Abstract
This paper serves as an introduction to the special issue of Prevention Science entitled, “Innovations and Applications of Integrative Data Analysis (IDA) and Related Data Harmonization Procedures in Prevention Science.” This special issue includes a collection of original papers from multiple disciplines that apply individual-level data synthesis methodologies, including IDA, individual participant meta-analysis, and other related methods to harmonize and integrate multiple datasets from intervention trials of the same or similar interventions. This work builds on a series of papers appearing in a prior Prevention Science special issue, entitled “Who Benefits from Programs to Prevent Adolescent Depression?” (Howe, Pantin, & Perrino, 2018). Since the publication of this prior work, the use of individual-level data synthesis has increased considerably in and outside of prevention. As such, there is a need for an update on current and future directions in IDA, with careful consideration of innovations and applications of these methods to fill important research gaps in prevention science. The papers in this issue are organized into two broad categories of (1) evidence synthesis papers that apply best practices in data harmonization and individual-level data synthesis and (2) new and emerging design, psychometric, and methodological issues and solutions. This collection of original papers is followed by two invited commentaries which provide insight and important reflections on the field and future directions for prevention science.
Similar content being viewed by others
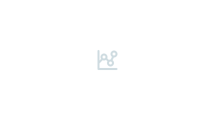
References
Ayer, L., Stevens, C., Reider, E., Sims, B., Colpe, L., & Pearson, J. (2023). Preventing youth suicide: Potential “crossover effects” of existing school-based programs. Prevention Science, 24, 382–392.
Barker, D. H., Bie, R., & Steingrimsson, J. (2023). Addressing systematic missing data in the context of causally interpretable meta-analysis. Prevention Science.
Bauer, D. J. (2017). A more general model for testing measurement invariance and differential item functioning. Psychological Methods, 22, 507–.
Bauer, D. J., & Hussong, A. M. (2009). Psychometric approaches for developing commensurate measures across independent studies: Traditional and new models. Psychological Methods, 14, 101–.
Berry, V., Melendez-Torres, G. J., Axford, N., Axberg, U., de Castro, B. O., Gardner, F., & Leijten, P. (2022). Does social and economic disadvantage predict lower engagement with parenting interventions? An integrative analysis using individual participant data. Prevention Science.
Brincks, A., Perrino, T., Howe, G., Pantin, H., Prado, G., Huang, S., & Brown, C. H. (2018). Preventing youth internalizing symptoms through the Familias Unidas intervention: Examining variation in response. Prevention Science, 19, 49–59.
Brown, C. H., Brincks, A., Huang, S., Perrino, T., Cruden, G., Pantin, H., & Sandler, I. (2018). Two-year impact of prevention programs on adolescent depression: An integrative data analysis approach. Prevention Science, 19, 74–94.
Cole, V. T., Hussong, A. M., Gottfredson, N. C., Bauer, D. J., & Curran, P. J. (2022). Informing harmonization decisions in integrative data analysis: Exploring the measurement multiverse. Prevention Science.
Connell, A. M., Seidman, S., Ha, T., Stormshak, E., Westling, E., Wilson, M., & Shaw, D. (2022). Long-term effects of the family check-up on suicidality in childhood and adolescence: integrative data analysis of three randomized trials. Prevention Science.
Connell, A. M., Stormshak, E., Dishion, T., Fosco, G., & Van Ryzin, M. (2018). The Family Check Up and adolescent depression: An examination of treatment responders and non-responders. Prevention Science, 19, 16–26.
Cooper, H., & Patall, E. A. (2009). The relative benefits of meta-analysis conducted with individual participant data versus aggregated data. Psychological Methods, 14, 165–.
Curran, P. J. (2009). The seemingly quixotic pursuit of a cumulative psychological science: Introduction to the special issue. Psychological Methods, 14, 77.
Curran, P. J., Hussong, A. M., Cai, L., Huang, W., Chassin, L., Sher, K. J., & Zucker, R. A. (2008). Pooling data from multiple longitudinal studies: The role of item response theory in integrative data analysis. Developmental Psychology, 44, 365–.
Dagne, G. A., Brown, C. H., Howe, G., Kellam, S. G., & Liu, L. (2016). Testing moderation in network meta‐analysis with individual participant data. Statistics in Medicine, 35, 2485–2502.
Diener, E., Northcott, R., Zyphur, M., & West, S. G. (2022). Beyond experiments. Perspectives in Psychological Science, 17,1101–1119.
Dong, N., Herman, K. C., Reinke, W. M., Wilson, S. J., & Bradshaw, C. P. (2022). Gender, racial, and socioeconomic disparities on social and behavioral skills for K-8 students with and without interventions: An integrative data analysis of eight cluster randomized trials. Prevention Science.
