Abstract
Context
Regional conservation efforts should incorporate fine scale landscape genetic and habitat suitability data for management decisions. This information permits conservation measures to be tailored to a specific landscape.
Objectives
We investigated the landscape determinants of gene flow and habitat suitability for the state-threatened Blanding’s turtle (Emydoidea blandingii) in northeastern New York (NNY). We applied the results from each to examine their complementary contributions to local connectivity and genetic structuring.
Methods
We conducted population and individual-based genetic analyses with microsatellite data to evaluate genetic structuring and landscape genetics in NNY. We coupled these genetic analyses with species distribution modeling (SDM) to estimate the extent of suitable habitat across this important region for species persistence in the state.
Results
Gene flow was strongly associated with open water and cultivated land, indicating the role open water channels play in connecting neighboring activity centers, and the propensity of females to select cultivated land to nest. Species distribution models based on Landsat-derived vegetation indices and percentage of scrub-shrub wetlands accurately identified Blanding’s turtle habitat. Connectivity estimates from our NNY focal area using landscape genetic and SDM resistance surfaces showed potential movement constraints between the two genetic clusters.
Conclusions
Land cover better explained genetic distance data than geographic distance for Blanding’s turtles in our focal area. Accurate SDMs were developed for our focal area with a small number of occurrences (< 50). Using both gene flow and habitat-informed resistance surfaces revealed localized connectivity constraints associated with each, permitting more comprehensive landscape planning.




Similar content being viewed by others
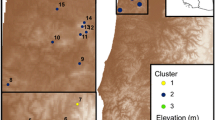
References
Andrews KM, Langen TA, Struijk R (2015) Reptiles: overlooked but often at risk from roads. In: Van der Ree R, Smith DJ (eds) Handbook of road ecology. Wiley, New York, pp 271–280
Barker R, King DJ (2012) Blanding’s Turtle (Emydoidea blandingii) potential habitat mapping using aerial orthophotographic imagery and object based classification. Remote Sens 4(1):194–219
Beaudry F, deMaynadier PG, Hunter ML (2008) Identifying road mortality threat at multiple spatial scales for semi-aquatic turtles. Biol Cons 141:2550–2563
Beaudry F, deMaynadier PG, Hunter ML (2009) Seasonally dynamic habitat use by Spotted (Clemmys guttata) and Blanding’s Turtles (Emydoidea blandingii) in Maine. J Herpetol 43:636–645
Benson CE, Carberry B, Langen TA (2018) Public–private partner-ship wetland restoration programs benefit Species of Greatest Conservation Need and other wetland-associated wildlife. Wetlands Ecol Manage 26:195–211
Bradley BA, Olsson AD, Wang O, Dickson BG, Pelech L, Sesnie SE et al (2012) Species detection vs. habitat suitability: are we biasing habitat suitability models with remotely sensed data? Ecol Model 244:57–64
Breiner FT, Guisan A, Bergamini A, Nobis MP (2015) Overcoming limitations of modelling rare species by using ensembles of small models. Methods Ecol Evol 6:1210–1218
Breiner FT, Nobis MP, Bergamini A, Guisan A (2018) Optimizing ensembles of small models for predicting the distribution of species with few occurrences. Methods Ecol Evol 9:802–808
Clarke RT, Rothery P, Raybould AF (2002) Confidence limits for regression relationships between distance matrices: estimating gene flow with distance. JABES 7:361
Cola VD, Broennimann O, Petitpierre B et al (2017) ecospat: an R package to support spatial analyses and modeling of species niches and distributions. Ecography 40:774–787
