Abstract
Landslides are often deadly natural events. Steep slopes and certain loose soil types are predestined areas for them. Moreover, in the context of climate change, extreme weather events such as heavy rainfall, which often trigger landslides, are becoming even more likely. While all this is well known, it, therefore, stands to reason that this knowledge will lead to the avoidance of these risks. On the other hand, however, there are highly dynamic urbanization processes that often overtake formal urban planning processes by rising population figures and areal expansion. In the course of these processes, economically deprived population groups often have no other option than to informally build on high-risk areas. Against these backgrounds, we systematically examine in this study how these risks develop over a 24-year period from 1994 to 2018 taking into account three time steps, with respect to the citywide exposure and in particular with respect to different social groups. For this purpose, we use heterogeneous input data from remote sensing, landslide hazard maps, and census data. Our case study is the city of Medellín in Colombia. We develop and apply a set of methods integrating the heterogenous data sets to map, quantify and monitor exposure and social vulnerability at a fine spatial granularity. Our results document first of all the highly dynamic growth in total population and urban areas. However, our results reveal that the city's expansion is socially unevenly distributed. People of higher vulnerability proxied by informal settlements are found to settle in considerably higher shares of areas exposed to landslides. This study proposes a methodological setup that allows for monitoring exposure and social vulnerability over long time spans at a fine spatial resolution, allows to bring inequality into the spotlight, and provides decision-makers with better information to develop socially responsible policies.
Similar content being viewed by others
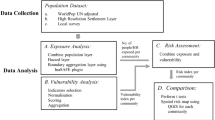
Avoid common mistakes on your manuscript.
1 Introduction
Landslides are one of the most frequent geological hazards in the world (Skrzypczak et al. 2021). Disasters related to landslides are directly associated with loss of lives as well as property, infrastructure, and environmental damages. Moreover, urbanization and raising population numbers with a potential simultaneous lack of urban planning practices have led to informal developments in hazardous hillslopes of urban agglomerations (Mendes et al. 2018; Kurniawan and Krol 2014). Especially, low-income populations are forced to settle in those hazard-prone areas (Müller et al. 2020; UN-Habitat 2016; UN 2015), increasing the landslide risk through man-made changes like vegetation removal or sewage disposal systems (Mendes et al. 2018; Reichenbach et al. 2014; Glade 2003). According to UN-Habitat, in 2016 four out of ten non-permanent houses in developing countries were built in areas threatened by natural disasters like landslides. Besides, climate change is increasing the occurrence and risk to experience damaging hazardous landslide events (UN-Habitat 2016).
As a consequence, the exposure of physical, social, economic, and environmental assets, known as the elements at risk, is increasing (Kurniawan and Krol 2014; Pellicani et al. 2013). In this context, risk reduction measures play a very important role in diminishing the impact in case of a landslide, especially since the magnitude of a disaster has been directly related to the vulnerability and exposure of the assets at risk (Carvalho de Assis Dias et al. 2018; Birkmann and Welle 2015). Similarly, the Sendai Framework 2015–2030 for Disaster Risk Reduction by the United Nations recommends that disaster risk assessment, prevention, mitigation, and implementation of response measures are established by analyzing the hazard in all its dimensions (i.e., vulnerability, capacity, exposure, hazard characteristics, and environment) (UNISDR 2015).
Landslide risk assessment depends on the complex interplay of the hazard, the elements at risk, and their vulnerability (e.g., Birkmann 2006). A hazard is defined as the probability of a disastrous event happening in a certain period, with particular intensity at a particular location (Unesco 1973). Indicators such as the probability of occurrence, intensity, or duration specify the hazard event (Geiß and Taubenböck 2013). Exposure refers to the elements present in the potentially affected area, such as people, infrastructure, or economic values, while vulnerability relates to the resilience of these elements. This can comprise, for example, the stability of building structures (Geiß et al. 2015), or the potential to recover from the effects of the natural hazard through economic reserves. Concerning the social component of vulnerability, it is generally understood that the vulnerability of economically deprived communities is higher due to more precarious conditions of their assets and livelihoods. Therefore, informal settlements are more impacted by natural disasters like landslides (Hallegatte et al. 2017; Wisner et al. 2003). Since risk is a multidimensional concept and based on their mutual influence, exposure and vulnerability are suggested to be analyzed together (UNISDR 2015). To this end, paying special attention to the social aspect is crucial. The same loss is more severe with increasing social vulnerability, which influences the ability of a community to cope, resist, and recover from a disaster (Carvalho de Assis Dias et al. 2018; World Bank Group 2017; Vranken et al. 2015). Nevertheless, risk studies in urban systems often analyze exposure independently as an individual part of risk assessment. An example is the identification of exposed people and housing through the spatial overlay with hazard susceptibility zones (Geiß et al. 2017).
However, studies focusing on the quantification of area-wide landslide exposure and vulnerability consider the latter mostly from an economic, physical, or environmental perspective, focusing on the monetary loss or severity of damage to buildings, infrastructure, or environment (e.g., Guillard-Gonçalves et al. 2016; Vega & Hidalgo 2016; Vranken et al. 2015; Galli & Guzzetti 2007). The inclusion of social vulnerability on the other hand is limited for landslide risk assessment analyses (Nor Diana et al. 2021; Puissant et al., 2014; Hollenstein 2005). To the knowledge of the authors, there are comparatively few exposure studies that consider the concept of social vulnerability as the socioeconomic level of the exposed assets to distinguish between certain degrees of severity and ability to recover from a disaster (e.g., Wijaya & Hong 2018; Carvalho de Assis Dias et al. 2018). Previous studies approached social vulnerability rather as the number of affected people regardless of their socioeconomic status (e.g., Kurniawan and Krol 2014; Puissant et al. 2014; Pellicani et al. 2013; Papathoma-Köhle et al. 2007), or work on comparatively low spatial resolutions, cover a smaller geographic extent, or are focused on multirisks (e.g., Aksha et al. 2020; Frigerio and De Amicis 2016; Guillard-Gonçalves et al. 2014).
Not only the definition of vulnerability varies in the literature but also the geographic scale for assessments. Landslide risk studies are mostly conducted on a macroscale level, where the study area covers a whole region, generally with the aim to identify and rank the elements at risk to produce risk maps (e.g., Promper et al. 2015; Vranken et al. 2015; Pellicani et al. 2013; Jaiswal et al. 2011). The mesoscale level is used for local planning purposes in cities or municipalities (e.g., Guillard-Gonçalves et al. 2016; Carvalho de Assis Dias et al. 2018). At this level, potential losses and consequences (i.e., physical or economic vulnerability) can already be partially quantified, for example, by clustering assets with similar characteristics (Puissant et al. 2014). Microscale level analyses are performed for local areas, quantifying physical, social, environmental, and economic vulnerabilities and aiming to implement technical and protective measures (e.g., Singh et al. 2019; Holcombe et al. 2012). Therefore, the amount of data required to conduct such studies is proportional to the detail of the analysis scale (Puissant et al. 2014).
Likewise, time plays an important role in risk assessment (UNISDR 2015) and should not be neglected. On the one hand, the temporal probability of landslide occurrences is based on historical records, and on the other hand, exposed elements and their vulnerability are subject to temporal changes (Van Westen et al. 2006). Few studies analyzed landslide exposure including the development of elements at risk (e.g., Promper et al. 2015; Kurniawan and Krol 2014), however, to the knowledge of the authors no reviewed study considered the temporal change of vulnerability. In this sense, multitemporal risk assessment requires consistent data over time.
