Abstract
This paper analyzes whether and how attending an internship during tertiary education affects income. We address endogeneity with an IV approach that exploits information regarding whether the internship was a mandatory component of the study. We further address selection into programs with mandatory internship by using the share of mandatory internships at the closest university, exploiting the low mobility of Swiss students. The results show that internships increase graduates’ incomes. We explore potential mechanisms for the effect of internships on income, finding that general human capital is the main mechanism rather than firm- or field-specific human capital, signaling, or screening. These results indicate that students should continue to invest in internships and that mandatory internships have a place in university curricula because they improve the quality of education.
Similar content being viewed by others
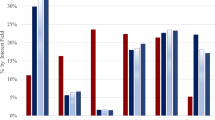
Avoid common mistakes on your manuscript.
Introduction
In response to criticism that they put too little emphasis on soft skills and experience (e.g., Boyce et al., 2001; Hancock et al., 2009) tertiary education institutions have addressed the issue by introducing (mandatory) internships (e.g. Silva et al., 2018). This method is particularly popular because internships do not prolong study time when they are part of the curriculum. In addition, internships provide structured learning at the workplace, which is not possible with student jobs. However, it is not clear from the evidence whether the investment in internships will pay off.
Moreover, the mechanism by which internships should affect employment outcomes are also unclear. Human capital theory assumes that students acquire skills during internships, increasing their productivity and thus employability (Becker, 1964). Those skills can be either general or specific to the company or occupational field of the internship. Alternatively, signaling and selection theories (Spence, 1973; Stiglitz, 1975) postulate that the internship helps the student communicate their skills to potential employers, reducing information asymmetries. Finally, Granovetter (1973) proposes that internships affect employment through the social capital and networks students develop in the workplace.
The question of internships’ impact and the mechanism through which it operates is of central importance for decision-makers in politics and universities. If the influence of internships comes from signaling or selection effects, then it is less important for universities to mandate or provide internships. However, if the effect operates through human capital, then universities can improve the quality of the education they offer by making internships a larger part of the curriculum.
Thus far, there is conflicting evidence on the effect of internships on income and employment, and scant information on the channel through which effects might flow. While a rich literature analyzes the perceived benefits of internships (e.g. Beck & Halim, 2008; Shoenfelt et al., 2013) and correlations between internships and labor market outcomes (e.g. Gault et al., 2000; Reimer & Schröder, 2006; Shaw, 2012), far fewer papers use identification strategies that can tackle the issue of self-selection. Klein and Weiss (2011) and Weiss et al. (2014) find no wage effects using a propensity score matching approach based on mandatory internships, while Margaryan et al. (2019) find positive wage effects based on changes in mandatory internships. McKenzie et al. (2016) evaluate a randomized experiment and find a 73% income increase from an internship program in Yemen. Nunley et al. (2016) exploit data from a résumé audit, suggesting that internship experience increases interview requests, as do Baert (2019). Both studies try to identify the channel through which internships create effects and whereas Nunley et al. attribute them to signaling (Spence, 1973), Baert (2019) find weak evidence for both human capital and signaling.
This paper analyzes the impact of internships during tertiary education on incomes 1 and 5 years after graduation, then explores which of the signaling, screening and human capital explanations for internships’ effects is supported by the evidence. We use data from three waves of the Swiss Graduate Survey, which contains information on graduates’ personal characteristics, education, transition to work, and current employment. To identify the effect of internship we first follow Margaryan et al. (2019) by exploiting variation in internships being mandatory for graduation. Since the presence of mandatory internships might affect the student’s choice of university department, we go further and take the average of mandatory internships at the closest university that offers a particular program as an instrument, exploiting the low mobility of Swiss students.
Our results show that completing a mandatory internship increases graduates’ income. We find evidence that the main mechanism is human capital, not signaling nor screening, and further that it is general human capital rather than the field- or firm-specific types. This contradicts the literature on experience, which often attribute benefits to more specific types of human capital. Overall, we find internships to have a positive impact on income because they foster general human capital accumulation. Since general human capital is related to soft skills and experience overall—rather than in one particular field or company, internships appear to be achieving their purpose. Furthermore, since internships affect incomes by increasing graduates’ skill level, they are an important part of university curricula.
We make three main contributions to the literature. First, we show that mandatory internships causally increase post-graduation incomes for university students, accounting for self-selection using an IV identification approach. Second, we explore the mechanism by which internships affect income and find evidence that general human capital is the primary channel through which internships affect incomes. Finally, this study uses various labor market outcomes on real data at two time points post-graduation, which is an extension of previous work relying on interview requests (i.e. Nunley et al., 2016).
Theoretical Background and Hypotheses
Education systems build human capital in societies by preparing participants for employment (Klieme et al., 2007). Therefore—in addition to many other purposes—the programs in an education system must impart knowledge and skills (human capital) that can help individuals be employable and productive in a firm. Although soft skills are in strong and increasing demand on the labor market (Deming, 2017; Salvisberg, 2010) and are important for success in life (Heckman & Kautz, 2012), they are insufficiently transmitted by tertiary education. Universities’ attempts to provide those skills have had limited results (Blackwell et al., 2001; Cranmer, 2006). Soft skills seem to require different learning processes than hard skills do (Raelin, 1997), though only limited evidence exists on the optimal learning environment of soft skills (e.g. Brunello & Schlotter, 2011; Shepherd, 1998).
Employers complain that tertiary education graduates do not always bring the right skills with them, especially when it comes to soft skills (Matsouka, 2016) and experience (Kavanagh & Drennan, 2008). However, employers can invest in and produce human capital just as educational institutions can (Becker, 1964). In fact, employers have a competitive advantage as providers of some skill types—the practical knowledge of experience is only gained at work, and Bolli and Renold (2017) show that soft skills are better learned in workplaces than in schools. This is the insight behind Wilson’s (2012) recommendation of facilitating tertiary graduates’ labor-market entry by equipping them with experience during their studies. His suggestions for providing experience are sandwich degree programs, internships, and work-based degree programs in tertiary education.