Congdon JD, Kinney OM, Nagle RD (2011) Spatial ecology and core-area protection of Blanding’s Turtle (Emydoidea blandingii). Can J Zool 89(11):1098–1106
Crockett, TJ (2008) Home range, movements, and habitat use of Blanding’s turtle (Emydoidea blandingii) in St. Lawrence County, New York. M.S. Thesis. SUNY Brockport, Brockport, NY. 86pp
Davy CM, Bernardo PH, Murphy RW (2014) A Bayesian approach to conservation genetics of Blanding’s turtle (Emys blandingii) in Ontario Canada. Conserv Genet 15(2):319–330
Dieringer D, Schlötterer C (2003) Microsatellite analyser (MSA): a platform independent analysis tool for large microsatellite data sets. Mol Ecol Notes 3:167–169
Duflot R, Avon C, Roche P, Bergès L (2018) Combining habitat suitability models and spatial graphs for more effective landscape conservation planning: An applied methodological framework and a species case study. J Nat Conserv 46:38–47
Dymond CC, Mladenoff DJ, Radeloff VC (2002) Phenological differences in Tasseled Cap indices improve deciduous forest classification. Remote Sens Environ 80:460–472
Edge CB, Steinberg BD, Brooks RJ, Litzgus JD (2015) Habitat selection by Blanding’s turtles (Emydoidea blandingii) in a relatively pristine landscape. Écoscience 17(1):90–99
Eisenstadt P, Moss LE (eds) (2005) The encyclopedia of New York State. Syracuse University Press, Syracuse
Evanno G, Regnaut S, Goudet J (2005) Detecting the number of clusters of individuals using the software STRUCTURE: a simulation study. Mol Ecol 14(8):2611–2620
Excoffier L, Lischer HEL (2010) Arlequin suite ver 3.5: a new series of programs to perform population genetics analyses under Linux and Windows. Mol Ecol Resour 10(3):564–567
Gallego-Garcia N, Vargas-Ramirez M, Forero-Medina G, Caballero S (2018) Genetic evidence of fragmented populations and inbreeding in the Colombian endemic Dahl’s toad-headed turtle (Mesoclemmys dahli). Conserv Genet 19(1):221–233
Gibbs JP, Steen DA (2005) Trends in sex ratios of turtles in the United States: implications of road mortality. Conserv Biol 19:552–556
Gilbert JM, Vidler N, Cloud Sr P, Jacobs D, Slavik E, Letourneau F, Alexander K. (2014) Phragmites australis at the crossroads: why we cannot afford to ignore this invasion. In: Proceedings of Great Lakes Wetlands Day. The Great Lakes Wetlands Conservation Action Plan, Toronto. pp 78–84
Grgurovic M, Sievert PR (2005) Movement patterns of Blanding’s turtles (Emydoidea blandingii) in the suburban landscape of eastern Massachusetts. Urban Ecosyst 8(2):203–213
Guillot G, Mortier F, Estoup A (2005) Geneland: a computer package for landscape genetics. Mol Ecol Notes 5:712–715
Guisan A, Tingley R, Baumgartner JB, Naujokaitis-Lewis I, Sutcliffe PR, Tulloch AIT et al (2013) Predicting species distributions for conservation decisions. Ecol Lett 16:1424–1435
Guzy JC, Price SJ, Dorcas ME (2013) The spatial configuration of greenspace affects semi-aquatic turtle occupancy and species richness in a suburban landscape. Landsc Urban Plan 117:46–56
Hamilton CM, Bateman BL, Gorzo JM et al (2018) Slow and steady wins the race? Future climate and land use change leaves the imperiled Blanding’s turtle (Emydoidea blandingii) behind. Biol Cons 222:75–85
Harrisson K, Pavlova A, Amos JN, Takeuchi N, Lill A, Radford JQ, Sunnucks P (2012) Fine-scale effects of habitat loss and fragmentation despite large-scale gene flow for some regionally declining woodland bird species. Landsc Ecol 27:813–827
Hart JP, Lovis WA (2013) Reevaluating what we know about the histories of maize in Northeastern North America: a review of current evidence. J Archaeol Res 21:175–216
Hirzel AH, Le Lay G, Helfer V et al (2006) Evaluating the ability of habitat suitability models to predict species presences. Ecol Model 199:142–152