Accordingly, Earth Observation (EO) has become more popular in the last decades in the landslide analysis domain as a source of data. The advances in the space-borne sector make EO techniques increasingly effective for landslide detection, mapping, monitoring, and hazard assessment (e.g., Novellino et al. 2021; Zhong et al. 2020; Casagli et al. 2017). For instance, the detection and mapping of landslides have been conducted by analyzing land cover changes through vegetation indices (e.g., Behling et al. 2014) or machine learning techniques (e.g., Ghorbanzadeh et al. 2019). Besides, remotely sensed data are also used to identify the exposed elements at risk over time (e.g., Promper et al. 2015; Kurniawan and Krol 2014). Particularly, land use and land cover (LULC) information is commonly applied to classify physical assets like built-up areas (Rahman and Di 2017). Whereas population distribution data, the social assets, can be estimated from official population counts supported by information derived from remotely sensed data (e.g., Sapena et al. 2022; Taubenböck et al. 2011). Likewise, the social component of the assets can be retrieved from space. Many studies demonstrated the possibility of identifying economically deprived settlements based on their morphological characteristics with high-resolution satellite data (e.g., Stark et al. 2020; Wurm et al. 2019, 2017).
The population in Medellín, Colombia, is growing fast and often informal, thus more and more people with a high social vulnerability live in the risk-prone hills of the city due to scarce land availability. This fact along with climate change-induced higher occurrences of heavy rainfalls leads to an increase in landslide events (IDEAM-UNAL 2018). Against this background, we conduct in this study a long-term multitemporal, intra-urban, and mesoscale landslide exposure and social vulnerability assessment in the city of Medellín. This is accomplished by utilizing multitemporal satellite and census data for three time steps (1994, 2006, and 2018), as well as machine learning algorithms and population disaggregation methods. We quantify the physical assets as the urban structures composed of buildings and infrastructure, and the social assets as the amount of population in those areas, separating between formal and informal settlements to approximate social groups and relate this to vulnerability. This analysis enables us to highlight different aspects of landslide risk assessment. We illustrate the evolution of built-up and population growth throughout time and on this basis investigate whether exposure and social vulnerability, in absolute and relative terms, have increased over time. The results intend to support urban planners and risk managers for informed decision-making.
2 Material and methods
This section introduces the general workflow of the study, the study area, the employed datasets, their processing steps, and the methodology of the exposure and social vulnerability analysis. Moreover, a conceptual note explains the assumptions taken in the context of this paper.
We developed the workflow shown in Fig. 1 for the long-term multitemporal, intra-urban, and mesoscale landslide exposure and social vulnerability assessment. First, for the generation of urban masks for three time steps (1994, 2006, and 2018), we perform land cover (LC) classifications based on Landsat mosaics (a); second, we divide the built-up area of the city into two thematic groups: using the informal settlement layer from Kühnl et al. (2021), we classify informal and formal settlement areas to approximate social groups (b); third, population is estimated at the pixel level using disaggregation methods (c) and specified into the population of informal settlements (d). These results (i.e., multitemporal urban masks, informal settlements, and population) are combined with the landslide hazard map for a multitemporal exposure and social vulnerability analysis (e).
Workflow of the study composed of land cover classifications based on Landsat data to conduct multitemporal urban masks (a), multitemporal informal mask calculations based on an informal settlement layer from Kühnl et al. (2021) (b), population disaggregation methods based on census data separating between formal (c) and informal areas (d) as well as the exposure and as social vulnerability analysis using all pre-processed data and a landslide hazard map (e)
2.1 Study area
Our study area, the city of Medellín, is the second largest city in Colombia. It is the capital of the Department of Antioquia as well as of the Metropolitan region of the Aburrá Valley, a political and administrative unit of ten municipalities with a population of 3.5 million (Fig. 1b; Garcia Ferrari et al. 2018; Hernandez Palacio 2012).
The municipality of Medellín (Fig. 2, white boundary) is composed of urban (Medellín and San Antonio) and rural parts (Fig. 2a, c). The area of interest (AOI) in this study refers to the urban, expansion, and urbanized areas of Medellín (Fig. 2a). Expansion areas are in the process of officially getting added to the administrative urban areas but do not yet fully belong to this planning level (Alcaldía de Medellín 2014a, b). Urbanized areas are characterized by high built-up density consisting mostly of informal urban structures, which have grown into the official administrative rural area of the municipality. By including them in our AOI besides urban and expansion areas, the analysis reflects the built-up conditions beyond administrative boundaries.
Location of the study area. Urban (= administrative urban areas), expansion (= in the process of officially getting added to the urban area) and urbanized areas (= areas with high built-up density, but officially classified as rural) of the Municipality of Medellín within the Aburrá Valley in Colombia. In the background, the hillshade of a digital elevation model shows the topography (DEM AMVA 2022: Open data Medellín)
Geographically, Medellín is situated in a 14 km north–south expansion in the Aburrá Valley between two mountain ranges of the Andes in the west and the east, crossed by the Medellín River running from north to south along the valley. The valley itself has a 10 km maximum width and the height difference between the highest and lowest point is about 1 km (Garcia Ferrari et al. 2018; Hernandez Palacio 2012). These characteristics lead to a very steep topography of the valley slopes in the east and west with a significant landslide risk (Claghorn and Werthmann 2015).
Socioeconomically, the living conditions get worse with distance to the Medellín River and higher up in the mountains. Especially, the landslide and flash-flood-prone slopes in the west and east of the city are mainly occupied by informal dwellers. Also, a north–south segregation is existent. The neighborhoods with low unemployment rates are located in the southeast of the city whereas the contrary is dominant in the northeast and -west (Garcia Ferrari et al. 2018).
2.2 Data
In this study we relied on long-term multitemporal data; we used Landsat satellite imagery at a 30-m resolution to derive LC maps, with a special focus on the urban layout. To do so, we created cloud-free Landsat mosaics for three time steps: 1994, 2006, and 2018. We used the Google Earth Engine platform (Gorelick et al. 2017) for building the cloud-free mosaics and downloading the resulting images. Since the period is fairly wide, we used the atmospherically corrected surface reflectance datasets from Landsat 5 ETM (L5), Landsat 7 ETM + (L7), and Landsat 8 OLI/TIRS (L8) sensors. We filtered cloudy pixels by masking low-quality pixels using the pixel_qa band. Due to the tropical climate in Medellin, the chances to get cloud-free pixels are quite low, and thus, we set long-term periods for the mosaicking to obtain good enough results for each date. Calculating the median, we used imagery from the year 1989 to 1994 to create the 1994 mosaic, images from 2003 to 2006 to create the 2006 mosaic, and from 2013 to 2018 to create the 2018 mosaic. For the LC classification, we selected a subset of spectral bands and calculated additional indices. On top of the visible red, green, and blue (RGB), near-infrared (NIR), and short-wave infrared (SWIR) bands (bands 1,2,3,4,5,7 in L5 and L7, and bands 2,3,4,5,6,7 in L8), we calculated the Normalized Difference Vegetation Index (NDVI), the Normalized Difference Water Index (NDWI) and the Normalized Difference Buildings Index (NDBI) as well as their 10th, 25th, 50th, 75th, and 90th percentiles. The NDVI gives information about the greenness of vegetation (Rouse et al. 1973), the NDWI indicates water features (positive values) or soil and terrestrial vegetation (negative values; McFeeters 1996), and the NDBI acts as an indicator for built-up areas (Zha et al. 2003). As a result, each image mosaic (see Fig. 1a) has 24 bands (composed of spectral bands, indices, and percentiles). These indices and their percentiles provide additional information for training the LC classification algorithm.