Various studies suggest that doing an internship during tertiary education has a positive impact on both soft skills and experience (e.g. Brooks et al., 1995; Sarcletti, 2009; Helyer & Lee, 2014; Silva et al., 2018). According to Brooks et al. (1995), senior students with internship experience have more work-relevant skills, specific knowledge of their occupation, and clearer career goals. In this line, Sarcletti (2009) argues that internships provide occupation-specific skills, and Helyer and Lee (2014) state that students with internships are more employable because of their experience. Weible (2009) summarizes the benefits of internships for students, employers, and universities. For students, the benefits include higher salaries, shorter job searching, more job offers, and improved skills.
Schambach and Dirks (2002) mention several potential benefits for students doing an internship, such as applying learnt knowledge in practice and reinforcing that knowledge, raising awareness of key soft skills such as communication or teamwork, and clarifying career paths and marketable job skills (experience). Van Belle et al. (2019) use a vignette study to determine what student jobs actually signal to employers, and find that employers assume those with jobs to have better work attitudes, larger social networks, and show more responsibility, motivation, and maturity. To sum up the anecdotal evidence, an internship combines the acquisition of soft skills and experience, thereby enhancing employability and facilitating the transition from tertiary education into the labor market.
Theoretically, an internship is an investment—paid for by accepting low or no wages—in skills that increases an individual’s productivity (human capital theory; Becker, 1964). Higher productivity in turn entails higher income on the labor market. Experience also directly increases income (Mincer, 1974). Internships should increase income through greater human capital and acquired experience. Therefore, we specify our first hypothesis that:
H1
Internships increase income among university graduates.
Existing studies examining the effect of internships on labor market outcomes causally have found mixed evidence for their effect on income. Klein and Weiss (2011) and Weiss et al. (2014) analyze mandatory internships in tertiary education with a propensity-score-matching approach and find no effect on wages 5 years after graduation. McKenzie et al. (2016) also find no significant effect of internships on average monthly income in a randomized experimental setting. In contrast, Margaryan et al.(2019) find substantial effects of internships during studies on graduates’ wage returns (about six percent) using the variation in mandatory internships as an instrument. Nunley et al. (2016) and Baert (2019) find a positive effect on interview rate (14.3% resp. 12.6%) for graduates with experience from internship based on a résumé audit study. These latter studies are in line with our expectations.
There are various channels through which an internship might influence income. Identifying the channel through which internships affect income—if they do so—is our second research question. One possible channel through which internships might work is signaling (Spence, 1973). Employers on the labor market suffer from information asymmetry as they are not able to observe the productivity of graduates before they hire them. Thus, employers look for signals indicating the graduate’s productivity. Completing an internship might serve as such a signal because more able graduates should more easily find an internship position. Furthermore, having had a position at a more prestigious firm also indicates graduate’s motivation and productivity (Sarcletti, 2009).
A second potential channel of internship effects on income is screening (Stiglitz, 1975). In this case, employers use the internship as an opportunity to evaluate potential future employees and to glean information about their actual productivity. This reduces the information asymmetry associated with hiring a worker of unknown productivity. According to Autor (2001) firms provide training to screen future employees and select higher-ability candidates. This could also apply to internships.
A third major channel through which internships might increase income is by improving human capital and thereby the productivity of graduates through the acquisition of skills (Becker, 1964). Becker (1964) differentiates between general human capital and specific human capital. General human capital would be the case where internship graduates learn transferable workplace skills that apply in any field or workplace. Specific human capital is acquired when the internship teaches occupation- or firm-specific skills.
There is a further way for internships to affect graduates’ income based on social network theory (Granovetter, 1973). Here, the idea is that graduates find employment through connections they make during their internships. However, our data does not allow us to test this possibility. We focus on signaling, screening, and human capital leaving the networking case and any other potential channel aside to further research.
According to Spence (1973) it is possible that both the human capital and signaling channels operate simultaneously. Therefore, we formulate hypotheses that specifically identify the expected impact of internships on income in the case of each channel of influence. We further differentiate between specific skills and general skills in the human capital channel.
We begin with the signaling channel. We expect a signaling effect if the internship has an effect on income in the short run, caused by a hiring bump from the signal. We do not expect any effect in the long run because the internship is affecting the graduates’ signaling power but not skills, and because employers have other, more reliable signals like references for experienced candidates. In the long run, there are other ways to signal the skills such as a reference letter of the current employer. If graduates’ and companies’ quality varies, we might expect an effect only for firm switchers (Hopkins, 2012). However, we cannot observe quality, so we do not hypothesize that signaling will systematically increase wages for firm switchers over stayers.
For screening, we also expect a short-term but not long-term effect on graduates’ wages. Like signaling, there is a hiring bump from the signal but no change in productivity, so the effect should not persevere into the long term as employers have other signals at their disposal too. In the screening case, however, we expect the effect to apply only to firm stayers, not firm switchers. The information asymmetry is resolved for the internship firm, but not for a switch firm. Therefore, we formulate the following hypothesis that applies to both signaling and screening:
H2
Internship increases income in the short-run but not in the long run.
If H2 is true, we need to differentiate between signaling and screening. Therefore, we test the following hypotheses when H2 is true, for signaling and screening respectively:
H2a
Internship increases short-run income for firm stayers and firm switchers.
H2b
Internship increases short-run income for firm stayers, not firm switchers.
We differentiate between interns who take post-graduation employment at the firm where they did their internship (firm stayers) and those who take employment at a different firm (firm switchers). This is distinct from employees who stay at or switch firms, because internships are temporary positions. In order for a former intern to become an employee, the firm must offer a position. The internship affects income in that case by making the firm more or less likely to prefer former interns. Therefore, whether the internship increases short-run income for firm stayers and firm switchers can demonstrate whether signaling and screening are important channels through which internships affect income.