Keeley ATH, Beier P, Keeley BW, Fagan ME (2017) Habitat suitability is a poor proxy for landscape connectivity during dispersal and mating movements. Landsc Urban Plan 161:90–102
Khimoun A, Peterman W, Eraud C, Faivre B, Navarro N, Garnier S (2017) Landscape genetic analyses reveal fine-scale effects of forest fragmentation in an insular tropical bird. Mol Ecol 26:4906–4919
Koen EL, Bowman J, Walpole AA (2012) The effect of cost surface parameterization on landscape resistance estimates. Mol Ecol Resour 12:686–696
Kopelman NM, Mayzel J, Jakobsson M, Rosenberg NA, Mayrose I (2015) Clumpak : a program for identifying clustering modes and packaging population structure inferences across K. Mol Ecol Resour 15(5):1179–1191
Kua ZX, Stella JC, Farrell JM (2020) Local disturbance by muskrat, an ecosystem engineer, enhances plant diversity in regionally-altered wetlands. Ecosphere 11(10):e03256
Landguth EL, Cushman SA, Schwartz MK, McKelvey KS, Murphy M, Luikart G (2010) Quantifying the lag time to detect barriers in landscape genetics. Mol Ecol 19:4179–4191
Li Y-L, Liu J-X (2018) StructureSelector: a web-based software to select and visualize the optimal number of clusters using multiple methods. Mol Ecol Resour 18:176–177
Liu C, White M, Newell G (2013) Selecting thresholds for the prediction of species occurrence with presence-only data. J Biogeogr 40:778–789
Mahdavi S, Salehi B, Granger J, Amani M, Brisco B, Huang W (2017) Remote sensing for wetland classification: a comprehensive review. GISCI Remote Sens. https://doi.org/10.1080/15481603.2017.1419602
Manel S, Holderegger R (2013) Ten years of landscape genetics. Trends Ecol Evol 28:614–621
Markle CE, Chow-Fraser P (2016) An integrative approach to regional mapping of suitable habitat for the Blanding’s turtle (Emydoidea blandingii) on islands in Georgian Bay, Lake Huron. Global Ecol Conserv 6:219–231
Markle CE, Chow-Fraser P (2018) Effects of European common reed on Blanding’s turtle spatial ecology. J Wildlife Manage 82(4):857–864
Marsack K, Swanson BJ (2009) A genetic analysis of the impact of generation time and road-based habitat fragmentation on Eastern Box Turtles (Terrapene c. carolina). Copeia 2009:647–652
Mateo-Sánchez MC, Balkenhol N, Cushman S, Pérez T, Domínguez A, Saura S (2015) Estimating effective landscape distances and movement corridors: comparison of habitat and genetic data. Ecosphere 6:59
McCluskey EM, Matthews SN, Ligocki IY, Holding ML, Lipps GJ, Hetherington TE (2018) The importance of historical land use in the maintenance of early successional habitat for a threatened rattlesnake. Global Ecol Conserv 13:145
McCluskey EM, Mockford SW, Sands K, Herman TB, Johnson G, Gonser RA (2016) Population genetic structure of Blanding’s turtles (Emydoidea blandingii) in New York. J Herpetol 50(1):70–76
McGarigal K, Cushman SA, Ene E (2012) FRAGSTATS v4: Spatial pattern analysis program for categorical and continuous maps. Computer software program produced by the authors at the University of Massachusetts, Amherst. Available at: http://www.umass.edu/landeco/research/fragstats/fragstats.html
McGuire JM, Scribner KT, Congdon JD (2013) Spatial aspects of movements, mating patterns, and nest distributions influence gene flow among population subunits of Blanding’s turtles (Emydoidea blandingii). Conserv Genet 14(5):1029–1042
McRae BH (2006) Isolation by resistance. Evolution 60(8):1551–1561
McRae BH (2012a) Barrier mapper connectivity analysis software. The Nature Conservancy, Seattle
McRae BH (2012b) Pinchpoint mapper connectivity analysis software. The Nature Conservancy, Seattle Washington. Available from http://www.circuitscape.org/linkagemapper.