Secondly, to retrieve information on social vulnerability, we proxied the socioeconomic status based on the morphologic characteristics of the living environment and built-up structures. We used an informal settlement mask based on a scene-based Local Climate Zone (LCZ) classification of Medellín, performed with the use of a very high-resolution satellite image from the year 2019 and urban blocks (Kühnl et al. 2021). The lightweight low-rise class is an urban structural type within the LCZ schema (Stewart & Oke 2012), which shows typical morphological features of informal settlements like high density, small and low-rise buildings, lightweight construction materials, and sparse vegetation. We extracted the lightweight low-rise polygons with their centroid in our AOI (Fig. 3a, 1b). Figure 3b, c shows two examples of the neighborhoods and building types identified as informal. The accuracy of the informal settlement layer has been measured at 86% (Kühnl et al. 2021). For this study, we manually checked and corrected over- and under-classifications to improve the informal settlement mask.

(© Google Street View 2021) of a characteristic slope covered with informal buildings and c of an exemplary housing structure in an informal settlement. d Landslide hazard map from the POT 2014 (Plan de Ordenamiento Territorial de Medellín). The map categorizes landslide hazard into very low, low, medium, and high. e, f are visual examples of the high hazardous slopes (own source)
a Location of formal and informal settlements in Medellín based on the improved lightweight low-rise built-up class. b Google Street View image
Thirdly, to estimate the amount of population at risk over time we used population data from the official population projections (Proyecciones de Población 1993–2005 a 2015 de Medellín; Alcaldía de Medellín 2015) for the years 1994 and 2006 and the Colombian census (Censo Nacional de Población y Vivienda; DANE 2018) for the year 2018 (Fig. 4). We used these dates since the projections coincide with former census surveys from 1993 to 2005 and the uncertainty in the projection is expected to be lower. Depending on the year, the population data are available in different spatial units (i.e., commune, neighborhood, sector, and section levels; Fig. 4). Data from 2018 are existing at different spatial levels, therefore we used the sector level to disaggregate the population to the pixel level and the section level, with higher detail, to validate the result.
Finally, we used an open source landslide hazard map for the whole municipality in vector format provided by the land use plan from Medellín (Plan de Ordenamiento Territorial de Medellín; POT 2014). The hazard map was created by combining all risk-related information available (Fig. 3d). It relies on hazard maps from the POT 2006 and the National University of Colombia from 2009, as well as mass movement inventories by the Administrative Department of Disaster Management (DAGRD), morphodynamic process maps, and all geotechnical and slope stability studies carried out for the municipality of Medellín since 2006. It categorizes landslide hazard into very low, low, medium, and high susceptibility zones (Alcaldía de Medellín 2014c). Hence, it is the best hazard map that is available at the city level, since it includes multi-source data and was created by local experts.
2.3 Conceptual note
Landslide estimation, exposure, and vulnerability of the population is a multifaceted and highly complex problem. This requires highly accurate, diverse, and, in our approach, multitemporal data sets. Since these are not available in the necessary spatial and thematic depth, nor are they consistent, complete, and given over time, we must make some conceptual assumptions based on the available data.
First, we analyze landslide risk using a hazard map from 2014 assuming that the hazard areas keep constant over time. In this study, we consider two risk levels; risk areas when the hazard of a landslide in the POT is medium or high, and non-risk areas when the hazard is low or very low.
Secondly, concerning exposure and the capabilities of remotely sensed data available since the 1990s, we make the following assumptions: pixels classified as urban that are categorized as ‘formal’ in 2018, are per definition also ‘formal’ in previous time steps if they were identified as urban in 1994 and 2006, otherwise, they are non-urban pixels. Similarly, urban pixels categorized as ‘informal’ in 2018 are also informal in previous time steps, when the pixels are urban. Therefore, formal development does not change to informal over time, since this transition is very unlikely. At the same time, we disregard informal settlement upgrade, since we do not have data on informality over time as our informal settlement mask is from 2019.
Thirdly, socioeconomic indicators allowing us to approach the social sphere in a multitemporal manner are inexistent, especially on such a high spatial granularity. Thus, we tackle social vulnerability using morphological parameters as a proxy. We assume that people living in informal settlements are more vulnerable. We assume their economic capabilities reduce the chances of recovering after a disaster. Besides, the definition of morphologic informality differs slightly from informal settlements from a socioeconomic perspective; however, as Kühnl et al. (2021) and Wurm and Taubenböck (2018) show, it is a fairly accurate proxy of precariousness and informality.
2.4 Land cover classification
Our focus for the analysis of exposure to landslide hazards is settlement areas. In this regard, we used Landsat mosaics for the three time steps to classify Medellín into basic land covers (urban, open vegetation, forest, and bare soil). We applied a supervised pixel-based classification method using the Random Forest (RF) machine learning algorithm (Breiman 2001). In preparation, we manually created ground truth sample data. This was done independently at each of the three time steps by visual image interpretation with the help of historical and very high-resolution images from Google Earth©. The sample data were polygons covering areas of homogeneous land cover, which were equally split into spatially independent training and testing polygons (50/50). The image pixels for the training and testing datasets were subsequently randomly selected from the respective polygons. We used the training dataset to build a RF model for each year that was validated against the testing dataset of the respective year. Using spatially independent pixels in the validation, we thus avoided spatial correlation between classification and evaluation. Table 1 shows the accuracy metrics of the RF classification for each time step for the different thematic classes. The User´s accuracy represents the percentage of correctly classified pixels in a class with respect to the total pixels classified as that class. The Producer´s accuracy represents the correct classified sample pixels in a class with respect to the total sample pixels of that class. While Overall accuracy is the ratio between the correct classified pixels from all classes and the total number of sample pixels (Congalton 1991).
We used the urban land covers to create the urban masks for 1994, 2006, and 2018. To diminish temporal fluctuation in the data, we applied a change trajectory analysis similar to the ones applied by Taubenböck et al. 2012. This approach solves errors from the classifier when it is unable to correctly detect urban areas over time. The assumption is that urban pixels cannot change to non-urban pixels. Therefore, several rules were established following the majority rule. For example, if a pixel is urban in 1994 and 2018, but it is classified non-urban in 2006, we change the status to an urban pixel in 2006 as well to keep consistency. Similarly, if a pixel is urban in 1994 and 2006, it should also be urban in 2018. However, if a pixel is urban in 1994, but it is non-urban in 2006 and 2018, the state of 1994 is changed to non-urban.
2.5 Identification of informal settlements
To produce the multitemporal informal settlement masks, we relied on the urban block level vector dataset with informal settlements in 2019 (see Sect. 2.2). We applied the morphological filters dilation and erosion (Soille 2004) to fill the gaps between urban blocks, such as roads, to obtain a continuous surface, and then it was transformed into raster format using the majority rule with a spatial resolution of 30 m. Finally, the informal settlement mask 2019 was spatially overlapped with the multitemporal urban masks, and matching pixels were considered informal settlements. As a result, we had three consistent informal settlement masks for the years 1994, 2006, and 2018.
2.6 Disaggregation of population to the pixel level
We estimated the population at the pixel level using a top-down binary dasymetric disaggregation method originally developed by Wright (1936). This method consists of redistributing population counts from larger spatial units into smaller spatial units (Reed et al. 2018; Stevens et al. 2015; Wu et al. 2005).
In this study, we used administrative boundaries as source zones where population counts are known (i.e., communes, neighborhoods, and sectors for 1993, 2006, and 2018, respectively) and urban pixels, from the urban masks 1994, 2006, and 2018, as target zones. In the first step, we calculated for each source zone the population density by dividing the population count by the urban area. Then, the population at target zones (pixels) was estimated by multiplying the area of the pixel by the previously calculated population density from the source zone where the pixel is located. For those remaining target zones outside of the boundary of a source zone (a few urban pixels in the west and east of Medellín are not covered by the administrative units), we used the population density of the closest urban pixel. Once we had the population at the pixel level for 2018, we extracted the pixels covered by the informal settlement mask from the same year to obtain the informal settlement population at the pixel level. Lastly, we validated the disaggregation method with official population counts at higher spatial detail. We used the population counts at the section level from the year 2018 as validation zones to evaluate the performance of the disaggregation method (Grippa et al. 2019). Therefore, the population at the pixel level was summarized using the boundaries of the validation zones, and subsequently, this sum was compared to the population counts from the validation zones to measure the root-mean-square error (RMSE) and the RMSE divided by the mean validation zone population count (%RMSE).
