Abstract
The multi-frequency hybrid signal is an important stimulus from the external environment on the neuronal networks for detection. The mechanism of the detection may be understood by the vibrational resonance, in which the moderate intensity of high-frequency force can amplify the response of neuronal systems to the low-frequency signal. In this paper, the effects of electrical and chemical autapses on signal transmission are investigated in scale-free and small-world neuronal networks, where an external two-frequency signal is introduced only to one neuron as a pacemaker. We observed that the inhibitory autapse can significantly enhance the signal propagation by the vibrational resonance, while the electrical and excitatory autapses typically weaken the signal transmission, indicating that the inhibitory autapse is more beneficial to transmit the rhythm of the pacemaker to the whole networks. These findings contribute to our understanding of signal detection and information processing in the autapic neuronal system.












Similar content being viewed by others
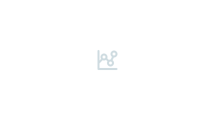
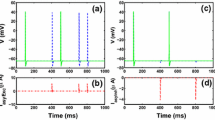
References
Wiesenfeld, K., Moss, F.: Stochastic resonance and the benefits of noise: from ice ages to crayfish and SQUIDs. Nature 373, 33–36 (1995)
Gammaitoni, L., Hanggi, P., Jung, P., Marchesoni, F.: Stochastic resonance. Rev. Mod. Phys. 70, 223 (1998)
Leonard, D.S., Reichl, L.E.: Stochastic resonance in a chemical reaction. Phys. Rev. E 49, 1734 (1994)
Bezrukov, S.M., Voydanoy, I.: Noise-induced enhancement of signal transduction across voltage-dependent ion channels. Nature 378, 362–364 (1995)
Benzi, R., Parisi, G., Sutera, A., Vulpiani, A.: Stochastic resonance in climatic change. Tellus 34, 10–16 (1982)
Stacey, W.C., Durand, D.M.: Stochastic resonance improves signal detection in hippocampal CA1 neurons. J. Neurophysiol. 83, 1394–1402 (2000)
Kaplan, D.T., Clay, J.R., Manning, T., Glass, L., Guevara, M.R., Shrier, A.: Subthreshold dynamics in periodically stimulated squid giant axons. Phys. Rev. Lett. 76, 4074 (1996)
Ozer, M.: Frequency-dependent information coding in neurons with stochastic ion channels for subthreshold periodic forcing. Phys. Lett. A 354, 258–263 (2006)
Ozer, M., Uzuntarla, M., Kayikcioglu, T., Graham, L.J.: Stochastic resonance on Newman-Watts networks of Hodgki-Huxley neurons with local periodic driving. Phys. Lett. A 373, 964–968 (2008)
Yu, Y., Wang, W., Wang, J.F., Liu, F.: Resonance-enhanced signal detection and transduction in the Hodgkin-Huxley neuronal systems. Phys. Rev. E 63, 021907 (2001)
Kawaguchi, M., Mino, H., Durand, D.M.: Stochastic resonance can enhance information transmission in neural networks. IEEE Trans. Biomed. Eng. 58, 1950–1958 (2011)
Ozera, M., Perc, M., Uzuntarla, M., Koklukayab, E.: Weak signal propagation through noisy feedforward neuronal networks. NeuroReport 21, 338–343 (2010)
Bolhasani, E., Azizi, Y., Valizadeh, A.: Direct connection sassist neurons to detect correlation in small amplitude noises. Front. Comput. Neurosci. 7, 108 (2013)
Yao, C.G., Ma, J., Zhiwei He, Z.W., Qian, Y., Liu, L.P.: Transmission and detection of biharmonic envelope signal in a feed-forward multilayer neural network. Physica A 523, 797–806 (2019)
Esfahani, Z.G., Gollo, L.L., Alireza Valizadeh, A.: Stimulus-dependent synchronization in delayed-coupled neuronal networks. Sci. Rep. 6, 23471 (2016)
Perc, M., Gosak, M.: Pacemaker-driven stochastic resonance on diffusive and complex networks of bistable oscillators. New J Phys. 10, 053008 (2008)
Perc, M.: Stochastic resonance on excitable small-world networks via a pacemaker. Phys. Rev. E 76, 066203 (2007)
Winfree, A.T.: The Geometry of Biological Time. Springer, New York (1980)
Blasius, B., Huppert, A., Stone, L.: Complex dynamics and phase synchronization in spatially extended ecological systems. Nature 399, 354–359 (1999)
Landa, P.S., McClintock, P.V.E.: Vibrational resonance. J. Phys. A: Math. Gen. 33, L433–L438 (2000)