There is evidence in the literature for the signaling channel. Weiss et al. (2014) find significant effects of internship on labor market outcomes right after graduation but not in the long run. Therefore, they come to the conclusion that the channel is signaling. Nunley et al. (2016) cite four pieces of evidence from their résumé audit study that support the internship-as-signal concept: no positive interaction of internship with post-graduation work experience, an internship effect size almost as large as that of post-graduate work experience, 4-year-past internships still having an effect, and effects concentrated in the initial stage of the hiring process.
Our third hypothesis concerns the human capital channel generally. If the experience gained in internships increase graduates’ skills and therefore productivity, they should increase their earnings both in the short- and long runs. Therefore, the channel of impact is human capital if:
H3: Internships increase income in the short run and in the long run.
The final set of hypotheses differentiate between general and specific human capital. We test these when H2 is not true and H3 is true. An internship would work through general human capital if the graduate acquires soft skills and experience that are transferable to other firms and other occupations. Calling soft skills general human capital is plausible, as those skills can be transferred to other occupations and firms. For example working in a team or being able to communicate can be used anywhere. Therefore, general skills should be applicable in any firm or field, regardless of firm switching or horizontal mismatch.
H3a
Internships increase income for firm stayers and firm switchers.
H3b
Internships increase income for field stayers and field switchers.
Thus far, there is no causal evidence identifying general human capital as the channel through which internships affect income. Skills gained in an internship may be a mix between general and specific human capital (Stevens, 1994), making them partially transferable to other occupations or firms. In the case where internships provide specific human capital, the skills a graduate gains during their internship are only useful when working in the same field as the one studied, or in the same firm where the internship took place (e.g. Neal, 1999; Sullivan, 2010).
For example, if skills are firm-specific human capital (Derek, 1995), then the skills acquired during an internship are useful in a specific firm, as in the case of proprietary machinery or practices. Accordingly, our hypothesis testing for firm-specific skills looks for income improvement only when graduates remain in the firm where they did their internship, stating:
H3c
Internships increases income for firm stayers, not firm switchers.
The empirical evidence on the effect of experience through firm-specific human capital finds no effect on labor market outcomes (Dustmann & Meghir, 2005; Weber & Falter, 2011).
For field-specific human capital, the graduate would do an internship in an occupation that matches their field of study, then go on to work in that field. This is also in line with the skills-beget-skills approach of Cunha and Heckman (2007), which states that there is a multiplier effect when earlier investments in skills are followed by later investments. In that case, the field of work needs to match the field of study for internships to have positive effects. Our hypothesis to test for occupation-specific human capital is:
H3d
Internships increase income for field stayers, not field switchers.
Evidence on the effect of occupation-specific (also called field-related) human capital on labor market outcomes is plentiful (e.g. Brennan et al., 2002; Sarcletti, 2009; Weber & Falter, 2011; Geel & Backes-Gellner, 2012; Weiss et al., 2014). The only study finding causal evidence for occupation-specific human capital from internships is Weiss et al. (2014). Table 1 summarizes our hypotheses by channel.
Data and Econometric Framework
To test our hypotheses, we analyze data from three waves of the Swiss Graduate Survey.Footnote 1 Our sample includes all Swiss university graduates in 2006, 2008 and 2010, surveyed at 1 and 5 years after graduation—so 2007 and 2011, 2009 and 2013, and 2011 and 2015, respectively. Appendix Table 7 describes all of the variables we use in our estimations and Table 2 provides the corresponding summary statistics.
The Swiss higher education sector includes Universities and Universities of Applied Sciences. We focus on Universities. There are 12 major universities in Switzerland, 10 of which are large cantonal universities (i.e. University of Zurich, University of St. Gallen) and two of which are Federal Institutes of Technology (ETH Zurich and EPFL). All universities are autonomous and publicly funded. Swiss universities follow the Bologna system, offering bachelor’s, master’s, and doctoral degrees. Students have free choice of which university they attend and what subject they study. All students who have completed the appropriate upper-secondary level education can enroll.Footnote 2
Some degree programs require mandatory internships, which range from a few weeks to 6 months. The specific content of the internship varies according to each degree program. Universities often have some partner companies that regularly take interns, but students are typically encouraged to find their own internship places.Footnote 3
The Swiss Graduate Survey, run by the Federal Statistical Office, evaluates higher education graduates’ education and employment participation 1 year after graduation and 5 years after graduation. The data is plausibility checked and includes survey sample weights, but we do not include those because they are mostly unnecessary in causal estimations (Solon et al., 2015). The average response rate 1 year post-graduation is 60% of all higher education graduates. Those who respond and agree to participate in future surveys are contacted again 5 years post-graduation, at which point 70% of that sample respond. Non-response bias is possible, potentially influencing the size of the coefficients in our estimation. However, this potential bias is only a problem in this case if the survey participation of higher education graduates who did internships systematically differs from the participation of those who did not do internships. We see no reason that graduates’ participation should vary according to internship participation.
In order to ensure sample homogeneity, we drop PhD students. Furthermore, we balance the sample by dropping students who are not employed either 1 or 5 years after graduation. We account for selection into employment with a Probit estimation for employment and include the predicted inverse Mills ratio in all estimations. The instrument used in the selection equation is the number of dependent children and its interaction with gender (see, e.g., Schwiebert, 2012; Huber & Mellace, 2014). While this instrument might be endogenous from a theoretical point of view (see, e.g., Fleisher & Rhodes, 1979; Martins, 2001; Mulligan & Rubinstein, 2008), Lee (2009) and Chang (2011) do not find evidence for violations of the identifying assumptions.Footnote 4
Switzerland has two university types: universities of applied sciences and conventional universities. We focus on only the graduates of conventional universities to maintain generalizability. Dropping observations with missing values yields a sample of 8615 total graduates. This is obviously < 3 full cohorts even in a small country like Switzerland. The Swiss Graduate Survey samples a representative portion of every cohort, and reports response rates ranging from approximately 30–60% in the three waves we use.
As shown in Table 2, the dataset is a balanced panel with observations at two points in time, 1 and 5 years after graduation. The table shows the short-run, long-run, and time-invariant summary statistics for the sample.