The same process was then applied to the remaining time steps 1994 and 2006 using the respective commune and neighborhood levels.
2.7 Quantifying exposure and social vulnerability to landslides
To quantify the development of exposure and social vulnerability in Medellín over time, we spatially overlapped the multitemporal assets and people (urban and informal settlement masks and pixel population, Fig. 1a–d) with the landslide hazard map (Fig. 1e).
To estimate how much urban area and population are at risk, we first intersected the multitemporal urban masks with the hazard map to summarize the total urban area for each hazard level and year. For those pixels crossed by the boundary of a hazard level, only the proportional area of the pixel covered was summarized. Secondly, and similarly, the multitemporal population at the pixel level was intersected with the hazard map and the total population was summarized. Likewise, when a pixel was crossed by the boundary only the proportional population of the pixel was summarized for each hazard level and year, and this was calculated using the population density of the pixel and the proportional area of the pixel covered. Finally, this process was replicated with the multitemporal informal settlement masks as well as the informal population at the pixel level.
The results of these spatial analyses enabled the calculation of the amount of exposed and socially vulnerable areas and their population based on their spatial localization for the years 1994, 2006, and 2018 by separating them into no-risk and risk areas. Since the landslide hazard map consists of four levels (very low, low, medium, and high; see Sect. 2.2), we calculated the results based on this division but focus on risk (medium and high landslide hazard) and no-risk (very low and low landslide hazard) areas in the interpretation. We also calculated the ratio between formal and informal settlements over time, using both, the area and population. In addition, we monitored the development of urban areas and population concerning their risk to answer the question of whether exposure and social vulnerability have relatively increased over time in Medellín.
3 Results: spatial and statistical development of landslide risk over time
In 1994, we found 4% (2 km2) of the entire settlement areas (51 km2) in Medellín in landslide-prone areas (Table 2: Urban settlements). In the 12 years up to 2006, the settlement areas expanded to 61 km2. In this context, settlement areas have been increasingly built into landslide-prone areas in the east and west of the city (see Fig. 5a): They grew to a spatial share of 7% (4 km2). And until 2018, the city extended to 77 km2, with the share in landslide-prone areas steadily growing to 9% (7 km2). Thus, considering the spatial location of the city growth from 1994 to 2018 (see Fig. 5a, b), it is clear that urbanization in Medellín occurred disproportionately on these exposed slopes, predominantly in the east and west of the city. Landslide risk was increasing.
a Urban settlement masks 1994–2018 extracted from the Land Cover (LC) classifications implemented with Landsat data. The urban settlements include formal and informal areas of the city. b Informal urban settlement masks 1994–2018 calculated based on the informal settlement mask 2019 (Kühnl et al. 2021) and the urban settlement masks 1994–2018 based on the LC classifications. c Population estimation 2018 per pixel in the extent of the urban settlement mask 2018 through disaggregation and extrapolation based on the census 2018. Since the estimates are based on official data, their administrative boundaries are still visible to a certain extent. d Spatial localization of at risk areas in Medellín for the time-step 2018. The areas at risk are separated according to their social vulnerability status proxied by formality and informality
Focusing on the informal settlements, proxying the social group of higher vulnerability, we found for the year 1994 that 24% (12 km2) of the whole urban layout was classified as informal (Table 2: Informal Settlements). Of these informal areas, 16% (2 km2) were located in risk areas at that time. It is interesting to see, how by 2006, the share of informal areas grew to 26% (16 km2) and that the share at locations of risk was also increasing to 23% (4 km2). Until 2018 the expansion of informal areas continued, however at slower rates to around 20 km2 (~ 26% of the urban layout). The informal areas at risk remained at around a quarter (26%; 5 km2).
In this sense, a large number of settlement areas at risk in 1994 were of informal character (1.98 out of 2.23 km2). This means that 89% of the landslide-prone elements were based on our proxy socially of higher vulnerability. Similar results were calculated for the years 2006 (3.71 out of 4.19 km2, 89%) and 2018 (5.23 out of 6.54 km2, 80%). However, even though the total settlement areas at risk grew from 1994 until 2018 (from 2.23 to 6.54 km2) and also the informal settlements at risk increased (from 1.98 to 5.23 km2), the relative share of informal settlements at risk became smaller (from 89 to 80%). This indicates that formal settlements with lower social vulnerability were also built in landslide-prone areas over the last 24 years. Figure 5d separates risk areas into formal and informal settlements for 2018, illustrating this finding.
Regarding the population, the %RMSE was measured with an error of 30%, which is around 695 people. Figure 5c shows the result of the pixel-level population disaggregation for the year 2018. The results for the years 1994 and 2006 can be found in the appendix. It can be seen that especially in the northeastern and the western slopes, where informal settlements are predominantly located, the population density is high. In contrast, lower densities are found in the heart of the city and along the Medellín River. In 1994 Medellín was inhabited by around 1.7 million people. Out of them, 6% (109,000 people) were exposed to landslides (Table 3: Urban settlements). Until 2006 the population grew to 2.2 million, with 10% (around 217,000 people) now located in areas prone to landslides. This means that the total number of people living in areas at risk doubled in 12 years. Afterward, population growth slowed down reaching 2.3 million people by 2018. Similarly, the share of people in risk areas decreased compared to the period from 1994 to 2006. In 2018, 13% of the population lived in landslide-prone sites (around 290,000 people).
When we consider the vulnerability of the population by our proxy, we find that in 1994, 35% of the total population was living in informal settlements, with a share of 16% (around 99,000 people) located simultaneously in landslide-prone areas (5.6% of the total population). In the years up to 2006, the share of people living in informal settlements grew to 40% of the total population. Regarding the total number of people exposed to landslide risk and living in informal settlements, the value doubled (around 194,000 people, 22%), which represents 9% of the total population in 2006. Similar to the total settlement and population growth rates between 2006 and 2018, population growth in informal settlements also slowed down until 2018. In this case, around 41% of the total population was located in informal areas in 2018, and 26% of them (around 236,000 people) were located in risk areas at the same time (10.4% of the total population). Therefore, the increase in the share of people at risk was coupled with an increase in the number of socially vulnerable people. However, over the 24 years of monitoring, there has been a decrease in the relative share of exposed people living in informal settlements (1994: 90%, 2006: 89%, 2018: 82%), despite the increase in absolute figures. This indicates that formal settlements were also developed on landslide-prone slopes.
4 Discussion
Increasingly frequent landslides due to climate change and uncontrolled urbanization are the cause of huge human and economic losses worldwide. Multi-source data from Earth Observation in combination with hazard maps and census data can provide key information for supporting risk management. Objective, accurate, up-to-date, and frequent data can be produced on several scales. However, area-wide multitemporal risk assessment studies that tackle exposure and vulnerability of people and assets at the same time, especially from a social perspective, at a fine spatial resolution are still limited. With this study, we show an approach that helps to close this gap: We performed a long-term multitemporal analysis on the evolution of landslide exposure and social vulnerability in a large, complex, and fast-growing urban area, the city of Medellín, Colombia.
