j.eswa.2022.117562
Zheng L, Lu W, Zhou Q (2023) Weather image-based short-term dense wind speed forecast with a ConvLSTM-LSTM deep learning model, Building and Environment, Volume 239. ISSN 110446:0360–1323. https://doi.org/10.1016/j.buildenv.2023.110446
Zhou Y, Wang J, Lu H, Zhao W (2022) Short-term wind power prediction optimized by multi-objective dragonfly algorithm based on variational mode decomposition, Chaos, Solitons & Fractals, Volume 157. ISSN 111982:0960–779. https://doi.org/10.1016/j.chaos.2022.111982
Zhu Y (2023) Research on adaptive combined wind speed prediction for each season based on improved gray relational analysis. Environ Sci Pollut Res 30:12317–12347. https://doi.org/10.1007/s11356-022-22957-2
Funding
This work was supported by the General Scientific Research Funding of the Science and Technology Development Fund (FDCT) in Macao under grant 0150/2022/A and the Faculty Research Grants of Macau University of Science and Technology (FRG-22–074-FIE).
Author information
Authors and Affiliations
Contributions
Conceptualization: Jianzhou Wang. Methodology: Jingrui Li. Formal analysis and investigation: Runze Li. Writing—original draft preparation: Runze Li. Writing—review and editing: Jianzhou Wang. Funding acquisition: Jianzhou Wang. Resources: Menggang Kou. Supervision: Jianzhou Wang
Corresponding author
Ethics declarations
Ethics approval
Not applicable
Consent to participate
Not applicable
Consent for publication
Not applicable
Competing interests
The authors declare no competing interests.
Disclosure
We have not submitted our manuscript to a preprint server before submitting it to Environmental Science and Pollution Research.
Additional information
Responsible Editor: Marcus Schulz
Publisher's Note
Springer Nature remains neutral with regard to jurisdictional claims in published maps and institutional affiliations.
Rights and permissions
Springer Nature or its licensor (e.g. a society or other partner) holds exclusive rights to this article under a publishing agreement with the author(s) or other rightsholder(s); author self-archiving of the accepted manuscript version of this article is solely governed by the terms of such publishing agreement and applicable law.
About this article
Cite this article
Li, R., Wang, J., Li, J. et al. Long, short, and medium terms wind speed prediction model based on LSTM optimized by improved moth flame optimization algorithm. Environ Sci Pollut Res 31, 37256–37282 (2024). https://doi.org/10.1007/s11356-024-33580-8
Received:
Accepted:
Published:
Issue Date:
DOI: https://doi.org/10.1007/s11356-024-33580-8