We use a generalized Mincerian specification that includes work experience (Ashworth et al., 2017), estimating the following OLS estimations with the wage of student i at time after graduation t (= t1, t5) measured in 2015 Swiss Francs as the dependent variable. The year of the survey, y, depends on the wave and the time after graduation:
\({\alpha }_{t}\) represents a fixed effect for the surveys 5 years after graduation. t1 and t5 refer to dummy variables for measurements 1 or 5 years after graduation, respectively. The main coefficients of interest are \({\beta }_{1}\) and \({\beta }_{2}\), which capture the effect of doing an internship 1 year and 5 years after graduation, respectively. Since we are interested in whether the two coefficients differ to test H2 and H3, we also estimate a version of (1) that reveals the difference of the two coefficients:
The vector of observable characteristics, xit, includes age (Mincer, 1974), dummy variables for gender (Altonji & Blank, 1999), having Swiss nationality (Müller & Ramirez, 2009), education of the mother and father (Altonji & Dunn, 1995; Black & Devereux, 2010), and pre-university canton of residence (Leimgruber, 2020). Most importantly, we include two dummy variables indicating whether the student worked while studying and whether or not that job was related to their field of study. These dummies capture unobserved heterogeneity regarding the choice to work while studying.
We include fixed effects for the survey year (\({\alpha }_{y})\), plus time-specific fixed effects for university (\({\alpha }_{tu}\), 13 universities), scientific field (\({\alpha }_{tf}\), 19 fields)Footnote 5 and education level (\({\alpha }_{tl}\), Lizenziat [pre-Bologna Reform university degree], Bachelor, Master). Thereby, we control for different trends of wages across universities, scientific field and education level after graduation (Grave & Goerlitz, 2012; Altonji et al., 2012; Federal Statistical Office [FSO], 2017). In addition to the theoretical justification for their inclusion, all of the observable characteristics and fixed effects covariates have empirically demonstrable effects on income in Switzerland (FSO, 2020). ε denotes the robust error term clustered at the university-field level.
Equation 1 tests H1. Looking at the effects in t = 1 and t = 5 also tests H2 and H3, which rely on whether internships increase wages in the short and long runs. For H2, internships should increase short-run income because they operate through the signaling channel. For H3, internships should increase wages in both the short- and long runs because they work by increasing human capital.
The next step of the analysis consists of analyzing firm switchers and stayers. Firm stayers are students who work in the same firm after graduation until the survey year (SameEmp). Firm switchers change the firm they work for between graduation and the survey year.
H2b and H3d look at the difference in internships’ impact on wages for firm switchers and stayers, while H3b expects no difference between the two groups. In H2b, firm switchers should have greater wage effects from internships because the internship is a signal for potential outside employers. In H3d, firm stayers should have greater wage effects because they use firm-specific human capital. H3b holds that internships effect wages though general human capital, so there should be no difference between firm stayers and switchers. In order to test these hypotheses, we estimate
The last step analyzes field switchers and stayers. Field stayers work in an occupation that is related to the study (Match). Field switchers work in an occupation that is unrelated to the study (Mismatch).
H3a and H3c deal with the difference between working in one’s field of study or in a different field. H3c suggests that internships increase wages more for students who work in a job related to their field of study because the human capital is field-specific. H3b, in contrast, holds that internships generate general human capital and therefore should improve wages regardless of occupational field. We test these hypotheses by estimating
We start our analysis based on the conditional correlations based on formulas (1), (2), (3) and (4). However, these naïve estimates might be biased due selection of students into doing an internship. For example, more affluent students might be less interested in internships because they are less reliant on the salary. This potential selection might bias conditional correlations downward. Another example is that more able students might make more internships because they find it easier to find a suitable internship position. This potential selection might bias conditional correlations upward. Therefore, it remains unclear in which direction the selection of students biases the conditional correlations.
To address the potential endogeneity of doing an internship, we use an instrumental variable approach with two instruments. The first instrument exploits information on how frequent mandatory internships are in each university-field. Following Margaryan et al. (2019), we instrument doing an internship with mandatory internship in the study program tacking advantage of the changes in mandatory internship over time. The intuition of the instrument is that the students have no effect on the share of mandatory internships in a program. At the same time a higher share of mandatory internships in a program increases the probability that a student does an internship.
However, this instrument might be biased because the presence of mandatory internships might affect the choice of university departments. Concretely, students may select universities according to how many mandatory internships are in their program. Margaryan et al. (2019) tackle this issue of mandatory internship in a program affecting the choice of university or study field by providing arguments backed with literature and additional evidence from further data analyses. They find no evidence for such an effect. Despite that effort to show that their instrument is valid and strong, there is still some doubt because—although they went so far as to ask students about their selection criteria when choosing a university—the students may still unconsciously consider mandatory internship for university choice. Therefore, we use a different approach for tackling potential selection of students into universities based on mandatory internships.
Instead of taking Margaryan et al.’s (2019) approach of validating the instrument through additional data, we apply a second instrument that uses the share of mandatory internships at the closest university offering a particular field for the choice of doing an internship. This approach exploits the fact that Swiss students tend to go to the university closest to where they lived before studying (Denzler & Wolter, 2011). Card (1993) also shows that distance to university is a valid instrument for continuing with education using US data. Therefore, the share of mandatory internships at the closest university offering a particular field predicts whether a student makes an internship. At the same time, it remains unaffected by selection of students according to how many mandatory internships are in their program. Hence, it combines information about mandatory internships with the idea that distance affects college choice.
Formally, we estimate 2SLSFootnote 6 estimations that combine Eq. (1) with the following first stages:
and
We report the strength of the two instruments based on the F-statistics of the partial R-square. Therefore, formula (1) becomes
The 2SLS equations for Eqs. 2, 3 and 4 are similar.
In order to test the robustness of our results, we present estimates that exclude the control variables x. Even though it does not show instrumental validity, this robustness test demonstrates that the variation we exploit is orthogonal to the observed control variables.