First, because area-wide and open source satellite data with resolutions in the 1-m range did not emerge until the early 2000s, a long-term study since the 1990s requires lower resolution data. The use of medium-resolution Landsat images and machine learning algorithms allowed us to produce this long-term LC information with fairly good quality (overall accuracies above 90%) and resolution for a citywide analysis, even if certain uncertainties had to be accepted due to the tropical climate conditions. To create cloud-free mosaics, we used the median of several years. This method may have led to an under-classification of urban pixels in the following LC classification for example. Different statistics for the mosaicking process could be tested to reduce potential errors. And still, Landsat data are not suitable for detailed intra-urban studies. Alternative open satellite imagery such as Sentinel-2 or PlanetScope can overcome this problem. However, only in exchange for a more recent and thus shorter temporal monitoring period. Nevertheless, the LC classifications based on Landsat data are very reliable providing a good basis for the urban mask extraction for three time steps, proxying the elements that may be exposed to landslide hazards. The results were consistent over time and minor gaps were solved using the temporal series. With this approach, it becomes possible to determine the urban development over time, spatially and quantitatively: We quantified that in the last 24 years Medellín grew by 50% of its total settlement area. The spatial analysis with the hazard zoning map showed that urban areas exposed to landslide risks (medium and high hazard levels) have tripled in this period. Medellín had 4% of the urban areas in exposed slopes in 1994 and this value has increased to 9% in 2018. The average growth rate of exposed areas in the city is 0.2 km2/year, indicating that indeed the city is growing toward hazardous areas.
Secondly, regarding the social component of risk assessment, it is widely understood that a disaster affects differently depending on who experiences it. The same loss is more severe for low-income people since they have fewer resources to recover. However, data on the socioeconomic status are often outdated or even nonexistent, especially at the high spatial resolution applied in this study. We assumed that people living in informal settlements have a lower socioeconomic status than their pairs living in urban formal settlements, and thus they are more vulnerable. This allowed us to proxy the vulnerability of social groups based on the morphology of the built-up structures. We are aware that this approach can only spatially proxy a social group and it does not do justice to the complexity of the social concept in reality. But, as Wurm and Taubenböck (2018) have shown, building structures of this type are certainly a legitimate proxy when other data are not available. Using this conceptualization, we produced informal settlement masks for the three time steps. In the setting of this study, with limited data availability, it was not possible to consider informal settlement upgrades over time, and additionally, we assumed that urban formal settlements do not change to informal. We quantified that the ratio between formal and informal settlements was quite constant in the last 24 years, one quarter of the urban settlements are in precarious conditions in Medellín. Similar numbers are also found in the literature. For instance, Echeverri Restrepo and Orsini (2012) identified 25% informal settlements in the city of Medellín, while URBAM (2012) measured 31% in the whole municipality, which is in-line with our calculations. We show that informal settlements are growing at a similar trend as the overall growth of the city. However, when we measured the share of informal settlements that are exposed to landslide hazards, we found an increase from 16 to 26% over the monitoring period compared to an increase from 1 to 2% for urban formal areas. This confirms that informal settlements are in comparison to formal settlements more exposed to landslide hazards. What is particularly interesting is that more than 89% of the exposed areas in the city were informal settlements in 1994, and this was measured with 80% in 2018, indicating that formal settlements are being established on exposed slopes more often than before.
Thirdly, for the assessment of population density, we produced multitemporal pixel-level population maps using urban masks and official population counts. We validated the disaggregation approach using population data on two spatial levels in 2018: We measured an error of 30% in the mean population at the section level, which is lower than in other studies (e.g., Grippa et al. 2019; Stevens et al. 2015). This shows that this approach is able to estimate the population at a very high resolution with a high accuracy. This is crucial because landslides are often small-scale, local events, and therefore estimates on an administrative level are spatially unsuitable. The population maps were estimated both, for the entire city and particularly for the informal settlements. We found that in general population has grown immensely since 1994, in total 30% percent, which is more than half a million inhabitants. In the first place, we measured a sharp increase in the exposed population between 1994 and 2018 from 6 to 13%. Figures of people exposed have doubled with more than 180 thousand new people living in landslide-prone areas in 2018. This result implies that population growth is taking place in hazardous areas. This is probably due to uncontrolled, informal urbanization and lack of available land forcing people into unsuitable, exposed areas. In the second place, we were able to estimate the share of people living in informal settlements. In 1994, 35% of the city population was living in informal settlements, and this amount raised to 41% in 2018, which is around 300 thousand more people living in precarious settlements. It shows that informal urban growth exceeds formal urban growth in Medellin. This 41% is higher compared to the share of informal settlement areas (26%) because these tend to be more densely populated. What is more, 6% (98,589) of the total population in Medellín was exposed to landslides and at the same time had a high social vulnerability in 1994, which increased to 10% (235,973) in 2018. In absolute terms, this is an increase of 137 thousand people in 24 years. A similar number was found by URBAM (2012). They stated that around 284,000 people were at risk of landslides and at the same time had a higher social vulnerability in the Aburrá Valley in the year 2010. This is similar to our estimations, considering that we are only working in the city of Medellín and not the entire valley. Focusing on the exposed population, this study documents how unequal and different risks are for different social groups, and specifically, people living in informal settlements are more exposed to risks. One out of ten people in Medellin lived in informal settlements and at the same time was exposed to landslide risks in 2018. However, the share of informality in exposed populations decreased from 90% in 1994 to 82% in 2018. This result is in-line with the areal analysis, showing that less vulnerable populations as well are settling in exposed areas of the city in the last decades.
With this analysis, we showed the importance of quantifying populations at a high spatial resolution, as natural hazards like landslides do not follow administrative units and are often very local events. Area-wide analyses beyond official borders are also particularly important, as urban agglomerations, especially in the dynamic cities of the global south, outgrow these units. Due to the delimitation of jurisdictional spatial entities, exposed dwellers close to the official administrative city, therefore, get neglected in statistics and thus risk management approaches. As we could show, these are mainly people living in settlements with an informal character, built at the same time in the most exposed areas. On the one hand, our study, thus, confirms individual results of previous analyses. For example, Garcia et al. (2016), Bhaduri et al. (2007), and Dobson et al. (2000), also see the necessity to quantify population in sufficient resolution outside of administrative units for risk management approaches. And Müller et al. (2020) and Baker (2012), exemplarily, found evidence that informal settlements are more exposed to natural disasters, such as hazardous events like floods or landslides. On the other hand, we combine these results and approaches and with it go beyond: mapping the most affected areas and population by potential landslides over the course of 24 years, detached from administrative boundaries, and in comparatively high resolution.
In this sense, we have seen that the combination of EO data with other data sets has a huge capacity to improve knowledge of natural hazard risks of many urban dwellers. Our results are a reliable citywide estimation of exposed locations, urban structures, people, and social groups. We are aware that these assessments are influenced by the spatial resolution of the urban masks or misclassifications and errors in the population estimation. While due to the dynamics of the urbanization process, data, and methodological issues, one might take the absolute numbers cautiously; however, we expect our relative results to be a realistic picture that is consistent in itself. With it, we provide an approach for a comprehensive picture of environmental, economic, and social risks as a basis for informed decision-making leaving no one behind. An extension of interdisciplinary approaches, e.g., with demographers, landslide modelers, and structural engineers, among others promises great potential for further development. In the domain of open EO data and techniques, our approach was developed to increase the potential transferability to other geographical regions in the world. This is especially interesting as we showed that the results from the exposure and vulnerability analyses based on the area and population are quite similar and show similar trends. This means that the methods based on urban areas over time could be replicated in areas where no population data is available to obtain good estimations of populations at risk.