Baltanás, J.P., López, L., Blechman, I.I., Landa, P.S., Zaikin, A., Kurths, J., Sanjuán, M.A.F.: Experimental evidence, numerics, and theory of vibrational resonance in bistable systems. Phys. Rev. E 67, 066119 (2003)
Blekhman, I.I., Landa, P.S.: Conjugate resonances and bifurcations in nonlinear systems under biharmonical excitation. Int. J Non-Linear Mech. 39(3), 421–426 (2004)
Ullner, E., Zaikin, A., Garcia-Ojalvo, J., Bascones, R., Kurths, J.: Vibrational resonance and vibrational propagation in excitable systems. Phys. Lett. A 312, 348–354 (2003)
Yao, C.G., Zhan, M.: Signal transmission by vibrational resonance in one-way coupled bistable systems. Phys. Rev. E 81, 061129 (2010)
Casado-Pascual, J., Baltanás, J.P.: Effects of additive noise on vibrational resonance in a bistable system. Phys. Rev. E 69, 046108 (2004)
Yao, C.G., Liu, Y., Zhan, M.: Frequency-resonance-enhanced vibrational resonance in bistable systems. Phys. Rev. E 83, 061122 (2011)
Chizhevsky, V.N., Smeu, E., Giacomelli, G.: Experimental evidence of “Vibrational Resonance” in an optical system. Phys. Rev. Lett. 91, 220602 (2003)
Yang, L., Liu, W., Yi, M., Wang, C., Zhu, Q., Zhan, X., Jia, Y.: Vibrational resonance induced by transition of phase-locking modes in excitable systems. Phys. Rev. E 86, 016209 (2012)
Wu, X.X., Yao, C.G., Shuai, J.W.: Enhanced multiple vibrational resonances by \(Na^+\) and \(K^+\) dynamics in a neuron model. Sci. Rep. 5, 7684 (2015)
Yao, C.G., He, Z.W., Nakano, T., Shuai, J.W.: Spiking patterns of a neuron model to stimulus: Rich dynamics and oxygen’ s role. Chaos 28, 083112 (2018)
Maksimov, A.: On the subharmonic emission of gas bubbles under two-frequency excitation. Ultrasonics 35(1), 79–86 (1997)
Victor, J.D., Conte, M.M.: Two-frequency analysis of interactions elicited by Vernier stimuli. Vis. Neurosci. 17(6), 959–973 (2000)
Gherm, V., Zernov, N., Lundborg, B., Vastberg, A.: The two-frequency coherence function for the fluctuating ionosphere: narrowband pulse propagation. J. Atmos. Sol.-Terr. Phys. 59(4), 1831–1841 (1997)
Pariz, A., Esfahani, Z.G., Parsi, S.S., Valizadeh, A., Canals, S., Mirasso, C.R.: High frequency neurons determine effective connectivity in neuronal networks. NeuroImage 166, 349–359 (2018)
Gerhardt, H.C.: Significance of two frequency bands in long distance vocal communication in the green treefrog. Nature 261, 692–694 (1976)
Heiligenberg, W.: Neural Nets in Electric Fish. MIT Press, Cambridge (1991)
Middleton, J., Longtin, A.J.B., Maler, L.: The cellular basis for parallel neural transmission of a high-frequency stimulus and its low-frequency envelope. Proc. Natl. Acad. Sci. USA 103(39), 14596–14601 (2006)
Van, H., Der, L., Glaser, E.M.: Autapses in neocortex cerebri: synapses between a pyramidal cell’ s axon and its own dendrites. Brain Res. 48, 355–360 (1972)
Bekkers, J.M.: Neurophysiology: are autapses prodigal synapses? Curr. Biol. 8, R52–R55 (1998)
Flight, M.H.: Axon degeneration: committing to a break up. Nat. Rev. Neurosci. 10, 316–317 (2009)
Bekkers, J.M., Stevens, C.F.: Excitatory and inhibitory autaptic currents in isolated hippocampal neurons maintained in cell culture. Proc. Natl. Acad. Sci. USA 88, 7834–7838 (1991)
Bekkers, J.M.: Synaptic transmission: functional autapses in the cortex. Curr. Biol. 13, R433–R435 (2003)
Bacci, A., Huguenard, J.R.: Enhancement of spike-timing precision by autaptic transmission in neocortical inhibitory interneuronsoriginal research. Neuron 49, 119–130 (2006)
Bacci, A., Huguenard, J.R., Prince, D.A.: Functional autaptic neurotransmission in fast-spiking interneurons: a novel form of feedback inhibition in the neocortex. J. Neurosci. 23, 859–866 (2003)
Qin, H., Ma, J., Wang, C., Wu, Y.: Autapse-induced spiral wave in network of neurons under noise. PLoS ONE 9, e100849 (2014)
Qian, Y., Liu, F., Yang, K., Zhang, G., Yao, C.G., Ma, J.: Spatiotemporal dynamics in excitable homogeneous random networks composed of periodically self-sustained oscillation. Sci. Rep. 7, 11885 (2017)