Table 8 in the appendix shows the full estimates of short-run and long-run effects Appendix Table 9 shows the first-stage estimates of short-run and long-run effects. Appendix Table 10 shows the full estimates of effect heterogeneity for firm switchers and firm stayers. Finally, Tables 14 and 15 shows the first-stage results for field stayers and field switchers.
Results
We start by testing whether internships have an effect on graduates’ wages (H1). We find that, with controls (Appendix Table 7) and accounting for endogeneity with an instrumental variable approach, internships have a 17.0% short-term and 14.9% long-term positive impact on wages. Table 3 shows all estimations. The naïve estimate is indeed biased, showing a downward bias in the short-run where M1 is negative and the IV models are all positive. This might, for example, indicate that more-able students do more internships because they are better able to find satisfactory positions. Taking the IV estimates, we conclude that internships do increase income, accepting H1.
Next, we test whether the effect comes through a signaling/screening or human capital channel by examining whether it affects short-term wages only (H2, signaling/screening) or both short- and long-term wages (H3 human capital). From the same results in Table 3, we reject H2 and accept H3, indicating that the wage impact operates through a human capital channel. Therefore, H2a (signaling) and H2b (screening) are automatically rejected.
To test whether the human capital effect is due to general skills or specific skills, we test the wage effects of internships on firm and field stayers and switchers (H3a and H3b, general skills; H3c and H3d, specific skills). We begin with firm switchers and firm stayers. If both firm stayers and switchers have wage improvements, the mechanism is firm-general human capital (H3a). If firm stayers have positive wage impacts but firm switchers do not, this is evidence for firm-specific human capital (H3c).
Table 4 compares firm switchers and firm stayers. As shown in the descriptive statistics, approximately 6% of interns stay at their host firms in the short run and 3% do so in the long run. With controls and the instrumental variable approach, the effect of internships is stronger for firm switchers, who do better than stayers in the short run (17.3% wage improvement compared to no effect) and do the same in the long run (16.3% improvement and no effect for switchers and stayers, respectively). This supports the general human capital mechanism, but because there is no effect for firm stayers—rather than the predicted similar positive effect—it does not match the hypothesis perfectly. This may be because of the very low number of individuals who are still with their internship firm 5 years after graduation. It may also be driven at least in part by firm switchers accepting outside offers. Once again, the naïve estimation is downward biased in the short-run estimation for firm switchers. Therefore, we reject H3c (firm-specific human capital) and accept H3a that the channel is general human capital with regard to firm-related skills. However, we need to reinforce this finding with field-related skills to complete the analysis and because the results did not match H3a perfectly.
Finally, we examine the difference between field stayers and field switchers to determine whether the human capital effect has to do with occupation- or field-specific skills (H3d) or general skills that apply across occupations or fields (H3b). We expect that internships increase income for field stayers, not field switchers if the skills are field-specific (H3d), and that the effect is positive for both field stayers and switchers if the skills are general (H3b). Table 5 shows the difference in effects depending on field match or mismatch. With controls and the instrumental variable approach, there is no short-term effect for stayers but some advantage for switchers who do an internship. In the long run, both field stayers and switchers are better off for having done an internship (15.4% and 16.1% better, respectively). Therefore, we reject H3c and accept H3a that the main channel is general human capital, not field- or occupation-specific human capital.
Table 6 provides an overview of how the empirical tests allow inference about the channel through which internships affect wages. To summarize, we find that internships do increase income for university graduates. They do so through the human capital channel, by imparting general skills. We do not find evidence for signaling, screening, or specific human capital related to either firm or field.
Comparing the results of our main model 2 to model 3 tests whether students select universities according to how many mandatory internships are in their program, since model 3 only uses variation in the closest university. The results suggest that this type of selection is only minor issue. Furthermore, including observable control variables in model 4 has little effect on the estimates. This suggests that the variation we exploit is orthogonal to these observable characteristics, thereby supporting the validity of the IV estimates.
Conclusions
Internships are increasingly common as universities attempt to ensure that graduates successfully enter the labor market and earn good wages. The justifications for internships range from general real-life experience to gaining specific job-specific, company-specific, or field-specific skills. Students compete to earn internships in prestigious companies to signal their skills and pass internal screenings. Other potential benefits of internships are creating a professional network and, for employers, screening potential workers before committing to hiring them.
This paper analyzes the impact of university internships on income and explores where that effect comes from—through human capital channels such as experience and skills, or through recruiting practices like signaling and screening. We find that university internships do increase income, and that they do so not through screening, signaling, or any specific skills but through general human capital.
These findings are in line with Nunley et al. (2016) and Margaryan et al. (2019) in finding that internships have positive effects on labor market outcomes. However our findings contradict studies that identify signaling as the dominant channel (e.g. Weiss et al., 2014; Nunley et al., 2016). This may be because we look at mandatory internships, which are part of the university curriculum and perhaps therefore of higher quality in terms of skills content. On the other hand, mandatory internships may obscure the signaling channel if firms cannot use them to differentiate among students.
It appears that the skills learned during an internship matter, but more because internships teach young people to function in a workplace than because they teach any detailed process. This may indicate that internships are less valuable for students with any work experience, as it appears that any experience will do. Universities may consider broadening the scope of what students can count for mandatory internship requirements, and may consider waiving those requirements for students who have already worked. In countries with vocational education and training programs that include apprenticeship-style workplace learning, any students who have come from that to university may not need internships as much as their pure-academic peers (Oswald-Egg & Renold, 2019).
We add to the literature by considering degree programs across many universities and fields, rather than one university (Callanan & Benzing, 2004) or one field like business administration (Knouse & Fontenot, 2008), accounting (Beck & Halim, 2008), or marketing (Divine et al., 2007). Because of the high-quality dataset we use, we can consider the effects of internship on income 5 years after graduation and can simultaneously consider and compare various channels.