5 Conclusion
In this study, we demonstrated how a long-term analysis of a city's landslide risk can be mapped and quantified with high accuracy from the combination of remote sensing data, hazard maps, and census data. We documented how the total population as well as the total urban area increased considerably from 1994 until 2018 in Medellín, Colombia. We identified that every year more and more settlement areas and people are exposed to landslide hazards. However, this is especially critical for the social group of higher vulnerability, which accounts for the majority of occupied landslide risk areas. We observed that the total number of socially vulnerable people at risk doubled between 1994 and 2018. Although in recent years, the share of population in formal settlements in hazardous landslide areas has increased as well. To conclude, our analysis shows how inequality can be mapped and measured with these heterogeneous data. It is a way to bring this inequality into the spotlight and provide decision-makers with better information to develop socially responsible policies.
References
Aksha SK, Resler LM, Juran L, Carstensen LW Jr (2020) A geospatial analysis of multi-hazard risk in Dharan. Nepal Geom Nat Hazards Risk 11(1):88–111. https://doi.org/10.1080/19475705.2019.1710580
Alcaldía de Medellín (2015) Proyecciones de Población 1993–2005 a 2015 de Medellín. https://www.medellin.gov.co/irj/portal/medellin?NavigationTarget=navurl://61486d6864f92753697d31db00277a63. Accessed 05 July 2021
Alcaldía de Medellín (2014a) Acuerdo 48 de 2014. Medellín. https://www.medellin.gov.co/irj/portal/medellin?NavigationTarget=navurl://0d6e1cabff217197f515823e5bb58bb6. Accessed 11 Mar 2021
Alcaldía de Medellín (2014b) El nuevo POT. Una ciudad para la gente, una ciudad para la vida. Medellín. https://acimedellin.org/wp-content/uploads/2017/06/RevistaPOT2014a.pdf. Accessed 25 Mar 2021
Alcaldía de Medellín (2014c) Revisión y ajuste del Plan de Ordenamiento Territorial de Medellín. Evaluación y Seguimiento—Tomo IIIB. Versión 3-Concertación con Autoridades Ambientales. Medellín. https://www.medellin.gov.co/irj/go/km/docs/pccdesign/SubportaldelCiudadano_2/PlandeDesarrollo_0_17/ProgramasyProyectos/Shared%20Content/Documentos/2014b/PropuestaPOT/POT_IIIB_Evaluaci%C3%B3nSeguimiento.pdf. Accessed 05 July 2021
Baker JL (2012) Climate change, disaster risk, and the urban poor: cities building resilience for a changing world. World Bank, Washington, DC
Behling R, Roessner S, Kaufmann H, Kleinschmitt B (2014) Automated spatiotemporal landslide mapping over large areas using rapideye time series data. Remote Sens 6:8026–8055. https://doi.org/10.3390/rs6098026
Bhaduri B, Bright E, Coleman P, Urban ML (2007) LandScan USA: a high-resolution geospatial and temporal modeling approach for population distribution and dynamics. GeoJournal 69:103–117. https://doi.org/10.1007/s10708-007-9105-9
Birkmann J (2006) Measuring vulnerability to natural hazards—towards disaster resilient societies. United Nations University, New York
Birkmann J, Welle T (2015) Assessing the risk of loss and damage: exposure, vulnerability and risk to climate-related hazards for different country classifications. Int J Glob 8(2):191–212. https://doi.org/10.1504/IJGW.2015.071963
Breiman L (2001) Random forests. Mach Learn 45:5–32
Casagli N, Frodella W, Morelli S, Tofani V, Ciampalini A, Intrieri E, Raspini F, Rossi G, Tanteri L, Lu P (2017) Spaceborne, UAV and ground-based remote sensing techniques for landslide mapping, monitoring and early warning. Geoenviron Disasters 4(9):1–23. https://doi.org/10.1186/s40677-017-0073-1
Claghorn J, Werthmann C (2015) Shifting ground: landslide risk mitigation through community-based landscape interventions. J Landsc Archit 10(1):6–15. https://doi.org/10.1080/18626033.2015.1011419
Congalton RG (1991) A review of assessing the accuracy of classifications of remotely sensed data. Remote Sens Environ 37(1):35–46. https://doi.org/10.1016/0034-4257(91)90048-B
Dane (2018) Colombia-Censo Nacional de Población y Vivienda—CPNV 2018. http://microdatos.dane.gov.co/index.php/catalog/643. Accessed 05 July 2021
DEM AMVA—ServiciosImagen\Modelo_digital_de_elevacion_AMVA. https://www.medellin.gov.co/mapas/rest/services/ServiciosImagen/Modelo_digital_de_elevacion_AMVA/ImageServer. Accessed 12 Jan 2022
Carvalho de AssisMidori Saito MS, Dos Santos AlvaláStenner RCC, Pinho G, Nobre CA, De Souza Fonseca MR, Santos C, Amadeu P, Silva D, Oliveira Lima C, Ribeiro J, Nascimento F, De Oliveira CC (2018) Estimation of exposed population to landslides and floods risk areas in Brazil, on an intra-urban scale. Int J Disaster Risk Reduct 31:449–459. https://doi.org/10.1016/j.ijdrr.2018.06.002
Dobson JE, Bright EA, Coleman PR, Durfee RC, Worley BA (2000) LandScan: a global population database for estimating populations at risk. Photogramm Eng Remote Sens 66(7):849–857
Echeverri Restrepo A, Orsini FM (2012) Informality and social urbanism in Medellín. In: Hermelin M, Echeverri Restrepo A, Ramírez JG (eds) Medellín: environment, urbanism, and society. Universidad EAFIT, Centro de Estudios Urbanos y Ambientales URBAM, Medellín, pp 132–156
Frigerio I, De Amicis M (2016) Mapping social vulnerability to natural hazards in Italy: A suitable tool for risk mitigation strategies. Environ Sci Policy 63:187–196. https://doi.org/10.1016/j.envsci.2016.06.001
Galli M, Guzzetti F (2007) Landslide vulnerability criteria: a case study from Umbria, Central Italy. Environ Manag 40:649–664. https://doi.org/10.1007/s00267-006-0325-4
Garcia RAC, Oliveira SC, Zêzere JL (2016) Assessing population exposure for landslide risk analysis using dasymetric cartography. Nat Hazards Earth Syst Sci 16:2769–2782. https://doi.org/10.5194/nhess-16-2769-2016
Garcia Ferrari S, Smith H, Coupe F, Rivera H (2018) City profile: Medellin. Cities 74:354–364. https://doi.org/10.1016/j.cities.2017.12.011
Geiß C, Taubenböck H (2013) Remote sensing contributing to assess earthquake risk: from a literature review towards a roadmap. Nat Hazards 68:7–48. https://doi.org/10.1007/s11069-012-0322-2
Geiß C, Aravena Pelizari P, Marconcini M, Sengara W, Edwards M, Lakes T, Taubenböck H (2015) Estimation of seismic building structural types using multi-sensor remote sensing and machine learning techniques. ISPRS J Photogramm Remote Sens 104:175–188. https://doi.org/10.1016/j.isprsjprs.2014.07.016
Geiß C, Schauß A, Riedlinger T, Dech S, Zelaya C, Guzmán N, Hube MA, Arsanjani JJ, Taubenböck H (2017) Joint use of remote sensing data and volunteered geographic information for exposure estimation: evidence from Valparaíso. Chile Nat Hazards 86(1):81–105. https://doi.org/10.1007/s11069-016-2663-8
Ghorbanzadeh O, Blaschke T, Gholamnia K, Meena SR, Tiede D, Aryal J (2019) Evaluation of different machine learning methods and deep-learning convolutional neural networks for landslide detection. Remote Sens 11(196):1–21. https://doi.org/10.3390/rs11020196
Glade T (2003) Landslide occurrence as a response to land use change: a review of evidence from New Zealand. CATENA 51:297–314. https://doi.org/10.1016/S0341-8162(02)00170-4
