Abstract
The forecast of clean energy power generation is of major prominence to energy structure adjustment and the realization of sustainable economic development in China. In order to scientifically predict clean energy power generation data, a structure-adaptive nonlinear grey Bernoulli model submitted to the new information priority criterion (abbreviated as IANGBM) is established. Firstly, an improved conformable fractional accumulation operator that conforms to the priority of new information is proposed, which can effectively extract the information from small samples. Then, IANGBM is derived from the Bernoulli differential equation, and the perturbation bound theory proves that this model is suitable for the analysis of small sample data. In addition, the grey wolf optimization algorithm is utilized to optimize the model parameters to make the model more adaptable and generalized. To verify the superiority of the model, two cases consisting of wind and nuclear power generation prediction are implemented by comparing eight benchmark models involving IANGBM, GM, FGM, FANGBM, LR, SVM, BPNN, and LSTM. The experiment results demonstrate that the proposed model achieves higher prediction accuracy compared to the other seven competing models. Finally, the future nuclear and wind power generation from 2023 to 2030 are predicted by adopting the IANGBM(1,1) model. For the next 8 years, nuclear power generation will maintain stable development, while wind energy power generation will grow rapidly.












Similar content being viewed by others
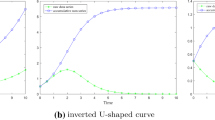
Data availability
The authors confirm that the data supporting the findings of this study are available within the article.
References
Abdel-Basset M, Hawash H, Chakrabortty RK, Ryan M (2021) PV-Net: An innovative deep learning approach for efficient forecasting of short-term photovoltaic energy production. J Clean Prod 303:127037. https://doi.org/10.1016/j.jclepro.2021.127037
Al-qaness MA, Dahou A, Ewees AA, Abualigah L, Huai J, Abd Elaziz M, Helmi AM (2023b) ResInformer: residual transformer-based artificial time-series forecasting model for PM2. 5 concentration in three major Chinese cities. Mathematics 11(2):476. https://doi.org/10.3390/math11020476
Al-qaness MA, Dahou A, Ewees AA, Abualigah L, Huai J, Abd Elaziz M, Helmi AM (2023c) ResInformer: residual transformer-based artificial time-series forecasting model for PM2. 5 concentration in three major Chinese cities. Mathematics 11(2):476. https://doi.org/10.3390/math11020476
Al-qaness MA, Ewees AA, Fan H, Abualigah L, Elsheikh AH, Abd Elaziz M (2023d) Wind power prediction using random vector functional link network with capuchin search algorithm. Ain Shams Eng J 14(9):102095. https://doi.org/10.1016/j.asej.2022.102095
Al-qaness MA, Ewees AA, Thanh HV, AlRassas AM, Abd Elaziz M (2022) An optimized neuro-fuzzy system using advance nature-inspired Aquila and Salp swarm algorithms for smart predictive residual and solubility carbon trapping efficiency in underground storage formations. J Energy Storage 56:106150. https://doi.org/10.1016/j.est.2022.106150
Cai L, Duan J, Lu X, Luo J, Yi B, Wang Y, Jin D, Lu YH, Qiu LY, Chen S, Zhang H, Wang L (2022) Pathways for electric power industry to achieve carbon emissions peak and carbon neutrality based on LEAP model: a case study of state-owned power generation enterprise in China. Comput Ind Eng 170:108334. https://doi.org/10.1016/j.cie.2022.108334
Chen C-I, Chen HL, Chen S-P (2008) Forecasting of foreign exchange rates of Taiwan’s major trading partners by novel nonlinear grey Bernoulli modelNGBM(1,1). Commun Nonlinear Sci Numer Simul 13(6):1194–1204. https://doi.org/10.1016/j.cnsns.2006.08.008
De Giorgi MG, Congedo PM, Malvoni M (2014) Photovoltaic power forecasting using statistical methods: impact of weather data. IET Sci Meas Technol 8(3):90–97. https://doi.org/10.1049/iet-smt.2013.0135
Deng JL (1982) Control problems of grey systems. Syst Control Lett 1(5):288–294. https://doi.org/10.1016/S0167-6911(82)80025-X
Ding S, Li R, Tao Z (2021) A novel adaptive discrete grey model with time-varying parameters for long-term photovoltaic power generation forecasting. Energy Convers Manag 227:113644. https://doi.org/10.1016/j.enconman.2020.113644
Ding S, Tao Z, Li R, Qin X (2022) A novel seasonal adaptive grey model with the data-restacking technique for monthly renewable energy consumption forecasting. Expert Syst Appl 208:118115. https://doi.org/10.1016/j.eswa.2022.118115
Gao M, Yang H, Xiao Q, Goh M (2022) A novel method for carbon emission forecasting based on Gompertz's law and fractional grey model: evidence from American industrial sector. Renew Energy 181:803–819. https://doi.org/10.1016/j.renene.2021.09.072.