We do not rule out the networking channel, and look forward to future research where the data enables testing that possibility. We use an instrumental variable approach that satisfactorily accounts for endogeneity, but no instrument is perfect. Instrumental variable approaches generally yield high coefficients, so we prefer to focus on the interpretation of results rather than effects sizes. In addition, these approaches capture local average treatment effects, yielding no information on untreated groups or those far from the margin in the treatment group. Our estimation may be subject to some sample selection bias if the functional form of the selection equation is insufficient for identification.
We control for the key observable factors but cannot completely account for all variation. Our analysis does not measure skills directly, instead developing and testing hypotheses for indications of skills. Finally, our results show that general human capital is the main channel but do not eliminate other channels. We argue that the Swiss data used for this study can be generalized to a global context since Swiss universities and employers are similar to their international peers. However, these findings should be validated in other contexts.
Evaluation of internships’ effects on the labor market and the channel through which internships affect graduates’ success are very important for universities and students. We confirm that internships do increase wages. This tells students it is worthwhile to invest in internships—within reason—and reinforces the demand for internships. We show that internships increase wages by increasing students’ skills. For University decision-makers, this shows that an internship is a valuable learning experience and earns its place in the curriculum. We are able to show that internships cause increased wages and do so through general human capital, extending the rigor and specificity of the current literature on internships and labor market outcomes.
This study has broad implications because Swiss universities are a highly generalizable case. They follow the Bologna Process, making these findings directly applicable to other European countries and strongly applicable to any country where universities deliver bachelor’s, master’s, and doctoral degrees. Swiss students tend to find jobs rather quickly after graduation, reducing the risk of selection bias. Given that relatively many Swiss university students in our sample do internships (57%), the effects we find here may be even stronger in contexts where fewer students gain general human capital through internships.
Mandatory student internships have been introduced widely in many countries with limited knowledge of their actual impact. These internships can be costly for students and universities, so evidence on their returns is highly important. This study shows that a curriculum demanding learning at the workplace is useful for higher education students. Further, we demonstrate that broadly applicable skills are the most important takeaway from these workplace learning experiences, which should discourage university curriculum designers from creating high-cost requirements that severely limit students’ internship choices.
Change history
25 September 2021
The following OA funding note is addressed in the funding section: Open access funding provided by Swiss Federal Institute of Technology Zurich.
Notes
Relying on the non-linearity of the first stage yields qualitatively the same results that are available upon request.
The scientific field corresponds to the 2-digit level of the categories used by the Swiss Statistical Office (SFO, 2018): Theology, Languages and Literature, History and Cultural Sciences, Social Sciences, Interdisciplinary/Other Humanities and Social Sciences, Economics and Business, Law, Exact Sciences, Natural Sciences, Interdisciplinary/Other Exact Sciences and Natural Sciences, Human Medicine, Dentistry, Veterinary Medicine, Pharmacy, Interdisciplinary/Other Medicine and Pharmacy, Construction and Surveying, Mechanical and Electrical Engineering, Agricultural Science and Forestry, Interdisciplinary/Other Engineering, Architecture, Interdisciplinary/Other. Using the 1-digit or 3-digit level yields qualitatively the same results that are available upon request.
Accounting for the non-continuous character of dependent variables yields qualitatively the same results.
References
Altonji, J. G., & Dunn, T. A. (1995). The effects of school and family characteristics on the return to education (No. w5072). National Bureau of Economic Research.
Altonji, J. G., & Blank, R. M. (1999). Race and gender in the labor market. Handbook of Labor Economics, 3, 3143–3259.
Altonji, J. G., Blom, E., & Meghir, C. (2012). Heterogeneity in human capital investments: High school curriculum, college major, and careers. Annual Review of Economics, 4(1), 185–223.
Ashworth, J., Hotz, V., Maurel, A., & Ransom, T. (2017). Changes across cohorts in wage returns to schooling and early work experiences. National Bureau of Economic Research, No. w24160.
Autor, D. H. (2001). Why do temporary help firms provide free general skills training? The Quarterly Journal of Economics, 116(4), 1409–1448.
Baert, S. N. (2019). Student Internships and Employment Opportunities after Graduation: A Field Experiment. Institute for the Study of Labor (IZA), (No. 12183).
Beck, J. E., & Halim, H. (2008). Undergraduate internships in accounting: What and how do Singapore Interns learn from experience? Accounting Education, 17(2), 151–172.
Becker, G. (1964). Human capital. NBER.
Black, S. E., & Devereux, P. J. (2010). Recent developments in intergenerational mobility (No. w15889). National Bureau of Economic Research.
Blackwell, A., Bowes, L., Harvey, L., Hesketh, A. J., & Knight, P. T. (2001). Transforming work experience in higher education. British Educational Research Journal, 27(3), 269–285.
Bolli, T., & Renold, U. (2017). Comparative advantages of school and workplace environment in competence acquisition: Empirical evidence from a survey among professional tertiary education and training students in Switzerland. Evidence-Based HRM: A Global Forum for Empirical Scholarship, 5(1), 6–29.
Boyce, G., Williams, S., Kelly, A., & Yee, H. (2001). Fostering deep and elaborative learning and generic (soft) skill development: The strategic use of case studies in accounting education. Accounting Education: An International Journal, 10(1), 37–60.
Brennan, J., Blasko, Z., Little, B. M., & Woodley, A. (2002). UK graduates and the impact of work experience. Centre for Higher Education Research and Information (CHERI).
Brooks, L., Cornelius, A., Greenfield, E., & Joseph, R. (1995). The relation of career-related work or internship experiences to the career development of college seniors. Journal of Vocational Behavior, 46, 332–349.
Brunello, G., & Schlotter, M. (2011). Non-cognitive Skills and Personality Traits: Labour Market Relevance and Their Development in Education & Training Systems. IZA Discussion Paper(5743).
Callanan, G., & Benzing, C. (2004). Assessing the role of internships in the career‐oriented employment of graduating college students. Education+ Training.
Card, D. (1993). Using geographic variation in college proximity to estimate the return to schooling (No. w4483). National Bureau of Economic Research.