Gorelick N, Hancher M, Dixon M, Ilyushchenko S, Thau D, Moore R (2017) Google Earth Engine: planetary-scale geospatial analysis for everyone. Remote Sens Environ 202(3):1–10. https://doi.org/10.1016/j.rse.2017.06.031
Grippa T, Linard C, Lennert M, Georganos S, Mboga N, Vanhuysse S, Gadiaga A, Wolff E (2019) Improving urban population distribution models with very-high resolution satellite information. Data 13:1–17. https://doi.org/10.3390/data4010013
Guillard-Gonçalves C, Cutter SL, Emrich CT, Zêzere L (2014) Application of Social Vulnerability Index (SoVI) and delineation of natural risk zones in Greater Lisbon, Portugal. J Risk Res 18(5):651–674. https://doi.org/10.1080/13669877.2014.910689
Guillard-Gonçalves C, Zêzere JL, Pereira S, Garcia AC (2016) Assessment of physical vulnerability of buildings and analysis of landslide risk at the municipal scale: application to the Loures municipality. Portugal Nat Hazards Earth Syst Sci 16:311–331. https://doi.org/10.5194/nhess-16-311-2016
Hallegatte S, Vogt-Schilb A, Bangalore M, Rozenberg J (2017) Unbreakable: building the resilience of the poor in the face of natural disasters. Climate Change and Development Series. The World Bank, Washington, DC
Hernandez Palacio FA (2012) Sprawl and Fragmentation. The Case of Medellin Region in Colombia. In: Papa R (ed) Landscape of Urban Sprawl. TeMA—Journal of Land Use, Mobility and Environment 5 (1): 102–120. https://doi.org/10.6092/1970-9870/762
Holcombe H, Smith S, Wright E, Anderson MG (2012) An integrated approach for evaluating the effectiveness of landslide risk reduction in unplanned communities in the Caribbean. Nat Hazards 61(2):351–385. https://doi.org/10.1007/s11069-011-9920-7
Hollenstein K (2005) Reconsidering the risk assessment concept: standardizing the impact description as a building block for vulnerability assessment. Nat Hazards Earth Syst Sci 5:301–307. https://doi.org/10.5194/nhess-5-301-2005
IDEAM-UNAL—Institute of Hydrology, Meteorology and Environmental Studies & Universidad Nacional de Colombia (2018) Variabilidad Climática y Cambio Climático en Colombia. Bogotá
Jaiswal P, van Westen CJ, Jetten V (2011) Quantitative assessment of landslide hazard along transportation lines using historical records. Landslides 8:279–291. https://doi.org/10.1007/s10346-011-0252-1
Kühnl M, Sapena M, Taubenböck H (2021) Categorizing urban structural types using an object-based image analysis and the local climate zone classification scheme in Medellín, Colombia. Proceedings of the Real CORP, Real CORP 2021, 07.–10 Sept 2021, Wien, Österreich
Kurniawan A, Krol (Bart) BGCM (2014) Spatio temporal analysis of land use change for supporting landslide exposure assessment. Indonesian J Geogr 46(2):104–124. https://doi.org/10.22146/ijg.5781
McFeeters SK (1996) The use of the Normalized Difference Water Index (NDWI) in the delineation of open water features. Int J Remote Sens 17(7):1425–1432. https://doi.org/10.1080/01431169608948714
Mendes RM, de Andrade MRM, Tomasella J, de Moraes MAE, Scofield GB (2018) Understanding shallow landslides in Campos do Jordão municipality—Brazil: disentangling the anthropic effects from natural causes in the disaster of 2000. Nat Hazards Earth Syst Sci 18:15–30. https://doi.org/10.5194/nhess-18-15-2018
Müller I, Taubenböck H, Kuffer M, Wurm M (2020) Misperceptions of predominant slum locations? Spatial analysis of slum locations in terms of topography based on earth observation Data. Remote Sens 12(2474):1–19. https://doi.org/10.3390/rs12152474
Nor Diana MI, Muhamad N, Taha MR, Osman A, Alam MM (2021) Social vulnerability assessment for landslide hazards in Malaysia: a systematic review study. Land 10(3):315. https://doi.org/10.3390/land10030315
Novellino A, Cesarano M, Cappelletti P, Di MD, Di NM, Ramondini M, Sowter A, Calcaterra D (2021) Slow-moving landslide risk assessment combining machine learning and InSAR techniques. CATENA 203:105317. https://doi.org/10.1016/j.catena.2021.105317
Papathoma-Köhle M, Neuhäuser B, Ratzinger K, Wenzel H, Dominey-Howes D (2007) Elements at risk as a framework for assessing the vulnerability of communities to landslides. Nat Hazards Earth Syst Sci 7:765–779. https://doi.org/10.5194/nhess-7-765-2007
Pellicani R, Van Westen CJ, Spilotro G (2013) Assessing landslide exposure in areas with limited landslide information. Landslides 11(3):463–480. https://doi.org/10.1007/s10346-013-0386-4
POT—Plan de Ordenamiento Territorial: GDP POT Acuerdo48 de 2014. Medellín. https://geomedellin-m-medellin.opendata.arcgis.com/datasets/gdb-pot-acuerdo48-de-2014. Accessed 11 Mar 2021
Promper C, Gassner Ch, Glade T (2015) Spatiotemporal patterns of landslide exposure - a step within landslide risk analysis on a regional scale applied in Waidhofen/Ybbs Austria. Int J Disaster Risk Reduct 12:25–33. https://doi.org/10.1016/j.ijdrr.2014.11.003
Puissant A, Van den Eeckhaut M, Malet J-P, Maquaire O (2014) Landslide consequence analysis: a region-scale indicator-based methodology. Landslides 11:843–858. https://doi.org/10.1007/s10346-013-0429-x
Rahman MS, Di L (2017) The state of the art of spaceborne remote sensing in flood management. Nat Hazards 85:1223–1248. https://doi.org/10.1007/s11069-016-2601-9
Reed FJ, Gaughan AE, Stevens FR, Yetman G, Sorichetta A, Tatem AJ (2018) Gridded population maps informed by different built settlement products. Data 3(33):1–11. https://doi.org/10.3390/data3030033
Reichenbach P, Busca C, Mondini AC, Rossi M (2014) The influence of land use change on landslide susceptibility zonation: the Briga Catchment Test Site (Messina, Italy). Environ Manag 54:1372–1384. https://doi.org/10.1007/s00267-014-0357-0
Rouse Jr JW, Haas RH, Schell JA, Deering DW (1973) Monitoring the vernal advancement and retrogradation (green wave effect) of natural vegetation. NASA/Goddard Space Flight Center Type II Report for Period September 1972–March 1973, Greenbelt, Maryland
Sapena M, Kühnl M, Wurm M, Patino JE, Duque JC, Taubenböck H (2022) Empiric recommendations for population disaggregation under different data scenarios. PLoS ONE 17(9):e0274504. https://doi.org/10.1371/journal.pone.0274504
Singh A, Kanungo DP, Pal S (2019) Physical vulnerability assessment of buildings exposed to landslides in India. Nat Hazards 96:753–790. https://doi.org/10.1007/s11069-018-03568-y
Skrzypczak I, Kokoszka W, Zientek D, Tang Y, Kogut J (2021) Landslide hazard assessment map as an element supporting spatial planning: the Flysch Carpathians region study. Remote Sens 13(2):317. https://doi.org/10.3390/rs13020317
Soille P (2004) Morphological image analysis, principles and applications. Springer, Berlin
Stark T, Wurm M, Zhu XX, Taubenböck H (2020) Satellite-based mapping of urban poverty with transfer learned slum morphologies. IEEE J Sel Top Appl Earth Observ Remote Sens 13:5251–5263. https://doi.org/10.1109/JSTARS.2020.3018862
Stevens FR, Gaughan AE, Linard C, Tatem AJ (2015) Disaggregating census data for population mapping using random forests with remotely-sensed and ancillary data. PLoS ONE 10(2):e0107042. https://doi.org/10.1371/journal.pone.0107042