McRae BH, Beier P (2007) Circuit theory predicts gene flow in plant and animal populations. Proc Natl Acad Sci USA 104(50):19885–19890
McRae BH, Kavanagh DM (2011) Linkage mapper connectivity analysis software. The Nature Conservancy, Seattle
Millar CS, Blouin-Demers G (2011) Spatial ecology and seasonal activity of Blanding’s turtles (Emydoidea blandingii) in Ontario Canada. J Herpetol 45(3):370–378
Millar CS, Blouin-Demers G (2012) Habitat suitability modelling for species at risk is sensitive to algorithm and scale: a case study of Blanding’s turtle, Emydoidea blandingii, in Ontario Canada. J Nat Conserv 20(1):18–29
Mockford SW, Herman TB, Snyder M, Wright JM (2007) Conservation genetics of Blanding’s turtle and its application in the identification of evolutionarily significant units. Conserv Genet 8(1):209–219
Mui AB, Caverhill B, Johnson B, Fortin M-J, He Y (2017) Using multiple metrics to estimate seasonal landscape connectivity for Blanding’s turtles (Emydoidea blandingii) in a fragmented landscape. Landsc Ecol 32(3):531–546
Mui AB, Edge CB, Paterson JE, Caverhill B, Johnson B, Litzgus JD, He Y (2015) Nesting sites in agricultural landscapes may reduce the reproductive success of populations of Blanding’s Turtles (Emydoidea blandingii). Can J Zool 94(1):61–67
Oyler-McCance SJ, Fedy BC, Landguth EL (2013) Sample design effects in landscape genetics. Conserv Genet 14:275–285
Peterman WE (2018) ResistanceGA: An R package for the optimization of resistance surfaces using genetic algorithms. Methods Ecol Evol 9(6):1638–1647
Peterman WE, Connette GM, Semlitsch RD, Eggert LS (2014) Ecological resistance surfaces predict fine-scale genetic differentiation in a terrestrial woodland salamander. Mol Ecol 23(10):2402–2413
Peterman WE, Crawford JA, Kuhns AR (2013) Using species distribution and occupancy modeling to guide survey efforts and assess species status. J Nat Conserv 21:114–121
Phillips SJ, Anderson RP, Schapire RE (2006) Maximum entropy modeling of species geographic distributions. Ecol Model 190(3–4):231–259
Phillips SJ, Dudík M (2008) Modeling of species distributions with Maxent: new extensions and a comprehensive evaluation. Ecography 31(2):161–175
Piepgras SA, Lang JW (2000) Spatial ecology of Blanding’s turtle in central Minnesota. Chelonian Conserv Biol 3:589–601
Pimm SL, Dollar L, Bass OL (2006) The genetic rescue of the Florida panther. Anim Conserv 9(2):115–122
Pritchard JK, Stephens M, Donnelly P (2000) Inference of population structure using multilocus genotype data. Genetics 155(2):945–959
Proulx CL, Fortin G, Blouin-Demers G (2013) Blanding’s turtles (Emydoidea blandingii) avoid crossing unpaved and paved roads. J Herpetol 48:267–271
Puechmaille SJ (2016) The program structure does not reliably recover the correct population structure when sampling is uneven: subsampling and new estimators alleviate the problem. Mol Ecol Resour 16:608–627
Radford HV (1907) History of the Adirondack beaver. New York State Forest, Fish and Game Commission Annual Report for 1904, 1905, 1906. 389–418.