Chang, S.-K. (2011). Simulation estimation of two-tiered dynamic panel Tobit models with an application to the labor supply of married women. Journal of Applied Econometrics, 26, 854–871.
Cranmer, S. (2006). Enhancing graduate employability: Best intentions and mixed outcomes. Studies in Higher Education, 31(2), 169–184.
Cunha, F., & Heckman, J. (2007). The technology of skill formation. American Economic Review, 97(2), 31–47.
Deming, D. J. (2017). The growing importance of social skills in the labor market. The Quarterly Journal of Economics, 132(4), 1593–1640.
Denzler, S., & Wolter, S. (2011). Too Far to Go? Does Distance Determine Study Choice? IZA Discussion Papers, 5712.
Derek, N. (1995). Industry-specific human capital: Evidence from displaced workers. Journal of Labor Economics, 13(4), 653–677.
Divine, R. L., Linrud, J. K., Miller, R. H., & Wilson, J. H. (2007). Required internship programs in marketing: Benefits, challenges and determinants of fit. Marketing Education Review, 17(2), 45–52.
Dustmann, C., & Meghir, C. (2005). Wages, experiences and seniority. Review of Economic Studies, 72(1), 77–108.
FSO (Federal Statistical Office). (2017). Von der Hochschule ins Berufsleben: Ergebnisse zur Schweizer Hochschulabsolventenbefragung der Abschlussjahrgänge 2012 und 2016. Federal Statistical Office.
FSO (Federal Statistical Office. (2020). Standardisiertes Bruttoerwerbseinkommen der Hochschulabsolvent/innen ein Jahr nach Studienabschluss. Federal Statistical Office.
Fleisher, B. M., & Rhodes, G. F. (1979). Fertility, women’s wage rates, and labor supply. The American Economic Review, 69, 14–24.
Gault, J., Redington, J., & Schlager, T. (2000). Undergraduate business internships and career success: Are they related? Journal of Marketing Education, 22(1), 45–53.
Geel, R., & Backes-Gellner, U. (2012). Earning while learning: When and how student employment is beneficial. Labour, 26(3), 313–340.
Granovetter, M. S. (1973). The strength of weak ties. American Journal of Sociology, 78(6), 1360–1380.
Grave, B. S., & Goerlitz, K. (2012). Wage differentials by field of study—The case of German university graduates. Education Economics, 20(3), 284–302.
Hancock, P., Howieson, B., Kavanagh, M., Kent, J., Tempone, I., & Segal, N. (2009). Accounting for the future: More than numbers: A collaborative investigation into the changing skill set for professional accounting graduates over the next ten years and strategies for embedding such skills into professional accounting programs. Australian Learning and Teaching Council.
Heckman, J. J., & Kautz, T. (2012). Hard evidence on soft skills. Labour Economics, 19, 451–464.
Helyer, R., & Lee, D. (2014). The role of work experience in the future employability of higher education graduates. Higher Education Quarterly, 68(3), 348–372.
Hopkins, E. (2012). Job market signaling of relative position, or Becker married to Spence. Journal of the European Economic Association, 10(2), 290–322.
Huber, M., & Mellace, G. (2014). Testing exclusion restrictions and additive separability in sample selection models. Empirical Economics, 47(1), 75–92.
Kavanagh, M. H., & Drennan, L. (2008). What skills and attributes does an accounting graduate need? Evidence from student perceptions and employer expectations. Accounting & Finance, 48, 279–300.
Kleibergen, F., & Paap, R. (2006). Generalized reduced rank tests using the singular value decomposition. Journal of Econometrics, 133, 97–126.
Klein, M., & Weiss, F. (2011). Is forcing them worth the effort? Benefits of mandatory internships for graduates from diverse family backgrounds at labour market entry. Studies in Higher Education, 36(8), 969–987.
Klieme, E., Avenarius, H., Baethge, M., Döbert, H., Hetmeier, H.-W., Meister-Scheufelen, G., Rauschenbach, T., & Wolter, A. (2007). Grundkonzeption der Bildungsberichterstattung für Deutschland. In H.-H. Krüger, T. Rauschenbach, & U. Sander (Eds.), Bildungs- und Sozialberichterstattung (pp. 129–145). VS Verlag für Sozialwissenschaften.
Knouse, S. B., & Fontenot, G. (2008). Benefits of the business college internship: A research review. Journal of Employment Counseling, 45(2), 61–66.
Lee, D. S. (2009). Training, wages, and sample selection: Estimating sharp bounds on treatment effects. Review of Economic Studies, 76, 1071–1102.
Leimgruber, W. (2020). Rich country—‘Poor’ regions: Fighting regional disparities in Switzerland. In E. Nel & S. Pelc (Eds.), Responses to geographical marginality and marginalization. Perspectives on geographical marginality (Vol. 5). Cham: Springer. https://doi.org/10.1007/978-3-030-51342-9_4.
Margaryan, S., Saniter, N., Schumann, M., & Siedler, T. (2019). Do Internships Pay Off? The Effects of Student Internships on Earnings. IZA Dicussion Paper No. 12478.
Martins, M. (2001). Parametric and semiparametric estimation of sample selection models: An empirical application to the female labour force in Portugal. Journal of Applied Econometrics, 16, 23–39.
Matsouka, K. (2016). Graduates’ employability: What do graduates and employers think? Industry and Higher Education, 30(5), 321–326.
McKenzie, D., Assaf, N., & Cusolito, A. P. (2016). The demand for, and impact of, youth internships: Evidence from a randomized experiment in Yemen. IZA Journal of Labor and Development, 5(1), 1–15.
Mincer, J. (1974). Schooling, experience, and earnings. National Bureau of Economic Research.
Müller, T., & Ramirez, J. (2009). Wage inequality and segregation between native and immigrant workers in Switzerland: Evidence using matched employee-employer data. Research on Economic Inequality, 17, 205–243.
Mulligan, C. B., & Rubinstein, Y. (2008). Selection, investment, and women’s relative wages over time. Quarterly Journal of Economics, 123, 1061–1110.