Garber, J., Weersing, V. R., Hollon, S. D., Porta, G., Clarke, G. N., Dickerson, J. F., & Brent, D. A. (2018). Prevention of depression in at-risk adolescents: Moderators of long-term response. Prevention Science, 19, 6–15.
Gardner, F., Leijten, P., Mann, J., Landau, S., Harris, V., Beecham, J., ... & Scott , S. (2017). Could scale-up of parenting programmes improve child disruptive behaviour and reduce social inequalities? Using individual participant data meta-analysis to establish for whom programmes are effective and cost-effective. Public Health Research, 5(10).
Glass, G. V. (1976). Primary, secondary, and meta-analysis of research. Educational Researcher, 5(10), 3–8.
Glass, G. V. (2000). Meta-analysis at 25.
Goldstein, A. B., & Avenevoli, S. (2018). Strength in numbers. Prevention Science, 19, 109–111.
Gottfredson, N. C., Sterba, S. K., & Jackson, K. M. (2017). Explicating the conditions under which multilevel multiple imputation mitigates bias resulting from random coefficient-dependent missing longitudinal data. Prevention Science, 18, 12–19.
Grant, S., Wendt, K. E., Leadbeater, B. J., Supplee, L. H., Mayo-Wilson, E., Gardner, F., & Bradshaw, C. P. (2022). Transparent, open, and reproducible prevention science. Prevention Science, 23(4), 701–722. https://doi.org/10.1007/s11121-022-01336-w
Hensums, M., De Mooij, B., Kuijper, S. C., Fekkes, M., & Overbeek, G. (2022). What works for whom in school-based anti-bullying interventions? An individual participant data meta-analysis. Prevention Science.
Howe, G. W., & Brown, C. H. (this issue) Retrospective psychometrics and effect heterogeneity in integrated data analysis: Commentary on the special issue. Prevention Science.
Howe, G. W., Pantin, H., & Perrino, T. (2018). Programs for preventing depression in adolescence: Who benefits and who does not? An introduction to the supplemental issue. Prevention Science, 19, 1–5.
Huh, D., Mun, E. Y., Walters, S. T., Zhou, Z., & Atkins, D. C. (2019). A tutorial on individual participant data meta-analysis using Bayesian multilevel modeling to estimate alcohol intervention effects across heterogeneous studies. Addictive Behaviors, 94, 162–170.
Hussong, A. M., Curran, P. J., & Bauer, D. J. (2013). Integrative data analysis in clinical psychology research. Annual Review of Clinical Psychology, 9, 61–89.
Keller, B. T., & Enders, C. K. (2021). Blimp user’s guide (Version 3). Retrieved From www.appliedmissingdata.com/multilevel-imputation.html
Kerr, D. C. R., Reinke, W. M., & Eddy, L. M. (2012). Trajectories of depressive symptoms and externalizing behaviors across adolescence: Associations with histories of suicide attempt and ideation in early adulthood. Suicide and Life-Threatening Behavior, 43(1), 50–66.
Kush, J. M., Masyn, K. E., Amin-Esmaeili, M., Susukida, R., Wilcox, H. C., & Musci, R. J. (2023). Utilizing moderated non-linear factor analysis models for integrative data analysis: A tutorial. Structural Equation Modeling: A Multidisciplinary Journal, 30, 149–164.
Lubinski, D., & Humphreys, L. G. (1996). Seeing the forest from the trees: When predicting the behavior or status of groups, correlate means. Psychology, Public Policy, and Law, 2, 363–376.
MacKinnon, D. P., Valente, M. J., & Gonzalez, O. (2020). The correspondence between causal and traditional mediation analysis: The link is the mediator by treatment interaction. Prevention Science, 21, 147–157.
Magee, K. E., Connell, A., Hipwell, A. E., Shaw, D., Westling, E., Keenan, K., & Stepp, S. (2022). Developmental models of depression, externalizing problems, and self-regulatory processes: Integrated data analysis across four longitudinal studies of youth. Prevention Science.
Mauricio, A. M., Mazza, G. L., Berkel, C., Tein, J. Y., Sandler, I. N., Wolchik, S. A., & Winslow, E. (2018). Attendance trajectory classes among divorced and separated mothers and fathers in the New Beginnings Program. Prevention Science, 19, 620–629.
McDaniel, H. L., Saavedra, L. M., Morgan-López, A. A., Bradshaw, C. P., Lochman, J. E., Kaihoi, C. A., & Yaros, A. C. (2023). Harmonizing social, emotional, and behavioral constructs in prevention science: Digging into the weeds of aligning disparate measures. Prevention Science.
McNeish, D. (2022). Psychometric properties of sum scores and factor scores differ even when their correlation is 0.98: A response to Widaman and Revelle. Behavior Research Methods, 1–22.