Jamil R (2020) Hydroelectricity consumption forecast for Pakistan using ARIMA modeling and supply-demand analysis for the year 2030. Renew Energy 154:1–10. https://doi.org/10.1016/j.renene.2020.02.117
Jiang J, Wu WZ, Li Q, Zhang Y (2021) A PSO algorithm-based seasonal nonlinear grey Bernoulli model with fractional order accumulation for forecasting quarterly hydropower generation. J Intell Fuzzy Syst 40(1):507–519. https://doi.org/10.3233/JIFS-200113
Li Y, Bai X, Liu B (2022) Forecasting clean energy generation volume in China with a novel fractional time-delay polynomial discrete grey model. Energ Buildings 271:112305. https://doi.org/10.1016/j.enbuild.2022.112305
Lin GQ, Li LL, Tseng ML, Liu HM, Yuan DD, Tan RR (2020) An improved moth-flame optimization algorithm for support vector machine prediction of photovoltaic power generation. J Clean Prod 253:119966. https://doi.org/10.1016/j.jclepro.2020.119966
Liu JF, Liu SF, Wu LF, Fang ZG (2016) Research on fractional order reverse accumulative NHGM (1, 1, k) model and its application. Syst Eng Theory Pract 36(4):1033–1041. https://doi.org/10.12011/1000-6788(2016)04-1033-09
Liu Y, Yang Y, Pan F, Xue D (2022) A conformable fractional unbiased grey model with a flexible structure and it’s application in hydroelectricity consumption prediction. J Clean Prod 367:133029. https://doi.org/10.1016/j.jclepro.2022.133029
Ma H (2022) Prediction of industrial power consumption in Jiangsu Province by regression model of time variable. Energy 239:122093. https://doi.org/10.1016/j.energy.2021.122093
Ma X, Wu W, Zeng B, Wang Y, Wu X (2020) The conformable fractional grey system model. ISA Trans 96:255–271. https://doi.org/10.1016/j.isatra.2019.07.009
Mirjalili S, Mirjalili SM, Lewis A (2014) Grey wolf optimizer. Adv Eng Softw 69:46–61. https://doi.org/10.1016/j.advengsoft.2013.12.007
Pali BS, Vadhera S (2018) A novel pumped hydro-energy storage scheme with wind energy for power generation at constant voltage in rural areas. Renew Energy 127:802–810. https://doi.org/10.1016/j.renene.2018.05.028
Şahin U (2020) Projections of Turkey's electricity generation and installed capacity from total renewable and hydro energy using fractional nonlinear grey Bernoulli model and its reduced forms. Sustain Prod Consum 23:52–62. https://doi.org/10.1016/j.spc.2020.04.004
Su Q, Yan S, Wu L, Zeng X (2022) Online public opinion prediction based on a novel seasonal grey decomposition and ensemble model. Expert Syst Appl 210:118341. https://doi.org/10.1016/j.eswa.2022.118341
Sui A, Qian W (2022) Intelligent grey forecasting model based on periodic aggregation generating operator and its application in forecasting clean energy. Expert Syst 39(3):e12868. https://doi.org/10.1111/exsy.12868
Wang H, Zhang Z (2022) A novel grey model with conformable fractional opposite-direction accumulation and its application. Appl Math Model 108:585–611. https://doi.org/10.1016/j.apm.2022.04.020
Wang K, Qi X, Liu H (2019) A comparison of day-ahead photovoltaic power forecasting models based on deep learning neural network. Appl Energy 251:113315. https://doi.org/10.1016/j.apenergy.2019.113315
Wang Y, Chi P, Nie R, Ma X, Wu W, Guo B (2022a) Self-adaptive discrete grey model based on a novel fractional order reverse accumulation sequence and its application in forecasting clean energy power generation in China. Energy 253:124093. https://doi.org/10.1016/j.energy.2022.124093
Wang Y, H. & Lu, J. (2020) Improvement and application of GM (1, 1) model based on multivariable dynamic optimization. J Syst Eng Electron 31(3):593–601. https://doi.org/10.23919/JSEE.2020.000024
Wang Y, He X, Zhang L, Ma X, Wu W, Nie R, Chi P, Zhang Y (2022b) A novel fractional time-delayed grey Bernoulli forecasting model and its application for the energy production and consumption prediction. Eng Appl Artif Intel 110:104683. https://doi.org/10.1016/j.engappai.2022.104683
Wang Y, Yang Z, Wang L, Ma X, Wu W, Ye L, Zhou Y, Luo Y (2022c) Forecasting China's energy production and consumption based on a novel structural adaptive Caputo fractional grey prediction model. Energy 259:124935. https://doi.org/10.1016/j.energy.2022.124935
Wu L, Liu S, Yao L, Yan S, Liu D (2013) Grey system model with the fractional order accumulation. Commun Nonlinear Sci Numer Simul 18(7):1775–1785. https://doi.org/10.1016/j.cnsns.2012.11.017