Stewart ID, Oke TR (2012) Local climate zones for urban temperature studies. Bull Am Meteor Soc 93(12):1879–1900. https://doi.org/10.1175/BAMS-D-11-00019.1
Taubenböck H, Wurm M, Netzband M, Zwenzner H, Roth A, Rahman A, Dech S (2011) Flood risk in urbanized areas—multi-sensoral approaches using remotely sensed data for risk assessment. Nat Hazards Earth Syst Sci 11:431–444. https://doi.org/10.5194/nhess-11-431-2011
Taubenböck H, Esch T, Felbier A, Wiesner M, Roth A, Dech S (2012) Monitoring of mega cities from space. Remote Sens Environ 117:162–176. https://doi.org/10.1016/j.rse.2011.09.015
UN, United Nations (2015) Habitat III Issue Papers. 22—Informal Settlements. Conference on Housing and Sustainable Urban Development. New York
UNESCO (1973) Annual Summary of Information on Natural Disasters. Unesco, Paris
UN-Habitat (2016) Urbanization and development: emerging futures. World Cities Report 2016. Nairobi, Kenya
UNISDR—The United Nations Office for Disaster Risk Reduction (2015) Sendai Framework for Disaster Risk Reduction 2015–2030. UNISDR, Geneva
URBAM—Centro de Estudios Urbanos y Ambientales—Universidad EAFIT, Social Agency Lab—Harvard Graduate School of Design (2012) Shifting ground. Precarious Settlements and Geological Hazard in Medellín
Van Westen CJ, Van Asch TWJ, Soeters R (2006) Landslide hazard and risk zonation—why is it still so difficult? Bull Eng Geol Env 65(2):167–184. https://doi.org/10.1007/s10064-005-0023-0
Vega JA, Hidalgo CA (2016) Quantitative risk assessment of landslides triggered by earthquakes and rainfall based on direct costs of urban buildings. Geomorphology 273:217–235. https://doi.org/10.1016/j.geomorph.2016.07.032
Vranken L, Vantilt G, Van den Eeckhaut M, Vandekerckhove L, Poesen J (2015) Landslide risk assessment in a densely populated hilly area. Landslides 12(4):787–798. https://doi.org/10.1007/s10346-014-0506-9
Wijaya AP, Hong J-H (2018) Quantitative assessment of social vulnerability for landslide disaster risk reduction using GIS approach (case study: Cilacap Regency, Province of Central Java, Indonesia). Int Arch Photogramm Remote Sens Spatial Inf Sci XLII–4:703–709. https://doi.org/10.5194/isprs-archives-XLII-4-703-2018
Wisner B, Blaikie P, Cannon T, Davis I (2003) At risk: natural hazards, people’s vulnerability and disasters, 2nd edn. Routledge, New York
World Bank Group (2017) Unbreakable. Building the resilience of the poor in the face of natural disasters. Climate Change and Development Series, Washington, DC
Wright JK (1936) A method of mapping densities of population: with cape cod as an example. Geogr Rev 26(1):103–110. https://doi.org/10.2307/209467
Wu S-S, Qiu X, Wang L (2005) Population estimation methods in GIS and remote sensing: a review. Gisci Remote Sens 42(1):58–74. https://doi.org/10.2747/1548-1603.42.1.80
Wurm M, Taubenböck H (2018) Detecting social groups from space—remote sensing-based mapping of morphological slums and assessment with income data. Remote Sens Lett 9(1):41–50. https://doi.org/10.1080/2150704X.2017.1384586
Wurm M, Taubenböck H, Weigand M, Schmitt A (2017) Slum mapping in polarimetric SAR data using spatial features. Remote Sens Environ 194:190–204. https://doi.org/10.1016/j.rse.2017.03.030
Wurm M, Stark T, Zhu XX, Weigand M, Taubenböck H (2019) Semantic segmentation of slums in satellite images using transfer learning on fully convolutional neural networks. ISPRS J Photogramm Remote Sens 150:59–60. https://doi.org/10.1016/j.isprsjprs.2019.02.006
Zha Y, Gao J, Ni S (2003) Use of normalized difference built-up index in automatically mapping urban areas from TM imagery. Int J Remote Sens 24(3):583–594. https://doi.org/10.1080/01431160304987
Zhong C, Liu Y, Gao P, Chen W, Li H, Hou Y, Nuremanguli T, Ma H (2020) Landslide mapping with remote sensing: challenges and opportunities. Int J Remote Sens 41(4):1555–1581. https://doi.org/10.1080/01431161.2019.1672904
Acknowledgements
This research was funded by the German Federal Ministry of Education and Research as part of the FONA Client II initiative, grant number 03G0883A-F, Inform@Risk—Strengthening the Resilience of Informal Settlements against Slope Movements. German partners: Leibniz Universität Hannover (Coordinator), Technische Hochschule Deggendorf, Technische Universität München, Deutsches Zentrum für Luft-und Raumfahrt e.V., AlpGeorisk, Sachverständigenbüro für Luftbildauswertung und Umweltfragen; Colombian partners: EAFIT University—URBAM, Alcaldía de Medellín, Área Metropolitana del Valle de Aburrá, Sistema de Alerta Temprana del Valle de Aburrá, Sociedad Colombiana de Geología, Colectivo Tejearañas, Corporación Convivamos, Fundación Palomá, Red Barrial Bello Oriente.
Funding
Open Access funding enabled and organized by Projekt DEAL. Marlene Kühnl, Marta Sapena, Michael Wurm, Christian Geiß, and Hannes Taubenböck have received funding from the German Federal Ministry of Education and Research as part of the FONA Client II initiative, Grant Number 03G0883A-F.
Author information
Authors and Affiliations
Contributions
All authors contributed to the study conception and design. Material preparation, data collection, and analysis were performed by MK and MS. The first draft of the manuscript was written by MK and all authors commented on previous versions of the manuscript. All authors read and approved the final manuscript.
Corresponding author
Ethics declarations
Conflict of interests
The authors have no relevant financial or non-financial interests to disclose.
Additional information
Publisher's Note
Springer Nature remains neutral with regard to jurisdictional claims in published maps and institutional affiliations.
Appendix
Appendix
See Fig. 6
Population estimation 1994 (a) and 2006 (b) per pixel in the extent of the respective urban settlement mask through disaggregation and extrapolation based on the population projection data 1994 and 2006. Since the estimates are based on official data, their administrative boundaries are still visible to a certain extent
Rights and permissions
Open Access This article is licensed under a Creative Commons Attribution 4.0 International License, which permits use, sharing, adaptation, distribution and reproduction in any medium or format, as long as you give appropriate credit to the original author(s) and the source, provide a link to the Creative Commons licence, and indicate if changes were made. The images or other third party material in this article are included in the article's Creative Commons licence, unless indicated otherwise in a credit line to the material. If material is not included in the article's Creative Commons licence and your intended use is not permitted by statutory regulation or exceeds the permitted use, you will need to obtain permission directly from the copyright holder. To view a copy of this licence, visit http://creativecommons.org/licenses/by/4.0/.
About this article
Cite this article
Kühnl, M., Sapena, M., Wurm, M. et al. Multitemporal landslide exposure and vulnerability assessment in Medellín, Colombia. Nat Hazards 119, 883–906 (2023). https://doi.org/10.1007/s11069-022-05679-z
Received:
Accepted:
Published:
Issue Date:
DOI: https://doi.org/10.1007/s11069-022-05679-z