Morgan-López, A. A., Hien, D. A., Saraiya, T. C., Saavedra, L. M., Norman, S. B., Killeen, T. K., & Consortium on Addiction, Stress and Trauma (CAST). (2022a). Estimating posttraumatic stress disorder severity in the presence of differential item functioning across populations, comorbidities, and interview measures: Introduction to Project Harmony. Journal of Traumatic Stress, 35, 926–940.
Morgan-López, A. A., McDaniel, H. L., Bradshaw, C. P., Saavedra, L. M., Lochman, J. E., Kaihoi, C. A., & Yaros, A. C. (2022b). Design and methodology for an integrative data analysis of coping power: Direct and indirect effects on adolescent suicidality. Contemporary Clinical Trials, 115, 106705.
Morgan-Lopez, A. A., Saavedra, L. M., Ramirez, D. D., Smith, L. M., & Yaros, A. C. (2022c). Adapting the multilevel model for estimation of the reliable change index (RCI) with multiple timepoints and multiple sources of error. International Journal of Methods in Psychiatric Research, 31(2), e1906.
Morgan‐López, A. A., Saavedra, L. M., Hien, D. A., Norman, S. B., Fitzpatrick, S. S., Ye, A., & Back, S. E. (2023). Differential symptom weighting in estimating empirical thresholds for underlying PTSD severity: Toward a “platinum” standard for diagnosis? International Journal of Methods in Psychiatric Research, e1963.
Mun, E. Y., Jiao, Y., & Xie, M. (2016). Integrative data analysis for research in developmental psychopathology. Developmental Psychopathology: Theory and Method, 1, 1042–1087.
Mun, E. Y., Zhou, Z., Huh, D., Tan, L., Li, D., Tanner-Smith, E. E., ... & Larimer, M. E. (this issue). Brief Alcohol Interventions are Effective through 6 Months: Findings from marginalized zero-inflated poisson and negative binomial models in a two-step IPD meta-analysis. Prevention Science.
Musci, R. J., Kush, J. M., Masyn, K. E., Esmaeili, M. A., Susukida, R., Goulter, N., & Wilcox, H. C. (2023). Psychosis symptom trajectories across childhood and adolescence in three longitudinal studies: An integrative data analysis with mixture modeling. Prevention Science.
Muthén, L. K., & Muthén, B. O. (1998–2017). Mplus user’s guide. Eighth Edition. Los Angeles, CA: Muthén & Muthén.
Pearson, J. L., & Sims, B. E. (2023). Commentary on the special issue “innovations and applications of integrative data analysis (IDA) and related data harmonization procedures in prevention science.” Prevention Science.
Reider, E. E., & Sims, B. E. (2016). Family-based preventive interventions: Can the onset of suicidal ideation and behavior be prevented? Suicide and Life-Threatening Behavior, 46, S3–S7.
Rosenbaum, P. R. (1984). The consequences of adjustment for a concomitant variable that has been affected by the treatment. Journal of the Royal Statistical Society: Series A (general), 147(5), 656–666.
Russell, M. A., Coatsworth, J. D., Brown, A., Zaharakis, N., Mennis, J., Rodriguez, G. C., & Mason, M. J. (2022). Peer network counseling effects on substance use: An individual participant data meta-analysis integrating three randomized controlled trials. Prevention Science.
Saavedra, L. M., Lochman, J. E., Morgan-López, A. A., McDaniel, H. L., Bradshaw, C. P., Powell, N. P., Qu, L., Budavari, A. C., & Yaros, A. C. (in press) Collateral effects of coping power on caregiver symptoms of depression and long-term changes in child behavior. Development and Psychopathology.
Saavedra, L. M., Morgan-Lopez, A. A., Hien, D. A., Lopez-Castro, T., Ruglass, L. M., Back, S. E., & Hamblen, J. (2021). Evaluating treatments for posttraumatic stress disorder, alcohol and other drug use disorders using meta-analysis of individual patient data: Design and methodology of a virtual clinical trial. Contemporary Clinical Trials, 107, 106479.
Saavedra, L. M., Morgan-López, A. A., West, S. G., Alegría, M., & Silverman, W. K. (this issue). Mitigating multiple sources of bias in a quasi-experimental integrative data analysis: Does treating childhood anxiety prevent substance use disorders in late adolescence/young adulthood?. Prevention Science.
Saavedra, L. M., Silverman, W. K., Morgan-Lopez, A. A., & Kurtines, W. M. (2010). Cognitive behavioral treatment for childhood anxiety disorders: Long-term effects on anxiety and secondary disorders in young adulthood. Journal of Child Psychology and Psychiatry, 51, 924–934.
Schweer-Collins, M. L., Parr, N. J., Saitz, R., & Tanner-Smith, E. E. (2023). Investigating for whom brief substance use interventions are most effective: An individual participant data meta-analysis. Prevention Science.