Neal, D. (1999). The complexity of job mobility among young men. Journal of Labor Economics, 17(2), 237–261.
Nunley, J. M., Pugh, A., Romero, N., & Seals, R. A. (2016). College major, internship experience, and employment opportunities: Estimates from a résumé audit. Labour Economics, 38, 37–46.
Oswald-Egg, M. E., & Renold, U. (2019). No Experience, No Employment: The Effect of Work. KOF Working Papers. Manuscript in preparation.
Raelin, J. A. (1997). A model of work-based learning. Organization Science, 8(6), 563–578.
Reimer, D., & Schröder, J. (2006). Tracing the gender wage gap: Income differences between male and female university graduates in Germany. Journal for Labour Market Research, 39(2), 235–253.
Salvisberg, A. (2010). Soft Skills auf dem Arbeitsmarkt: Bedeutung und Wandel. Seismo.
Sarcletti, A. (2009). Die Bedeutung von Praktika und studentischen Erwerbstätigkeiten für den Berufseinstieg bayerischer Hochschulabsolventen. Eine Untersuchung anhand der Daten des Bayerischen Absolventenpanels. Dissertation. Studien zur Hochschulforschung 77. München: Bayerisches Staatsinstitut für Hochschulforschung und Hochschulplanung.
Schambach, T., & Dirks, J. (2002). Student perceptions of internship exerperience. Proceedings of the 17th Annual Conference of the International Academy for Information Management (pp. 1–9).
Schwiebert, J. (2012). Revisiting the Composition of the Female Workforce-A Heckman Selection Model with Endogeneity (No. 502). Diskussionsbeitrag.
SFSO. (2018). Classification of scientific fields. https://www.bfs.admin.ch/bfs/de/home/statistiken/bildung-wissenschaft/nomenklaturen/fkatuni.assetdetail.7166845.html
Shaw, A. (2012). The value of work experience in outcomes for students: An investigation into the importance of work experience in the lives of female undergraduates and postgraduate job seekers. Journal of Vocational Education and Training, 64(2), 155–168.
Shepherd, I. (1998). Work experience: Who needs it? Journal of Geography in Higher Education, 22(1), 135–145.
Shoenfelt, E. L., Stone, N. J., & Kottke, J. L. (2013). Internships: An established mechanism. Industrial and Organizational Psychology, 6(1), 24–28.
Silva, P., Lopes, B., Costa, M., Melo, A. I., Dias, G. P., Brito, E., & Seabra, D. (2018). The million-dollar question: Can internships boost employment? Studies in Higher Education, 43(1), 2–21.
Solon, G., Haider, S. J., & Wooldridge, J. M. (2015). What are we weighting for? Journal of Human Resources, 50(2), 301–316.
Spence, M. (1973). Job market signaling. The Quarterly Journal of Economics, 87(3), 355–374.
Stevens, M. (1994). A theoretical model of on-the-job training with imperfect competition. Oxford Economic Papers, 46(4), 537–562.
Stiglitz, J. E. (1975). The theory of "Screening, " education, and the distribution of income. The American Economic Review, 65(3), 283–300.
Stock, J. H., & Yogo, M. (2005). Testing for weak instruments in linear IV regression. In D. W. K. Andrews & J. H. Stock (Eds.), Identification and inference for econometric models: Essays in honor of Thomas Rothenberg (pp. 80–108). Cambridge University Press.
Sullivan, P. (2010). Empirical evidence on occupation and industry specific human capital. Labour Economics, 17(3), 567–580.
Van Belle, E., Caers, R., Cuypers, L., De Couck, M., Neyt, B., Van Borm, H., & Baert, S. (2019). What do student jobs on graduate CVs signal to employers? GLO Discussion Paper, No. 356.
Weber, S., & Falter, J.-M. (2011). General education, vocational education, and wage growth over the lifecycle. Available at SSRN: https://ssrn.com/abstract=1543906 or https://doi.org/10.2139/ssrn.1543906.
Weible, R. (2009). Are universities reaping the available benefits internship programs offer? Journal of Education for Business, 85(2), 59–63.
Weiss, F., Klein, M., & Grauenhorst, T. (2014). The effects of work experience during higher education on labour market entry: Learning by doing or an entry ticket? Work, Employment & Society, 28(5), 788–807.
Wilson, T. (2012). A review of business-University Collaboration. Department for Business, Energy & Industrial Strategy, UK.
Acknowledgements
We are grateful to the participants in the KOF Brownbag Seminar, the COPE conference, and to the reviewers for their invaluable feedback.
Funding
Open Access funding provided by ETH Zurich. Open access funding provided by Swiss Federal Institute of Technology Zurich.
Author information
Authors and Affiliations
Corresponding author
Additional information
Publisher's Note
Springer Nature remains neutral with regard to jurisdictional claims in published maps and institutional affiliations.
Appendix
Appendix
See Tables 7, 8, 9, 10, 11, 12, 13, 14 and 15.
Rights and permissions
Open Access This article is licensed under a Creative Commons Attribution 4.0 International License, which permits use, sharing, adaptation, distribution and reproduction in any medium or format, as long as you give appropriate credit to the original author(s) and the source, provide a link to the Creative Commons licence, and indicate if changes were made. The images or other third party material in this article are included in the article's Creative Commons licence, unless indicated otherwise in a credit line to the material. If material is not included in the article's Creative Commons licence and your intended use is not permitted by statutory regulation or exceeds the permitted use, you will need to obtain permission directly from the copyright holder. To view a copy of this licence, visit http://creativecommons.org/licenses/by/4.0/.
About this article
Cite this article
Bolli, T., Caves, K. & Oswald-Egg, M.E. Valuable Experience: How University Internships Affect Graduates’ Income. Res High Educ 62, 1198–1247 (2021). https://doi.org/10.1007/s11162-021-09637-9
Received:
Accepted:
Published:
Issue Date:
DOI: https://doi.org/10.1007/s11162-021-09637-9