Wu LF, Liu SF, Yao LG (2015) Grey model with Caputo fractional order derivative. Syst Eng Theory Pract 35(5):1311–1316. https://doi.org/10.1016/j.chaos.2020.110285
Wu W, Ma X, Zeng B, Lv W, Wang Y, Li W (2020a) A novel grey Bernoulli model for short-term natural gas consumption forecasting. Appl Math Model 84:393–404. https://doi.org/10.1016/j.apm.2020.04.006
Wu W, Ma X, Zhang Y, Li W, Wang Y (2020b) A novel conformable fractional non-homogeneous grey model for forecasting carbon dioxide emissions of BRICS countries. Sci Total Environ 707:135447. https://doi.org/10.1016/j.scitotenv.2019.135447
Xie W, Yu G (2020) A novel conformable fractional nonlinear grey Bernoulli model and its application. Complexity 2020:1076–2787. https://doi.org/10.1155/2020/9178098
Xu Z, Liu L, Wu L (2021) Forecasting the carbon dioxide emissions in 53 countries and regions using a non-equigap grey model. Environ Sci Pollut Res 28(13):15659–15672. https://doi.org/10.1007/s11356-020-11638-7
Xue C, Shahbaz M, Ahmed Z, Ahmad M, Sinha A (2022) Clean energy consumption, economic growth, and environmental sustainability: what is the role of economic policy uncertainty? Renew Energy 184:899–907. https://doi.org/10.1016/j.renene.2021.12.006
Yan B, Mu R, Guo J, Liu Y, Tang J, Wang H (2022) Flood risk analysis of reservoirs based on full-series ARIMA model under climate change. J Hydrol 610:127979. https://doi.org/10.1016/j.jhydrol.2022.127979
Yan C, Wu LF, Liu LY, Zhang K (2020) Fractional Hausdorff grey model and its properties. Chaos Solitons Fractals 138:109915. https://doi.org/10.1016/j.chaos.2020.109915
Yildiz C, Acikgoz H, Korkmaz D, Budak U (2021) An improved residual-based convolutional neural network for very short-term wind power forecasting. Energy Convers Manag 228:113731. https://doi.org/10.1016/j.enconman.2020.113731
Zeng B, Ma X, Zhou M (2020) A new-structure grey Verhulst model for China’s tight gas production forecasting. Appl Soft Comput 96:106600. https://doi.org/10.1016/j.asoc.2020.106600
Zhang H, Ma R, Cui L, Tian G, Ma Z (2022) Exploring the impacts of energy and environmental constraints on China’s urbanization process. Comput Ind Eng 169:108170. https://doi.org/10.1016/j.cie.2022.108170
Zheng C, Wu WZ, Xie W, Li Q, Zhang T (2021) Forecasting the hydroelectricity consumption of China by using a novel unbiased nonlinear grey Bernoulli model. J Clean Prod 278:123903. https://doi.org/10.1016/j.jclepro.2020.123903
Acknowledgements
We would like to thank the editor and the anonymous reviewers for their helpful comments.
Funding
This work is supported by the National Natural Science Foundation of China (Nos. 72371194).
Author information
Authors and Affiliations
Contributions
Jiangxin Xiao: methodology, data curation, writing—original draft, visualization, formal analysis; Xinping Xiao: conceptualization, methodology, supervision, writing—review and editing
Corresponding author
Ethics declarations
Ethics approval and consent to participate
There is no ethical approval and patient consent to participate is required for this study.
Consent for publication
The authors confirm that the final version of the manuscript has been reviewed, approved, and consented for publication by all authors.
Competing interests
The authors declare no competing interests.
Additional information
Responsible Editor: Roula Inglesi-Lotz
Publisher’s note
Springer Nature remains neutral with regard to jurisdictional claims in published maps and institutional affiliations.
Rights and permissions
Springer Nature or its licensor (e.g. a society or other partner) holds exclusive rights to this article under a publishing agreement with the author(s) or other rightsholder(s); author self-archiving of the accepted manuscript version of this article is solely governed by the terms of such publishing agreement and applicable law.
About this article
Cite this article
Xiao, ., Xiao, X. Forecast of clean energy generation in China based on new information priority nonlinear grey Bernoulli model. Environ Sci Pollut Res 30, 110220–110239 (2023). https://doi.org/10.1007/s11356-023-30035-4
Received:
Accepted:
Published:
Issue Date:
DOI: https://doi.org/10.1007/s11356-023-30035-4