Seidman, S., Connell, A., Stormshak, E., Westling, E., Ha, T., & Shaw, D. (2022). Disrupting maternal transmission of depression: Using integrative data analysis (IDA) to examine indirect effects of the family check-up (FCU) across three randomized trials. Prevention Science.
Siddique, J., de Chavez, P. J., Howe, G., Cruden, G., & Brown, C. H. (2018). Limitations in using multiple imputation to harmonize individual participant data for meta-analysis. Prevention Science, 19, 95–108.
Stewart, L. A., & Parmar, M. K. (1993). Meta-analysis of the literature or of individual patient data: Is there a difference? The Lancet, 341, 418–422.
Stuart, E. A., Cole, S. R., Bradshaw, C. P., & Leaf, P. J. (2011). The use of propensity scores to assess the generalizability of results from randomized trials. Journal of the Royal Statistical Society Series a: Statistics in Society, 174, 369–386.
Tanner-Smith, E., Darlington, T., Gelberg, L., & Saitz, R. (2018). Brief substance use counseling interventions to reduce consumption and consequences among patients in general healthcare settings: Understanding variability in effects. https://www.crd.york.ac.uk/prospero/display_record.php?ID=CRD42018086832
Tanner-Smith, E. E., Grant, S., & Mayo-Wilson, E. (2022). Modern meta-analytic methods in prevention science: Introduction to the special issue. Prevention Science, 23, 341–345.
Tiberio, S. S., Pears, K. C., Buchanan, R., Chamberlain, P., Leve, L. D., Price, J. M., & Hussong, A. M. (2023). An integrative data analysis of main and moderated crossover effects of parent-mediated interventions on depression and anxiety symptoms in youth in foster care. Prevention Science.
Van Buuren, S., & Groothuis-Oudshoorn, K. (2011). mice: Multivariate imputation by chained equations in R. Journal of Statistical Software, 45, 1–67.
Vasilenko, S. A., Odejimi, O. A., Glassman, J. R., Potter, S. C., Drake, P. M., Coyle, K. K., & Clark, L. F. (2022). Who benefits from school-based teen pregnancy prevention programs? Examining multidimensional moderators of program effectiveness across four studies. Prevention Science.
Vidot, D. C., Huang, S., Poma, S., Estrada, Y., Lee, T. K., & Prado, G. (2016). Familias Unidas’ crossover effects on suicidal behaviors among Hispanic adolescents: Results from an effectiveness trial. Suicide and Life-Threatening Behavior, 46, S8–S14.
Wall, M. M., Park, J. Y., & Moustaki, I. (2015). IRT modeling in the presence of zero-inflation with application to psychiatric disorder severity. Applied Psychological Measurement, 39, 583–597.
Zhao, X., Coxe, S., Sibley, M. H., Zulauf-McCurdy, C., & Pettit, J. W. (2022). Harmonizing depression measures across studies: A tutorial for data harmonization. Prevention Science.
Zurovaca, J., Cook, T. D., Deke, J., Finucane, M. M., Chaplin, D., Coopersmith, J. S., & Forrow, L. V. (2021). Absolute and relative bias in eight common observational study designs: Evidence from a meta-analysis. arXiv preprint arXiv:2111.06941
Acknowledgements
This paper benefitted greatly from input and conversations with Drs. Lissette M. Saavedra and Stephen G. West.
Funding
Support for the writing of this paper comes in part from grants from the National Institute of Mental Health (3R01MH124438-03S1 and 3R01MH124438), and the Institute of Education Sciences (R305A220244).
Author information
Authors and Affiliations
Corresponding author
Ethics declarations
Ethics Approval
This article does not include human subjects.
Consent to Participate
This article does not include human subjects.
Conflict of Interest
Co-author Catherine Bradshaw is the editor of the journal Prevention Science, and both Antonio A. Morgan-López and Rashelle Musci are associate editors of Prevention Science; however, another associate editor not involved with this paper managed the peer-review process. The authors have no other conflicts of interests or competing interests to report.
Disclaimer
The views expressed are those of the authors and do not reflect those of either institute.
Additional information
Publisher's Note
Springer Nature remains neutral with regard to jurisdictional claims in published maps and institutional affiliations.
Rights and permissions
Springer Nature or its licensor (e.g. a society or other partner) holds exclusive rights to this article under a publishing agreement with the author(s) or other rightsholder(s); author self-archiving of the accepted manuscript version of this article is solely governed by the terms of such publishing agreement and applicable law.
About this article
Cite this article
Morgan-López, A.A., Bradshaw, C.P. & Musci, R.J. Introduction to the Special Issue on Innovations and Applications of Integrative Data Analysis (IDA) and Related Data Harmonization Procedures in Prevention Science. Prev Sci 24, 1425–1434 (2023). https://doi.org/10.1007/s11121-023-01600-7
Accepted:
Published:
Issue Date:
DOI: https://doi.org/10.1007/s11121-023-01600-7