Reddoch JM, Reddoch AH (2005) Consequences of beaver, Castor canadensis, flooding on a small shore fen in Southwestern Quebec. Can Field-Nat 119:385–394
Reid BN, Peery MZ (2014) Land use patterns skew sex ratios, decrease genetic diversity and trump the effects of recent climate change in an endangered turtle. Divers Distrib 20(12):1425–1437
Reid BN, Thiel RP, Palsboll PJ, Peery MZ (2016) Linking genetic kinship and demographic analyses to characterize dispersal: methods and application to Blanding’s turtle. J Hered 107(7):603–614
Rhoden CM, Peterman WE, Taylor CA (2017) Maxent-directed field surveys identify new populations of narrowly endemic habitat specialists. PeerJ. https://doi.org/10.7717/peerj.3632
Ross AM, Johnson G (2018) Conservation plan for populations of the Blanding’s turtle (Emydoidea blandingii). New York State Department of Environmental Conservation, Albany
Ross DA, Anderson RK (1990) Habitat use, movements, and nesting of Emydoidea blandingii in Central Wisconsin. J Herpetol 24:6–12
Rowe JW, Moll EO (1991) A radiotelemetric study of activity and movements of the Blanding’s turtle (Emydoidea blandingii) in northeastern Illinois. J Herpetol 25:178–185
Ruiz-Lopez MJ, Barelli C, Rovero F, Hodges K, Roos C, Peterman WE et al (2016) A novel landscape genetic approach demonstrates the effects of human disturbance on the Udzungwa red colobus monkey (Procolobus gordonorum). Heredity 116(2):167–176
Sanderson EW, Redford KH, Chetkiewicz CLB, Medellin RA, Rabinowitz RA, Robinson JG, Taber AB (2002) Planning to save a species: the jaguar as a model. Conserv Biol 16(1):58–72
Segelbacher G, Cushman SA, Epperson BK, Fortin M-J, Francois O, Hardy OJ, Holderegger R, Manel S (2010) Applications of landscape genetics in conservation biology: concepts and challenges. Conserv Genet 11(2):375–385
Sethuraman A, McGaugh SE, Becker ML, Chandler CH, Christiansen JL, Hayden S et al (2014) Population genetics of Blanding’s turtle (Emys blandingii) in the midwestern United States. Conserv Genet 15(1):61–73
Steen DA, Barrett K (2015) Should states in the USA value species at the edge of their geographic range? J Wildlife Manage 79(6):872–876
Steen DA, Gibbs JP (2004) Effects of roads on the structure of freshwater turtle populations. Conserv Biol 18:1143–1148
Stryszowska KM, Johnson G, Mendoza LR, Langen TA (2016) Species distribution modeling of the threatened Blanding’s turtle’s (Emydoidea blandingii) range edge as a tool for conservation planning. J Herpetol 50(3):366–373
Thompson JR, Carpenter DN, Cogbill CV, Foster DR (2013) Four centuries of change in northeastern United States forests. PLoS ONE 8:72540
Thuiller W, Lafourcade B, Engler R, Araújo MB (2009) BIOMOD: a platform for ensemble forecasting of species distributions. Ecography 32:369–373
Travis KB, Haeckel I, Stevens G, Tesauro J, Kiviat E (2018) Bog Turtle (Glyptemys muhlenbergii) dispersal corridors and conservation in New York, USA. Herpetol Conserv Bio 13:257–272
van Etten J (2014) gdistance: distances and routes on geographical grids. R package version 1.1–5. http://CRAN.R-project.org/package=gdistance
Wang IJ, Savage WK, Shaffer HB (2009) Landscape genetics and least-cost path analysis reveal unexpected dispersal routes in the California tiger salamander (Ambystoma californiense). Mol Ecol 18:1365–1374
Winiarski KJ, Peterman WE, McGarigal K (2020) Evaluation of the R package ‘resistancega’: a promising approach towards the accurate optimization of landscape resistance surfaces. Mol Ecol Resour 20:1583–1596
Acknowledgements
We thank T. Crockett and the many field technicians for their contributions to the northeastern New York Blanding’s turtle monitoring project. We also thank Jamie Kass for his helpful suggestions related to the SDM. Support for WEP was provided by the USDA National Institute of Food and Agriculture, Hatch Project 1020979.
Funding
The authors have not disclosed any funding.
Author information
Authors and Affiliations
Corresponding author
Ethics declarations
Conflict of interest
The authors have not disclosed any competing interests.
Additional information
Publisher's Note
Springer Nature remains neutral with regard to jurisdictional claims in published maps and institutional affiliations.
Supplementary Information
Below is the link to the electronic supplementary material.
Rights and permissions
About this article
Cite this article
McCluskey, E.M., Lulla, V., Peterman, W.E. et al. Linking genetic structure, landscape genetics, and species distribution modeling for regional conservation of a threatened freshwater turtle. Landsc Ecol 37, 1017–1034 (2022). https://doi.org/10.1007/s10980-022-01420-0
Received:
Accepted:
Published:
Issue Date:
DOI: https://doi.org/10.1007/s10980-022-01420-0