Wu, Y., Gong, Y., Wang, Q.: Autaptic activity-induced synchronization transitions in Newman–Watts network of Hodgkin–Huxley neurons. Chaos 2, 043113 (2015)
Wang, H., Wang, L., Chen, Y., Chen, Y.: Effect of autaptic activity on the response of a Hodgkin–Huxley neuron. Chaos 24, 033122 (2014)
Chen, J.X., Xiao, J., Li Qiao, L.Y., Xu, J.R.: Dynamics of scroll waves with time-delay propagation in excitable media. Commun. Nonlinear Sci. Numer. Simul. 59, 331–337 (2018)
Li, Y., Schmid, G., Haggi, P., Schimansky-Geier, L.: Spontaneous spiking in an autaptic Hodgkin–Huxley setup. Phys. Rev. E 82, 061907 (2010)
Hashemi, M., Valizadeh, A., Azizi, Y.: Effect of duration of synaptic activity on spike rate of a Hodgkin–Huxley neuron with delayed feedback. Phys. Rev. E 85, 021917 (2012)
Song, X., Wang, H.T., Chen, Y.: Autapse-induced firing patterns transitions in the Morris–Lecar neuron model. Nonlinear Dyn. 96, 2341–2350 (2019)
Sun, X.J., Li, G.F.: Synchronization transitions induced by partial time delay in a excitatory-inhibitory coupled neuronal network. Nonlinear Dyn. 96, 2509–2522 (2017)
Zhao, Y.T., Wei, Y.H., Shuai, J.M., Wang, Y.: Fitting of the initialization function of fractional order systems. Nonlinear Dyn. 93, 1599–1618 (2018)
Zhang, X.H., Liu, S.Q.: Nonlinear delayed feedback control of synchronization in an excitatory-inhibitory coupled neuronal network. Nonlinear Dyn. 96, 2509–2522 (2019)
Qian, N., Sejnowski, T.J.: When is an inhibitory synapse effective? Proc. Natl. Acad. Sci. USA 87, 8145–8149 (1990)
Eccles, J.C.: The synapse: from electrical to chemical transmission. Annu. Rev. Neurosci. 5, 325–339 (1982)
Hodgkin, A.L., Huxley, A.F.: A quantitative description of membrane current and its application to conduction and excitation in nerve. J. Physiol. 117, 500–544 (1952)
Connelly, W.M., Lees, G.: Modulation and function of the autaptic connections of layer V fast spiking interneurons in the rat neocortex. J. Physiol. 588, 2047–2063 (2010)
Swadlow, H.A.: Physiological properties of individual cerebral axons studied in vivo for as long as one year. J. Neurophysiol. 54, 1346–1362 (1985)
Wang, S., Wang, W., Liu, F.: Propagation of firing rate in a feed-forward neuronal network. Phys. Rev. Lett. 96, 018103 (2006)
Pyragas, K.: Continuous control of chaos by self-controlling feedback. Phys. Lett. A 170, 421–428 (1992)
Barabási, A., Albert, R.: Emergence of scaling in random networks. Science 286, 509–512 (1999)
Watts, D.J., Strogatz, S.H.: Collective dynamics of ’small-world’ networks. Nature 393, 440–442 (1998)
Lübke, J., Markram, H., Frotscher, M., Sakmann, B.: Frequency and dendritic distribution of autapses established by layer 5 pyramidal neurons in the developing rat neocortex: comparison with synaptic innervation of adjacent neurons of the same class. J. Neurosci. 16, 3209 (1996)
Wang, C., Guo, S., Xu, Y., Ma, J., Tang, J., Alzahrani, F., Hobiny, A.: Formation of autapse connected to neuron and its biological function. Complexity 2017, 5436737 (2017)
Xu, Y., Ying, H., Jia, Y., Ma, J., Hayat, T.: Autaptic regulation of electrical activities in neuron under electromagnetic induction. Sci. Rep. 7, 43452 (2017)
Acknowledgements
This work was supported partially by the National Natural Science Foundation of China under Grant Nos. 11675112, 11675134 and 11874310; and the 111 Project under Grant No. B16029.
Author information
Authors and Affiliations
Corresponding author
Ethics declarations
Conflicts of interest
The authors declare that there is no conflict of interest to this work.
Additional information
Publisher's Note
Springer Nature remains neutral with regard to jurisdictional claims in published maps and institutional affiliations.
Rights and permissions
About this article
Cite this article
Yao, C., He, Z., Nakano, T. et al. Inhibitory-autapse-enhanced signal transmission in neural networks. Nonlinear Dyn 97, 1425–1437 (2019). https://doi.org/10.1007/s11071-019-05060-z
Received:
Accepted:
Published:
Issue Date:
DOI: https://doi.org/10.1007/s11071-019-05060-z